- 1Laboratory of Genetics, Biotechnology and Seed Sciences (GBioS), Faculty of Agronomic Sciences (FSA), University of Abomey-Calavi (UAC), Cotonou, Benin
- 2Department of Rainfed Crop Production (DCP), National Institute of Agronomic Research of Niger (INRAN), Niamey, Niger
- 3Department of Crop Production & Landscape Management, Faculty of Agriculture & Natural Resources Management, Ebonyi State University, Abakaliki, Nigeria
- 4Centre d'Étude Régional pour l'Amélioration de l'Adaptation à la Sécheresse (CERAAS), Institut Sénégalais de Recherches Agricoles (ISRA), Thiès, Senegal
- 5UMR AGAP Institut, Univ Montpellier, CIRAD, INRAE, Institut Agro, Montpellier, France
White fonio (Digitaria exilis) is a staple food for millions of people in arid and semi-arid areas of West Africa. Knowledge about nutritional and health benefits, insights into morphological diversity, and the recent development of genomic resources call for a better understanding of the genetic structure of the extant germplasm gathered throughout the region in order to set up a robust breeding program. We assessed the genetic diversity and population structure of 259 fonio individuals collected from six countries from West Africa (Nigeria, Benin, Guinea, Mali, Burkina Faso and Niger) in this study using 688 putative out of 21,324 DArTseq-derived SNP markers. Due to the inbreeding and small population size, the results revealed a substantial level of genetic variability. Furthermore, two clusters were found irrespective of the geographic origins of accessions. Moreover, the high level of linkage disequilibrium (LD) between loci observed resulted from the mating system of the crop, which is often associated with a low recombination rate. These findings fill the gaps about the molecular diversity and genetic structure of the white fonio germplasm in West Africa. This was required for the application of genomic tools that can potentially speed up the genetic gain in fonio millet breeding for complex traits such as yield, and other nutrient contents.
Introduction
Plant breeders often use the diversity of plant genetic resources to create new and improved cultivars with desirable features such as production potential, nutritional quality, large seed size, and resistance to biotic and abiotic stresses (Govindaraj et al., 2015). Modern crop varieties are typically inappropriate for low-input agriculture in marginal environments, despite the fact that they were created largely for high yielding potential under well-defined conditions (Evenson and Gollin, 2003), compared to traditional varieties or landraces that are well-adapted to various farming systems under different ecological regions, thus contributing to food and nutrition security of subsistent small-scale farmers (Ceccarelli and Grando, 2002). Among traditional cereals, fonio is one of West Africa's oldest native cereal crops, with social, economic, and nutritional significance (Dansi et al., 2010). Fonio grain is used to make salads, couscous, stews, candies, and alcoholic and non-alcoholic beverages, as well as traditional porridges, fonio Jollof, and flour creams (Abdul and Jideani, 2019). Furthermore, the ecological versatility of fonio, makes the crop an important component to be considered for tackling issues related to food insecurity and malnutrition in the future (Raneri et al., 2019). However, in spite of its inherent advantages in playing a critical role for food and nutrition security, and income generation, no improved varieties have been developed so far. With the current advances in molecular breeding, additional efforts are therefore needed to bridge the gaps and generate knowledge that can contribute significantly to increase precision and accuracy in fonio improvement using molecular data.
During the last decades, the efficiency and precision of conventional breeding have been remarkedly improved through the application of molecular tools in both basic and applied research (e.g., diversity studies, phylogenetic and evolutionary analyses, linkage QTL mapping, association mapping studies, marker-assisted selection, genomic-assisted selection) (Gross-German and Viruel, 2013). Various molecular markers have been reported earlier in fonio millet (Digitaria exilis [Kippist] Stapf) for diversity, phylogenetic and evolutionary studies. These markers included isozyme (Adoukonou-Sagbadja et al., 2010), Random Amplified Polymorphic DNA (RAPD) (Hilu et al., 1997; Kuta et al., 2005), Inter-Simple Sequence Repeat (ISSR) (Animasaun et al., 2018), and Simple Sequence Repeat (SSR) (Barnaud et al., 2012; Ngom et al., 2018; Olodo et al., 2019). Amplified Fragment Length Polymorphism (AFLP) markers were found to be effective in detecting genetic diversity and determining population differentiation, and moderate levels of genetic diversity were detected in Digitaria exilis (Adoukonou-Sagbadja et al., 2007). Furthermore, SSR markers were frequently used for diversity, phylogenetic, and evolutionary investigations (Ellegren, 2002) due to their co-dominance, high polymorphism, high global mutation rates, and repeatability, even if designing primers requires a thorough understanding of DNA sequences (Xu and Crouch, 2008; Mondini et al., 2009). With the recent advances in genotyping by sequencing (GBS) technologies, single nucleotide polymorphisms (SNPs) are becoming the markers of choice in crop genetic studies because of their bi-allelic nature, which allows for accurate variant calling, high reproducibility, and the fact that they provide a rapid, high-throughput, and cost-effective tool for exploring plant genetic diversity on a genome-wide scale. Ching et al. (2002), Chagné et al. (2008),and Studer and Kölliker (2013) without prior knowledge of the species of interest's genome. Most importantly, these markers enabled dissection of the genetic basis underlying complex quantitative traits through biparental linkage and mapping approaches (Sehgal et al., 2020) as well as comparative genomics (Abrouk et al., 2020; Wang et al., 2021). Unlike linkage mapping, the association mapping approach, also known as linkage disequilibrium (LD) mapping, makes use of the existing genetic diversity in natural germplasm populations and exploits their historic recombination events to map or fine map genes with greater precision (Buckler IV and Thornsberry, 2002). Thus, for a successful association mapping and genomic prediction, a better understanding of LD's patterns and decay within a population is very critical to figure out the number of markers needed (Porto-Neto et al., 2014). Khatkar et al. (2008) reported that when low LD levels exist in a population, to capture the genetic variation across the genome, a higher marker density is required. To date, the most commonly used measure of LD is the r2 being the squared value of the correlation coefficient between two polymorphic loci's allelic states (Pritchard and Przeworski, 2001). LD patterns based on SNP markers have been widely studied in different crop species including cereals (Chen et al., 2017; Serba et al., 2019) and legumes (Otyama et al., 2019; Sodedji et al., 2020). However, assessment of genetic diversity, population structure analysis, and Linkage Disequilibrium patterns of fonio millet at genome-wide scale were not performed. Therefore, to better characterize the diversity of the current collection of fonio at genomic level and understand the population structure at regional level, advanced high throughput genotyping platforms is needed. This is the first time, as far as we know that genome-wide diversity is explored on a regional basis in fonio millet including collections from Guinea, Mali, Niger, Benin, Nigeria and Burkina Faso. The paper intends to respond to the following questions: (a) What is the genetic basis of the population structure? (b) how are fonio individuals from various ecological regions related? (c) Does population differentiation link with geographical patterns? and What are the LD patterns? The aims of this research are to investigate the genetic diversity in fonio millet accessions, to determine the structure and level of population differentiation, and to measure LD. We assume that investigating the genetic diversity on a genome wide-scale using genotyping by sequencing analysis will reveal association that is useful in fonio molecular breeding.
Materials and Methods
Description of Plant Materials
The plant material included 259 fonio millet accessions collected across six West African countries (Benin, Burkina Faso, Guinea, Mali, Niger, and Nigeria) and kept at the genebank of the Laboratory of Genetics, Biotechnology and Seed Science (GBioS) of the University of Abomey-Calavi (UAC) in Benin. The diversity panel has also been collected from a range of ecological regions including arid, semi-arid and sub-humid. The most accessions in the collection were from semi-arid ecology (183), followed by arid (50) and sub-humid (26) ecologies. According to the country of provenance, 42 fonio accessions were collected from Benin, four from Burkina Faso, 29 from Guinea, 28 from Mali, 28 from Niger, and 128 from Nigeria. The distribution map of fonio accessions collected from six West African countries is presented below (Figure 1).
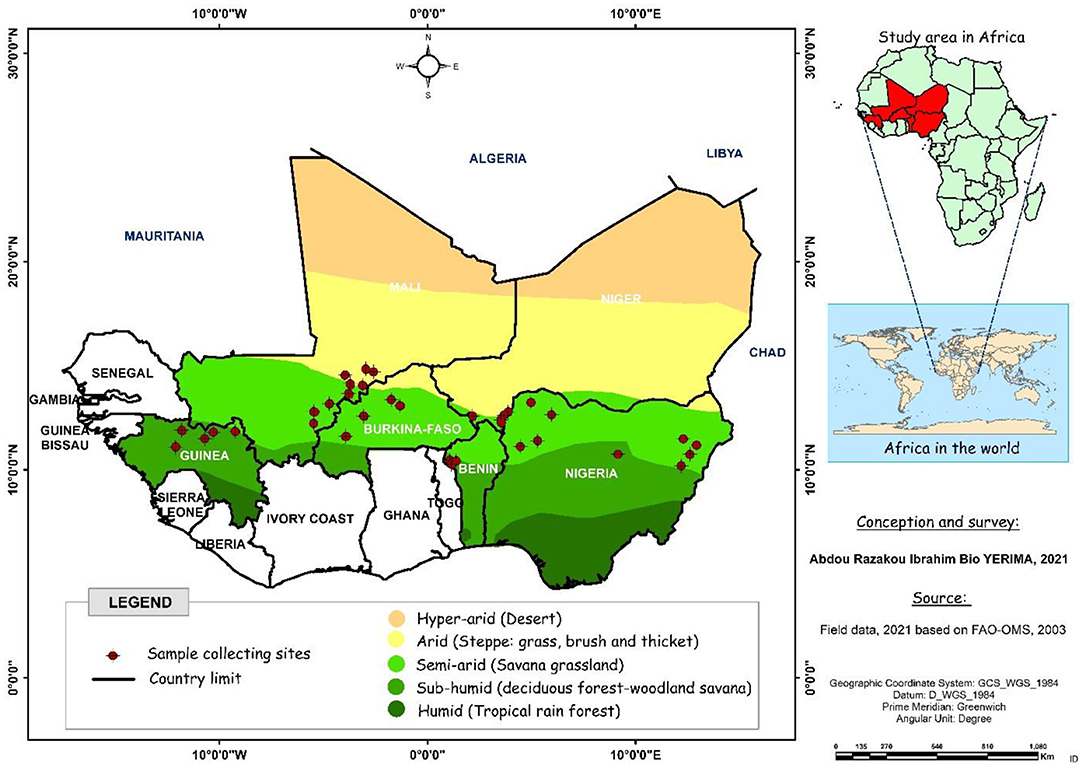
Figure 1. Distribution map of fonio accessions collected from six West African countries (Benin, Burkina Faso, Guinea, Mali, Niger and Nigeria).
