- 1Council for Scientific and Industrial Research (CSIR)-Soil Research Institute, Private Mail Bag, Academy Post Office, Kumasi, Ghana
- 2Department of Agricultural and Biosystems Engineering, Kwame Nkrumah University of Science and Technology, Kumasi, Ghana
Quantitative information on soil loss is relevant for devising soil conservation and crop management strategies to ensure sustainable fertility management and crop production. Estimations from runoff/erosion plots are expensive and laborious and thus requiring the exploration of other less expensive but reliable methods such as modeling. This study aimed to estimate current and future rates of soil loss for conservation planning toward sustainable crop production in the semi-deciduous forest zone of Ghana. The Universal Soil Loss Equation (USLE), which took into consideration the rainfall characteristics of the study area, inherent soil physicochemical and hydraulic properties, variations in slopes and terrain differences, land cover types, and soil management practices, was employed to estimate the magnitude and rate of soil loss in the study area. Output from three regional climate models (RCMs) from Coordinated Regional Climate Downscaling Experiment including CCCma-CanESM2, GFDL-ESM2M, and HadGEM2-ES were used to estimate the impact of climate change on soil erosion in the study area. The results showed that soil loss estimated for bare soils was high ranging from 12.7 to 163.8 t ha−1 year−1 largely due to variation in slopes coupled with soil physicochemical and hydraulic properties. The simulated annual soil losses under various land cover options showed variable degrees of soil loss for maize cultivation under conventional tillage (8.2–106.5 t ha−1 year−1), soya bean monocropping (4.4–57.3 t ha−1 year−1), and low soil loss for oil palm plantation with grass or leguminous cover (2.5–32.8 t ha−1 year−1). Evaluation of the RCMs showed excellent performance for CCCma-CanESM2 and GFDL-ESM2M. Predictions of climate change impact using outputs from CCCma-CanESM2 and GFDL-ESM2M indicated that 9–39% increase in soil loss is expected by 2070, and it will be more severe (16–42%) by 2100. The model predictions indicate that the adoption of site-specific land cover management strategies such as tree–cover crop intercropping and reduced tillage has a huge potential to reduce soil loss and sustain soil fertility. The model can be used as an advisory tool for mapping areas for appropriate cropping systems for a particular site.
Introduction
Achieving a land degradation-free world by 2030 requires the implementation of urgent actions including the accurate estimation of the extent of soil erosion toward restoring degraded land and soils (Griggs et al., 2013; Keesstra et al., 2018a). These mostly short-term actions aimed at providing sustainable solutions should be embedded in long-term landscape planning, as the realization of the aforementioned United Nations' Sustainable Development Goal (SDG) of land degradation neutrality and restoration (i.e., SDG 15.3) requires a transition toward integrated nature-based solutions founded on an eco-socioeconomic system analysis (Keesstra et al., 2018a). Furthermore, a combination of initiatives, steering of knowledge flows, and regular assessment of the status of the transition process for planning further steps in the transition framework are crucial for achieving the SDGs by 2030 (Visser et al., 2019). Soil erosion, the most visible and widespread form of land degradation, coupled with low fertilizer application is the major cause of nutrient depletion in most sub-Saharan African countries resulting in low agricultural productivity and thereby a threat to food security (Obalum et al., 2012; Bashagaluke et al., 2018). The on-site effect of soil erosion on arable land is evidenced by the loss of the topsoil that supports crop productivity (Stocking, 2003). Apart from the reduction in soil depth, soil loss almost always occurs alongside depletion of soil organic matter and plant nutrients referred to as fertility erosion (Mesele, 2014).
In sub-Sahara Africa (SSA), soil degradation is driven by a myriad of interrelated natural and anthropogenic factors including, but not limited to, high population growth and unemployment rate, agricultural expansion and intensification, poor agronomic practices, land tenure insecurity, and climate change impact (Tully et al., 2015; Nkonya et al., 2016; Borrelli et al., 2020). Sand winning from inland valleys and lowlands, whose naturally nutrient-rich status supports livelihoods of smallholder farmers through year-round crop production and the provision of ecosystem services, is currently a major problem. Inland valleys (i.e., “flat-floored and shallow valleys that occur in the extensive plains and plateaus found across the African landscape”) and lowlands cover an area of 1.9 M ha in Ghana and 190 M ha in SSA and support the production of food crops like rice, maize, sorghum, cowpea, soybean, vegetables, oil palm, cocoa, oranges, cassava, yam, plantain, and mangoes (Namara et al., 2012). Unfortunately, the aforementioned potential of inland valleys and lowlands is being threatened by indiscriminate sand winning across SSA (Aromolaran, 2012; Salifu, 2016). In Ghana, poor agronomic practices such as plowing along the slope and low fertilizer use by poor smallholder farmers result in increased soil erosion and nutrient mining, respectively (Buri et al., 2015). Excessive burning of vegetation (during land preparation) and biomass after harvesting is another farmer practice that degrade the soil by killing beneficial macroorganisms (e.g., mites, earthworms, millipedes, etc.) and microorganism (e.g., fungi) living in it (Tully et al., 2015) as well as oxidization of some important elements such as nitrogen (N), phosphorus (P), and potassium (K). The occurrence of bushfire is prevalent in northern Ghana, especially in the dry season when the vegetation is very dry. The region is consequently prone to wind erosion. Overgrazing also causes soil degradation in SSA, and especially in nomadic communities (Nkonya et al., 2016). Uncontrolled grazing by livestock renders the soil bare and prone to water and wind erosion (Tully et al., 2015; Zingore et al., 2015). According to the FAO and ITPS (2015) report, overgrazing accounts for 50% of physical soil degradation in Africa. Land tenure insecurity is a primary driver of soil degradation in Ghana and SSA at large. Smallholder tenant farmers are unwilling or averse to investing in good but expensive and/or laborious sustainable land management practices (Namara et al., 2012; Vlek et al., 2017), as they are not sure if they could keep their croplands in the next season. Land owners could easily abrogate a contract or amend it for their own interest engendering insecurity among migrant or tenant farmers.
Soil erosion (by water) is the largest threat to soil productivity in SSA, as it could occur gradually over a long period of time and elude detection until its adverse impacts are realized in low crop yields (Obalum et al., 2012; Desta et al., 2021). Soil erosion by water has a greater potential to limit soil productivity in most African regions characterized by humid tropical climate due to the torrential nature of rainfall in those regions (Obalum et al., 2012) as well as across the Mediterranean region (Rodrigo-Comino et al., 2020; Novara et al., 2021).
Although very few studies have estimated rates of soil loss from agricultural lands in Ghana (e.g., Owusu, 2012; Badmos et al., 2015; Bashagaluke et al., 2018, 2019), to the best of our knowledge, none has predicted the impact of climate change on soil erosion considering increasing rainfall variability in the region.
Methods commonly used for soil erosion estimation including runoff/erosion plots (Bashagaluke et al., 2018, 2019) and remote sensing and modeling (Owusu, 2012; Badmos et al., 2015; Gelagay and Minale, 2016; El Jazouli et al., 2017; Diwediga et al., 2018) are either laborious and expensive or qualitative (and therefore subjective). The Universal Soil Loss Equation (USLE) developed by Wischmeier and Smith (1978), which predicts long-term average annual soil loss comes in handy to provide easy but rigorous quantitative assessment of the magnitude of erosion, which is a prerequisite in designing an appropriate strategy for controlling soil erosion. According to Benavidez et al. (2018), USLE and related models including the Revised Universal Soil Loss Equation (RUSLE), the Revised Universal Soil Loss Equation version 2 (RUSLE2), and the Modified Universal Soil Loss Equation (MUSLE) have been applied globally to improve the estimation of soil loss. Furthermore, regional climate models (RCMs) from the Rossby Center Regional Atmospheric model (RCA4) and the Coordinated Regional Climate Downscaling Experiment (CORDEX) project at 44 km spatial resolution have been used in projecting climate change impact in many part of Africa (Vanvyve et al., 2008; Giorgi et al., 2009; Hewitson et al., 2012; Laprise et al., 2013; Dosio and Panitz, 2016; Nikiema et al., 2016; Sylla et al., 2016; Kisembe et al., 2018) and in Ghana (Owusu and Kluste, 2013; Bessah et al., 2018, 2020; Okafor et al., 2019).
