- 1Food Systems Graduate Program, University of Vermont, Burlington, VT, United States
- 2Gund Institute for the Environment, University of Vermont, Burlington, VT, United States
- 3Department of Nutrition and Food Sciences, University of Vermont, Burlington, VT, United States
Farmers across the globe are experiencing compounding shocks that make evident the need to better understand potential drivers and barriers to strengthen adaptive capacity. This is especially true in the context of a disaster, where a disruption in the natural and built environment hinders livelihood strategies and exposes the underlying dynamics that perpetuate vulnerability to natural hazards. As such, the interconnections of structural and individual attributes must be considered when evaluating adaptive capacity. This paper uses a convergent mixed-methods approach to assess Puerto Rican farmers' actual and intended adoption of adaptation practices, in light of the obstacles they faced toward recovery after 2017's category four Hurricane Maria, to contribute to better understanding adaptive capacity. This study uses data from 405 farmers across Puerto Rico (87% response rate), surveyed 8 months after Maria by agricultural agents of the Extension Service of the University of Puerto Rico at Mayagüez. Quantitative data was assessed through negative binomial regressions (actual adoption) and generalized linear models (intended adoption), while qualitative data (reported obstacles) were analyzed through thematic analysis. This study found that almost half of farmers adopted an adaptation practice after Maria, and that in many cases, broader structures, such as systems of governance, farmers' social networks, and infrastructure, affect adaptive capacity more than individual perceptions of capacity. Future adaptation strategies and interventions, especially in the context of disaster, should consider the extent to which structural factors hinder individuals' ability to prepare for, respond, and recover from the impacts of these shocks. Our results show that there might be opportunity to enact new systems in light of catastrophic events, but this does not solely depend on individual actions. The mixed-methods approach used can inform future studies in better assessing adaptive capacity from a standpoint that incorporates individual and structural components.
Introduction
Farmers across the globe are facing multiple compounding shocks, such as devastating hurricanes and severe droughts. These impacts are likely to become more frequent or intense in a changing climate (IPCC, 2014; Zhang et al., 2020), and thus it is increasingly important to understand the barriers and drivers to strengthen farmers' adaptive capacity—the available resources or assets to mitigate, prepare for, counter, and recover from impacts (Brooks and Adger, 2005; Gallopín, 2006; López-Marrero, 2010; Wisner et al., 2012; Cinner et al., 2018; Barnes et al., 2020; Phillips et al., 2020). Since farmers are embedded within social-ecological systems, it is important to recognize that adaptive capacity is comprised of various determinants that may extend beyond the individual to the institutional or systemic levels: political regulations, poverty, vulnerability to extreme events, and others (Reed et al., 2013; Shinbrot et al., 2019; Doran et al., 2020). Hence, when evaluating adaptive capacity, its determinants must be considered across scales (Adger, 2006; López-Marrero, 2010).
This assertion is especially true in the context of a disaster, where a disruption of the built and natural environments, as well as to day-to-day livelihood activities, reflects what resources and abilities people have available to manage the situation (Quarantelli, 1992; Wisner et al., 2004, 2012; Adger, 2006; Clay et al., 2018). Disasters are social-historical products that highlight people's vulnerability to natural hazards, which to a great extent is driven by people's exposure and sensitivity to those shocks, as well as their adaptive capacity (Brooks and Adger, 2005; Gallopín, 2006; Smit and Wandel, 2006; Ribot, 2014). Decreasing exposure and sensitivity to natural hazards—the rate of being subject to impacts, and the degree of change due to impacts, respectively—is difficult in places that due to geophysical and geographical conditions experience a higher prevalence of natural hazards (Gallopín, 2006; Smit and Wandel, 2006; López-Marrero, 2010; López-Marrero and Wisner, 2012). Thus, focusing on strengthening adaptive capacity is a crucial step in decreasing vulnerability.
As such, to better understand the adaptive capacity of farmers in a disaster context from both the individual and societal level, this paper uses a mixed-methods convergent design (Creswell and Plano-Clark, 2018) to examine the intended and actual adoption of adaptation practices and strategies of Puerto Rican farmers in the aftermath of Hurricane Maria, in light of the obstacles they faced to post-hurricane recovery. Hurricane Maria was the strongest category four hurricane to hit Puerto Rico in 89 years, and the first one to make a direct impact in 19 years (Castro Rivera et al., 2018; Bang et al., 2019). The hurricane made landfall in September 20, 2017, and it triggered a disaster that made visible how social and political dynamics in Puerto Rico exacerbate vulnerability to natural hazards (Moulton and Machado, 2019; Bonilla, 2020). Almost 3,000 deaths are attributed to the lack of access to electricity, water, healthcare, and other basic needs after Maria's passage (Santos-Burgoa et al., 2018; Bonilla, 2020).
Prior to Maria, agriculture in Puerto Rico was experiencing advancements in production and its recognition as an important sector in a place where around 85% of food is imported (Comas-Pagán, 2014; Irizarry-Ruiz, 2016; Álvarez-Berríos et al., 2018). Hurricane Maria changed this trajectory; the Puerto Rico Department of Agriculture reported that 80% of agricultural infrastructure and production were lost due to the winds and rains of Hurricane Maria, which made landfall just 2 weeks after category five Hurricane Irma impacted the territory. Both storms were part of the 2017 Atlantic hurricane season, which surpassed meteorological standards and was the costliest season in record (Bang et al., 2019). Both hurricanes affected many islands in the Caribbean, making evident that improving adaptive capacity among island systems is key to furthering adaptation.