DNA Extraction and Genotyping
Fonio plants were grown in plastic bags in the GBioS laboratory at the University of Abomey-Calavi (Benin) and three weeks old leaves were sampled from a single plant and collected into 96 deep well samples collection plates and sent to the Integrated Genotyping Service and Support (IGSS) platform (https://ordering.igssafrica.org/cgibin/order/login.pl) now SEQART AFRICA located at Biosciences Eastern and Central Africa (BecA-ILRI) Hub in Nairobi for Genotyping. The Nucleomag Plant Genomic DNA extracting kit was used to extract genomic DNA from 50–100 ng/ul, with the quality and quantity of the DNA extracted being checked on 0.8% agarose. DArT-Seq has been optimized for D. exilis by choosing the best method to reduce complexity (PstI-MseI restriction enzymes). The DNA samples were processed using Kilian et al. (2012) digestion and ligation reactions method, but they replace a single PstI-compatible adapter with two different adapters that match two different restriction enzymes. The Illumina flow cell attachment sequence, sequencing primer sequence, and staggered, varying length barcode have been built into PstI-compatible adapter while the reverse adapter contained the flow cell fitting region and the overhang sequence that was MseI-compatible. PCR was used to amplify only mixed fragments (Psti-MseI) following these reactions conditions: initial denaturation stage (1 min at 94°C); denaturation stage (30 rounds each consisting of 20 s at 94°C); annealing stage (30 s at 58°C); extension stage (45 s at 72°C); and finally, an extension step of 7 min at 72°C. Following PCR, equimolar amounts of amplification products were bulked and applied to c-Bot bridge PCR for each sample of the 96-well microtiter plate and sequenced on Illumina Hiseq2500. Single read sequencing took place over 77 cycles. Sequences produced by every lane were processed using proprietary DArT analytical pipelines. In order to filter poor-quality sequences, the FASTQ files were first processed in the primary pipeline with more stringent selection criteria compared to the remaining sequence in the barcode region. The sequences of specific samples contained in the barcode split-step were therefore assigned more consistently. The barcode/sample sequences were used in the marker calling with ~2,500,000 (±7%). At the end, the same sequences were collapsed into “fastqcall files.” and these files were used in the secondary pipeline for DArT P/L's proprietary SNP and SilicoDArT (present = 1 and absent = 0) calling algorithms (DArTsoft14). The sequence data were processed using analytical pipeline. SNP markers were aligned to the reference genome of Fonio CM05836 (D. exilis) (Abrouk et al., 2020) to identify chromosome positions.
Data Cleaning, Imputation and Filtering
TASSEL (Trait Analysis by aSSociation, Evolution and linkage) software version 5.2.56 (Bradbury et al., 2007) was used for data cleaning or quality control. Prior to the analysis of genetic parameters, tree filters were generated before missing data imputation, whereby loci with minor alleles frequency (MAF) <0.05 along with loci departing from Hardy–Weinberg equilibrium at 5% and loci with a call rate <70 % were pruned using the PCCA algorithm in KDCompute (https://kdcompute.igss-africa.org/kdcompute/login). The imputed and filtered data were used for structure and genetic relatedness analyses, the analysis of molecular variance (AMOVA), and as well as the discriminant analysis of principal components (DAPC).
Genetic Diversity Analysis
Parameters of genetic diversity such as observed and expected heterozygosities and inbreeding coefficient were estimated at different levels including the entire panel, ecological regions and as well as country of provenance using the package “adegenet” (Jombart, 2008) implemented in R software version 3.6.1 (Core Team, R, 2019). The analysis of molecular variance (AMOVA) was estimated using the package “poppr” (Kamvar et al., 2014) implemented also in R software.
Population Structure Analysis
In order to cluster fonio accessions onto different groups, population structure was investigated using DArTseq-derived SNP markers distributed across the fonio genome using the Bayesian clustering approach in STRUCTURE version 2.3.4 (Pritchard et al., 2000). The analysis included three ecological regions (e.g., arid, semi-arid and sub-humid zones) as putative phytogeographic origins of accessions. A burn-in period of 10,000 Markov Chain Monte Carlo (MCMC) iterations and 10,000-run length, with an admixture model following Hardy-Weinberg equilibrium and correlated allele frequencies were considered in the structure analysis (Evanno et al., 2005). For each value of K (number of subpopulations), 10 independent runs were performed ranging from 1 to 7. The Structure Harvester (Earl, 2012) was used to analyze the results from the Structure, which enabled the identification of the best K-value as the distinct peak in the change of likelihood (ΔK) (Evanno et al., 2005). The extent of genetic differentiation (Fst) between the three ecological regions was estimated with Structure harvester simulation results (Supplementary File 1). DArTseq SNP data were numerically coded as follows: A = 1, T = 2, C = 3, G = 4 as suggested in Structure V2.3.4 user manual.
Genetic Relatedness and Population Differentiation
A Neighbor-Joining (NJ) dendrogram was drawn using Darwin software V6.0.21 (Perrier and Jacquemoud-Collet, 2016), in order to investigate the genetic relatedness among accessions. Prior to the analysis, the 259 accessions were grouped according to their country of provenance to describe the distribution in the identified clusters. Furthermore, discriminant analysis of principal components (DAPC) was performed to infer and describe clusters in population of genetically related individuals using ≪ adegenet ≫ (Jombart, 2008) and ≪ hierfstat≫ (Goudet, 2005) packages implemented in R V.3.6.1 (Core Team, R, 2019). In DAPC, data were set first for principal components analysis (PCA) and subsequently clustered using discriminant analysis (DA) with respective eigenvalues. Moreover, in order to estimate genetic differentiation among fonio clusters, Weir and Cockerham's Fst-values (Weir and Cockerham, 1984) were calculated using R software.
Linkage Disequilibrium (LD)
Genome-wide LD patterns of known position of SNPs on the chromosomes (Huang et al., 2012) out of the complete set of 21,324 polymorphic markers were estimated using TASSEL (Trait Analysis by aSSociation, Evolution and Linkage) V5.3.1 software (Bradbury et al., 2007). LD patterns between markers was estimated using r2, which is the correlation between alleles at two loci, as well as an indicator for evaluating the resolution of association approaches. Farnir et al. (2000) stated that for all possible combination of alleles and then weighting them according to the allele's frequency, a weighted mean of r2 was calculated between the two loci. In order to test the LD's significance, we also obtained P-values determined by permutational test to calculate the ratio of permuted gamete distributions that was less likely than the gamete distribution observed under the null independence hypothesis (Weir, 1996). Furthermore, the rate of LD decay was drawn as the variation r2 and the physical genetic distance (Mb) between pairs of SNP markers using “dartR” (Gruber et al., 2018) and “Sommer” (Covarrubias-Pazaran, 2016) packages implemented in R.
Results
Genetic Diversity
A total of 21,324 codominant single nucleotide polymorphisms (SNPs) were obtained from the DArTseq analysis with 46.12% of missing data of the overall fonio accessions. The call rate ranged from 37.83 to 100% with a mean of 53.87% ± 0.080. These markers showed high reproducibility varying from 0.90 to 1.00 with an average of 0.99 ± 0.011. The minor allele frequency (MAF) ranged from 0.002 to 0.5 with a mean of 0.153 ± 0.08 while the major allele frequency (MaF) varied from 0.5 to 0.99 with an average of 0.84 ± 0.08 (Table 1). Furthermore, 17,821 markers (83.57%) had minor allele frequency >0.05 and the majority (83.83%) had a polymorphic information content (PIC) value >0.1 with a mean value of 0.25 ± 0.11. About 80% (17,045) of SNPs were aligned to the reference genome of fonio CM05836. These markers were almost uniformly distributed across the genome with the most SNPs observed on chromosome 09B (2,004 markers) followed by chromosome 05B (1,590 markers). The lowest numbers of SNP markers were observed on chromosomes 06A and 08A with 406 and 386 markers, respectively (Supplementary Figure 1).