From the foregoing context, this study aimed to estimate current and future rates of soil loss for soil conservation planning toward sustainable crop production in the Semi-deciduous Forest Zone of Ghana. The objectives of the study, therefore, include the following: (i) to quantitatively estimate soil loss under different land cover and soil management options using the USLE and (ii) to assess the impact of climate change and variability on soil erosion using outputs of applicable RCMs from CORDEX including CCCma-CanESM2, GFDL-ESM2M, and HadGEM2-ES.
Materials and Methods
Study Area Description
The study was conducted in 2014 at Kwame Nkrumah University of Science and Technology (KNUST) research field at Anwomaso located in the Ejisu Municipality of the Ashanti region of Ghana (Figure 1). It lies within latitude 6° 40′57″N and 6° 42′30″N longitude 1° 30′2″W and 1° 31′44″W. The area covers ~50 ha of semi-deciduous forest with some patches of cultivated oil palm, citrus, and cassava. The site is currently being encroached by urbanization (Figure 1C). The study area is characterized by a bimodal rainfall pattern occurring in March–July (major season) and September–November (minor season), which allows for two cropping seasons within a year. The total annual rainfall in the semi-deciduous forest agroecological zone of Ghana ranges between 1,300 and 1,500 mm (Fuji et al., 2009; Nkrumah and Adukpo, 2014). However, the total rainfall amount in a major and minor cropping season in the area is averagely 834 and 412 mm, respectively, for the period 1991–2014 (Bessah et al., 2021). Predominant crops cultivated by smallholder farmers in the area include oil palm, cassava, maize, cowpea, and soya bean. The soils of the site are mainly developed over deeply weathered granitic parent material consisting of heterogeneous soil series along the catena including Boamang, Bomso, Kotei, Akroso, and Nta (Table 1).
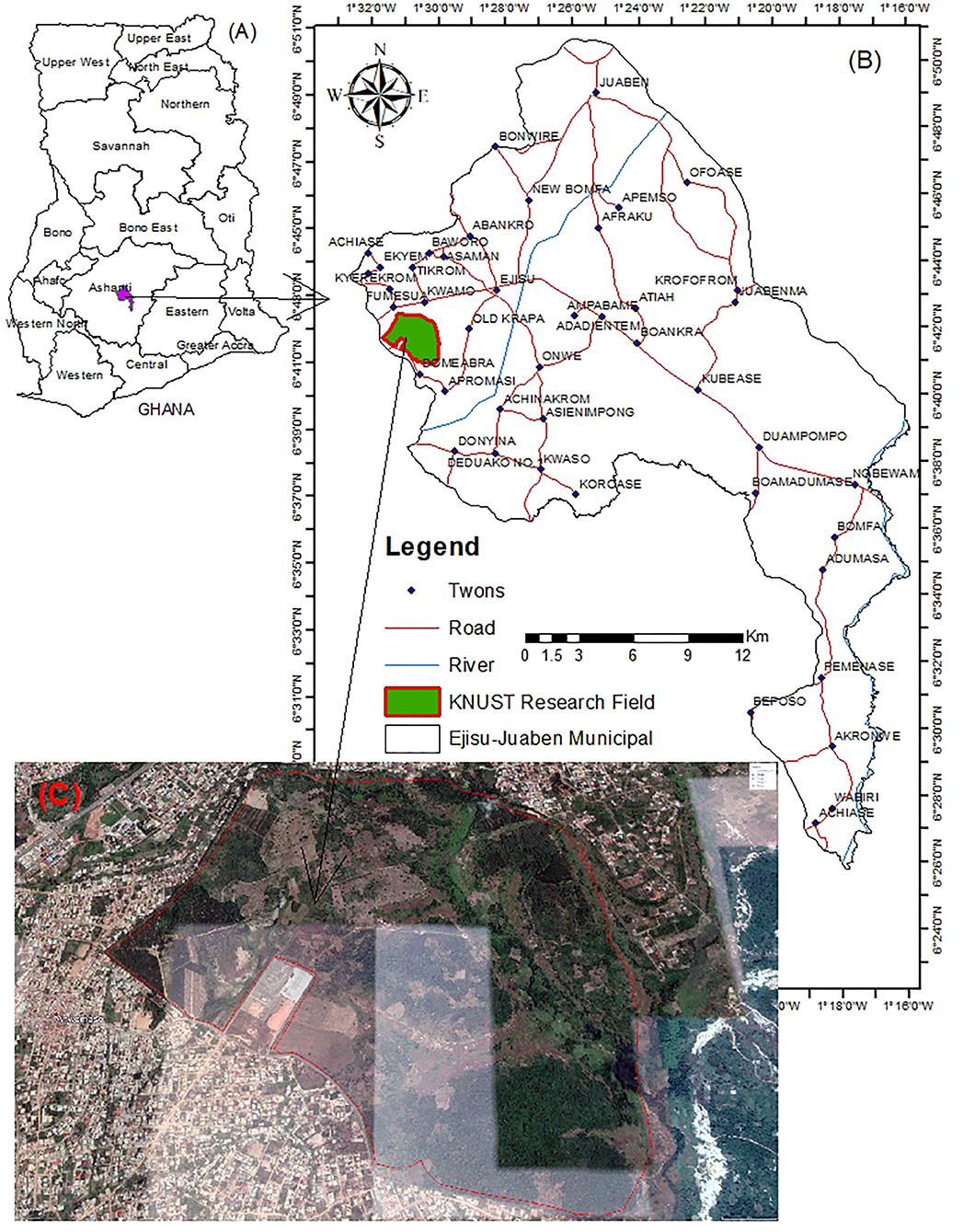
Figure 1. Map of the study site showing (A) regions in Ghana, (B) Ejisu Municipality, and (C) KNUST Research Field at Anwomaso from Google Earth Image on 21 May 2021.
Model Description
The USLE (Equation 1) is an empirical erosion model that estimates the long-term mean annual rate of soil loss on a specific field slope based on salient biophysical variables including rainfall, topography, soil type, and cropping system, as well as soil management practices (Wischmeier and Smith, 1978; Renard et al., 1997). Although USLE has been developed for application in cropping and soil management systems and rangeland, it is applicable to non-agricultural conditions such as construction sites (Renard et al., 1997). Furthermore, USLE only predicts the amount of soil erosion resulting from sheet or rill erosion on a specific slope but does not account for additional soil losses that might occur from gully, wind, or tillage erosion (Wischmeier and Smith, 1978). Input parameters for simulations in USLE are as follows: (i) rainfall–runoff erosivity factor (R)—a function of rainfall amount and its maximum intensity in 30 min—measures the erosive force and intensity of rain in a normal year and represents a geographical location within an agroecological zone; (ii) soil erodibility factor (K), measures the susceptibility of soil particles to detachment and transport by rainfall and runoff and affected by soil structure, organic matter, and permeability; (iii) slope length and steepness factor (LS), quantifies the combined effect of slope length and slope steepness and represents a ratio of soil loss per unit area on a specific site corresponding to soil loss from a 22.13-m long experimental plot with a slope steepness of 9%; (iv) land cover management factor (C), the ratio of soil loss from land under specified crop or mulch conditions to the corresponding soil loss from tilled, bare soil and determines the relative effectiveness of soil and crop management systems for preventing soil loss; and (v) support practice factor (P), the ratio of soil loss with a given surface condition to soil loss with up-and-down hill plowing and reflects the effectiveness of the support practices that will reduce the amount and rate of runoff, and thus reduce the amount of erosion; the most commonly used supporting cropland practices are cross-slope cultivation, contour farming, and strip cropping. Each of the aforementioned factors is the numerical estimate of a specific condition that affects the severity of erosion at a specific location.
where A is the annual amount of soil loss (t ha−1 year−1); R is the rainfall erosivity (MJ mm ha−1 h−1 year−1); K is the soil erodibility (t ha h ha−1 MJ−1 mm−1); LS is the slope length and steepness factor (dimensionless); C is the land cover management factor (dimensionless); and P is the support practice factor (dimensionless).
