- 1Department of Agricultural Research for Northern Sweden, Swedish University of Agricultural Sciences, Umeå, Sweden
- 2Department of Crop Production Ecology, Swedish University of Agricultural Sciences, Uppsala, Sweden
Ongoing climate change is already affecting crop production patterns worldwide. Our aim was to investigate how increasing temperature and CO2 as well as changes in precipitation could affect potential yields for different historical pedoclimatic conditions at high latitudes (i.e., >55°). The APSIM crop model was used to simulate the productivity of four annual crops (barley, forage maize, oats, and spring wheat) over five sites in Sweden ranging between 55 and 64°N. A first set of simulations was run using site-specific daily weather data acquired between 1980 and 2005. A second set of simulations was then run using incremental changes in precipitation, temperature and CO2 levels, corresponding to a range of potential future climate scenarios. All simulation sets were compared in terms of production and risk of failure. Projected future trends showed that barley and oats will reach a maximum increase in yield with a 1°C increase in temperature compared to the 1980–2005 baseline. The optimum temperature for spring wheat was similar, except at the northernmost site (63.8°N), where the highest yield was obtained with a 4°C increase in temperature. Forage maize showed best performances for temperature increases of 2–3°C in all locations, except for the northernmost site, where the highest simulated yield was reached with a 5°C increase. Changes in temperatures and CO2 were the main factors explaining the changes in productivity, with ~89% of variance explained, whereas changes in precipitation explained ~11%. At the northernmost site, forage maize, oats and spring wheat showed decreasing risk of crop failure with increasing temperatures. The results of this modeling exercise suggest that the cultivation of annual crops in Sweden should, to some degree, benefit from the expected increase of temperature in the coming decades, provided that little to no water stress affects their growth and development. These results might be relevant to agriculture studies in regions of similar latitudes, especially the Nordic countries, and support the general assumption that climate change should have a positive impact on crop production at high latitudes.
Introduction
Climate change is expected to have both positive and negative impacts on crop production and food security around the world (Schleussner et al., 2018; Ray et al., 2019), depending on the area considered and on adaptation strategies (Olesen et al., 2011). In the Nordic countries and more generally at high latitudes, the agricultural sector could benefit from an increased arable area and longer growing seasons, due to temperature rise (King et al., 2018) and CO2 fertilization (e.g., Thivierge et al., 2016). Suitable areas for the cultivation of maize and, to a lesser extent, for wheat, might increase, while no change is expected for oats under future climate for high latitudes (Elsgaard et al., 2012). However, it is also expected that adverse effects could happen at such latitudes, mostly because of extreme climate events, such as droughts (Trnka et al., 2014; Wiréhn, 2018). In Sweden, this was recently illustrated with the extreme drought observed during the summer of 2018 (Wilcke et al., 2020). Swedish agriculture is built to a large extent on rainfed systems, and is dependent upon a recharged soil water profile in spring, and subsequent regular precipitation. Many farmers in 2018 were not able to sow their crops due to low surface soil water content, and even perennial forages were yielding lower than normal, eventually resulting in the slaughtering of livestock. For annual crops, reduced and poor quality yields were obtained due to the combined effects of water stress and high temperatures. Quantifying the potential impacts of climate change on Swedish agricultural systems could help to identify upcoming opportunities and challenges.
One way to perform such quantification is to use mechanistic crop models and assess their response to various climate scenarios. Mechanistic crop models are versatile numerical tools that are widely used to predict potential yields (e.g., van Ittersum et al., 2013; Silva et al., 2017), identify optimal farming practices (e.g., O'Grady and O'Hare, 2017; Pelak et al., 2017; Rodriguez et al., 2018) or forecast the effects of climate change on crops (e.g., Bindi et al., 2015; Ewert et al., 2015).
In the Nordic countries, examples include the work from Rötter et al. (2013), who simulated barley climate-induced stresses using the WOFOST crop model for Finnish conditions. They showed that CO2 fertilization and adjusted sowing windows can lead to small increase barley yields for most regions of Finland. Eckersten et al. (2012) and Nkurunziza et al. (2014) used the MAISPROQ model to simulate the potential yield of maize in Sweden under a changing climate. Their results suggest that the likeliness of reaching an appropriate fodder quality would increase for most regions in Sweden, with some exceptions due to the effects of water stress, and that yield should not significantly increase under future climate conditions. More recently, Macholdt et al. (2021) used the DAISY crop model to simulate the impact of future climate on yield risk of winter wheat in eastern Denmark. There results showed that adapted nitrogen supply and soil organic contents could mitigate the effects of climate change on the yield.
Another relevant option to evaluate the effects of climate change on crop production is the APSIM crop model (Keating et al., 2003). Although it has not previously been applied for climate change scenario analysis in the context of Nordic countries, APSIM has an open source code, is supported by an active user and developer community, and most importantly, already includes useful submodules to simulate the crops assessed in this study and the effects of changes in precipitation and temperature on the phenology and yield of the crops. Examples of APSIM being used for such tasks include Pembleton et al. (2016), Phelan et al. (2014), or Bahri et al. (2019).
We chose four major cereals (barley, maize, oat, and spring wheat) and used the APSIM crop model to simulate the growth and phenology across five pedo-climatic representative areas of Sweden. The broader aim of the research was to examine how these crops may perform in the future, and explore possibilities to revise, adapt, and formulate new production scenarios to increase the benefits for producers while reducing the risks. The specific aim of this study was to simulate potential trends of future crop production in Sweden using combined incremental changes in temperatures, CO2, and precipitation.
Materials and Methods
Crop Modeling
Simulations of plant growth and development were performed using the mechanistic crop model APSIM (version 7.10). Mechanistic crop models can simulate crop growth and development as a function of soil, climate and agronomic management, providing information on interactions of genotype, environment and management (GxExM). The minimum weather information required to run APSIM includes daily precipitation, minimum and maximum air temperatures, and solar radiation. The reader is referred to Keating et al. (2003) and Holzwortha et al. (2014) for a detailed description of the general framework of APSIM. Soil and climate data were organized for each location, along with farming practices for each crop, as inputs for APSIM.