The discovered SNPs were also categorized according to nucleotide substitutions as either transitions (A↔G or C↔T) or transversions (A↔C, C↔G, A↔T, G↔T). Our results of nucleotide changes showed that guanine (G) and cytosine (C) nucleotides mutate at 1.2-fold higher rates than adenine (A) and thymine (T) nucleotides (Table 2). The results also indicated that at a higher proportion of missing data, both minor allele frequency (MAF) and proportion of heterozygous reached lower values (Figure 2). Six hundred and eighty-eight (688) putative out of 21,324 SNP markers obtained after filtering and imputation were used for downstream analyses. The gene diversity (GD) analysis revealed that the entire panel varied from 0.09 to 0.50 with an average of 0.30 ± 0.12. Moreover, among the three ecological regions, the highest level of genetic diversity was observed in accessions collected from sub-humid ecology (0.201), and the lowest in the arid ecology (0.004). Also, based on country of provenance, accessions from Guinea and Nigeria showed the highest level of genetic diversity with respectively 0.209 and 0.200 while the least level was observed in accessions from Niger (0.003) (Table 3). The AMOVA results indicated that a large proportion of total genetic variation (67%) segregates among fonio accessions rather than between ecological zones, as well as the country of provenance (Table 4).
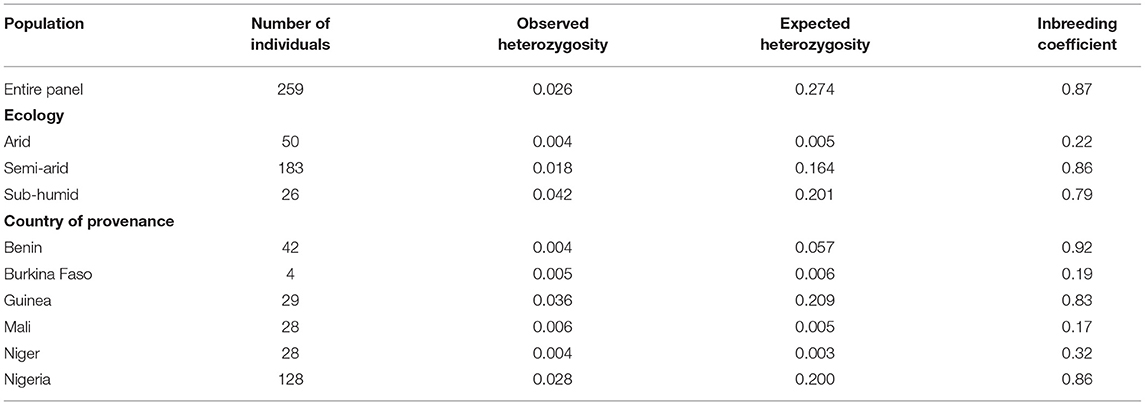
Table 3. Genetic diversity estimations of 259 fonio accessions based on ecological regions and country of provenance.
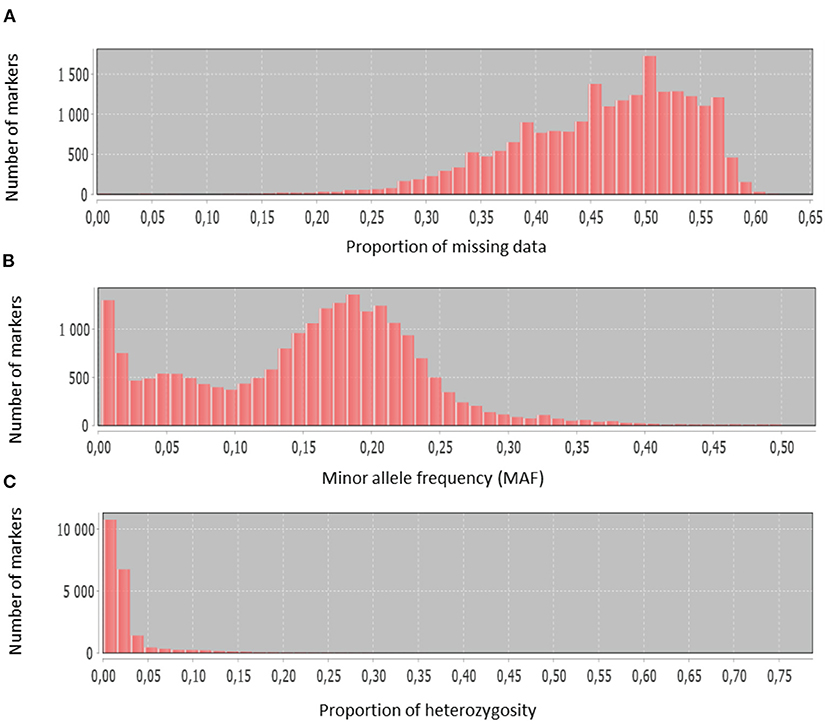
Figure 2. Histograms showing basic SNP characteristics: (A) proportion of missing data, (B) minor allele frequency, (C) proportion of heterozygosity.
Population Structure and Genetic Relatedness
The outputs of the structure analysis indicated that the likelihood of Delta K (ΔK) peaked at K =2 (Figure 3A) revealing that two sub-populations (population I and population II) showed the highest probability for population clustering (Figure 3B). The distribution of fonio accessions among the two sub-populations revealed that population II had more fonio accessions (61.39%) than population I (38.61%). The accessions from population I were mainly composed of accessions from arid and semi-arid ecologies (Nigeria, Mali and Niger) whereas most accessions from population II were from semi-arid and sub humid ecologies (Benin, Guinea, Burkina Faso). In addition, both of the two sub-populations diverged significantly as explained by the mean value of fixation index (Fst) for each of the sub-populations with 0.355 and 0.935 for population I and population II respectively (Table 5).

Table 5. Proportion of membership, estimate of genetic diversity parameters obtained from Structure results.
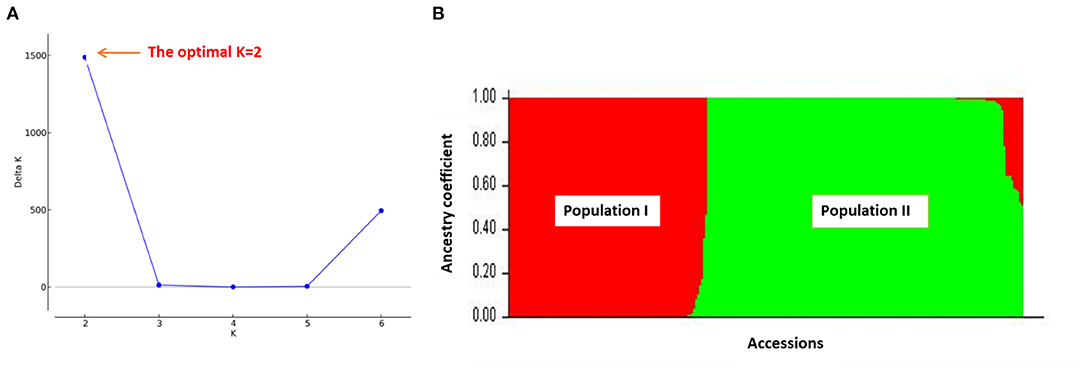
Figure 3. The STRUCTURE results of 259 fonio accessions using 688 SNP markers: (A) the optimal value of K (K = 2); (B) Bar plot of accessions showing two subpopulations (Population I = red color and Population II = green color).
Furthermore, the Neighbor-Joining dendrogram results based on dissimilarity among 259 fonio accessions revealed also two heterogenetic clusters confirming the results of the Structure analysis. Cluster I (45.18%) was mainly composed of accessions originating from Benin, Niger and Mali while Cluster II (54.82%) was essentially composed of accessions from Nigeria, Guinea, Mali, and Burkina Faso (Figure 4).
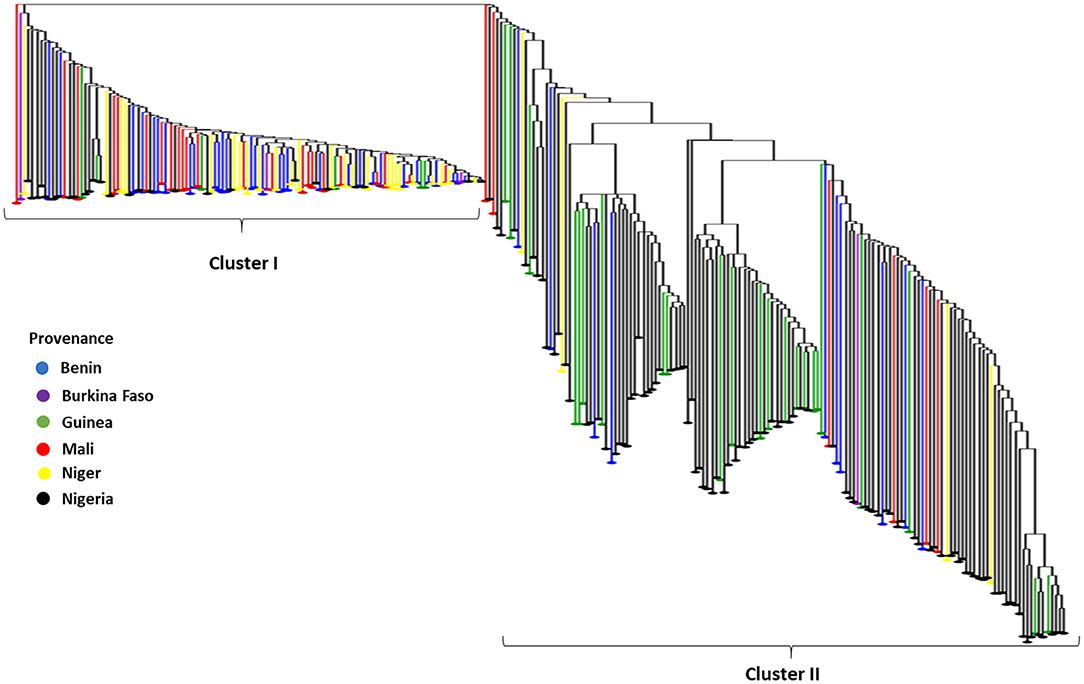
Figure 4. The Neighbor-Joining dendrogram based on dissimilarity among 259 fonio accessions using 688 SNP markers. NB: Depending on the provenance accessions were colored as follows: Benin=blue, Burkina Faso = purple, Guinea = green, Mali = red, Niger = yellow and Nigeria = black.