Data Collection and Analysis
Landscape and Vegetation Survey
A baseline and traverses were demarcated throughout the field at predetermined distances (100 m) using compasses, ranging poles, and a 100-m tape measure. Chisel and auger holes were dug along the grid points of 50 m on the traverses to examine, identify, and describe the soils at specific recording points. Sampling points were also recorded on the base map, and boundaries of soil units were drawn by interpolation of the points identified to create a rigid grid on the base map. The line level method was used to measure the length and steepness of slope of the identified soils in the study area. Records of observed vegetation types were taken.
Soil Profile Sampling and Analysis
Five soil profile pits were dug on identified soil series after the soil survey for a detailed soil profile description. A double ring infiltrometer was installed in situ for the measurement of the soil–water infiltration rate of the identified soil types (Touma and Albergel, 1992). The soil physical (texture, bulk density), chemical (organic carbon), and hydraulic (moisture content) properties were determined using standard procedures as described by Allison (1960) and Ibitoye (2006) at the analytical laboratory of the CSIR-Soil Research Institute in Ghana.
Rainfall Data
Rainfall data for the period 1981–2005 were accessed from the Kumasi synoptic station of Ghana Meteorological Agency (GMet) as well as simulated historical data (1981–2005) and future rainfall data (2011–2100) from CORDEX via Climate for Impact platform of the EU (climate4impact.eu). Climate model outputs were extracted in R software and bias corrected with the quantile–quantile mapping method in CMhyd software (Teutschbein and Seibert, 2012).
Estimation of Soil Loss for Different Land Cover Options
The USLE was used to predict the amount of soil loss from the identified soil series in the study area under different land cover/crop management options including oil palm with cover crops, soya bean monocropping, and maize under conservation tillage. To this end, the input parameters of USLE for the different land cover options based on the field survey and land information were determined as follows.
Rainfall–Runoff Erosivity Factor
The modified Fournier index (MFI) method, which has proven to be suitable for the tropical region, was used to determine R by adopting the interpolation table of Elbasit et al. (2013). Based on the rainfall and erosivity interpolation table, 150 mm rainfall corresponds to 400 MJ mm ha−1 h−1 year−1 erosivity (Elbasit et al., 2013).
Soil Erodibility Factor
K was read from a soil erodibility nomograph for the identified soil types using site-specific physical and hydraulic soil characteristics including soil structure (very fine granular–blocky or massive), soil permeability (very slow–rapid), soil texture (% sand, % silt + very fine sand), and soil organic matter (low) (Wischmeier et al., 1971).
Slope Length and Steepness Factor
The slope steepness determined for the identified soil types from the field survey and the predetermined slope length of 100 m were used to calculate the LS values using Equation (2) (Stone and Hilborn, 2012). LS values for the different soil types include 0.26 (Boamang), 0.59 (Bomso), 2.10 (Kotei), 1.93 (Akroso), and 1.84 (Nta).
where m = 0.5 if the percentage slope is ≥5, 0.4 on slopes in the range of 3.5–4.5%, 0.3 on slopes of 1–3%, and on 0.2 on uniform gradients of <1%.
Land Cover Management Factor
C was adopted from Roose (1975) and Stone and Hilborn (2012) for the land cover options under study including bare soil (1.0), sole maize (0.65), oil palm with cover crop (0.2), and sole soya bean (0.35).
Support Practice Factor
P was assigned 1.0 for the entire study area, as farmers applied no soil conservation measure there (Shiono et al., 2002; Stone and Hilborn, 2012).
Climate Change Impact Assessment
Outputs from three RCMs from CORDEX were used to estimate the impact of climate change on soil erosion for all the different land cover types under study. The RCMs applied include the second-generation Canadian Earth System Model (CCCma-CanESM2) from the Canadian Center for Climate Modeling and Analysis, the General Fluid Dynamics Laboratory Earth System Model (GFDL-ESM2M), and the Hadley Global Environment Model (HadGEM2-ES). CCCma-CanESM2 and GFDL-ESM2M have been found to be good models for projecting temperature and rainfall over the Pra River Basin where the current study is located (Bessah et al., 2018, 2020). HadGEM2-ES has been used by several studies over Ghana (Okafor et al., 2019). The emission scenario selected for this study was the Representative Concentration Pathways (RCP) 4.5, as tropical West Africa is reported to be a hotspot of climate change under RCP4.5 pathway projected to occur by late 2030s to early 2040s (Diffenbaugh and Giorgi, 2012; Mora et al., 2013).
Statistical Analysis
The data were subjected to analysis of variance (ANOVA) using Genstat package. Significant influence of soil properties on soil loss were separated using least significant difference (LSD) at 5% probability.
Evaluating the Performance of the Regional Climate Models
The performance of the RCMs was assessed with multiple statistical indicators using estimated erosivity based on observed rainfall data for the period 1981–2005 and those estimated with simulated historical rainfall data for the same period (i.e., 1981–2005). The statistical indicators include (i) the coefficient of determination (R2), indicating the proportion of the variance in observed data explained by the model and ranges between 0 (no agreement) and 1 (perfect agreement); typically, R2 > 0.5 is acceptable for watershed simulations (Moriasi et al., 2007); (ii) Nash–Sutcliffe model efficiency coefficient (EF), quantifying the relative magnitude of the residual variance in comparison to the variance of the observed data, and ranges from 1 (perfect match) to –∞ (poor predictability); (iii) Willmott's index of agreement (d), quantifying the extent to which the observed data correlates with the simulated data and ranges between 0 (no agreement) and 1 (perfect agreement); and (iv) normalized root mean square error (NRMSE), signifying the relative difference between the simulated results and the measured data, with NRMSE <10% showing excellent model performance, 10–20% (good), 20–30% (fair), and >30% (poor performance) (Loague and Green, 1991).
Results and Discussion
Identified Soil Types in the Study Area
The identified soil types include Orthic–Ferric Acrisol, Plinthic–Ferric Acrisol, Albic Arenosol, Gleyic Lixisol, and Gleyic Arenosol (Table 1). The soils are well-drained to imperfectly drain from the uplands to the bottom soils. The texture was sandy loam to loamy sand, with soil depth above 1.8 m with no gravels except in parts of the middle slope (Kotei series) (Table 2). The soils studied have a detrimental tendency of forming a crystallized, cemented, and hardened irreversible ferric oxide (Petro-plinthite) beneath the surface that could restrict infiltration and aid excessive runoff during rainfall (Adu, 1969).