Island states and territories are known to face additional vulnerabilities to climate change because of characteristics, such as exposure to sea-level rise and constant shocks, coupled with their small economies and territories, isolation, and dependence on imports (Graham, 2012; IPCC, 2014; Scobie, 2018; Kim and Bui, 2019). The 2017 hurricane season made evident those underlying conditions, including Caribbean governance structures that reflect neocolonial relationships (Bang et al., 2019; Borges-Méndez and Caron, 2019; Bonilla, 2020), and perpetuate the vulnerability of social-ecological systems (Quarantelli, 1992; Ribot, 2014). Given the importance of local agriculture for island food security in the context of response and recovery from shocks, understanding farmers' adaptation to climate change in light of a disaster—the set of decisions and processes that allow them to secure agricultural production while safeguarding their livelihoods (Brooks and Adger, 2005; Jezeer et al., 2019; Shinbrot et al., 2019)–may provide us with a clearer picture of the interplay between individual and structural factors in adaptive capacity.
The adoption of agricultural practices and management strategies, such as farm diversification of products and energy sources, amongst others, allow farmers to offset impacts in a changing climate and are key to adaptations that can support livelihood outcomes such as food security (Caswell et al., 2016; Akhter and Erenstein, 2017; Niles and Salerno, 2018; Fernandez and Méndez, 2019; Anderzén et al., 2020). These actions can allow farming systems to either return to the prior state before the event (i.e., “bounce back”), or to transform into new systems that are better suited to changing climatic circumstances (i.e., “bounce forward”) (Payne et al., 2021). Either option, whether incremental (e.g., adopting cover crops) or transformative (e.g., changing from monoculture to diverse farming) is dependent on farmers' adaptive capacity—resources or assets farmers have access to, which play a key role in such decisions (Holt-Giménez, 2002; Caswell et al., 2016; Barnes et al., 2020; Wilson et al., 2020).
Adaptive capacity is multidimensional, and its determinants span from individual attributes, such as gender and financial assets, to material and governmental resources (Table 1). Approaching climate change adaptation by acknowledging adaptive capacity as a multidimensional component provides a framework to define what resources are needed to counter and recover from shocks in a given context (López-Marrero, 2010; López-Marrero and Yarnal, 2010; López-Marrero and Wisner, 2012). Understanding the role of how different capacities affect Puerto Rican farmers' actual and intended adoption of agricultural practices and management strategies after Maria, and their obstacles for recovery, can enable a more systematic assessment of the barriers and drivers to strengthen farmers' adaptive capacity, further providing us a better picture of how broader structures, beyond individual attributes, effect action (Rodríguez-Cruz and Niles, 2021).
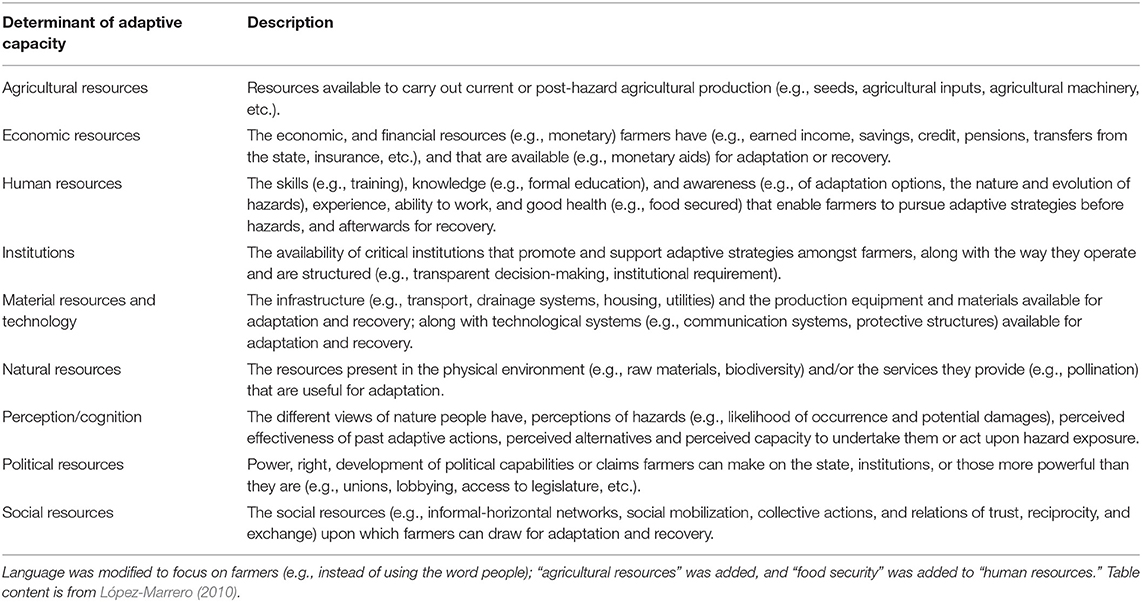
Table 1. Determinants of adaptive capacity as delineated by López-Marrero and Yarnal (2010).
Farmers' decision-making around climate change adaptation has been studied from various disciplinary perspectives (Prokopy et al., 2019; Ranjan et al., 2019; Foguesatto et al., 2020), with mixed conclusions on the extent to which adaptive capacities impact adaptation outcomes. For example, in contrast to studies in low and middle-income countries (e.g., Kassie et al., 2015), adoption of new behaviors among mainland US farmers is not highly dependent on natural and agricultural resources (e.g., land tenure, farm size, etc.) (Prokopy et al., 2019). Furthermore, social, governmental, and institutional resources, such as belonging to farmer networks, subsidies, and regulations, influence the degree to which farmers adopt new practices. For example, access to information sources through social or institutional networks of support that increase farmers' knowledge on what strategies benefit them, and how to carry them out, has been shown to be positive for farmers' adaptation (Dang et al., 2018; Bagagnan et al., 2019; Luu et al., 2019; Raza et al., 2019). Studies have also shown that psychological factors, such as perceived vulnerability and capacity, for example, play a role in farmers' adaptation decisions. Wang et al. (2019) found that perceived vulnerability and severity had an effect on Chinese farmer's intention to adopt pro-environmental practices, and Niles et al. (2016) found that perceived capacity linked to both intended and actual adoption of new practices among New Zealand farmers.