Population Differentiation
The degree of differentiation among fonio genotypes from different ecological regions and countries of provenance are shown in Table 6. Significant genetic differentiation was found among fonio accessions from different ecologies as indicated by the overall Weir and Cockerham's Fst-value of 0.24. Pairwise Fst-values varies from 0.19 to 0.71. The lowest Fst-value (0.19) was observed between arid and sub humid ecologies while the highest Fst-value (0.71) was observed between arid and sub-humid ecologies. However, the overall Weir and Cockerman's Fst-value based on country of provenance of fonio accessions was merely higher (0.26) compared to that of different ecological regions (0.24). Furthermore, pairwise Fst-values varied from 0.10 to 0.59. The highest Fst-value was observed between Nigeria and Benin (0.59) while the lowest value was observed between Burkina Faso and Guinea (0.10) (Table 6).
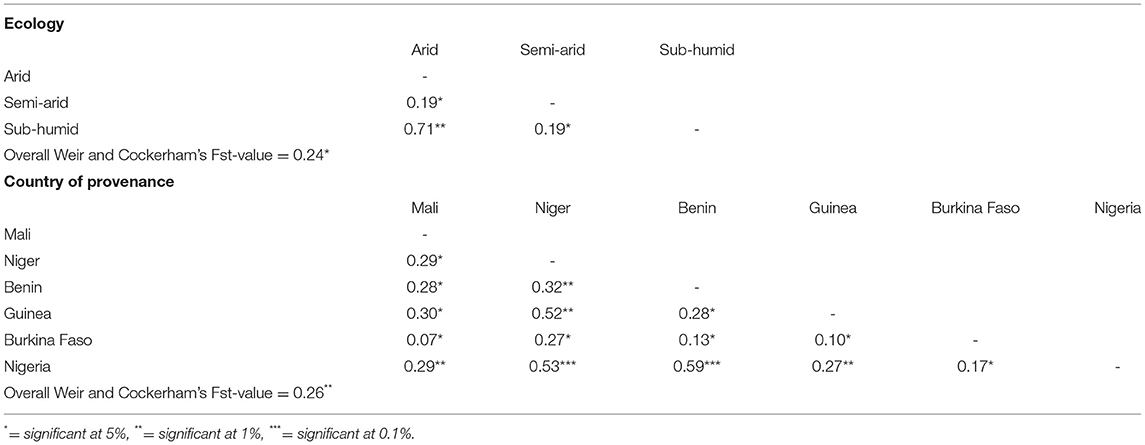
Table 6. Pairwise Fst analysis results among ecological regions and countries of provenance of 259 fonio millet accessions.
Discriminant analysis of principal components (DAPC) was estimated using the detected number of clusters. The 10 first PCs (60% of variance) of PCA and two discriminant eigenvalues were retained (Figure 5). The results showed that fonio accessions can be clustered in three groups and discriminant function 1 clearly differentiated cluster 2 from cluster 3. Cluster 1 consisted of 66.02% (171 accessions), while cluster 3 and cluster 1 had 20.46% (53 accessions) and 13.52% (35 accessions), respectively. Accessions from Benin, Burkina Faso, Mali, and Niger were more dominant in cluster 1 whereas the majority of accessions from clusters 2 and 3 were originated from Nigeria and Guinea. Results of pairwise Fst revealed high genetic differentiation among clusters with overall Weir and Cockerham's Fst-value of 0.68. Pairwise Fst-values ranged from 0.31 to 0.72 (Supplementary Table 1). The lowest Fst-value (0.31) was found between cluster 2 and cluster 3. An intermediate Fst-value (0.69) was observed between cluster 1 and cluster 2. Moreover, the highest Fst-value (0.72) was detected between cluster 1 and cluster 3.
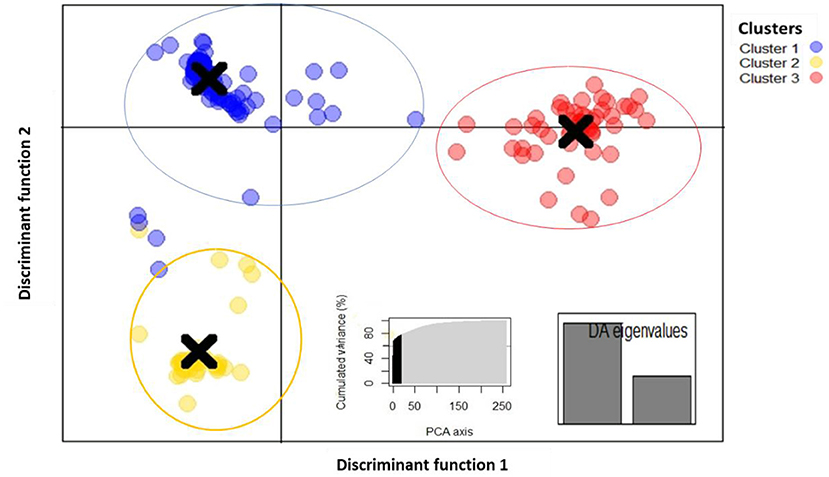
Figure 5. Discriminant analysis of principal components plot of the 259 fonio genotypes. using 688 SNP markers grouped into 3 clusters using 2 discriminant functions. Within the graph PCA axis (left) and eigenvalues (right).
Linkage Disequilibrium (LD)
LD was analyzed across the fonio genome. Of the 21,324 SNPs, 17,045 (80%) covering the entire genome were in true LD based on the Comparison between pairs of markers. The LD patterns analysis revealed that the large red blocks of haplotypes along the diagonal of the triangle plot indicate the high level of LD between the loci in the blocks (Figure 6).
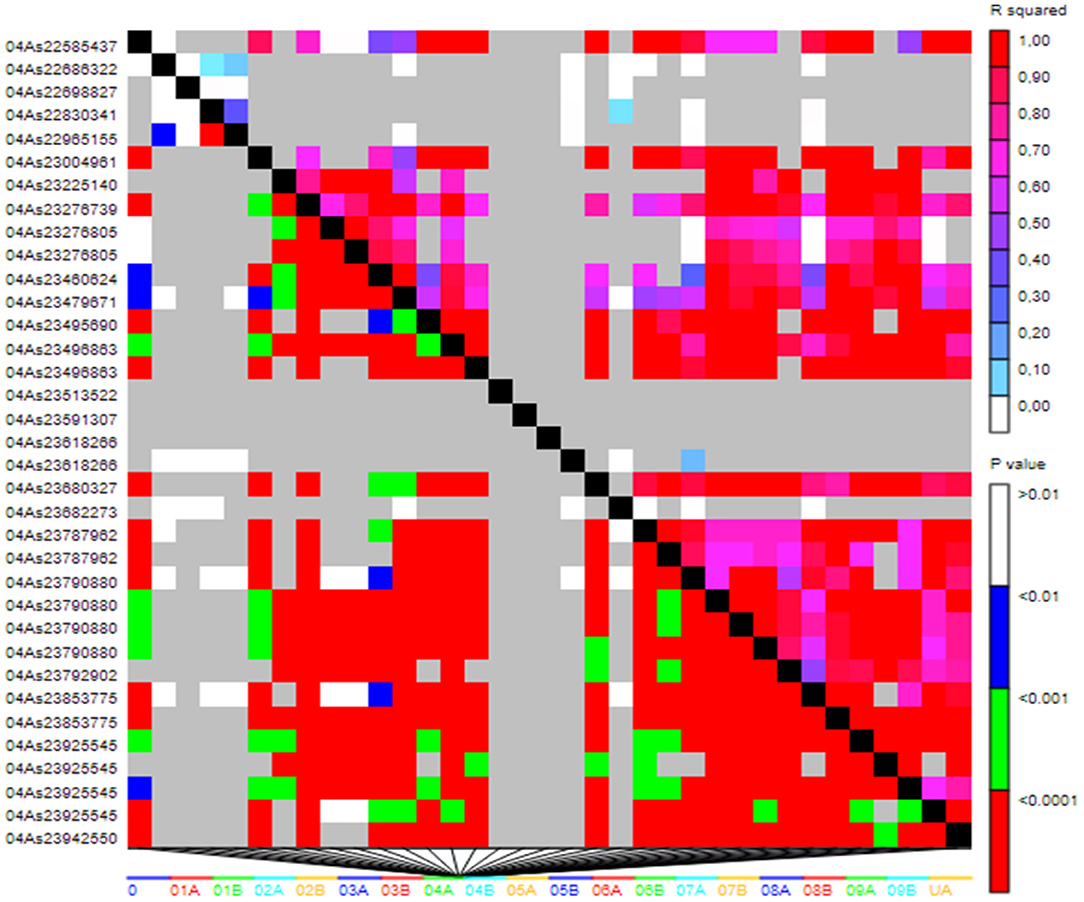
Figure 6. LD patterns plot based on 17,045 SNP markers. The relationship between two markers is represented by each cell with the color codes for the presence of significant LD. In both diagonals, colorful bar codes are shown for the significant threshold levels.