Rainfall–Runoff Erosivity and Soil Erodibility Factors
The mean observed (1981–2005) R applied in the study was 287.1547 MJ mm ha−1 h−1 year−1, whereas the mean simulated historical (1981–2005) R values were 245.5028, 261.7396, and 112.4751 MJ mm ha−1 h−1 year−1 for CCCma-CanESM2, GFDL-ESM2M, and HadGEM2-ES, respectively (Table 3). Furthermore, mean R for the future periods (i.e., 2011–2040, 2041–2070, 2071–2100) estimated based on simulated rainfall data from the three RCMs using the aforementioned procedure have been presented in Table 4. The R values estimated from both observed and simulated rainfall data were found to be very high. According to Balogun et al. (2012), R values >160 MJ mm ha−1 h−1 year−1 are considered very high and characteristic of high erosion risk zones. Essel et al. (2016) reported R values in the range of 73.5–200.4 MJ mm ha−1 h−1 year−1 for a location within the coastal savanna agro-ecological zone of Ghana. Similarly, Roose (1976) found high R values ranging between 200 and 650 MJ mm ha−1 h−1 year−1 in Burkina Faso (formerly Upper Volta). However, very high R values in the range of 414.9–701.1 MJ mm ha−1 h−1 year−1 have been estimated in the Guinea-sudano savanna zone of Ghana (Badmos et al., 2015).
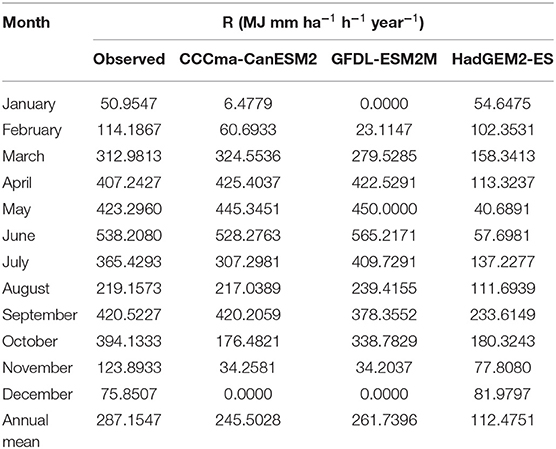
Table 3. Estimated erosivity based on observed (1981–2005) rainfall data from the Kumasi synoptic station and simulated observed rainfall data from three regional climate models for the period 1981–2005.
Estimated K values for this study ranged between 0.17 and 0.31 (Table 5). These K values were considered moderate to high on tropical soils (Roose, 1976). K values in the range of 0.02–0.32 have been recorded across West Africa (Roose, 1976).
Effect of Soil Physicochemical Properties on Erosion
The results from the soil survey conducted showed variations in the indices related to the estimated erosion. There were significant differences between soil properties of the different soil series studied along the catena, namely, Boamang, Bomso, Kotei, Akroso, and Nta, and subsequent estimated soil losses (Tables 2, 5). A surface (0–20 cm) bulk density in the range of 1.3–1.5 g cm−3 (Table 5) suggests that soils were loose and showed less sign of compaction and thus will enhance infiltration during rainfall. The generally low soil organic matter content (1.01–2.12%) coupled with a compacted subsoil (i.e., bulk density ≥1.8 g m−3) will most likely restrict water infiltration into the soil and thus facilitate excess runoff causing detachment of loose soil particles. Increased soil erosion could result from the combined effect of low organic matter content, high bulk density, and low soil–water infiltration (Charman and Roper, 2007; Novara et al., 2021).
Effect of Slope Steepness on Soil Erosion
The results show a positive linear relationship (R2 = 0.78) between slope steepness and soil loss from bare soil resulting from a respective increase in the velocity and volume of surface runoff. The steepness of the slopes (1.8–8.9%) coupled with the soil physicochemical and hydraulic properties contributed to the varying amounts of eroded soil ranging from 12.7 to 163.8 t ha−1 year−1 estimated for the various soil series (Figure 2). Boamang series found on the summit with a gentle slope (1.4–2.1%) experience less runoff that culminated in lower soil loss (12.7 t ha−1 year−1) compared with the other soil series along the middle slope to the lower slope including Bomso, Kotei, Akroso, and Nta (37.3–163.8 t ha−1 year−1). There is, thus, the need to reduce the effect of the slope characteristics on soil erosion within the catchment area by planting strip crops (e.g., Vertiver spp.) or terracing to break the slopes and reduce the volume and speed of runoff (Stone and Hilborn, 2012; Cerdà and Rodrigo-Comino, 2019). However, Cerdà and Rodrigo-Comino (2019) found that runoff and soil erosion were evenly distributed along the top, middle, and lower slopes in a Mediterranean vineyard, which could be due to a homogenization effect of a millennia-old tillage practice in the study area.
Effect of Vegetation Cover Type on Soil Erosion Control
Under oil palm with cover crop, quantity of eroded soil was similar for all the soil series (ranging from 2.5 to 32.8 t ha−1 year−1) (Figure 3). This shows that growing oil palm with cover crops on any soil of similar characteristics will not result into excessive soil erosion even at 10% slope. Erosion is reduced when raindrop impact energy is absorbed on non-erodible surfaces like vegetation and mulches, and thus, initial detachment and subsequent transport of particles is reduced (Keesstra et al., 2018b).
Soil erosion under sole soya bean crop was similar for Boamang (1.8% slope) and Bomso (3.7% slope) soil series ranging from 4.4 to 13.0 t ha−1 year−1 (Figure 3). Growing soya bean on these soils is recommended since soil erosion is not severe. Under the same crop management (i.e., sole soya bean), soil erosion for Kotei (8.9% slope), Akroso (8.4% slope), and Nta (8.1%) were similar and higher than Boamang and Bomso soil series ranging from 33.0 to 57.3 t ha−1 year−1. Sole cropping of soya bean or any crop of similar structure on slopes of above 5% will most likely result in excessive soil erosion of above 33 t ha−1 year−1. Maize cultivation under conventional tillage is most appropriate in Boamang (8.2 t ha−1 year−1 soil loss) followed by Bomso (24.2 t ha−1 year−1 soil loss) soil series (Figure 3). However, soil erosion for Kotei, Akroso, and Nta were similar and significantly higher than Boamang and Bomso. Soil erosion ranged from 61.2 to 106.5 t ha−1 year−1 for these soil series and highly inappropriate for maize cultivation under conventionally tilled conditions. Issaka et al. (2015) reported soil erosion of 8.2–10.7 t ha−1 year−1 when maize was cultivated on a 5° (8.8%) slope under tilled conditions as against relatively low eroded soil (1.5–3.2 t ha−1 year−1) under no-tilled conditions. With increasing slope, tillage should be reduced, and the use of cover crops or mulch should be considered (Cerdà and Rodrigo-Comino, 2019).
The rates and quantities of soil loss depend on the cultural practices adopted by farmers. Estimates of soil loss for maize with no-till and oil palm with cover crop gave lower soil losses as compared with maize under conventional tillage on the same stretch of land. Capello et al. (2019) reported a reduction in runoff by 76% and soil loss by 83% under soil management with grass cover compared with tillage in northwest Italy. Soil is likely to be affected by the disturbance of the soil surface that affects soil aggregates especially during tillage; the soil is easily pulverized by the plow. The use of tractors for land preparation and other farm practices affect the structure and hydraulic properties of the soil (Krebstein et al., 2014; García-Tomillo et al., 2018; Novara et al., 2021), and thus, periodic subsoiling to reduce the compaction of the lower depths could be helpful. The soil with low clay and low organic matter contents lends its particles easily to detachment when pulverized, and they are splashed and carried away by runoff (Krebstein et al., 2014; García-Tomillo et al., 2018).