Parameterization of APSIM
Soil Data
All simulations were conducted as a succession of independent growing seasons, i.e., all soil initial conditions were reset at the start of each simulation. The soil water content was set to full capacity at sowing due to the snow melt in spring, as proposed by Eckersten et al. (2012).
For all locations except Uppsala (Figure 1), soil profiles were collected by the authors for 10 layers (Umeå) or 6 layers (Kristianstad, Lidköping, and Färjestaden) to a depth of 120 cm.
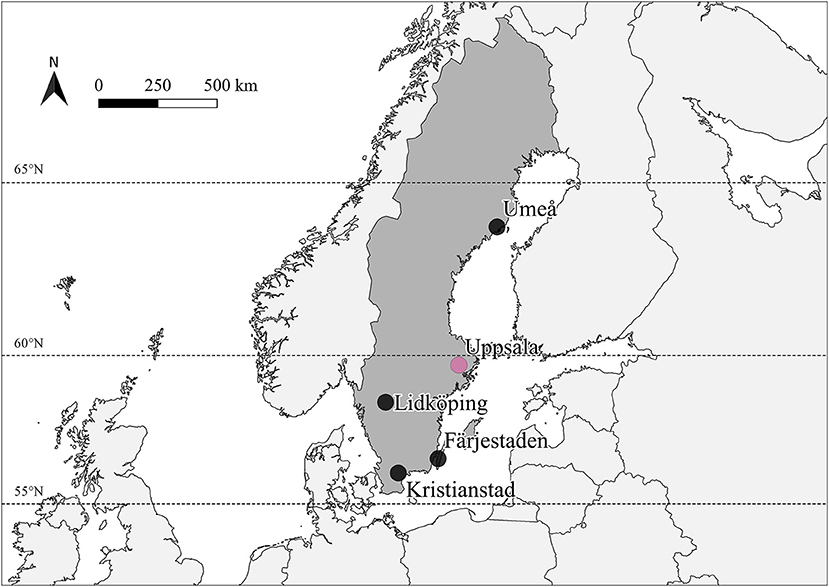
Figure 1. Locations used in the study. Black circles indicate locations with soil sampling performed by the authors. The pink circle shows the location for which soil data were acquired from a previous report (Wiklert et al., 1983).
Soil physical and chemical characteristics were measured using laboratory methods and are available in a public dataset (Morel et al., 2019). For Uppsala, soil data acquired by Wiklert et al. (1983) were used to compute an estimation of the soil water characteristics using the SPAW model (Saxton and Rawls, 2006). Soil data are available in Supplementary Materials.
Farming Practices
Farming practices (including sowing dates, plant densities and depths) were defined for each crop based on local expert knowledge (Table 1). Sowing and cut-off harvest dates were defined based on usual practices for each crop whenever possible.
In the case of spring wheat and forage maize in Umeå, dates of sowing and harvest were estimated based on expert knowledge, as these crops are not currently cultivated in this area. Nitrogen fertilizer rates were higher than recommended rates, to avoid any potential influence of nitrogen-related stress on crop photosynthesis and production.
Climate Datasets
Quantification of the impact of climate on crop yields and food security requires long-term historical daily weather data to obtain a robust conclusion. Long term daily weather data are not easily available for many regions. To overcome these limitations, gridded weather databases are available, for which the data are typically derived from (1) global circulations models (GCM), (2) interpolated weather station data, or (3) remotely sensed surface data from satellites. The research program on Climate Change, Agriculture and Food Security (CCAFS) is one of such platforms that provides downscaled gridded data of a region from GCMs outputs. Based on the coordinates of the locations of interest, data can be downloaded for climate impact studies.
In a context of climate change, increases in temperature for Sweden under the IPCC scenario with highest emissions (RCP8.5) are around 3°C by 2050, when compared to the baseline 1961–1990 (Hagman et al., 2018). The predicted increase is higher in northern Sweden (around 5°C). Similarly, an increase in precipitation by 10% is project for Sweden, and 20–25% when considering only the northern region. The prediction for CO2 concentration is between 450 and 600 ppm by 2050, depending on the emission scenario (IPCC AR5). In order to account for all these potential changes, the synthetic data was genereated to quantify different combinations of the predicted climate factors for selected locations in north and south Sweden. Two sets of climate data were used in this study, each of them containing daily rainfall, solar radiation, and minimum and maximum air temperatures.
The first dataset (hereinafter refered to as the baseline scenario) consisted of a daily data series from 1980 to 2005 of bias-corrected gridded weather data downloaded for each location from the CCAFS website (see http://www.ccafs-climate.org/ for more details). A summary of the main climate variables is provided in Table 2.
A second weather dataset (hereinafter refered to as future scenarios) was constructed using an incremental method as used by Lana et al. (2017). Incremental methods are commonly applied in climate scenario construction to study the sensitivity of an exposure unit (like e.g., yield) to a wide range of variations in climate and to construct impact response surfaces over multivariate climate spaces. Using the APSIM factorial tool, daily maximum and minimum temperatures were increased in 1°C steps until a total increment of +5°C, concomitantly with CO2 concentrations increasing from 350 to 560 ppm. Precipitation amount was changed by applying multiplication factors from −20, −10, −5, +5, and +15% to each precipitation event from the baseline scenario. Each temperature/CO2 combination was then factorially combined with changes in daily precipitation, resulting in 30 future scenarios.
Varietal Calibration
The values of the parameters describing the crop cultivars used in this study were obtained from previous studies: Kumar and Parsons (2020) and (Kumar et al., 2021) for barley and oats, Morel et al. (2020b) for maize and Asseng et al. (2019) for spring wheat. The reader is referred to the Supplementary Materials for further details on the calibration of the cultivars used in the study.
Approach to Compare the Baseline and Future Scenarios
APSIM output files were imported and analyzed with R 3.6.2 (R Core Team, 2019). The crop production response was assessed using two indicators: yield change and yield variability.
Yield change (δy, expressed in %) is crop and site specific and was computed as
where my is the median yield of a given crop for a given climate scenario and site, and ry is the reference yield of the given crop, which was computed as
where bn is the median yield of a given crop under the baseline climate, for the nth location.