The LD decay plot showed that few LD values were found over a short physical genetic distance which decreased rapidly, with very low decline at longer distances between markers across the genome. In the present study, LD decayed rapidly below r2 = 0.64, reaching 0.56 at a distance of 5 Mb (Figure 7).
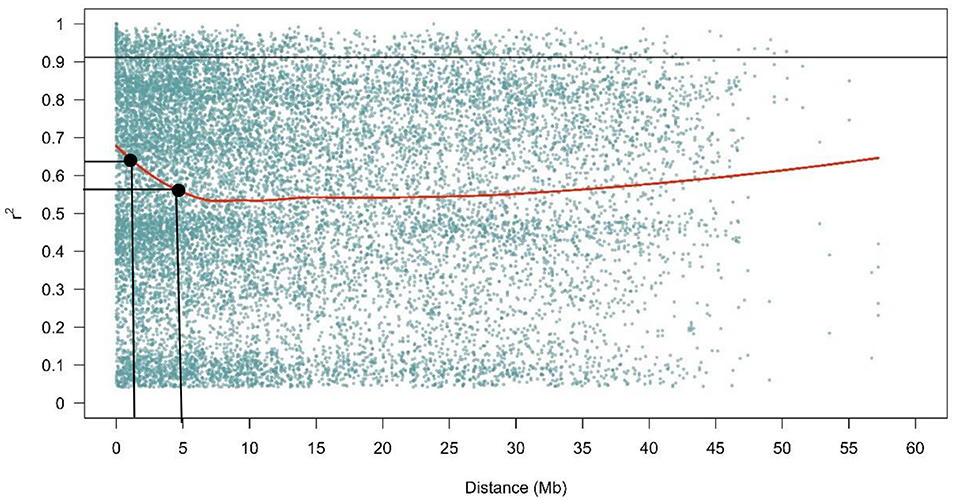
Figure 7. The LD decay plot in the fonio diversity panel. The values on the Y-axis represent r2 while the X- axis constitute the physical distance in Mb.
Discussion
SNP Markers Discovery and Genetic Diversity
Genotype-by-sequencing (GBS) provides new technologies for breeders with cost-effective, high-depth coverage, and high throughput sequencing platforms (Elshire et al., 2011; Fu and Peterson, 2011; Egan et al., 2012; Poland and Rife, 2012; Mir et al., 2013; Kim et al., 2016; Voss-Fels and Snowdon, 2016). The high throughput multiplexing genotyping platforms used in this study for assessing genetic diversity and population structure in a panel of 259 fonio accessions yielded 21,324 quality SNP markers. The relatively high proportion of missing data observed in this study might be due to the low read coverage in this dataset during genotyping because SNPs with low read coverage are more prone to errors than SNPs with high read coverage (Furuta et al., 2017). This shows the importance of sequencing to higher read depths to minimize missing data. On the other hand, this could also be explained by DNA contamination during leaf sampling which affects the quality of DNA. This result is in accordance with that of Taberlet et al. (1996) who reported that both DNA quality and or low quantity cause genotyping errors by favoring allelic dropouts and false alleles. Furthermore, in this study, the observed nucleotide changes may be due to inherent characteristics of DNA (e.g., cytosine deamination) that often cause a mutational bias in plant genomes (Gaut et al., 2011). However, the mutation rate obtained in this study was lower compared to that reported earlier in maize (Morton et al., 2006).
Previously, genetic diversity of fonio millet was investigated with a different type of markers and based on small data sets limiting the chance of getting high polymorphic and informative markers (Hilu et al., 1997; Adoukonou-Sagbadja et al., 2007; Animasaun et al., 2018). The results revealed that the markers developed in this study were highly reproducible, with a high call rate and high polymorphism information content suggesting that these markers are very important for future fonio genetic improvement. This shows the extreme importance of genotyping by sequencing analysis for SNPs discovery, harnessing genetic diversity, and genotyping in most orphan small cereals where reference genome is not yet available. The high polymorphic markers obtained in this study are within the range of values reported in earlier SNP-based genetic diversity studies in other small millets (Kumar et al., 2016; Johnson et al., 2019). However, since genetic diversity is a true indicator of the degree of genetic variance within individuals in a population, in this study a moderate level of genetic diversity was observed among fonio accessions. This was further supported by the result of AMOVA, with a large proportion of total genetic variance found within fonio accessions reflecting that to some extent our collection has captured the diversity in the crop. This result is also consistent with that reported earlier by Adoukonou-Sagbadja et al. (2007) who assessed genetic diversity of 122 fonio accessions using AFLP markers. Furthermore, compared to these results a lower level of genetic diversity was reported in foxtail millet (Wang et al., 2010). This could be attributed to factors such as inbreeding due to the self-pollinated nature and in other hand the small population size (Barnaud et al., 2012; Abrouk et al., 2020). These factors have been reported to contribute to a reduction in genetic diversity in crop plants (Bhandari et al., 2017). The results revealed also that the genetic diversity in D. exilis is concentrated in sub humid and semi-arid ecologies of West Africa comprising the Upper Niger basin (Guinea and Mali), Northwestern Benin Republic, Southwestern Burkina Faso and Northern Nigeria. This result confirms the Upper Niger basin as center of diversity of D. exilis as reported earlier by Portères and Harlan (1976). However, the level of genetic diversity observed in Nigeria suggests the proposition of Northern Nigeria as the second center of diversity of white fonio. The low genetic diversity found in the arid ecology of Niger and part of Mali may therefore be related to the founder effect that occurs when a small group of individuals that is not genetically representative of the population from which they came establish in a new area. Similar results were reported by Adoukonou-Sagbadja et al. (2007) who stated that founder effect was the main cause of low genetic variation observed in white fonio from the Atacora mountain zone (Benin and Togo areas).
Clustering and Genetic Relatedness of Fonio Accessions
Structure analyses revealed two divergent subpopulations while the Neighbor-Joining dendrogram clustered fonio genotypes in two distinct groups based on ecological regions and country of provenance, respectively. On the contrary, previous studies revealed that fonio accessions clustered in three groups and six subpopulations based on principal component analysis (PCA) and structure analysis, respectively (Abrouk et al., 2020). This difference in clustering fonio accessions could be attributed to the sample size and geographical sources where fonio accessions were collected, as well as the number of SNP markers used. For instance, in our study, we used 21,324 SNP markers and 259 fonio accessions collected from six West African countries while in previous studies, Abrouk et al. (2020) used 11,046,501 SNP markers and 166 D. exilis accessions collected from seven West African countries (Guinea, Mali, Benin, Togo, Burkina Faso, Ghana, and Niger). Nonetheless, Wang et al. (2021) used 13,546 SNP markers and identified three clusters while assessing the genetic diversity of 130 fonio accessions collected from Mali and Niger based on principal coordinates and dendrogram relationship analyses. This confirms our results of discriminant analysis of principal components, although the numbers of countries where fonio germplasm was collected, sample size, and SNP markers were lower compared to in our study. This shows the importance of diversifying sources of germplasm collection and using dense markers in order to capture the maximum diversity. Furthermore, the discrepancies between the results of our study and those of Adoukonou-Sagbadja et al. (2007) may be due to different type of markers (SNP vs. AFLPs markers) and the small data sets used, although fonio accessions were collected from nearly the same countries of provenance.
Varshney et al. (2007) reported that AFLP and SSR markers were more appropriate for fingerprinting and diversity analysis while SNP markers were considered as the best class for characterizing and conserving the genebank materials. Importantly, SNP markers can be digitalized or represented as graphic formats (Garafutdinov et al., 2020) across different genotypes making them the markers of choice for genetic diversity studies with recent advances in next generation sequencing technologies (NGS). The results of clustering fonio accessions in different groups demonstrate the influence of environmental factors and human activities on genetic variability as reported earlier (Abrouk et al., 2020). According to Wondimu et al. (2021), populations were structured based on geographical patterns and botanical races in sorghum. This is supported by the finding of Deu et al. (2010) who stipulated that seed exchange and food preferences were other factors that affected population structure in sorghum. However, in this study fonio landraces clustered irrespective of their ecological regions and country of provenance backgrounds implying that alleles from different sources co-occur within individuals. This shows the importance of admixture, a phenomenon that potentially increases genetic variation by decreasing inbreeding depression (Shi et al., 2018). This phenomenon could likely be due to the existence of gene flow through germplasm exchanges that frequently occur between different regions as reported earlier (Sidibé et al., 2020). This result is consistent with that of Kabbaj et al. (2017) who reported among wheat genotypes including landraces, varieties, and elite lines collected from diverse origins higher admixtures. The difference observed between the two clustering approaches Structure model and DAPC analysis could be attributed to the sensitivity of the Bayesian clustering algorithm to sample size, number of populations, number of loci scored and the type of markers (Evanno et al., 2005). On the other hand, with DAPC a considerable inaccuracy in terms of both the suggested number of clusters and the placement of individuals in those clusters was reported when the migration rates were high (Miller et al., 2020).