Estimated losses from the various crop factors used recorded a comparatively higher rate apart from the no-till maize and oil palm with cover crop. The values could as well be lowered when conservation practices were put in place. Farmers should engage in contour farming practices that would slow down runoff and thereby enhance water infiltration with a resultant reduction in soil loss (Stone and Hilborn, 2012; Desta et al., 2021). For example, planting of strips of Vertiver spp. at 30-m intervals on moderate slopes could also be a useful strategy to reduce surface runoff. Planting Vicia sativa is also recommended for controlling soil and water losses at the early stages of vineyard plantation (Rodrigo-Comino et al., 2020).
Performance Evaluation of Climate Models Applied
Evaluation of the performance of the RCMs for simulation showed excellent performance (i.e., R2, E, and d approximately = 1, and NRMSE <10%) for CCCma-CanESM2 and GFDL-ESM2M (Figures 4A,B). However, the performance of HadGEM2-ES was only moderate, as R2 <0.5 and NRMSE was in the range of 10–20% (Figure 4C). Hence, model outputs from CCCma-CanESM2 and GFDL-ESM2M were more reliable. The excellent performance of these two RCMs confirms similar reports by Bessah et al. (2020) and Bessah et al. (2018). Sekyi-Annan et al. (2018) reported EF and d in the range of 0.65–0.83 and 0.87–0.96, respectively, and NRMSE between 17.7 and 42%, indicating good performance of an agro-hydrological model (i.e., AquaCrop) for simulating dry aboveground biomass of tomato in the Guinea-sudano-savanna agroecological zone of Ghana.
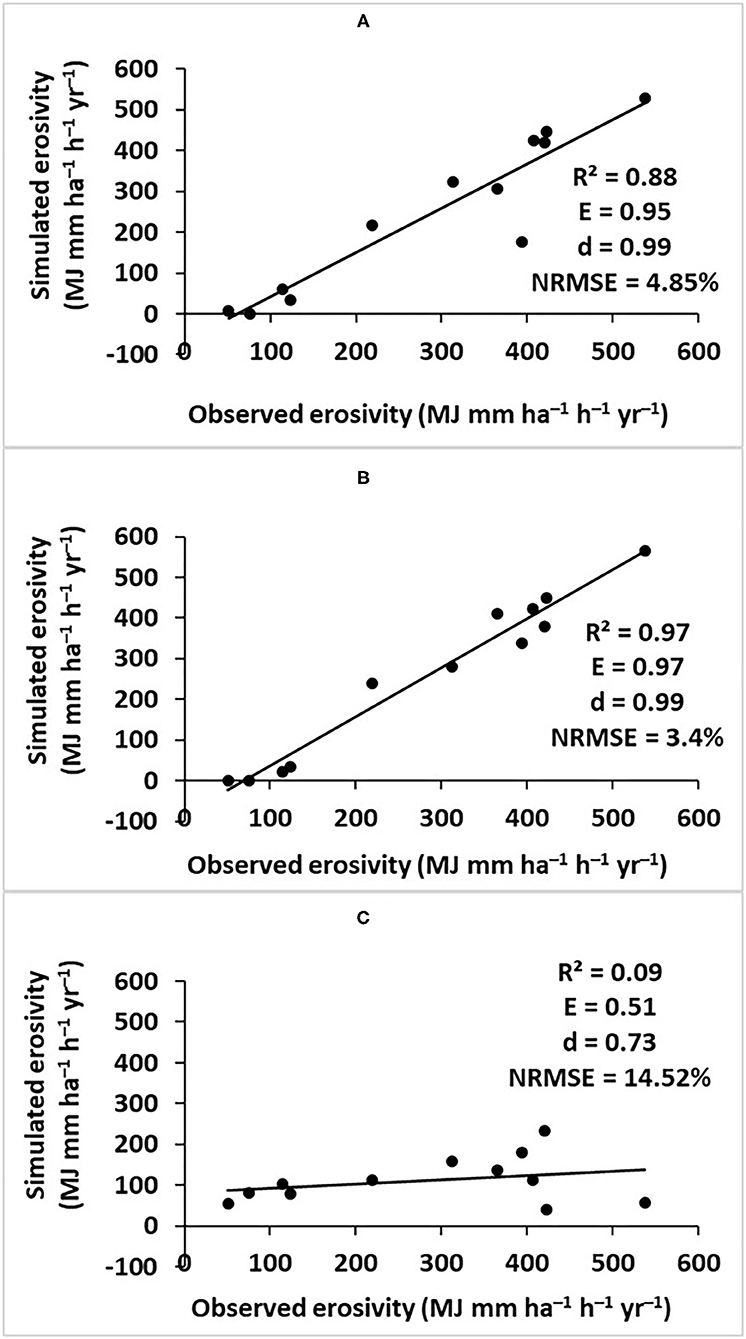
Figure 4. Performance evaluation of regional climate models including (A) CCCma-CanESM2, (B) GFDL-ESM2M, and (C) HadGEM2-ES.
Impact of Climate Change on Soil Loss
Simulation of the impact of climate change on soil loss across all land cover types in the study area varied based on RCMs applied (Figure 5). For CCCma-CanESM2, the rate of soil loss is expected to increase by 9% during 2011–2040 and 2041–2070 and 16% during 2071–2100. For GFDL-ESM2M, increasing rate of soil loss by 38, 39, and 42% are expected during the periods 2011–2040, 2041–2070, and 2071–2100, respectively. HadGEM2-ES predicted 29, 34, and 48% increase in soil loss for the same periods.
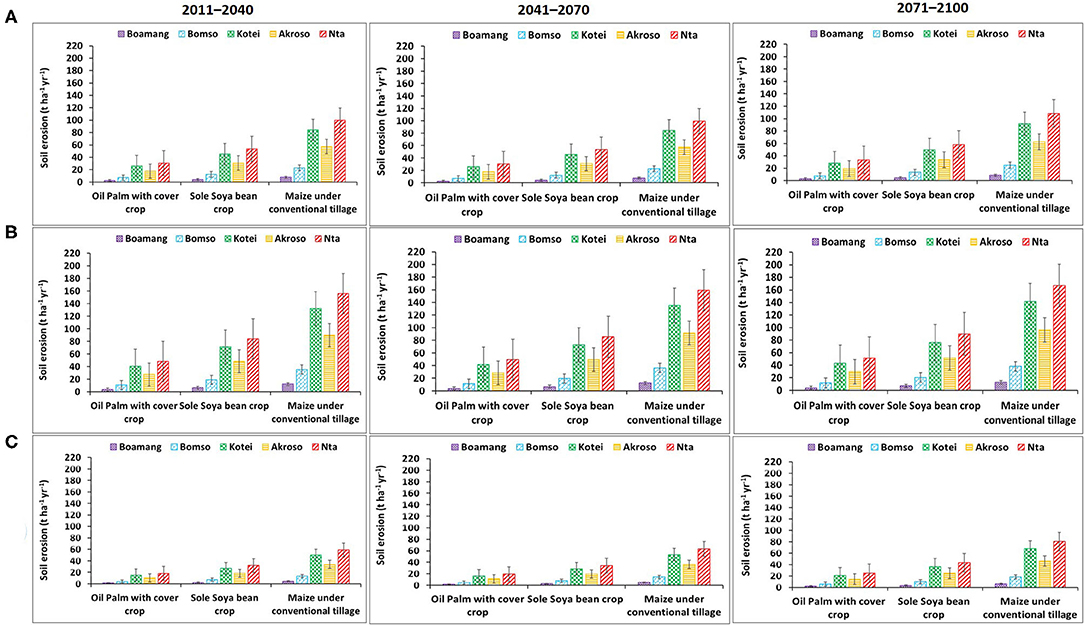
Figure 5. Predicted soil loss under climate change impact using (A) CCCma-CanESM2, (B) GFDL-ESM2M, and (C) HadGEM2-ES model outputs.