Yield variability (vy, expressed in %) was calculated as the coefficient of variation of the yield of a given crop-location-climate scenario combination
where σy and μy are, respectively, the standard deviation and the mean of the yields for a given crop-location-climate scenario combination.
Rules to consider a given crop as a failure were defined based on simulated phenological stages in APSIM. Forage maize was considered a failure if the grain filling process was less than half completed. For barley, oats, and spring wheat, the crop was considered a failure if the physiological maturity stage was not completed. Crop failure risk was computed as the percentage of failures for a given crop-location-climate scenario combination.
Results
The results obtained for the baseline dataset are consistent with the expected productivity in farmer fields for each crop, with average grain yields of 5.4, 4.4, and 5.7 t.ha−1 for barley, oats and spring wheat, respectively, and an average dry matter yield of 11.5 t.ha−1 for forage maize. The reader is referred to the Supplementary Materials for a more formal comparison of simulated and observed yields (Supplementary Figure 1).
Changes in Yields
The results presented in this section refer to relative yield (dry matter of grain yield for threshed cereals or aboveground dry matter yield for forage maize).
Barley
The simulated barley yields are lower in Umeå than at the locations in southern Sweden (Figure 2A). Yield variability, particularly in scenarios with increases in temperature, are also higher in Umeå than for the other locations, where barley yields decrease with increasing temperatures. The productivity is not affected by changes in precipitation. In Uppsala, maximum yield is observed with a 1°C increase in temperature. Subsequent increases in temperature lead to a decreasing yield. Changes in precipitation do not affect the yield, irrespective of temperature increase.
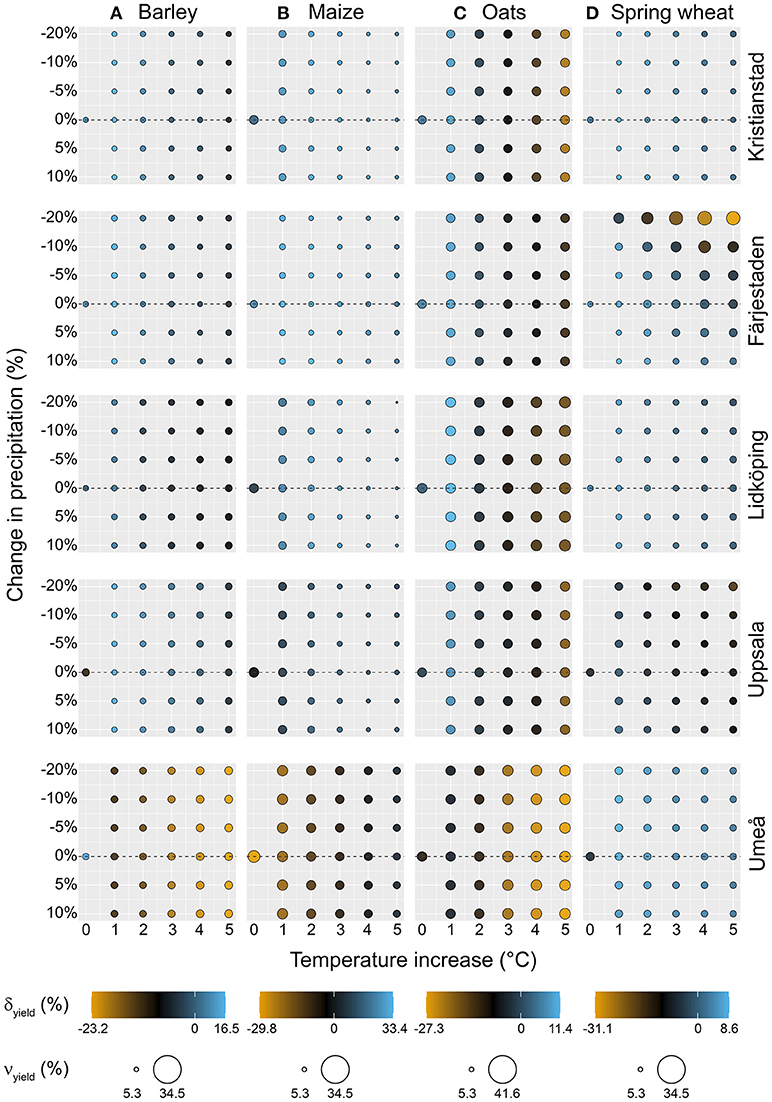
Figure 2. Changes in crops yields for the five locations of the study. Brown colors indicate a decrease in yield while blue colors indicate an increase in yield. The size of the points indicates the variability of the yield (coefficient of variation). The dashed line indicates a 0% change in precipitation. The yield reference value (0%) was computed as the mean of the baselines of the five locations. νyield: yield variability. δyield: yield change. (A) Barley, (B) Maize, (C) Oats, and (D) Spring wheat.
At Lidköping, simulated yields for the baseline climate are higher compared to Uppsala, whereas increasing temperature leads to a greater decrease in yield compared to Uppsala. Similar yields are observed for all future scenarios with 3 to 5°C of temperature increase. Yield variability is similar for all scenarios, including the baseline. At Färjestaden, the baseline yield is similar to Lidköping. The maximum yield is observed with a 1°C increase in temperature, although higher precipitation results in slightly higher yields. Subsequent increases in temperature lead to a decrease in yield. The yield variability does not change meaningly with the combinations of temperature and precipitation. At Kristianstad, the yields and yield variabilities of all combinations of temperature and precipitation are similar to Färjestaden, with a maximum yield obtained for a 1°C temperature increase and the minimum yield obtained for an increase of 5°C.
Maize
The results suggest that maize should benefit from the expected increase of temperature, with higher yields and reduced variability observed for all locations (Figure 2B). However, the optimal conditions vary depending on the location. In Kristianstad, the simulation results show that the aboveground dry matter yield of maize increases along with temperature when compared to baseline results, while the yield variability decreases. The maximum increase of yield is obtained for a temperature increase of 2–3°C.