Regional Differentiation
A large genetic differentiation was found among fonio accessions based on country of provenance compared to that of ecological regions suggesting that the regional differentiation of fonio accessions is a country based rather than ecological regions. This may be likely due to farmers' management practices that are specific to ethnolinguistic groups because fonio accessions used in this study were provided by different ethnic groups of farmers from different countries. This is supported by the findings of Abrouk et al. (2020) who cited farmers' ethnolinguistic groups as among the factors that shaped genetic diversity in fonio. A possible explanation can be related to the self-pollination nature of the crop (Barnaud et al., 2012) that limits gene flow among fonio genotypes. This result corroborates the findings of Adoukonou-Sagbadja et al. (2007) who reported a high degree of differentiation within fonio accessions. Conversely, a low level of regional differentiation was reported by Wondimu et al. (2021) for 338 cultivated sorghum in Ethiopia. However, the low degree of differentiation observed between semi-arid and sub-humid zones can be explained by seed exchanges among fonio farmers due to the proximity of these zones. This finding is in agreement with that reported previously by Akohoue et al. (2020) in Kersting's groundnut and confirms the high genetic distance observed between arid and sub-humid ecologies probably due to isolation by distance (Wright, 1946). Furthermore, the great divergence observed between fonio accessions from Nigeria and the remaining countries could be also explained by geographic isolation leading to speciation which is the formation of distinct species in the course of evolution. Surprisingly, fonio accessions from Nigeria and Benin Republic for instance were collected from the same ecology (semi-arid region), but the highest divergence among fonio was observed between these two countries confirming the hypothesis that regional differentiation was country-based. Since the two groups of population (Nigeria and Benin) are thriving in the same ecology, the divergence may likely arise from management practices firstly because farmers are from different ethnolinguistic groups (e.g., Hausa ethnic group from Northern Nigeria and Ditamari from the North-western Benin Republic). This is further supported by Abrouk et al. (2020) who highlighted the strong impact of anthropogenic factors on shaping the genetic diversity of fonio. Thus, future research should investigate drivers that influence management practices of fonio millet (D. exilis) across different ethnolinguistic groups of West Africa. Secondly, the two populations might have experienced different pressures of biotic and abiotic stresses and thirdly, the informal seed systems that limit the seed exchange of improved fonio varieties (Adoukonou-Sagbadja et al., 2006; Sekloka et al., 2015) may probably cause this genetic divergence.
Linkage Disequilibrium (LD) Patterns
Even though any quantitative traits loci (QTLs) and/or markers associated with different traits on the chromosomes have been reported, yet in fonio the results show potential markers distributed on different chromosomes in the genome that were in true LD, paving ways for genome- wide association studies and genomic selection. Hence, further investigation should be made in order to map genomic regions associated with important well-preferred traits for both farmers and consumers. Furthermore, the results indicated a high level of non-random association between the loci in the blocks suggesting that there has been a low or no recombination rate since LD block formations. Moreover, our finding revealed that on average fonio germplasm was characterized by a high linkage disequilibrium with slow LD decay over long physical distances between markers. This could be attributed to the mating system of the crop because selfing limits heterozygosity, while increasing homozygosity with the consequence of reducing the effectiveness of recombination rate. As a result LD is expected to be maintained over long physical distances (Gaut and Long, 2003). Similar results were reported in wild barley (Morrell et al., 2005). The application of artificial hybridization techniques between distant genetic parents, mutagenesis and transgenesis could potentially increase recombination rate, thereby increase genetic diversity among fonio germplasm. In addition, the results of LD patterns provide relevant information on the recombination history under natural selection since no breeding efforts through artificial hybridization have been reported yet. Therefore, future LD patterns analysis should be investigated in-depth in order to shed light on other factors that alter certain genomic regions leading to the differentiation in the LD patterns among fonio accessions.
Conclusion
The present study demonstrated the potential of genotyping by sequencing (GBS) to discover SNPs, to assess genetic diversity and population structure of fonio millet accessions. The results indicated also that grouping of fonio accessions was irrespective of geographical origin backgrounds while the regional differentiation was based on the country of provenance rather than the ecological regions. Furthermore, the high genetic divergence observed between accessions from Nigeria to the other countries particularly the Benin Republic suggests geographic isolation. Moreover, the relatively high non-random association between alleles at two loci observed in this study could be attributed to the selfing nature of the crop, which reduces the recombination rate. Finally, our findings highlighted the importance of population structure, and Linkage Disequilibrium patterns in genetic diversity study and provide relevant information for association mapping and genomic selection in fonio millet.
Data Availability Statement
The datasets presented in this study can be found in online repositories. The names of the repository/repositories and accession number(s) can be found below: Dryad and https://doi.org/10.5061/dryad.vmcvdncsr.
Author Contributions
AI and EA-D conceptualized the study, wrote the manuscript, and edited the manuscript. AI, KI, and AM carried out data analysis. EA-D, CA, KI, AM, HO, MG, and CB critically revised the draft and updated the manuscript for publication. All authors contributed to the article and approved the submitted version.
Funding
AI, from the National Institute of Agronomic Research of Niger (INRAN), is a scholar of the Intra-Africa Academic Mobility Scheme under the project grant number 2016–2988 on Enhancing training and research mobility for novel crops breeding in Africa (MoBreed) funded by the Education, Audiovisual and Culture Executive Agency (EACEA) of the European Commission. The project provided a scholarship for academic training and research mobility and a research grant to the AI to complete a PhD degree at the University of Abomey-Calavi (Republic of Benin).
Conflict of Interest
The authors declare that the research was conducted in the absence of any commercial or financial relationships that could be construed as a potential conflict of interest.
Publisher's Note
All claims expressed in this article are solely those of the authors and do not necessarily represent those of their affiliated organizations, or those of the publisher, the editors and the reviewers. Any product that may be evaluated in this article, or claim that may be made by its manufacturer, is not guaranteed or endorsed by the publisher.
Acknowledgments
The authors wish to thank MoBreed program for the financial support. We are thankful to Prof Simon Krattinger and Michael Abrouk for providing data for the annotation of fonio CM05836 genome. We also thank Félicien Akohoue and Florence Kombieni from GBioS Lab for their help in leaf DNA sampling.
Supplementary Material
The Supplementary Material for this article can be found online at: https://www.frontiersin.org/articles/10.3389/fsufs.2021.699549/full#supplementary-material
References
Abdul, S. D., and Jideani, A. I. (2019). “Fonio (Digitaria spp.) breeding,” in Advances in Plant Breeding Strategies: Cereals (New York, NY: Springer), 47–81. doi: 10.1007/978-3-030-23108-8_2
Abrouk, M., Ahmed, H. I., Cubry, P., Šimoníkov,á, D., Cauet, S., Pailles, Y., et al. (2020). Fonio millet genome unlocks African orphan crop diversity for agriculture in a changing climate. Nat. Commun. 11, 1–13. doi: 10.1038/s41467-020-18329-4
Adoukonou-Sagbadja, H., Dansi, A., Vodouh,è, R., and Akpagana, K. (2006). Indigenous knowledge and traditional conservation of fonio millet (Digitaria exilis, Digitaria iburua) in Togo. Biodivers. Conserv. 15, 2379–2395. doi: 10.1007/s10531-004-2938-3
Adoukonou-Sagbadja, H., Wagner, C., Dansi, A., Ahlemeyer, J., Daïnou, O., Akpagana, K., et al. (2007). Genetic diversity and population differentiation of traditional fonio millet (Digitaria spp.) landraces from different agro-ecological zones of West Africa. Theor. Appl. Genet. 115, 917–931. doi: 10.1007/s00122-007-0618-x
Adoukonou-Sagbadja, H., Wagner, C., Ordon, F., and Friedt, W. (2010). Reproductive system and molecular phylogenetic relationships of fonio millets (Digitaria spp., Poaceae) with some polyploid wild relatives. Trop. Plant Biol. 3, 240–251. doi: 10.1007/s12042-010-9063-0
Akohoue, F., Achigan-Dako, E. G., Sneller, C., Van Deynze, A., and Sibiya, J. (2020). Genetic diversity, SNP-trait associations and genomic selection accuracy in a west African collection of Kersting's groundnut [Macrotyloma geocarpum (Harms) Maréchal & Baudet]. PLoS ONE 15:e0234769. doi: 10.1371/journal.pone.0234769
Animasaun, D., Awujoola, K., Oyedeji, S., Morakinyo, J., and Krishnamurthy, R. (2018). Diversity level of genomic microsatellite among cultivated genotypes of Digitaria species in Nigeria. Afr. Crop Sci. J. 26, 305–313. doi: 10.4314/acsj.v26i2.11
Barnaud, A., Vignes, H., Risterucci, A. M., Noyer, J. L., Pham, J. L., Blay, C., et al. (2012). Development of nuclear microsatellite markers for the fonio, Digitaria exilis (Poaceae), an understudied West African cereal. Am. J. Bot. 99, e105–e107. doi: 10.3732/ajb.1100423
Bhandari, H., Bhanu, A., Srivastava, K., Singh, M., and Shreya, H. A. (2017). Assessment of genetic diversity in crop plants-an overview. Adv. Plants Agric. Res 7:00255. doi: 10.15406/apar.2017.07.00255
Bradbury, P. J., Zhang, Z., Kroon, D. E., Casstevens, T. M., Ramdoss, Y., and Buckler, E. S. (2007). TASSEL: software for association mapping of complex traits in diverse samples. Bioinformatics 23, 2633–2635. doi: 10.1093/bioinformatics/btm308
Buckler IV, E. S., and Thornsberry, J. M. (2002). Plant molecular diversity and applications to genomics. Curr. Opin. Plant Biol. 5, 107–111. doi: 10.1016/S1369-5266(02)00238-8
Ceccarelli, S., and Grando, S. (2002). “Plant breeding with farmers requires testing the assumptions of conventional plant breeding: lessons from the ICARDA barley program,” in Farmers, Scientists and Plant Breeding: Integrating Knowledge and Practice, eds D. A. Cleveland, and D. Soleri. (Wallingford: CABI) 297332.