Though these studies have shown the importance of integrating different aspects of adaptive capacity, there is still a gap in triangulating the role of individual and structural aspects of adaptation behaviors (Foguesatto et al., 2020; Wilson et al., 2020; Rodríguez-Cruz and Niles, 2021). Much of the literature has either focused on the intention to adopt or actual adoption, and has not considered both within the same population (Niles et al., 2016). Furthermore, adaptation literature has been limited in intersecting individual (intrapersonal) and societal (interpersonal) components. Here we address these gaps by focusing on Puerto Rican farmers during their recovery period from Hurricane Maria and examine the multiple facets of adaptive capacity and their relationship to intended and actual adaptation practice adoption. This paper intends to contribute to current conversations on how to understand and approach adaptive capacity in a way that analyzes multiple components. We do not aim to assess the efficacy of the practices and strategies toward adaptation. Rather, we aim to provide a different methodological perspective that can improve our assessment of what may be needed to improve adaptive capacity.
As such, we ask the following: (1) What obstacles did farmers experience that thwarted the recovery of their farms after Hurricane Maria, and what determinants of adaptive capacity are reflected within them? (2) What were farmers' actual and intended adoption of agricultural practices and management strategies after Hurricane Maria? (3) What determinants of adaptive capacity explain actual and intended adoption, and how do they compare? (4) How do farmers' reported obstacles to recovery post-Maria compare with intention to adopt and adoption of farm management practices and adaptation strategies??
The literature in the Caribbean and Central America, regions affected by Atlantic hurricanes, has shown that adaptation is highly dependent on structural (social, governmental, and institutional) and income-related factors (economic, material and technological). Thus, we expect that variables reflecting these factors will be significant in both actual and intended adoption (H1). Furthermore, though research has shown that perceptual factors are not pivotal to Puerto Rican farmers' adoption of practices (Rodríguez-Cruz and Niles, 2021), we expect these factors to be related to intended practices (H2). Lastly, it is known that Hurricane Maria caused significant damage in Puerto Rico, and that subsequent recovery efforts failed to safeguard lives and wellbeing (Santos-Burgoa et al., 2018; Bonilla, 2020). Thus, we expect that farmers' self-reported obstacles will reflect the role of broader structures (governmental, institutional, and societal) in the recovery of their farms (H3), as well in the type of practices they actualized, and intended to carry out (H4).
Materials and Methods
Survey Sample
A mixed-methods survey, informed by previous studies (Spence et al., 2011; Haden et al., 2012; Niles et al., 2015; Niles and Mueller, 2016; Singh et al., 2017), was developed in English, and translated to Spanish, to capture Puerto Rican farmers' perceptions and opinions around their experience with Hurricane Maria and climate change. The overall objectives of the main project focused on understanding farmers' adaptation and food security outcomes in light of farmers' recovery from Hurricane Maria (Rodríguez-Cruz and Niles, 2020). The study received approval by the Institutional Review Board of the University of Vermont in December 2017.
A pilot with a pool of diverse farmers (n = 31) was carried out in February 2018, and results were shared with partners at the Extension Service of the University of Puerto Rico at Mayagüez. The survey received minimal language and structure corrections.
Data used for the present study were a sub-set of survey questions (both closed response and open-ended), concerning farmers' demographics, questions that reflected adaptive capacity resources, adaptation perceptions, actual and intended practices, and reported obstacles. Some variables were converted (e.g., Likert scale to binary) to better group individuals, and because some had concentrated answers in two items (e.g., agree and strongly agree). Table 2 shows independent variables used in this study, and Table 3 shows agricultural practices and management strategies asked about in the survey.
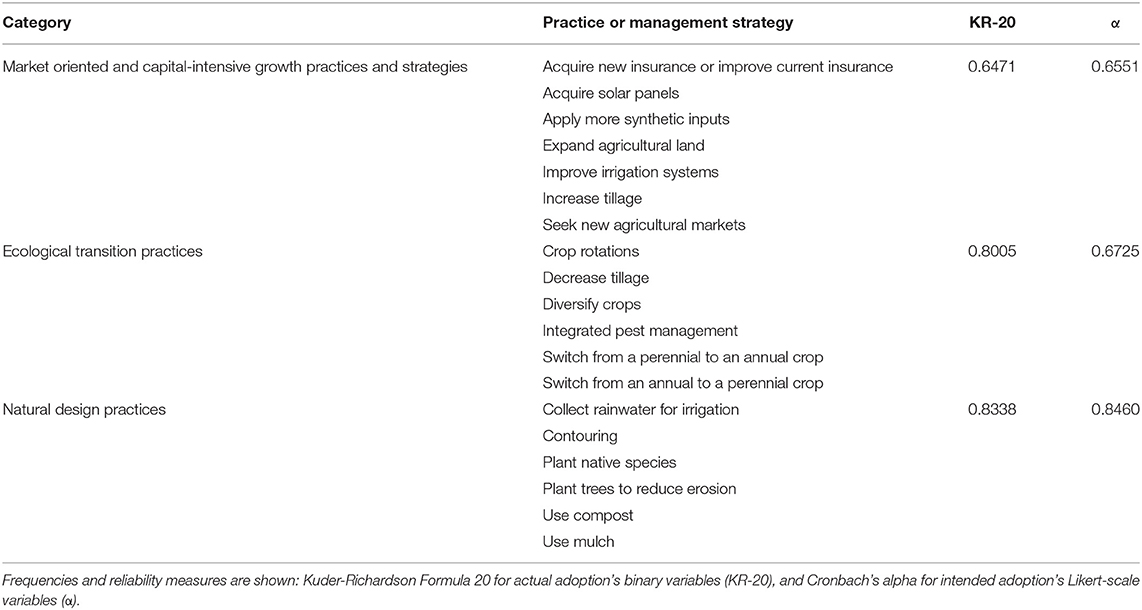
Table 3. Categorized agricultural practices and management strategies to adapt to future extreme weather events like Hurricane Maria asked to farmers.