Results are similar in Lidköping, with an overall increase in yield and a decrease in variability, and a maximum yield gain obtained with a 2–3°C increase. In Umeå, where maize is not currently cultivated, the baseline results showed a lower yield in comparison with the mean of all baseline simulations. The increase in temperatures results in a continuous increase of yield and a decrease in yield variability. In Uppsala, the baseline results are noticeably lower than the average of all locations, and the increase in temperature results in a progressive increase in yields, although the effects are less pronounced than in Umeå. In Färjestaden, the baseline results show the highest yield and a slightly reduced variability, and the maximum increase of production is observed for a temperature increase of 1–2°C. In all studied location, variations in precipitation do not influence the yield.
Oats
In general, the greatest decreases in oats yields at higher temperature are observed at Umeå and Lidköping. At all locations, maximum yield is observed with a 1°C increase in temperature (Figure 2C). Above a 1°C increase in temperature, the positive effect of CO2 fertilization does not reduce the negative impact of increased temperature on yield. At Umeå, maximum yield is observed with 1°C increase in temperature with lowest yield variability. Subsequent increase in temperature induces a decreasing yield, with the highest decrease at 5°C. Yield variabilities are similar from 2 to 5°C temperature increases with all precipitation combinations. In Uppsala, the maximum yield is observed at 1°C increase in temperature, with precipitation slightly influencing the performances. Subsequent increases in temperatures induce a decrease in yield, the lowest one being obtained with 5°C of temperatures increases. The yield variability is similar for all climate scenarios.
At Lidköping, similar to Uppsala, yields were higher at 1°C. Greater temperatures increases lead to a decreased yield and increased variability. The lowest yield is observed for a 5°C temperature increase with all combinations of precipitation. At Färjestaden and Kristianstad, similar to other southern locations, yield is maximum at 1°C and then decreases with further increasing temperature for all combinations of precipitation, the lowest yield being obtained with a 5°C increase of temperature. Yield variability is similar for all scenarios.
Spring Wheat
Wheat yield trends at all five sites are shown in Figure 2D. The impact of change in temperature at Kristianstad depicts a significant positive effect on grain yield for a 1°C increase, while the variability in yield increases at 3 to 5°C. Temperature and rainfall increase also show a positive effect on grain yield of wheat at Lidköping but with higher variability compared to Kristianstad.
Wheat yield in Umeå (where wheat is not currently cultivated) increases concomitantly with temperature. Higher temperature at Umeå depicts higher yield from 5 to 15% increase in rainfall, while variability in yield is slightly decreasing due to increased temperature under both 5 and 15% rainfall regimes. At Uppsala the trend in wheat yield shows that it remains highest at 1°C temperature for all rainfall change regimes. Further increases in temperature result in declined wheat yield for all precipitation change regimes. Wheat yields at Färjestaden are highest with 1°C of increase compared with higher temperature changes of 4 to 5°C. Yield outcomes decrease with decreased precipitation and increased temperature, accompanied by increasing yield variability at Färjestaden. The highest decline in yield is observed at 5°C with 20% decrease in rainfall. However, increased rainfall (15%) results in higher yield even at 5°C temperature compared with decreased rainfall.
Risk of Crop Failure
Crop failure as presented here describes the risk of the crop not reaching the physiological maturity stage (for barley, spring wheat, and oats) or, in the case of maize, having the grain filling process interrupted before it was half completed. For the baseline scenarios, risk of failure for threshed cereals was high in Umeå (27% for spring wheat, 23% for barley, and 21% for oats) when compared to other locations. Simulations with increased temperatures showed no negative effects on the risk of failure. In the case of barley, oats and spring wheat, failure risk showed little to no location or climate scenario-dependent variation, and are therefore not presented here. Results for maize showed a more pronounced dynamic, especially in Umeå (Table 3).
The risk of failure remains very low for Kristianstad, Lidköping, and Färjestaden. In Uppsala, the risk of failure for the baseline simulations is noticeably higher compared to future climate simulations. In Umeå, however, the risk of failure for the baseline simulations is very high, with 0% of baseline simulations reaching the maturity required for forage maize. The projected increases in temperatures rapidly lead to a reduction of the risk of failure, with virtually no risk for scenarios with temperatures increased by 4 and 5°C.
Discussion
Model Parameterization and Calibration
Modeling natural processes is a delicate exercise of balancing the simplification of a set of complex phenomena while maintaining a high level of accuracy in the final output. In this study, we used simplifications and approximations to define some of the driving parameters of APSIM (e.g., sowing dates, definition of a crop failure). Although the simplifications that we made can be eventually modified, we consider the results obtained as an acceptable first approximation of the potential trend of four important annual crops cultivated in Sweden in the incoming decades. The results are also an efficient way to point out the knowledge gaps that need to be further assessed to increase our understanding of what the future of food production could be in Sweden and other Nordic countries.
Limitations of Climate Scenarios
The method used here to define synthetic climate scenarios is also a simplification of a complex task—predicting climate change. The data used in this study account for a change in magnitude of the climate variables (warmer temperatures, more or less precipitation), but do not consider potential changes in the variability of the climate (e.g., distribution of precipitation), thus might not represent the current forecasted climate for the coming decades. However, we consider that this factorial combination of increased temperatures and associated CO2 concentrations, and both increased and decreased precipitation amounts as an important tool to assess the respective effects of temperature, CO2, and precipitation on the development and growth of the crops.
Production and Risks of Failure Predicted Trends
All crops show an increase of yield concomitant with temperatures and CO2 concentrations increases, although the optimal range of temperatures for increases varies from one crop and locations combination to another. This has been reported in previous studies, as e.g., in Rötter et al. (2011), Rötter et al. (2013), Ozturk et al. (2018), Macholdt et al. (2021), Palosuo et al. (2021). All these studies emphasize that crops in the Nordic countries can benefit from increased temperatures until a certain threshold, depending on the crop, location and model considered. To the best of our knowledge, the present study is the first to confirm these findings for Sweden.