Chagné, D., Gasic, K., Crowhurst, R. N., Han, Y., Bassett, H. C., Bowatte, D. R., et al. (2008). Development of a set of SNP markers present in expressed genes of the apple. Genomics 92, 353–358. doi: 10.1016/j.ygeno.2008.07.008
Chen, W., Hou, L., Zhang, Z., Pang, X., and Li, Y. (2017). Genetic diversity, population structure, and linkage disequilibrium of a core collection of Ziziphus jujuba assessed with genome-wide SNPs developed by genotyping-by-sequencing and SSR markers. Front. Plant Sci. 8:575. doi: 10.3389/fpls.2017.00575
Ching, A., Caldwell, K. S., Jung, M., Dolan, M., Smith, O. S. H., Tingey, S., et al. (2002). SNP frequency, haplotype structure and linkage disequilibrium in elite maize inbred lines. BMC Genet. 3, 1–14. doi: 10.1186/1471-2156-3-19
Core Team, R. (2019). R Foundation for Statistical Computing. R: A Language and Environment for Statistical Computing. Vienna: Scientific Research.
Covarrubias-Pazaran, G. (2016). Genome-assisted prediction of quantitative traits using the R package sommer. PLoS ONE 11:e0156744. doi: 10.1371/journal.pone.0156744
Dansi, A., Adoukonou-Sagbadja, H., and Vodouhe, R. (2010). Diversity, conservation and related wild species of Fonio millet (Digitaria spp.) in the northwest of Benin. Genet. Resour. Crop Evol. 57, 827–839. doi: 10.1007/s10722-009-9522-3
Deu, M., Sagnard, F., Chantereau, J., Calatayud, C., Vigouroux, Y., Pham, J.-L., et al. (2010). Spatio-temporal dynamics of genetic diversity in Sorghum bicolor in Niger. Theor. Appl. Genet. 120, 1301–1313. doi: 10.1007/s00122-009-1257-1
Earl, D. A. (2012). STRUCTURE HARVESTER: a website and program for visualizing STRUCTURE output and implementing the Evanno method. Conserv. Genet. Resour. 4, 359–361. doi: 10.1007/s12686-011-9548-7
Egan, A. N., Schlueter, J., and Spooner, D. M. (2012). Applications of next-generation sequencing in plant biology. Am. J. Bot. 99, 175–185. doi: 10.3732/ajb.1200020
Ellegren, H. (2002). Microsatellite evolution: a battle between replication slippage and point mutation. Trends Genet. 18:70. doi: 10.1016/S0168-9525(02)02631-8
Elshire, R. J., Glaubitz, J. C., Sun, Q., Poland, J. A., Kawamoto, K., Buckler, E. S., et al. (2011). A robust, simple genotyping-by-sequencing (GBS) approach for high diversity species. PLoS ONE 6:e19379. doi: 10.1371/journal.pone.0019379
Evanno, G., Regnaut, S., and Goudet, J. (2005). Detecting the number of clusters of individuals using the software STRUCTURE: a simulation study. Mol. Ecol. 14, 2611–2620. doi: 10.1111/j.1365-294X.2005.02553.x
Evenson, R., and Gollin, D. (2003). “Crop genetic improvement in developing countries: overview and summary,” in Crop Variety Improvement and its Effect on Productivity. The Impact of International Agricultural Research, ed R. Evenson (Wallingford, UK: CABI Publishing), 7–38. doi: 10.1079/9780851995496.0007
Farnir, F., Coppieters, W., Arranz, J.-J., Berzi, P., Cambisano, N., Grisart, B., et al. (2000). Extensive genome-wide linkage disequilibrium in cattle. Genome Res. 10, 220–227. doi: 10.1101/gr.10.2.220
Fu, Y.-B., and Peterson, G. W. (2011). Genetic diversity analysis with 454 pyrosequencing and genomic reduction confirmed the eastern and western division in the cultivated barley gene pool. Plant Genome 4, 226–237. doi: 10.3835/plantgenome2011.08.0022
Furuta, T., Ashikari, M., Jena, K. K., Doi, K., and Reuscher, S. (2017). Adapting genotyping-by-sequencing for rice F2 populations. G3 Genes Genomes Genet. 7, 881–893. doi: 10.1534/g3.116.038190
Garafutdinov, R. R., Sakhabutdinova, A. R., Slominsky, P. A., Aminev, F. G., and Chemeris, A. V. (2020). A new digital approach to SNP encoding for DNA identification. Forensic Sci. Int. 317:110520. doi: 10.1016/j.forsciint.2020.110520
Gaut, B., Yang, L., Takuno, S., and Eguiarte, L. E. (2011). The patterns and causes of variation in plant nucleotide substitution rates. Annu. Rev. Ecol. Evol. Syst. 42, 245–266. doi: 10.1146/annurev-ecolsys-102710-145119
Gaut, B. S., and Long, A. D. (2003). The lowdown on linkage disequilibrium. Plant Cell 15, 1502–1506. doi: 10.1105/tpc.150730
Goudet, J. (2005). Hierfstat, a package for R to compute and test hierarchical F-statistics. Mol. Ecol. Notes 5, 184–186. doi: 10.1111/j.1471-8286.2004.00828.x
Govindaraj, M., Vetriventhan, M., and Srinivasan, M. (2015). Importance of genetic diversity assessment in crop plants and its recent advances: an overview of its analytical perspectives. Genet. Res. Int. 2015:431487. doi: 10.1155/2015/431487
Gross-German, E., and Viruel, M. A. (2013). Molecular characterization of avocado germplasm with a new set of SSR and EST-SSR markers: genetic diversity, population structure, and identification of race-specific markers in a group of cultivated genotypes. Tree Genet. Genomes 9, 539–555. doi: 10.1007/s11295-012-0577-5
Gruber, B., Unmack, P. J., Berry, O. F., and Georges, A. (2018). dartr: an r package to facilitate analysis of SNP data generated from reduced representation genome sequencing. Mol. Ecol. Res. 18, 691–699. doi: 10.1111/1755-0998.12745
Hilu, K., M'ribu, K., Liang, H., and Mandelbaum, C. (1997). Fonio millets: ethnobotany, genetic diversity and evolution. S. Afr. J. Bot. 63, 185–190. doi: 10.1016/S0254-6299(15)30742-0
Huang, B. E., George, A. W., Forrest, K. L., Kilian, A., Hayden, M. J., Morell, M. K., et al. (2012). A multiparent advanced generation inter-cross population for genetic analysis in wheat. Plant Biotechnol. J. 10, 826–839. doi: 10.1111/j.1467-7652.2012.00702.x
Johnson, M., Deshpande, S., Vetriventhan, M., Upadhyaya, H. D., and Wallace, J. G. (2019). Genome-wide population structure analyses of three minor millets: kodo millet, little millet, and proso millet. Plant Genome 12:190021. doi: 10.3835/plantgenome2019.03.0021
Jombart, T. (2008). adegenet: a R package for the multivariate analysis of genetic markers. Bioinformatics 24, 1403–1405. doi: 10.1093/bioinformatics/btn129
Kabbaj, H., Sall, A. T., Al-Abdallat, A., Geleta, M., Amri, A., Filali-Maltouf, A., et al. (2017). Genetic diversity within a global panel of durum wheat (Triticum durum) landraces and modern germplasm reveals the history of alleles exchange. Front.Plant Sci. 8:1277. doi: 10.3389/fpls.2017.01277
Kamvar, Z., Tabima, J., and Grünwald, N. (2014). Poppr: an R package for genetic analysis of populations with clonal, partially clonal, and/or sexual reproduction. PeerJ 2:e281. doi: 10.7717/peerj.281
Khatkar, M. S., Nicholas, F. W., Collins, A. R., Zenger, K. R., Cavanagh, J. A., Barris, W., et al. (2008). Extent of genome-wide linkage disequilibrium in Australian Holstein-Friesian cattle based on a high-density SNP panel. BMC Genomics 9, 1–18. doi: 10.1186/1471-2164-9-187
Kilian, A., Wenzl, P., Huttner, E., Carling, J., Xia, L., Blois, H., et al. (2012). “Diversity arrays technology: a generic genome profiling technology on open platforms,” in Data Production and Analysis in Population Genomics, eds F. Pompanon,and A. Bonin (New York, NY: Springer), 67–89. doi: 10.1007/978-1-61779-870-2_5
Kim, C., Guo, H., Kong, W., Chandnani, R., Shuang, L.-S., and Paterson, A. H. (2016). Application of genotyping by sequencing technology to a variety of crop breeding programs. Plant Sci. 242, 14–22. doi: 10.1016/j.plantsci.2015.04.016
Kumar, A., Sharma, D., Tiwari, A., Jaiswal, J., Singh, N., and Sood, S. (2016). Genotyping-by-sequencing analysis for determining population structure of finger millet germplasm of diverse origins. Plant Genome 9, p. 1–15. doi: 10.3835/plantgenome2015.07.0058
Kuta, D. D., Kwon-Ngung, E., Dachi, S., Bakare, O., and Ogunkanmi, L. (2005). Optimization of protocols for DNA extraction and RAPD analysis in West African fonio (Digitaria exilis and Digitaria iburua) germplasm characterization. Afr. J. Biotechnol. 4.