Overall, predictions of climate change impact based on outputs from CCCma-CanESM2 and GFDL-ESM2M indicated that 9–39% increase in soil loss is expected in the study area by 2070, and it will be more severe (16–42%) by 2100. The USLE's estimated high annual soil erosion rates in the study area is predicted to be exacerbated by the impact of climate change requiring urgent soil management interventions such as tree–cover crop intercropping and reduced tillage practices. Similarly, Routschek et al. (2014) predicted significant increase in soil erosion by 2050 in Germany resulting from climate change, and thus, a refusal to adapt soil management and land use would aggravate soil erosion rates. Furthermore, Borrelli et al. (2020) forecast 30–66% increase in global soil erosion due to climate change by 2070. However, Routschek et al. (2014) predicted a partial decrease in soil loss rates by 2100 in Germany probably due to differences in climate (i.e., temperate vs. tropical) and soil properties.
Conclusions
Estimation of erosion loss and the effectiveness of erosion control measures are crucial in sustainable agricultural land management considering the increasing rate of land degradation and the impact of climate change across SSA. The USLE as a predictive tool provided a relatively easier but rigorous approach to estimating the rate and quantity of soil erosion, which serves as early warning signals for land users in order to implement appropriate erosion control measures. The extent of compaction indicated by high bulk density in the subsoil is useful information for agricultural extension staff in the planning and management of agricultural mechanization services. The simulated annual soil losses under various land cover options showed variable degrees of soil losses for maize cultivation under conventional tillage (8.2–106.5 t ha−1 year−1) and for soya bean monocropping (4.4–57.3 t ha−1 year−1) and low soil loss for oil palm plantation with grass or leguminous cover (2.5–32.8 t ha−1 year−1). There existed a strong correlation between observed and simulated soil loss for all three climate models applied including CCCma-CanESM2, GFDL-ESM2M, and HadGEM2-ES. Evaluation of the RCMs showed excellent performance for CCCma-CanESM2 and GFDL-ESM2M. Predictions of climate change impact based on outputs from CCCma-CanESM2 and GFDL-ESM2M indicated that soil loss in the study area will increase by 9–39% during 2011–2040 and 2041–2070, while it will be more severe (16–42%) during 2071–2100 owing to the impact of climate change and variability. Hence, the adoption of cropping systems and cultural practices that maintain a thick layer of mulch or surface contact vegetation to prevent exposure of the soil surface to the adverse effect of the weather is crucial. The model predictions indicate that the adoption of site-specific crop management strategies such as tree–cover crop intercropping and reduced tillage has a huge potential to reduce soil loss and sustain soil fertility. Additionally, the model can be used as an advisory tool for mapping areas for appropriate cropping system suitable for a particular site.
Data Availability Statement
The raw data supporting the conclusions of this article will be made available by the authors, without undue reservation.
Author Contributions
ES-A: conceptualization, data analysis, and writing original draft of manuscript. EG: conceptualization, data collection and analysis, and review and editing of manuscript. RI: data analysis and review and editing of manuscript. GQ: data collection and review and editing of manuscript. SA: review and editing of manuscript. EB: data collection and analysis and review and editing of manuscript. All authors contributed to the article and approved the submitted version.
Conflict of Interest
The authors declare that the research was conducted in the absence of any commercial or financial relationships that could be construed as a potential conflict of interest.
Acknowledgments
The authors were grateful to Prof. Charles Quansah of the Department of Crops and Soil Science at the Kwame Nkrumah University of Science and Technology for his guidance. Our sincere gratitude also goes to the Ghana Meteorological Agency (GMet) for observed data of Kumasi synoptic station and Climate for Impact of the EU (climate4impact.eu) for giving free access to CORDEX climate models. We also appreciate the development of CMhyd for free access to bias-correct the climate data.
References
Adu, S. V. (1969). Soils of the Navrongo-Bawku area, Upper Region, Ghana. Kumasi: Soil Research Institute, Council for Scientific and Industrial Research, 1–95.
Allison, L. E. (1960). Wet-combustion apparatus and procedure for organic and inorganic carbon in soil. Soil Sci. Soc. Am. Proc. 24, 36–40. doi: 10.2136/sssaj1960.03615995002400010018x
Aromolaran, A. K. (2012). Effects of sand winning activities on land in Agrarian communities of Ogun State, Nigeria. Cont. J. Agric. Sci. 6, 41–49. doi: 10.5281/zenodo.844178
Badmos, B. K., Agodzo, S. K., Villamor, G. B., and Odai, S. N. (2015). An approach for simulating soil loss from an agro-ecosystem using multi-agent simulation: a case study for semi-arid Ghana. Land 4, 607–626. doi: 10.3390/land4030607
Balogun, I., Adegun, O., and Adeaga, O. (2012). An assessment of rainfall erosivity in parts of eastern Nigeria: a case study of Owerri and Enugu. Hydrol. Disaster Manag. 259–265.
Bashagaluke, J. B., Logah, V., Opoku, A., Sarkodie-Addo, J., and Quansah, C. (2018). Soil nutrient loss through erosion: Impact of different cropping systems and soil amendments in Ghana. PLoS ONE 13:e0208250. doi: 10.1371/journal.pone.0208250
Bashagaluke, J. B., Logah, V., Opoku, A., Tuffour, H. O., Sarkodie-Addo, J., and Quansah, C. (2019). Soil loss and run-off characteristics under different soil amendments and cropping systems in the semi-deciduous forest zone of Ghana. Soil Use Manag. 35, 617–629. doi: 10.1111/sum.12531
Benavidez, R., Jackson, B., Maxwell, D., and Norton, K. (2018). A review of the (Revised) Universal Soil Loss Equation ((R)USLE): with a view to increasing its global applicability and improving soil loss estimates. Hydrol. Earth Syst. Sci. 22, 6059–6086. doi: 10.5194/hess-22-6059-2018
Bessah, E., Raji, A. O., Taiwo, O. J., Agodzo, S. K., and Ololade, O. O. (2018). Variable resolution modeling of near future mean temperature changes in the dry sub-humid region of Ghana. Model. Earth Syst. Environ. 4, 919–933. doi: 10.1007/s40808-018-0479-0
Bessah, E., Raji, A. O., Taiwo, O. J., Agodzo, S. K., and Ololade, O. O. (2020). The impact of varying spatial resolution of climate models on future rainfall simulations in the Pra River Basin (Ghana). J. Water Clim. Change 11, 1263–1283. doi: 10.2166/wcc.2019.258
Bessah, E., Raji, A. O., Taiwo, O. J., Agodzo, S. K., Ololade, O. O., Strapasson, A., et al. (2021). Gender-based variations in the perception of climate change impact, vulnerability and adaptation strategies in the Pra River Basin. Int. J. Clim. Change Strateg. Manag. doi: 10.1108/IJCCSM-02-2020-0018
Borrelli, P., Robinson, D. A., Panagos, P., Lugato, E., Yang, J. E., Alewell, C., et al. (2020). Land use and climate change impacts on global soil erosion by water (2015–2070). PNAS 117, 21994–22001. doi: 10.1073/pnas.2001403117
Buri, M. M., Issaka, R. N., Essien, A., and Biney, N. (2015). Effect of nitrogen rates on the growth and yield of three rice (Oryza sativa L.) varieties in rain-fed lowland in the forest agro-ecological zone of Ghana. Int. J. Agric. Sci. 5, 878–885.
Capello, G., Biddoccu, M., Ferraris, S., and Cavallo, E. (2019). Effects of tractor passes on hydrological and soil erosion processes in tilled and grassed vineyards. Water 11:2118. doi: 10.3390/w11102118
Cerdà, A., and Rodrigo-Comino, J. (2019). Is the hillslope position relevant for runoff and soil loss activation under high rainfall conditions in vineyards? Ecohydrol. Hydrobiol. 20, 59–72. doi: 10.1016/j.ecohyd.2019.05.006
Charman, P. E. V., and Roper, M. M. (2007). “Soil organic matter,” in Soils–Their Properties and Management, eds P. E. V. Charman and B. W. Murphy (Melbourne, VIC: Oxford University Press), 276–285.