The survey was deployed by local agricultural agents of the Extension Service who acted as enumerators of the survey, between May and July 2018, 8 months after Hurricane Maria. To access a diverse and substantial number of farmers, 440 surveys were distributed in the five regions that the Extension Service covers across Puerto Rico: Arecibo, Caguas, Mayagüez, Ponce, and San Juan. One hundred surveys were sent to each of the administrative offices of Caguas, Ponce, and San Juan; 70 were sent to each in Arecibo and Mayagüez, as per recommendation of Extension personnel. Agricultural agents then randomly surveyed farmers that were connected to Extension or had received services from it in municipalities of each region. This approach was recommended by Extension colleagues to access a diverse range of farmers (e.g., mixed, banana, plantain, dairy, poultry, etc.) from across Puerto Rico.
Place and Population
Puerto Rico is the smaller of the Greater Antilles of the Caribbean. It is an unincorporated territory of the United States, with a population of ~3.3 million people (US Census Bureau, 2020). As most islands in the region, Puerto Rico has seen a decrease in farms and food production since the 1990s due to trade liberalization and unbalances, economic situations, influx of imports, and other external and internal factors that make local food production and access difficult (Weis, 2007; FAO, 2014; Lowitt et al., 2015; Irizarry-Ruiz, 2016). While it produced about 40% of its food needs in the 1980s, Puerto Rico currently only produces around 15% (Carro-Figueroa, 2002; Gould, 2015; Irizarry-Ruiz, 2016; Gould et al., 2017). The territory is also undergoing a social and political crisis due to high debt (Bueno, 2017; Félix and Holt-Giménez, 2017; Bonilla, 2020). Within that context, the agricultural sector was experiencing improvements in production, access to local markets, and other opportunities prior to Maria (Comas-Pagán, 2014; Irizarry-Ruiz, 2016; Gould et al., 2017). Governmental and community-based efforts were focused on supporting current and new farmers before 2017's hurricanes. These efforts were halted by the impacts of both hurricanes Irma and Maria. The Puerto Rico Department of Agriculture (2018) reports that these two hurricanes caused $2 billion in damages, Maria being the most significant of the two in terms of agricultural losses ($228 million in production losses, and $1.8 billion in infrastructural losses). For example, reports indicate that the plantain sector suffered $72 million in damages, while the banana sector suffered $19 million. Other heavily affected sectors were coffee ($18 million), dairy ($14 million), and poultry ($6 million).
Though both impacts decimated Puerto Rico's agricultural sector, farmers have experienced a significant quantity of natural hazards since 2017, such as intense storms, and severe droughts (Gould, 2015; Díaz et al., 2018; López-Marrero and Castro-Rivera, 2018, 2019; Rodríguez-Cruz and Niles, 2021). Even a category one hurricane can easily damage local agriculture. Many of Puerto Rico's high value crops, such as coffee, bananas, and plantains, are very susceptible to temperature change and moderate winds. Moreover, important farmland is located in coastal areas, which is susceptible to erosion, and seawater intrusion to aquifers (Díaz et al., 2018). US Congress' Fourth National Climate Assessment (2018) concluded that rainfall patterns will change, and water availability will likely decline for Puerto Rico, coinciding with rising temperatures that contribute to the occurrence of recurring droughts in the future. Those impacts are occurring simultaneously with stronger storms (Díaz et al., 2018).
Puerto Rico's farmers produce mainly for domestic markets, and work small to medium farms according to the USDA. Subsistence farming is not typical in Puerto Rico. The 2018 census states that most farmers in the territory (or principal operators) have a household income < $20,000, significantly less than the US average, which exceeds $60,000 (USDA ERS, 2020). Puerto Rico's average household income is $20,539 (US Census Bureau, 2020). The USDA defines a Puerto Rican farm as a location where $500 or more of agricultural products are produced or sold. Between 2012 and 2018, Puerto Rico saw a decrease in farms (USDA NASS, 2020). There were 13,159 farms in 2012—when the last census was carried out–, with, 8,230 farms reported in the current census (USDA NASS, 2020). Today, most farms are <100 cuerdas (Puerto Rico's traditional land measure) or 97 acres (an average of 59.3 cuerdas), and are mostly family or individual farms. There are large farms that run extensive monocultures, but many of the small-medium farms produce a diverse array of agricultural products (Álvarez-Febles and Félix, 2020). It is important to note that many other farms, such as community-supported agriculture projects and others, are not counted in the census or are not directly linked to the Puerto Rico Department of Agriculture.
Qualitative Analysis
The survey asked farmers to state at least three obstacles faced during the recovery of that their farming operations. Farmers' responses to this open-ended question were analyzed using double coding through thematic analysis with a priori codes (Creswell, 2014, 2016). Responses were translated from Spanish into English by the first author, and then transcribed to a Microsoft Word document, which was uploaded to NVivo v.12 (QSR International, 2019). Given the purposes of this study, an a priori coding frame, accompanied by code definitions, was used to categorize the reported obstacles into nine nodes (or themes) following the nine determinants of adaptive capacity adapted from López-Marrero (2010) shown in Table 1. Authors LARC and MM (coders) first agreed upon the codes and coded the first 10 responses together. In order to establish transparency and consistency within the coding (O'Connor and Joffe, 2020), intercoder reliability (ICR) was evaluated following a first round of coding by quantifying the degree of consensus using percentage of agreement. More than 90% is considered highly reliable (Lavrakas, 2008). Two nodes, agricultural resources and economic resources, did not score more than 90% agreement after the first round of coding. Thus, after the coders discussed divergences and reached consensus, a second round of coding was undertaken. The second assessment successfully achieved more than 90% ICR in all nodes (Supplementary Table 1). Codes with the highest frequency within each theme, and quantification of such themes to identify coverage and percentages, were evaluated using Nvivo 12's hierarchy chart wizard and word cloud function.