From the perspective of risk of failure, the results obtained here suggest that there are few or no negative effects of climate change if the current management is kept constant. This is consistent with expectations as the increase of temperatures induces a reduction of the days required to reach grain maturity, provided that there is no or little water stress. The simulation averaged phenology-related water stress was zero for barley and spring wheat simulations, while a very limited stress was reported for maize simulations in Uppsala (Figure 3). Water stress affecting phenology was more frequent for oats, especially at Färjestaden, yet simulated stresses did not result in any noticeable change in terms of risk of failure. In Sweden, and more generally in the Nordic countries, crops are, in general, more prone to temperature-related stresses rather than water-related stresses. In the case of our study, this is illustrated in Figure 4, which shows a comparison of the respective effects of temperature and CO2 vs. precipitation on the variability of yields, expressed by coefficient of variation. Temperature and CO2 concentrations appear to largely influence the variability of yields compared to precipitation, with the exception of spring wheat in Färjestaden. This exception could be explained by the fact that Färjestaden is a relatively dry place compared to the other locations, and also because wheat has a long growing season, which can result in a greater sensitivity to a reduction of precipitation. Overall, temperature and CO2 explain more than 89% of the variability, while precipitation only accounts for 11%. One important aspect in Nordic regions is that frequently the soil soils are at field capacity at the beginning of the cropping season, allowing a better establishment of the crops and a lower dependency on the total precipitation during the cropping season.
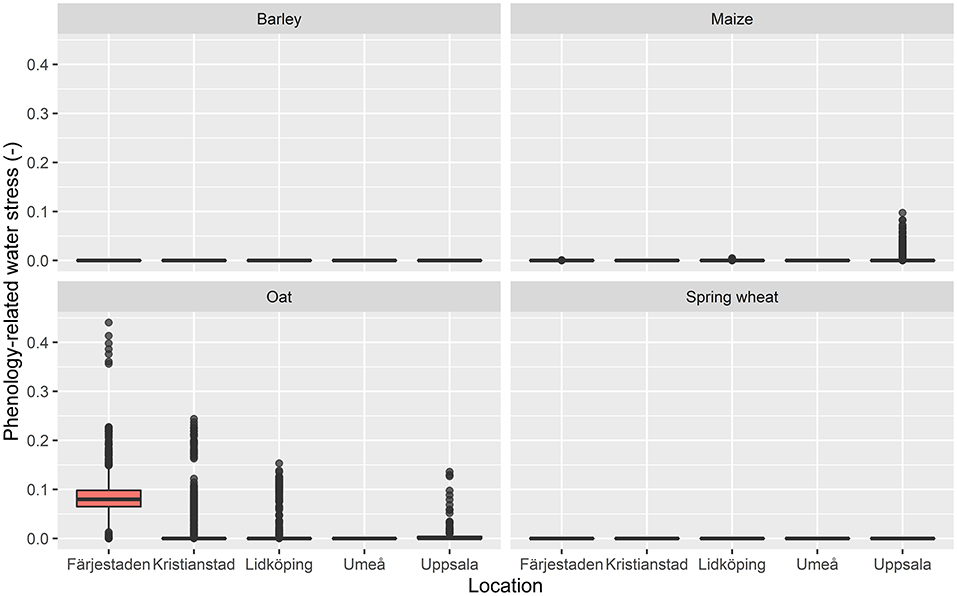
Figure 3. Simulation averaged phenology-related water stress. Barley, spring wheat and to a lesser extent, maize, showed little to no water stress. Oats, on the other hand, and especially at Färjestaden, appears to have a more prononced water stress affecting its phenology, although this does not impacted its risk of failure.
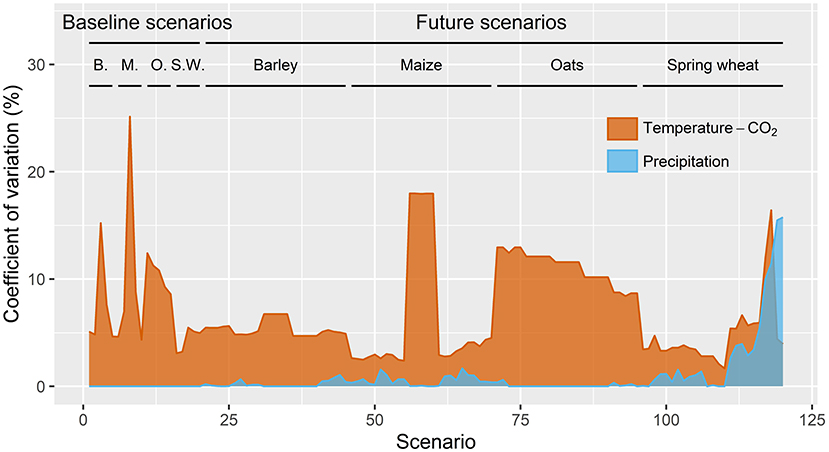
Figure 4. Comparison of temperature-CO2 vs. precipitation related coefficients of variation of simulated yields. The coefficient of variation is computed over the 26 years of simulation of a given scenario for the same site and crop and regrouped according to the increase in temperature and CO2 (to assess the effects of precipitation) or changes in precipitation (to assess the effects of temperature and CO2). B., M., O. and S. W. stand for barley, maize, oats, and spring wheat, respectively.
The absence of negative effects of mild climate change on crop production in Sweden could also partly be explained by the fact that increased CO2 levels can compensate to some extent for droughts effects, as reported in previous studies, as for example Manderscheid and Weigel (2007) or Robredo et al. (2007). However, this question needs to be further assessed in order to develop a better understanding of the physiological processes that link CO2 concentrations, water use efficiency and stresses affecting the plant, as suggested by Boote et al. (2013).
Simulated yields for high increase of temperatures and decrease of precipitation suggest that maize will perform better than other crops in conditions with increased risk of drought. Especially, barley and oats tend to be more sensitive to increases in temperature and CO2 (Figures 2A,C). This could be because, under increased temperatures, crops would progress more rapidly through phenological stages, thus having less time to accumulate biomass, as suggested by Wang et al. (2009). Maize, as a C4 plant, is expected to perform better with warmer temperatures than currently typical for Nordic countries. Another reason is that the root system of maize can penetrate soil deeper when compared to other crops, which limits the effects of low surface moisture levels.