Miller, J. M., Cullingham, C. I., and Peery, R. M. (2020). The influence of a priori grouping on inference of genetic clusters: simulation study and literature review of the DAPC method. Heredity 125, 269–280. doi: 10.1038/s41437-020-0348-2
Mir, R. R., Hiremath, P. J., Riera-Lizarazu, O., and Varshney, R. K. (2013). “Evolving molecular marker technologies in plants: from RFLPs to GBS,” in Diagnostics in Plant Breeding, eds T. Lübberstedt, and R. Varshney (New York, NY: Springer), 229–247. doi: 10.1007/978-94-007-5687-8_11
Mondini, L., Noorani, A., and Pagnotta, M. A. (2009). Assessing plant genetic diversity by molecular tools. Diversity 1, 19–35. doi: 10.3390/d1010019
Morrell, P. L., Toleno, D. M., Lundy, K. E., and Clegg, M. T. (2005). Low levels of linkage disequilibrium in wild barley (Hordeum vulgare ssp. spontaneum) despite high rates of self-fertilization. Proc. Natl. Acad. Sci. 102, 2442–2447. doi: 10.1073/pnas.0409804102
Morton, B. R., Bi, I. V., McMullen, M. D., and Gaut, B. S. (2006). Variation in mutation dynamics across the maize genome as a function of regional and flanking base composition. Genetics 172, 569–577. doi: 10.1534/genetics.105.049916
Ngom, A., Gueye, M. C., Gueye, M., Billot, C., Calatayud, C., Diop, B. M., et al. (2018). Cross-species amplification of microsatellite loci developed Digitaria exilis Stapf in related Digitaria species. J. Appl. Biosci. 129, 12982–12995. doi: 10.4314/jab.v129i1.2
Olodo, K. F., Gueye, M. C., Calatayud, C., Diop, B. M., Kane, N. A., Ngom, A., et al. (2019). EST-SSR development for Digitaria exilis and its relatives D. iburua and D. longiflora from transcriptome sequences. Plant Genet. Resour. 17, 280–284. doi: 10.1017/S1479262118000400
Otyama, P. I., Wilkey, A., Kulkarni, R., Assefa, T., Chu, Y., Clevenger, J., et al. (2019). Evaluation of linkage disequilibrium, population structure, and genetic diversity in the US peanut mini core collection. BMC Genomics 20, 1–17. doi: 10.1186/s12864-019-5824-9
Perrier, X., and Jacquemoud-Collet, J. (2016). DARwin Software: Dissimilarity Analysis and Representation for WINDOWS (Version 6.0.010), (Montpellier: Science Publishers).
Poland, J. A., and Rife, T. W. (2012). Genotyping-by-sequencing for plant breeding and genetics. Plant Genome 5, 92–102. doi: 10.3835/plantgenome2012.05.0005
Porto-Neto, L. R., Kijas, J. W., and Reverter, A. (2014). The extent of linkage disequilibrium in beef cattle breeds using high-density SNP genotypes. Genet. Sel. Evol. 46, 1–5. doi: 10.1186/1297-9686-46-22
Pritchard, J. K., and Przeworski, M. (2001). Linkage disequilibrium in humans: models and data. Am. J. Hum. Genet. 69, 1–14. doi: 10.1086/321275
Pritchard, J. K., Stephens, M., Rosenberg, N. A., and Donnelly, P. (2000). Association mapping in structured populations. Am. J. Hum. Genet.67, 170–181. doi: 10.1086/302959
Raneri, J. E., Padulosi, S., Meldrum, G., and King, O. I. (2019). Promoting Neglected and Underutilized Species to Boost Nutrition in LMICs. UNSCN Nutrition, (Rome: Science for Sustainable Development).
Sehgal, D., Rosyara, U., Mondal, S., Singh, R., Poland, J., and Dreisigacker, S. (2020). Incorporating genome-wide association mapping results into genomic prediction models for grain yield and yield stability in CIMMYT spring bread wheat. Front.Plant Sci. 11:197. doi: 10.3389/fpls.2020.00197
Sekloka, E., Adoukonou-Sagbadja, H., Paraïso, A. A., Yoa, B. K., Bachabi, F.-X., and Zoumarou-Wallis, N. (2015). Evolution de la diversité des cultivars de fonio pratiqués dans la commune de Boukoumbé au Nord-Ouest du Bénin. Int. J. Biol. Chem. Sci. 9, 2446–2458. doi: 10.4314/ijbcs.v9i5.16
Serba, D. D., Muleta, K. T., St. Amand, P., Bernardo, A., Bai, G., Perumal, R., et al. (2019). Genetic diversity, population structure, and linkage disequilibrium of pearl millet. Plant Genome 12:180091. doi: 10.3835/plantgenome2018.11.0091
Shi, J., Joshi, J., Tielbörger, K., Verhoeven, K. J., and Macel, M. (2018). Costs and benefits of admixture between foreign genotypes and local populations in the field. Ecol. Evol. 8, 3675–3684. doi: 10.1002/ece3.3946
Sidibé, A., Meldrum, G., Coulibaly, H., Padulosi, S., Traore, I., Diawara, G., et al. (2020). Revitalizing cultivation and strengthening the seed systems of fonio and Bambara groundnut in Mali through a community biodiversity management approach. Plant Genet. Resour. 18, 31–48. doi: 10.1017/S1479262120000076
Sodedji, F. A. K., Agbahoungba, S., Agoyi, E. E., Kafoutchoni, K. M., Kim, H.-Y., Nguetta, S.-P. A., et al. (2020). DArT-seq Based SNP Analysis of Diversity, Population Structure and Linkage Disequilibrium Among 274 Cowpea (Vigna unguiculata L. Walp.) Accessions, (The Plant Genome). doi: 10.21203/rs.3.rs-50796/v1
Studer, B., and Kölliker, R. (2013). “SNP genotyping technologies,” in Diagnostics in Plant Breeding, eds T. Lübberstedt, and R. Varshney (New York, NY: Springer), 187–210. doi: 10.1007/978-94-007-5687-8_9
Taberlet, P., Griffin, S., Goossens, B., Questiau, S., Manceau, V., Escaravage, N., et al. (1996). Reliable genotyping of samples with very low DNA quantities using PCR. Nucleic Acids Res. 24, 3189–3194. doi: 10.1093/nar/24.16.3189
Varshney, R. K., Chabane, K., Hendre, P. S., Aggarwal, R. K., and Graner, A. (2007). Comparative assessment of EST-SSR, EST-SNP and AFLP markers for evaluation of genetic diversity and conservation of genetic resources using wild, cultivated and elite barleys. Plant Sci. 173, 638–649. doi: 10.1016/j.plantsci.2007.08.010
Voss-Fels, K., and Snowdon, R. J. (2016). Understanding and utilizing crop genome diversity via high-resolution genotyping. Plant Biotechnol. J. 14, 1086–1094. doi: 10.1111/pbi.12456
Wang, C., Chen, J., Zhi, H., Yang, L., Li, W., Wang, Y., et al. (2010). Population genetics of foxtail millet and its wild ancestor. BMC Genet. 11, 1–13. doi: 10.1186/1471-2156-11-90
Wang, X., Chen, S., Ma, X., Yssel, A. E., Chaluvadi, S. R., Johnson, M. S., et al. (2021). Genome sequence and genetic diversity analysis of an under-domesticated orphan crop, white fonio (Digitaria exilis). GigaScience 10:giab013. doi: 10.1093/gigascience/giab013
Weir, B. S. (1996). Genetic Data Analysis II Sinauer. Sunderland, MA: Sinauer Associates, Inc. Publishers.
Weir, B. S., and Cockerham, C. C. (1984). Estimating F-statistics for the analysis of population structure. Evolution 38, 1358–1370. doi: 10.1111/j.1558-5646.1984.tb05657.x
Wondimu, Z., Dong, H., Paterson, A. H., Worku, W., and Bantte, K. (2021). Genetic diversity, population structure and selection signature in Ethiopian Sorghum (Sorghum bicolor L.[Moench]) germplasm. bioRxiv. doi: 10.1101/2021.01.19.427274
Wright, S. (1946). Isolation by distance under diverse systems of mating. Genetics 31:39. doi: 10.1093/genetics/31.1.39
Keywords: Digitaria exilis, staple food, genotyping by sequencing, genetic diversity, population structure, linkage disequilibrium patterns
Citation: Ibrahim Bio Yerima AR, Issoufou KA, Adje CA, Mamadou A, Oselebe H, Gueye MC, Billot C and Achigan-Dako EG (2021) Genome-Wide Scanning Enabled SNP Discovery, Linkage Disequilibrium Patterns and Population Structure in a Panel of Fonio (Digitaria exilis [Kippist] Stapf) Germplasm. Front. Sustain. Food Syst. 5:699549. doi: 10.3389/fsufs.2021.699549
Received: 23 April 2021; Accepted: 26 July 2021;
Published: 24 August 2021.
Edited by:
Matteo Balderacchi, Independent researcher, Piacenza, ItalyReviewed by:
Yuri Shavrukov, Flinders University, AustraliaCeline Blanc-Jolivet, Thünen Institute of Forest Genetics, Germany
Copyright © 2021 Ibrahim Bio Yerima, Issoufou, Adje, Mamadou, Oselebe, Gueye, Billot and Achigan-Dako. This is an open-access article distributed under the terms of the Creative Commons Attribution License (CC BY). The use, distribution or reproduction in other forums is permitted, provided the original author(s) and the copyright owner(s) are credited and that the original publication in this journal is cited, in accordance with accepted academic practice. No use, distribution or reproduction is permitted which does not comply with these terms.
*Correspondence: Abdou R. Ibrahim Bio Yerima, abdourazakoui01@gmail.com