Quantitative Analysis
The survey asked farmers to state the agricultural practices and management strategies that they were considering adopting in the future or that they had adopted since Hurricane Maria (~8 months prior to the survey) in a close-ended question with pre-coded responses. The responses included a list of practices which list of practices was developed based upon conversations and recommendations from colleagues at the Extension Service and at the University of Vermont with expertise in agriculture and climate change. The survey asked, “Which of these agricultural practices and management strategies, if any, might you adopt in the near future to adapt to future extreme events like Hurricane Maria?” (Table 3). Hereafter, these practices and strategies will be referred to as “adaptation practices.” The 22 practices were included as a list in a table. The first column stated, “Currently in use” (binary, yes or no), and the subsequent columns represented a 5-point Likert scale for adoption (from very unlikely to very likely). The binary column was used to assess actual adoption, and the Likert scale to assess intended adoption. The list was also intended to include practices recommended for adaptation or for conservation of natural resources, as well as other conventional or common practices in Puerto Rico and the contiguous United States. It is important to note that the objective was to assess what practices or strategies farmers understand to be helpful for adaptation, and not to evaluate if those decisions are adaptive or maladaptive. Variables that had n <20 were excluded from the analysis (e.g., “stop farming” and “forage conservation”), since they represented <5% of total respondents.
Actual Adoption
Actual adoption of agricultural and management strategies following Hurricane Maria was assessed through binary variables where farmers indicated “currently in use,” as noted above. We used Kuder-Richardson-20 Reliability Tests in Stata 15.1, which test internal consistency or scale reliability of binary items (Kuder and Richardson, 1937) and range from 0 to 1 in ascending reliability (Table 4). This test is similar to assessing Cronbach's alpha, which evaluates internal consistency of scale variables (Nunnally, 1978). Two categories had KR-20s > 0.80, which are acceptable determining internal consistency or reliability of a group of items, and one had a coefficient > 0.60, which is reasonable (Nunnally, 1978). Each groups' variables were summed to create three new aggregate count variables for analysis: (1) Market oriented and capital-intensive growth, (2) Ecological transition practices, and (3) Natural design, as well as fourth aggregate count variable of all practices combined (Table 3). The first group had seven practices; the second and third groups were composed of six.
Model development considered distribution of the count variables. We implemented a Poisson regression to test the model, but model assumptions were not met on multiple factors (Likelihood ratio test showed over dispersed data: LR test of alpha = 0: chibar2(01) = 649.22, Prob ≥ chibar2 = 0.000, and the Poisson regression assumption of identical means and variances was not met). Instead, we used a negative binomial regression (nbreg) to fit over-dispersed data. We developed four nbreg models, with clustered errors for municipalities, utilizing Stata 15.1.
Intended Adoption
Intended adoption was evaluated through scale variables (5-point Likert) of the list of practices and management strategies. Three scale variables were created to understand likelihood of intended adoption, using the same categorization of actual adoption scales, with Cronbach alpha acceptable at >0.65 (Nunnally, 1978). As with all of the actual adoption variables, a single variable was created with all the intended adoption practices (alpha = 0.86). We used generalized linear models with clustered errors around municipalities to account for spatial correlation (Nichols and Schaffer, 2007). Distribution of scale variables show similitude to both Gaussian and Gamma distributions. In order to choose the best family distribution to build the models, Akaike information criterion (AIC) and the Bayesian information criterion (BIC) were used to compare across generalized linear models with either Gaussian or Gamma distributions. The models yielded better fit with Gaussian distributions (Supplementary Table 2). Thus, the generalized linear models used Gaussian as the family choice, and “Identity” as the link choice.
Results
Descriptive Statistics
A total of 405 farmers answered the survey through Extension enumerators, resulting in an 87% response rate. Farmer and farm characteristics, which were categorized under different adaptive capacity resources, varied across respondents (Table 4). On average, farmers surveyed had 58 cuerdas (56.3 acres or 23 hectares), were 54 years old, and had been farming for 20 years; results that align with census data for Puerto Rican farmers (USDA NASS, 2020). The majority of respondents were male (86%) and reported a household income of $20,000 or more (64%), which also aligns with recent census data. Nevertheless, we had an overrepresentation of bona fide farmers (53%). Farmers reported being connected to an average of two organizations or institutions (min = 0, max = 11), that provide them support around climate change adaptation, and reported selling their products to one of the listed venues, on average (min = 1, max = 5).
Qualitative Analysis Results
While 345 farmers (90%) responded that they had faced significant obstacles toward recovery, only 333 provided responses to the open-ended question. Farmers identified many obstacles to their recovery related to their adaptive capacity (agricultural resources, economic resources, human resources, institutions, material resources and technology, natural resources, perception/cognition, political resources and social resources) (Table 1). Table 5 shows the coverage percentages of each of the identified themes, and also displays the most prevalent references within each theme. Obstacles most mentioned by farmers were related to institutions and institutional support (27.0%), material resources and technology (26.1%), economic resources (26.2%), and agricultural resources (24.3%). Obstacles that fell under themes of perception/cognition (0.07%), natural resources (2.6%), and social resources (4.3%) were the least mentioned. Within each theme, we also evaluated the most prevalent references within a theme.
In addition, from the cloud analysis, we can see that the top 10 words mentioned in farmer responses to our open-ended question regarding their top obstacles to recovery overall were: “lack,” “insurance,” “seeds,” “agricultural,” “electrical,” “energy,” “economic,” “aid,” “laborers,” and “water.” Many of these words reflected resources or structural components of a system (e.g., electricity, energy, aid) that are often related to institutional support.
Quantitative Analyses Results
Actual Adoption Results
Figure 1 shows farmers' reported actual adoption and management practices. Overall, the top five practices implemented after Hurricane Maria were: integrated pest management (n = 97, 24.4%), crop rotation (n = 84, 21.2%), crop diversification (n = 78, 19.6%), contouring (n = 68, 17.1%), and composting (n = 65, 16.2%). We find that 49% of farmers adopted any new practices after Hurricane Maria.