Desta, G., Tamene, L., Abera, W., Amede, T., and Whitbread, A. (2021). Effects of land management practices and land cover types on soil loss and crop productivity in Ethiopia: a review. Int. Soil Water Conserv. Res. doi: 10.1016/j.iswcr.2021.04.008
Diffenbaugh, N. S., and Giorgi, F. (2012). Climate change hotspots in the CMIP5 global climate model ensemble. Clim. Change 114, 813–822. doi: 10.1007/s10584-012-0570-x
Diwediga, B., Le, Q. B., Agodzo, S. K., Tamene, L. D., and Wala, K. (2018). Modelling soil erosion response to sustainable landscape management scenarios in the Mo River Basin (Togo, West Africa). Sci. Tot. Environ. 625, 1309–1320. doi: 10.1016/j.scitotenv.2017.12.228
Dosio, A., and Panitz, H.-J. (2016). Climate change projections for CORDEX-Africa with COSMO-CLM regional climate model and differences with the driving global climate models. Clim. Dyn. 46, 1599–1625. doi: 10.1007/s00382-015-2664-4
El Jazouli, A., Barakat, A., Ghafiri, A., El Moutaki, S., Ettaqy, A., and Khellouk, R. (2017). Soil erosion modeled with USLE, GIS, and remote sensing: a case study of Ikkour watershed in Middle Atlas (Morocco). Geosci. Lett. 4:25. doi: 10.1186/s40562-017-0091-6
Elbasit, A. M. A., Ojha, C. S. P., Jinbai, H., Yasuda, H., Kimura, R., and Ahmed, Z. (2013). Relationship between rainfall erosivity indicators under arid environments: case of Liudaogou basin in Chinese Loess Plateau. J. Food Agric. Environ. 11, 1073–1077.
Essel, P., Glover, E. T., Yeboah, S., Adjei-Kyereme, Y., Yawo, I. N. D., Nyarku, M., et al. (2016). Rainfall erosivity index for the Ghana Atomic Energy Commission site. SpringerPlus 5:465. doi: 10.1186/s40064-016-2100-1
FAO (2014) World reference base for soil resources. International soil classification system for naming soils and creating legends for soil maps. (Rome: Food and Agriculture Organization of the United Nations) 1–192.
FAO and ITPS (2015). Status of the World's Soil Resources (SWSR)—Main Report. Food and Agriculture Organization of the United Nations and Intergovernmental Technical Panel on Soils.
Fuji, H., Dawuni, B., Kulawardhana, W., Thenkabail, P., and Namara, R. (2009). Features of river flow in inland valleys in semi-deciduous forest zone in ghana.pdf. Trans. Jpn. Soc. Irrig. Drain. Rural Eng. 77, 637–644.
García-Tomillo, A., de Figueiredo, T., Dafonte, J. D., Almeida, A., and Paz-González, A. (2018). Effects of machinery trafficking in an agricultural soil assessed by Electrical Resistivity Tomography (ERT). Open Agric. 3, 378–385. doi: 10.1515/opag-2018-0042
Gelagay, H. S., and Minale, A. S. (2016). Soil loss estimation using GIS and remote sensing techniques: a case of Koga watershed, Northwestern Ethiopia. Int. Soil Water Conserv. Res. 4, 126–136. doi: 10.1016/j.iswcr.2016.01.002
Giorgi, F., Jones, C., and Asrar, G. R. (2009). Addressing climate information needs at the regional level: the CORDEX framework. World Meteorol. Organ. Bull. 58, 175–183.
Griggs, D., Stafford-Smith, M., Gaffney, O., Rockström, J., Öhman, M. C., Shyamsundar, P., et al. (2013). Policy: sustainable development goals for people and planet. Nature 495, 305–307. doi: 10.1038/495305a
Hewitson, B., Lennard, C., Nikulin, G., and Jones, C. (2012). CORDEX-Africa: A Unique Opportunity for Science and Capacity Building. CLIVAR Exchanges 60. Southampton: International CLIVAR Project Office, 6–7.
Ibitoye, A. A. (2006). Laboratory Manual on Basic Soil analysis, 2nd Edn. Akure: Foladave Nig. ltd., 32–36.
Issaka, R. N., Buri, M. M., and Dugan, E. (2015). Soil Research Institute Annual Report. Soil Research Institute, Council for Scientific and Industrial Reseach, Kumasi, Ghana.
Keesstra, S., Mol, G., de Leeuw, J., Okx, J., Molenaar, C., de Cleen, M., et al. (2018a). Soil-related sustainable development goals: four concepts to make land degradation neutrality and restoration work. Land 7:133. doi: 10.3390/land7040133
Keesstra, S., Nunes, J. P., Saco, P., Parsons, T., Poeppl, R., Masselink, R., et al. (2018b). The way forward: can connectivity be useful to design better measuring and modelling schemes for water and sediment dynamics? Sci. Tot. Environ. 644, 1557–1572. doi: 10.1016/j.scitotenv.2018.06.342
Kisembe, J., Favre, A., Dosio, A., Lennard, C., Sabiiti, G., and Imusiima, A. (2018). Evaluation of rainfall simulations over Uganda in CORDEX regional climate models. Theor. Appl. Climatol. 137, 1117–1134. doi: 10.1007/s00704-018-2643-x
Krebstein, K., von Janowsky, K., Kuht, J., and Reintam, E. (2014). The effect of tractor wheeling on the soil properties and root growth of smooth brome. Plant Soil Environ. 60, 74–79. doi: 10.17221/804/2013-PSE
Laprise, R., Hernandez-Diaz, L., Tete, K., Sushama, L., Separovic,', L., Martynov, A., et al. (2013). Climate projections over CORDEX Africa domain using the fifth-generation Canadian Regional Climate Model (CRCM5). Clim. Dyn. 41, 3219–3246. doi: 10.1007/s00382-012-1651-2
Loague, K., and Green, R. E. (1991). Statistical and graphical methods for evaluating solute transport models: overview and application. J. Contam. Hydrol. 7, 51–73. doi: 10.1016/0169-7722(91)90038-3
Mesele, S. A. (2014). Quantifying maize yield and erosion influencing factors for soil loss prediction under different tillage and soil amendments (M.Sc. thesis). Kwame Nkrumah University of Science and Technology, Kumasi, Ghana.
Mora, C. F. A. G., Longman, R. J., Dacks, R. S., Walton, M. M., Tong, E. J., Sanchez, J. J., et al. (2013). The projected timing of climate departure from recent variability. Nature 502, 183–187. doi: 10.1038/nature12540
Moriasi, D. N., Arnold, J. G., Liew, M. W. V., Bingner, R. L., Harmel, R. D., and Veith, T. L. (2007). Model evaluation guidelines for systematic quantification of accuracy in watershed simulations. Trans. ASABE 50, 885–900. doi: 10.13031/2013.23153
Namara, R., Hope, L., and Awuni, J. (2012). Inland Valleys in Ghana: Untapped Potential. AgWater Solutions Project Case Study. Accra: International Water Management Institute (IWMI). pp. 1–28.