Opportunities for Further Studies
The structure of APSIM is complex and requires fine-tuning of many parameters that heavily influence the final outputs of the model. This task is particularly hard when simulating crop production for various locations with different farming practices and soils. Different methods, including e.g., Bayesian statistics or satellite remote sensing should be tested, with the expectation of a reduced uncertainty on some of the most critical parameters of APSIM. In this study, fixed sowing dates were used for each crop and location to simulate the growth of the plants. Using sowing windows with flexible sowing rules could make results more representative of actual conditions, as suggested in Thornton et al. (2017). Similarly, simulations were set up in such a way that the nitrogen stress was kept low. Testing the effect of fertilization rates on crop development and growth might provide good farming strategies to mitigate the effects of increased water and temperature stresses. Another limitation comes from the fact that the current parameterization of APSIM 7.10 does not account for differences in transpiration efficiency between different plants, and more especially between C3 and C4 crops, which has been highlighted by Ainsworth and Long (2004). Finally, we emphasize that this work is an impact study using current management. Future adaptation studies can assess the opportunities for different adaptation options, such as breeding cultivars that take advantage of the extended growing season.
Conclusion
We used the APSIM crop model to simulate the growth of four crops in five locations in Sweden under various climate scenarios. Baseline climate (1980 to 2005) simulations were run and compared with synthetic future scenarios, with incremental increases in temperatures and both increases and decreases in precipitation. The results suggested that crops will perform differently depending on locations and climate scenarios: although all crops should benefit from an increase in temperatures, the maximum yield will often be reached with small (1°C) temperature increases for barley, oats and spring wheat, whereas forage maize can benefit from greater temperatures increases. Changes in temperature did not dramatically affect the risk of failure, with the exception of maize in Umeå, where risk decreased with increasing temperature. This change might have been partly reached, as Hogda et al. (2001) reported already 20 years ago that the length of the growing season considerably increased between 1981 and 1999. Changes in precipitation showed limited influence on both productivity and risk of failure of crops. In the case of a high increase of temperature and decrease in precipitation, oats was most negatively affected compared to the baseline simulations, whereas forage maize showed the highest increase in production. In conclusion, this work suggests that the cultivation of annual crops in Sweden should to some extent benefit from the expected rise in temperature linked with climate change, provided that little to no water stress affects the growth and development of the crops. Potential improvements in the methodology and in the research area have been highlighted and should help to increase the representativeness of this modeling exercise. Although focusing on Sweden only, these results might be relevant to agriculture in regions of similar latitudes, especially the Nordic countries.
Data Availability Statement
The raw data supporting the conclusions of this article will be made available by the authors, without undue reservation.
Author Contributions
JM, UK, ML, MA, DP, and GB: conceptualization. JM, UK, MA, and DP: modeling and formal analysis. JM, UK, MA, GB, ML, MH, and DP: writing, review, and editing. All authors have read and agreed to the published version of the manuscript.
Funding
This work was supported financially by SLU Future Food which is a platform for interdisciplinary research and collaboration for sustainable food systems at the Swedish University of Agricultural Sciences (SLU).
Conflict of Interest
The authors declare that the research was conducted in the absence of any commercial or financial relationships that could be construed as a potential conflict of interest.
Acknowledgments
Acknowledgments are made to the APSIM Initiative, which takes responsibility for quality assurance and a structured innovation program for APSIM. APSIM is provided free for research and development use (see www.apsim.info for details). Figures 2–4 were created using the ggplot2 R package (Wickham, 2016). This manuscript has been released as a report at SLU Future Food (Morel et al., 2020a).
Supplementary Material
The Supplementary Material for this article can be found online at: https://www.frontiersin.org/articles/10.3389/fsufs.2021.665025/full#supplementary-material
References
Ainsworth, E. A., and Long, S. P. (2004). What have we learned from 15 years of free-air CO2 enrichment (FACE)? A meta-analytic review of the responses of photosynthesis, canopy properties and plant production to rising CO2: Tansley review. New Phytol. 165, 351–372. doi: 10.1111/j.1469-8137.2004.01224.x
Asseng, S., Martre, P., Maiorano, A., Rötter, R. P., O'Leary, G. J., Fitzgerald, G. J., et al. (2019). Climate change impact and adaptation for wheat protein. Glob. Change Biol. 25, 155–173. doi: 10.1111/gcb.14481
Bahri, H., Annabi, M., M'Hamed, H. C., and Frija, A. (2019). Assessing the long-term impact of conservation agriculture on wheat-based systems in Tunisia using APSIM simulations under a climate change context. Sci. Total Environ. 692, 1223–1233. doi: 10.1016/j.scitotenv.2019.07.307