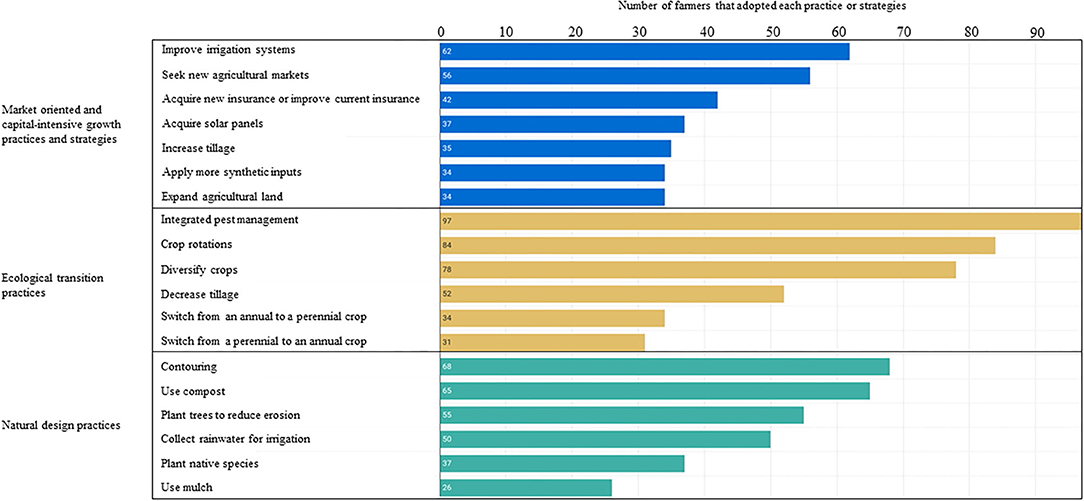
Figure 1. Farmers' reported actual adoption of adaptation practices and strategies after Hurricane Maria.
Table 6 shows results with significance for the four models that evaluated the relationship between actual adoption outcomes and adaptive capacity resources or assets. Supplementary Table 3 shows full model results. In the first model (actual adoption of all practices) we found that farmers with higher levels of education (β = 0.5780, IRR = 1.7824) and those that reported total loss of their farms (infrastructure and production) due to hurricane winds, rain, and landslides (β = 0.6665, IRR = 1.9474), were more likely to report higher number of practices adopted after Hurricane Maria (Table 6). We expected that variables around structural and financial assets, such as social, agricultural, economic, and material resources would be significant. These results were not aligned with that expectation (H1).
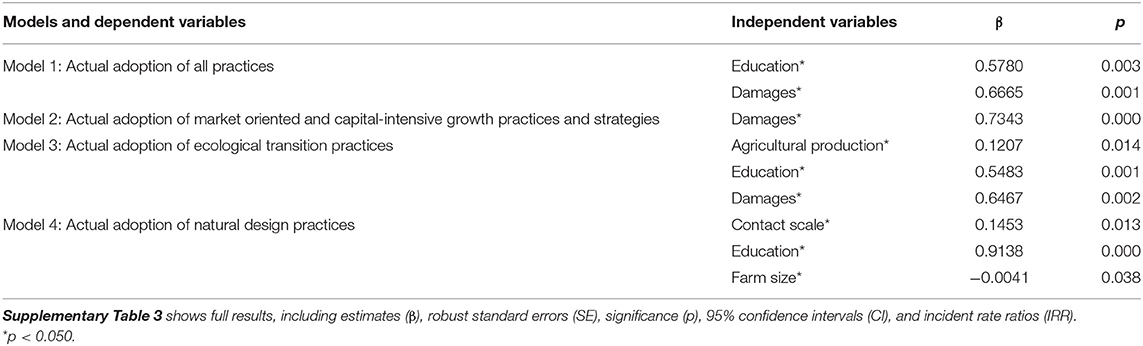
Table 6. Significant results for four separate negative binomial regression models predicting actual adoption of farm and management practices.
In examining the specific types of market oriented and capital-intensive growth practices and strategies adopted (Model 2), we found that total farm loss (damages) was the only significant variable (β = 0.7343, IRR = 2.0840, p < 0.05) (Table 6). This result is counterintuitive because no variable related to economic or material resources was found significant (H1). In model 3, actual adoption of ecological transition practices, we found that the number of agricultural products produced (β = 0.1207, IRR = 1.1282), farmers' levels of formal education (β = 0.5483, IRR = 1.7303), and reporting total loss of farms (β = 0.6467, IRR = 1.9093) were significant predictors of adoption (p < 0.05) (Table 6).
Finally, we found in the fourth model (Actual adoption of natural design practices) that the number of reported organization or institutions that have provided services to farmers (contact scale) (β = 0.1453, IRR = 1.1564), education (β = 0.9138, IRR = 2.4936), and farm size (β = −0.0041, IRR = 1.0000), were significantly correlated (p < 0.05) with conservation practice adoption after Hurricane Maria (Table 6). These results demonstrate that farms with higher number of contacts and higher education (or greater social and human capital) were more linked to adoption, while larger farms were less likely to have adopted conservation practices.
Intended Adoption Results
Figure 2 shows farmers' intended adoption practices and strategies, and Supplementary Table 4 shows the tabular results. Respondents' top reported practices and management strategies to adopt in the future (likely and very likely to adopt) were: integrated pest management (80.5%), diversification of crops (78.0%), seeking new agricultural markets (74.8%), acquiring new insurance or improving current insurance (72.4%), and crop rotations (71.4%). In general, intention to adopt results contrasted with those of actual adoption.