Nikiema, P. M., Sylla, M. B., Ogunjobi, K., Kebe, I., Gibbaa, P., and Giorgid, F. (2016). Multi-model CMIP5 and CORDEX simulations of historical summer temperature and precipitation variabilities over West Africa. Int. J. Climatol. 37, 2438–2450. doi: 10.1002/joc.4856
Nkonya, E., Johnson, T., Kwon, H. Y., and Kato, E. (2016). “Economics of land degradation in Sub-Saharan Africa,” in Economics of Land Degradation and Improvement–A Global Assessment for Sustainable Development, eds E. Nkonya, A. Mirzabaev, and J. von Braun (Washington, DC: International Food Policy Research Institute), 215–259.
Nkrumah, F., and Adukpo, D. C. (2014). Rainfall variability over Ghana: model versus rain gauge observation. Geoiogical Atmos. Sci. Publ. 5, 673–83. doi: 10.4236/ijg.2014.57060
Novara, A., Cerda, A., Barone, E., and Gristina, L. (2021). Cover crop management and water conservation in vineyard and olive orchards. Soil Tillage Res. 208:104896. doi: 10.1016/j.still.2020.104896
Obalum, S. E., Buri, M. M., Nwite, J. C., Hermansah, W. Y., Igwe, C. A., and Wakatsuki, T. (2012). Soil degradation-induced decline in productivity of sub-saharan african soils: the prospects of looking downwards the lowlands with the sawah ecotechnology. Appl. Environ. Soil Sci. 2012:673926. doi: 10.1155/2012/673926
Okafor, G., Annor, T., Odai, S., and Agyekum, J. (2019). Volta basin precipitation and temperature climatology: evaluation of CORDEX-Africa regional climate model simulations. Theor. Appl. Climatol. 137, 1–25. doi: 10.1007/s00704-018-2746-4
Owusu, G. (2012). A GIS-based estimation of soil loss in the Densu Basin in Ghana. West Afr. J. Appl. Ecol. 20, 41–51.
Owusu, K., and Kluste, N. A. B. (2013). Simulation of the rainfall regime over Ghana from CORDEX. Int. J. Geosci. 4, 785–791. doi: 10.4236/ijg.2013.44072
Renard, K. G., Foster, G. R., Weesies, G. A., McCool, D. K., and Yoder, D. C. (1997). Predicting Soil Erosion by Water: A guide to Conservation Planning with the Revised Universal Soil Loss Equation (RUSLE), Agriculture Handbook No. 703. Washington, DC: U.S. Department of Agriculture.
Rodrigo-Comino, J., Terol, E., Mora, G., Giménez-Morera, A., and Cerd,à, A. (2020). Vicia sativa Roth. Can reduce soil and water losses in recently planted vineyards (Vitis vinifera L.). Earth Syst. Environ. 4, 827–842. doi: 10.1007/s41748-020-00191-5
Roose, E. J. (1975). Natural mulch or chemical conditioner for reducing soil erosion in humid tropical areas. Soil Cond. 7, 131–138. doi: 10.2136/sssaspecpub7.c12
Roose, E. J. (1976). “Use of the universal soil loss equation to predict erosion in West Africa,” in Soil Erosion: Prediction and Control (Ankeny, IA: Soil Conservation Society of America), 21, 60–74.
Routschek, A., Schmidt, J., and Kreienkamp, F. (2014). Impact of climate change on soil erosion—a high-resolution projection on catchment scale until 2100 in Saxony/Germany. Catena 121, 99–109. doi: 10.1016/j.catena.2014.04.019
Salifu, B. (2016). Implications of sand mining on the environment and livelihoods in brong ahafo region (M.Phil. thesis). Kwame Nkrumah Universtity of Science and Technology (KNUST), Kumasi, Ghana, 1–100.
Sekyi-Annan, E., Tischbein, B., Diekkrüger, B., and Khamzina, A. (2018). Year-round irrigation schedule for a tomato–maize rotation system in reservoir-based irrigation schemes in Ghana. Water 10:624. doi: 10.3390/w10050624
Shiono, T., Kamimura, K., Okushima, S., and Fukumoto, M. (2002). Soil loss estimation on a local scale for soil conservation planning. JARO 36, 157–161. doi: 10.6090/jarq.36.157
Stocking, M. A. (2003). Erosion and Crop Yield in Marcel Dekker, Inc. Norwich: University of East Anglia.
Stone, R. P., and Hilborn, D. (2012). Universal Soil Loss Equation [Factsheet]. Ontario: Queens's Print for Ontario.
Sylla, B. M., Nikiema, P. M., Gibba, P., Kebe, I., and Klutse, N. A. B. (2016). “Climate change over West Africa: recent trends and future projections,” in Adaptation to Climate Change and Variability in Rural West Africa, eds Yaro, J. A. and Hesselberg, J. (Cham: Springer International), 25–40.
Teutschbein, C., and Seibert, J. (2012). Bias correction of regional climate model simulations for hydrological climate-change impact studies: review and evaluation of different methods. J. Hydrol. 456–457, 12–29. doi: 10.1016/j.jhydrol.2012.05.052
Touma, J., and Albergel, J. (1992). Determining soil hydrologic properties from rain simulator or double ring infiltrometer experiments: a comparison. J. Hydrol. 135, 73–86. doi: 10.1016/0022-1694(92)90081-6
Tully, K., Sullivan, C., Weil, R., and Sanchez, P. (2015). The state of soil degradation in sub-Saharan Africa: baselines, trajectories, and solutions. Sustainability 7, 6523–6552 doi: 10.3390/su7066523
Vanvyve, E., Hall, N., Messager, C., Leroux, S., and Van Ypersele, J. (2008). Internal variability in a regional climate model over West Africa. Clim. Dyn. 30, 191–202. doi: 10.1007/s00382-007-0281-6
Visser, S., Keesstra, S., Maas, G., de Cleen, M., and Molenaar, C. (2019). Soil as a basis to create enabling conditions for transitions towards sustainable land management as a key to achieve the SDGs by 2030. Sustainability 11:6792. doi: 10.3390/su11236792
Vlek, P. L. G., Khamzina, A., Azadi, H., Bhaduri, A., Bharati, L., Braimoh, A., et al. (2017). Trade-offs in multi-purpose land use under land degradation. Sustainability 9:2196. doi: 10.3390/su9122196
Wischmeier, W. H., Johnson, C. B., and Cross, B. V. (1971). Soil erodibility nomograph for farmland and construction sites. Soil Water Conserv. J. 26, 189–193.
Wischmeier, W. H., and Smith, D. D. (1978). Predicting Rainfall Erosion Losses: A Guide to Conservation Planning. USDA Handbook 537. Washington, DC: USDA.
Keywords: soil loss, universal soil loss equation, climate change, land use land cover, modeling, semi-deciduous forest of Ghana
Citation: Sekyi-Annan E, Gaisie E, Issaka RN, Quansah GW, Adams S and Bessah E (2021) Estimating Soil Loss for Sustainable Crop Production in the Semi-deciduous Forest Zone of Ghana. Front. Sustain. Food Syst. 5:674816. doi: 10.3389/fsufs.2021.674816
Received: 02 March 2021; Accepted: 31 May 2021;
Published: 05 July 2021.
Edited by:
Kwame Agyei Frimpong, University of Cape Coast, GhanaReviewed by:
Mohammed Jashimuddin, University of Chittagong, BangladeshArtemi Cerdà, University of Valencia, Spain
Copyright © 2021 Sekyi-Annan, Gaisie, Issaka, Quansah, Adams and Bessah. This is an open-access article distributed under the terms of the Creative Commons Attribution License (CC BY). The use, distribution or reproduction in other forums is permitted, provided the original author(s) and the copyright owner(s) are credited and that the original publication in this journal is cited, in accordance with accepted academic practice. No use, distribution or reproduction is permitted which does not comply with these terms.
*Correspondence: Ephraim Sekyi-Annan, c2VreWlhbm5hbkB5YWhvby5jb20=