Bindi, M., Palosuo, T., Trnka, M., and Semenov, M. (2015). Modelling climate change impacts on crop production for food security. Clim. Res. 65, 3–5. doi: 10.3354/cr01342
Boote, K. J., Jones, J. W., White, J. W., Asseng, S., and Lizaso, J. I. (2013). Putting mechanisms into crop production models. Plant Cell Environ. 36, 1658–1672. doi: 10.1111/pce.12119
Eckersten, H., Herrmann, A., Kornher, A., Halling, M., Sindhøj, E., and Lewan, E. (2012). Predicting silage maize yield and quality in Sweden as influenced by climate change and variability. Acta Agric. Scand. Sec. B Soil Plant Sci. 62, 151–165. doi: 10.1080/09064710.2011.585176
Elsgaard, L., Børgesen, C. D., Olesen, J. E., Siebert, S., Ewert, F., Peltonen-Sainio, P., et al. (2012). Shifts in comparative advantages for maize, oat and wheat cropping under climate change in Europe. Food Addit. Contam Part A 29, 1514–1526. doi: 10.1080/19440049.2012.700953
Ewert, F., Rötter, R. P., Bindi, M., Webber, H., Trnka, M., Kersebaum, K. C., et al. (2015). Crop modelling for integrated assessment of risk to food production from climate change. Environmental Modelling & Software 72, 287–303. doi: 10.1016/j.envsoft.2014.12.003
Hagman, J., Halling, M. A., Barrlund, M., and Larsson, S. (2018). Sortval 2018 - Stråsäd, Trindsäd Och Oljeväxter. Uppsala: Sveriges Lantbruksuniversitet. Available online at: https://www.slu.se/globalassets/ew/org/inst/vpe/resurser/sortprovning—ny/start/sortval_2018_ettariga_webb.pdf
Hogda, K. A., Karlsen, S. R., and Solheim, I. (2001). “Climatic change impact on growing season in fennoscandia studied by a time series of NOAA AVHRR NDVI data,” in IGARSS 2001 Scanning the Present and Resolving the Future. Proceedings. IEEE 2001 International Geoscience and Remote Sensing Symposium (Cat. No.01CH37217), Vol. 3 (Sydney, NSW), 1338–1340. doi: 10.1109/IGARSS.2001.976837
Holzwortha, D. P., HuthaPeter, N. I., deVoilb, P. G., Zurchera, E. J., Herrmanna, N. I., McLean, G., Chenu, K., et al. (2014). APSIM - Evolution towards a new generation of agricultural systems simulation. Environ. Model. Softw. 62, 327–350. doi: 10.1016/j.envsoft.2014.07.009
Keating, B. A., Carberry, P. S., Hammer, G. L., Probert, M. E., Robertson, M. J., Holzworth, D., et al. (2003). An overview of APSIM, a model designed for farming systems simulation. Euro. J. Agron. 18, 267–288. doi: 10.1016/S1161-0301(02)00108-9
King, M., Altdorff, D., Li, P., Galagedara, L., Holden, J., and Unc, A. (2018). Northward shift of the agricultural climate zone under 21st-century global climate change. Sci. Rep. 8:7904. doi: 10.1038/s41598-018-26321-8
Kumar, U., Morel, J., Bergkvist, G., Palosuo, T., Gustavsson, A.-M., Peake, A., et al. (2021). Comparative analysis of phenology algorithms of the spring barley model in APSIM 7.9 and APSIM next generation: a case study for high latitudes. Plants 10:443. doi: 10.3390/plants10030443
Kumar, U., and Parsons, D. (2020). Modelling Barley and Oat for Improved Decision Making in Northern Sweden. Available online at: https://www.slu.se/globalassets/ew/org/andra-enh/vh/rjn/slutrapporter/slutrapport-rjn-2_2018-modelling-barley-and-oats.pdf
Lana, M. A., Eulenstein, F., Schlindwein, S. L., Graef, F., Sieber, S., and von Hertwig Bittencourt, H. (2017). Yield stability and lower susceptibility to abiotic stresses of improved open-pollinated and hybrid maize cultivars. Agron. Sustain. Dev. 37:30. doi: 10.1007/s13593-017-0442-x
Macholdt, J., Gyldengren, J. G., Diamantopoulos, E., and Styczen, M. E. (2021). How will future climate depending agronomic management impact the yield risk of wheat cropping systems? A regional case study of Eastern Denmark. J. Agric. Sci. doi: 10.1017/S0021859620001045. [Epub ahead of print].
Manderscheid, R., and Weigel, H.-J. (2007). Drought stress effects on wheat are mitigated by atmospheric CO2 enrichment. Agron. Sustain. Dev. 27, 79–87. doi: 10.1051/agro:2006035
Morel, J., Kumar, U., Ahmed, M., Bergkvist, G., Lana, M., and Parsons, D. (2020a). Assessing the Impact of a Warmer and Drier Climate on Swedish Annual Crops. Umeå: Swedish University of Agricultural Sciences Available online at: https://pub.epsilon.slu.se/16843/1/morel_j_et_al_200408.pdf
Morel, J., Kumar, U., and Parsons, D. (2019). Laboratory Measured Characteristics of Six Swedish Soil Profiles. Available online at: https://data.mendeley.com/datasets/s3r4g8j6jj/1
Morel, J., Parsons, D., Halling, M. A., Kumar, U., Peake, A., Bergkvist, G., et al. (2020b). Challenges for simulating growth and phenology of silage maize in a nordic climate with APSIM. Agronomy 10:645. doi: 10.3390/agronomy10050645
Nkurunziza, L., Kornher, A., Hetta, M., Halling, M., Weih, M., and Eckersten, H. (2014). Crop genotype-environment modelling to evaluate forage maize cultivars under climate variability. Acta Agric. Scand. Sec. B Soil Plant Sci. 64, 56–70. doi: 10.1080/09064710.2014.885076
O'Grady, M. J., and O'Hare, G. M. P. (2017). Modelling the smart farm. Informat. Process. Agric. 4, 179–187. doi: 10.1016/j.inpa.2017.05.001
Olesen, J. E., Trnka, M., Kersebaum, K. C., Skjelvåg, A. O., Seguin, B., Peltonen-Sainio, P., et al. (2011). Impacts and adaptation of European crop production systems to climate change. Euro. J. Agron. 34, 96–112. doi: 10.1016/j.eja.2010.11.003
Ozturk, I., Kristensen, I. S., and Baby, S. (2018). Sensitivity of silage-maize to climate change in Denmark: A productivity analysis using impact response surface. Euro. J. Agron. 98, 55–64. doi: 10.1016/j.eja.2018.05.007
Palosuo, T., Hoffmann, M. P., Rötter, R. P., and Lehtonen, H. S. (2021). Sustainable intensification of crop production under alternative future changes in climate and technology: the case of the North Savo region. Agric. Syst. 190:103135. doi: 10.1016/j.agsy.2021.103135