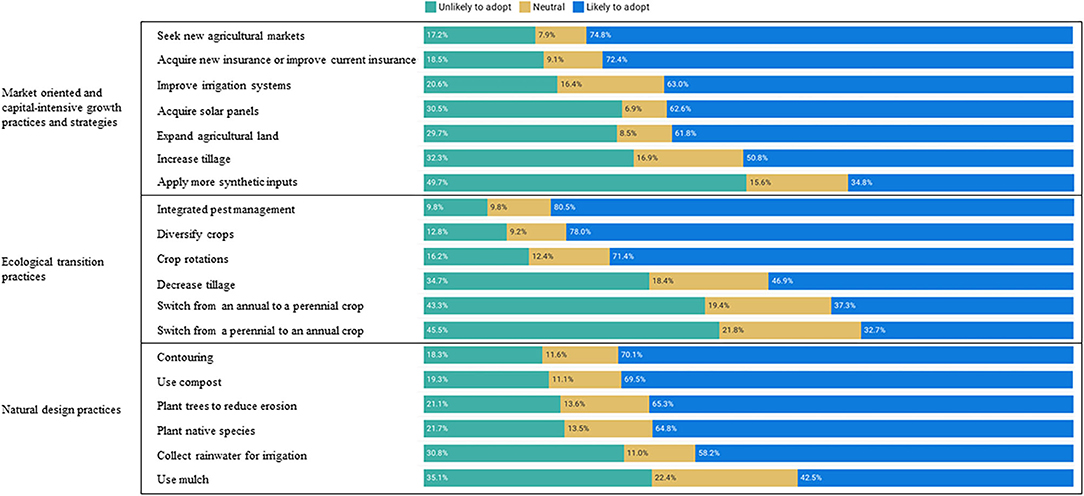
Figure 2. Farmers' intended adoption of adaptation practices and management strategies after Hurricane Maria.
Four generalized linear models were carried out to assess intended adoption outcomes (Table 7). Full model results are shown in Supplementary Table 5, while significant results are shown in Table 7. We found no significant variables in Model 5 (Intended adoption across all practices and management strategies). In Model 6 (Intention to adopt market oriented and capital-intensive growth practices and strategies), perceived capacity significant (β = 0.2343, p < 0.05) was correlated with higher adoption intention (Table 7). In the 7th model (Intended adoption of ecological transition practices) being a bona fide farmer (β = −0.3243, p < 0.05) was negatively correlated with intention to adopt, meaning that those that reported being part of that program of the Puerto Rico Department of Agriculture, had lower intention rates to adopt such practices (Table 7). In the last model (8), exploring intended adoption of natural design, two variables were significant (p < 0.05). Producing a higher number of agricultural products (Agricultural production) was correlated with higher intention to adopt conservation practices (β = 0.0902) while reporting a total loss (damages) (β = −0.2663) was negatively correlated with intention to adopt those practices.
Discussion
This paper assessed Puerto Rican farmers' actual and intended adoption of adaptation practices and management strategies in light of the obstacles they faced toward recovery after Hurricane Maria. It aimed to understand potential barriers and drivers for strengthening adaptive capacity through a mixed-methods approach, in order to provide a new approach to understanding adaptive capacity in a disaster context. We find that drivers for actual adoption vary from factors related to intended adoption of adaptation practices, and that almost half of all farmers in our survey had actually adopted a practice or strategy in the 8 months since Hurricane Maria. Furthermore, we find that the majority of farmers faced significant obstacles in their recovery, especially with institutional support, economic resources, and access to material resources and technology. Combining quantitative and qualitative data provided a richer understanding of how individual and structural factors intersect and reflect adaptive capacity.
Contrary to our expectations (H1), variables related to governmental, institutional, social, economic, and material resources were not the principal drivers for both actual and intended adoption. Instead, facing a total loss, and having a higher level of formal education were most related to actual adoption of adaptation practices. Furthermore, we did not find that perception factors were significantly related to intended adoption, rejecting H2. Instead, intention to adopt had varying factors across the different categories of practices and management strategies. Although variables used around the aforementioned resources were not significant, qualitative data analysis suggests that lack of broader structures of support, such as expected and timely aid, insurance payments, and access to services and supplies should be considered in farmers' decision-making around adaptation and recovery (H3 and H4). These findings reflect how we might include other questions in future surveys exploring disaster recovery. Future studies should consider how variables often used to assess the determinants of adaptive capacity, such as the ones used in this study, might not provide the nuanced information specific to a disaster context.
One of the major drivers for actual adoption in all models, except for natural design practices (Model 4), was “total loss.” This contrasts with research in Central America with tropical agriculture farmers subjected to Atlantic hurricanes, where farmers' adoption of new practices was not significantly driven by damage experiences from extreme weather events, likely because of pre-existing low adaptive capacity (Harvey et al., 2018). Nevertheless, experiencing risk or climate-related impacts has been found to be a precursor of adaptation (Salerno et al., 2019). Farmers in our study that reported total loss were more likely to report a higher number of practices adopted overall, and in the adoption of market oriented and capital-intensive growth practices and strategies, as well as higher adoption of ecological transition practices. These findings are critically important for considering opportunities to rethink agricultural systems, and provide evidence that farmers may be willing to reconsider transforming their farming systems after experiencing significant damages that change their farming landscapes.
The finding that higher levels of formal education were linked to all actual adoption models, except market oriented and capital-intensive growth practices and strategies (Model 2), suggests that human assets may open doors to access other resources important for recovery and adaptation, likely through enabling formation of ties that increase social and structural support (López-Marrero and Wisner, 2012; Kassie et al., 2015; Caswell et al., 2016; Shah et al., 2019). For example, Model 4 showed that education was positively linked to adoption of natural design practices, as well as access to information sources. These findings align with Caribbean research suggesting that farmers who have external support are likely to adopt practices that support the environment, while sustaining their production (Lowitt et al., 2015; Saint Ville et al., 2016; Paul et al., 2017). Research outside the Caribbean supports this as well (Bagagnan et al., 2019; Žurovec and Vedeld, 2019).