Pelak, N., Revelli, R., and Porporato, A. (2017). A dynamical systems framework for crop models: toward optimal fertilization and irrigation strategies under climatic variability. Ecol. Model. 365, 80–92. doi: 10.1016/j.ecolmodel.2017.10.003
Pembleton, K. G., Cullen, B. R., Rawnsley, R. P., Harrison, M. T., and Ramilan, T. (2016). Modelling the resilience of forage crop production to future climate change in the dairy regions of Southeastern Australia using APSIM. J. Agric. Sci. 154, 1131–1152. doi: 10.1017/S0021859615001185
Phelan, D. C., Parsons, D., Lisson, S. N., Holz, G. K., and MacLeod, N. D. (2014). Beneficial impacts of climate change on pastoral and broadacre agriculture in cool-temperate Tasmania. Crop Pasture Sci. 65:194. doi: 10.1071/C.P.12425
R Core Team (2019). R: A Language and Environment for Statistical Computing. Vienna, Austria: R Foundation for Statistical Computing. Available online at: https://www.R-project.org/
Ray, D., West, P., Clark, M., Gerber, J., Prishchepov, A., and Chatterjee, S. (2019). Climate change has likely already affected global food production. PLoS ONE 14:e0217148. doi: 10.1371/journal.pone.0217148
Robredo, A., Pérez-López, U., de la Maza, H. S., González-Moro, B., Lacuesta, M., Mena-Petite, A., et al. (2007). Elevated CO2 alleviates the impact of drought on barley improving water status by lowering stomatal conductance and delaying its effects on photosynthesis. Environ. Exp. Bot. 59, 252–263. doi: 10.1016/j.envexpbot.2006.01.001
Rodriguez, D., de Voil, P., Hudson, D., Brown, J. N., Hayman, P., Marrou, H., et al. (2018). Predicting optimum crop designs using crop models and seasonal climate forecasts. Sci. Rep. 8:2231. doi: 10.1038/s41598-018-20628-2
Rötter, R. P., Höhn, J., Trnka, M., Fronzek, S., Carter, T. R., and Kahiluoto, H. (2013). Modelling shifts in agroclimate and crop cultivar response under climate change. Ecol. Evolut. 3, 4197–4214. doi: 10.1002/ece3.782
Rötter, R. P., Palosuo, T., Pirttioja, N. K., Dubrovsky, M., Salo, T., Fronzek, S., et al. (2011). What would happen to barley production in Finland if global warming exceeded 4°C? A model-based assessment. Euro. J. Agron. 35, 205–214. doi: 10.1016/j.eja.2011.06.003
Saxton, K. E., and Rawls, W. J. (2006). Soil water characteristic estimates by texture and organic matter for hydrologic solutions. Soil Sci. Soc. Am. J. 70:1569. doi: 10.2136/sssaj2005.0117
Schleussner, C.-F., Deryng, D., Müller, C., Elliott, J., Saeed, F., Folberth, C., et al. (2018). Crop productivity changes in 1.5 °C and 2 °C worlds under climate sensitivity uncertainty. Environ. Res. Lett. 13:064007. doi: 10.1088/1748-9326/aab63b
Silva, J. V., Reidsma, P., and Ittersum, M. K. (2017). Yield gaps in Dutch arable farming systems: analysis at crop and crop rotation level. Agric. Syst. 158, 78–92. doi: 10.1016/j.agsy.2017.06.005
Thivierge, M.-N., Jégo, G., Bélanger, G., Bertrand, A., Tremblay, G. F., Rotz, C. A., et al. (2016). Predicted yield and nutritive value of an alfalfa-timothy mixture under climate change and elevated atmospheric carbon dioxide. Agron. J. 108, 585–603. doi: 10.2134/agronj2015.0484
Thornton, P., Aggarwal, P., and Parsons, D. (2017). Editorial: Prioritising climate-smart agricultural interventions at different scales. Agric. Syst. 151, 149–152. doi: 10.1016/j.agsy.2016.12.007
Trnka, M., Rötter, R. P., Ruiz-Ramos, M., Kersebaum, K. C., Olesen, J. E., Žalud, Z., et al. (2014). Adverse weather conditions for European wheat production will become more frequent with climate change. Nat. Clim. Change 4, 637–643. doi: 10.1038/nclimate2242
van Ittersum, M. K., Cassman, K. G., Grassini, P., Wolf, J., Tittonell, P., and Hochman, Z. (2013). Yield gap analysis with local to global relevance—a review. Field Crops Res. 143, 4–17. doi: 10.1016/j.fcr.2012.09.009
Wang, J., Wang, E., Luo, Q., and Kirby, M. (2009). Modelling the sensitivity of wheat growth and water balance to climate change in Southeast Australia. Clim. Change 96, 79–96. doi: 10.1007/s10584-009-9599-x
Wickham, H. (2016). ggplot2: Elegant Graphics for Data Analysis. New York, NY: Springer-Verlag. Available online at: https://ggplot2.tidyverse.org
Wiklert, P., Andersson, S., and Weidow, B. (1983). Studier av Markprofiler I Svenska Åkerjordar: En Faktasammanställning. D. 7. Uppsala: Institutionen för markvetenskap, Sveriges lantbruksuniversitet.
Wilcke, R. A. I., Kjellström, E., Lin, C., Matei, D., and Moberg, A. (2020). The extremely warm summer 2018 in Sweden – set in a historical context. Dynam. Earth Syst. Models. 11, 1107–1121. doi: 10.5194/esd-2020-25
Keywords: APSIM crop model, annual crop, climate change, high latitudes, Nordic countries, yield change, risk of failure
Citation: Morel J, Kumar U, Ahmed M, Bergkvist G, Lana M, Halling M and Parsons D (2021) Quantification of the Impact of Temperature, CO2, and Rainfall Changes on Swedish Annual Crops Production Using the APSIM Model. Front. Sustain. Food Syst. 5:665025. doi: 10.3389/fsufs.2021.665025
Received: 06 February 2021; Accepted: 03 May 2021;
Published: 28 May 2021.
Edited by:
Adrian Unc, Memorial University of Newfoundland, CanadaReviewed by:
Keith Pembleton, University of Southern Queensland, AustraliaRafael Martinez-Feria, Michigan State University, United States
Copyright © 2021 Morel, Kumar, Ahmed, Bergkvist, Lana, Halling and Parsons. This is an open-access article distributed under the terms of the Creative Commons Attribution License (CC BY). The use, distribution or reproduction in other forums is permitted, provided the original author(s) and the copyright owner(s) are credited and that the original publication in this journal is cited, in accordance with accepted academic practice. No use, distribution or reproduction is permitted which does not comply with these terms.
*Correspondence: Julien Morel, anVsaWVuLm1vcmVsQHNsdS5zZQ==