These results suggest that being able to adopt practices to adapt to climate change or re-envision agricultural systems may occur among farmers with higher levels of human capacity. Future studies should focus on farm recovery processes after a significant shock that alters the working landscape to understand decision-making processes in rebuilding the system. Taken together, this suggests that total loss events, while catastrophic, do present opportunities for reinvention, if people have access to the necessary resources. These results further highlight the need for institutional support and capacity for farmers without formalized education, or social networks.
Our results also reflect other research showing that actual and intended adoption may not be driven by the same variables (Niles et al., 2016). While we expected (H2) to see perceived capacity be a notable factor predicting intended adoption as reflected in the Theory of Planned Behavior (Ajzen, 1991), as well as perceived vulnerability, we only find this significant in intention to adopt ecological transition practices (Model 6). These results further support that perceptual factors around climate change may not be pivotal in advancing adaptation in places where shocks are consistently experienced (Rodríguez-Cruz and Niles, 2021). On the other hand, being a “bona fide” farmer decreased likelihood of intending to adopt ecological transition practices (Model 7), which may help in increasing farmers likelihood of recovering their farms after a hurricane (Holt-Giménez, 2002; Rosset et al., 2011). This finding was counterintuitive since bona fide farmers are recognized officially by the government, which often provides them access to governmental and institutional resources. However, given the large number of farmers who reported institutional obstacles for recovery, it is possible that bona fide farmers did not receive benefits that might otherwise have been available.
Results from the qualitative analysis highlighted that most of the obstacles reported by farmers stemmed from mechanisms of support (e.g., insurance payments, governmental aid, etc.) that were not available for a significant period of time following Hurricane Maria, varying from several months up to a year. Farmers across all regions of Puerto Rico voiced the challenges they experienced when attempting to access governmental agencies, services, and materials and supplies needed to repair their farms and recover, physically and financially, from the effects of the hurricane. These were exacerbated by Puerto Rico experiencing the longest blackout in the modern history of the United States; communications were downed, and many regions of Puerto Rico only received restored power and water months after the hurricanes' landfall (Masters and Houser, 2017; Bonilla, 2020). Farmers also noted these obstacles as the third most common. Furthermore, our analysis suggests that farmers also faced challenges when accessing social networks of support, likely further challenged by the lack of material resources and technology. Perfecto et al. (2019) assessed how coffee farms' management, whether incorporating agroforestry or performing an intensive style, related to the degree of damages experienced and farm recovery after Maria. The study found that coffee farmers' recovery after Maria was potentiated by assistance from their social and community networks of support. And that management style may come secondary in a catastrophic context (Perfecto et al., 2019). Nevertheless, our qualitative analysis suggests that farmers may have been constrained in accessing such networks, which likely affected their overall capacity for recovery. Furthermore, the analysis reflected farmers disappointment on the state, and unmet expectations regarding aid in a catastrophic event.
The lack of institutional, economic, and social support likely affected the way that farmers perceived the practices necessary to adopt to overcome future challenges. We found that one of the top intended future practices was to acquire new agricultural insurance or improve current insurance. Agricultural insurance is one key risk management tool that farmers in the US use to offset climate-related impacts (Claassen et al., 2017; Reyes et al., 2020), but this insurance may not be aligned with needs of farmers, the impacts they face, or their farming systems. On the other hand, it could be that insurance dynamics (e.g., making payments, answering claims, etc.) might not be adequately equipped to deal with emergencies such as the one triggered by Hurricane Maria. Mainland US research has demonstrated regional differences in the role of insurance as a risk management tool (i.e., important in Midwest, less so in New England) (Mase et al., 2017; White et al., 2018). In Puerto Rico, agricultural insurance is mostly managed by the Corporación de Seguros Agrícolas (CSA) of the local Department of Agriculture. Obtaining payments from this insurance was specifically mentioned numerous times as an obstacle in our analysis. For example, one farmer noted, “I had about an acre of yautía insured, and the insurance paid after seven months. I could not recover any of the yautía.” These results indicate that while insurance likely would have enabled increased capacity for farm recovery, it too faced many barriers, which prevented farmers from receiving the money from their insurance claims in a timely manner. This finding also suggests that in the aftermath of Maria, or in the context of disaster, where “normal” means of communication and accessing resources are disrupted, bureaucracy may not have the capacity to manage these challenges. Thus, there is an important need to improve agricultural insurance delivery in future disaster contexts, especially if more farmers intend to invest in these services.
Our study further suggests that broader structures, such as systems of governance, farmers' social networks, in relation to infrastructure, policy, and public health, play a significant role in farmers' adaptive capacity. Hurricane Maria, as a disaster, made evident that Puerto Rico's political and social characteristics must be taken into account when aiming to understand adaptive capacity.
We note several limitations of our study, all of which are important for future research. First, we did not ask farmers if they had insurance prior to Maria, so we do not know if their insurance adoption is new or additional. Nevertheless, the survey did ask farmers if they had insurance at the time (8 months after landfall), and 53% stated that they did. Most of them reported that their insurance was with the Corporation of Agricultural Insurance of the Puerto Rico Department of Agriculture. Much of this paper's qualitative data indicated that farmers had insurance at the time of Maria, and show the difficulties experienced in assessing the funds. Future research could look more deeply to the extent to which agricultural insurance in Puerto Rico relates to adaptive capacity outcomes. Second, we are assuming that reported actual adoption was indeed only adopted after Maria, and not just a continuation of practices prior to the hurricane. The table in which they reported actual adoption practices specified “currently in use,” though we did not ask about pre-hurricane adoption. Nevertheless, the survey question asked about new practices for future adoption (“Which of these agricultural practices and management strategies, if any, might you adopt in the near future to adapt to future extreme events like Hurricane Maria?”). Thus, we assumed those reported practices were only actualized after Maria. Third, we did not include in the models the type of farming system (e.g., dairy, mixed, coffee, etc.), and instead use a proxy for diversity (number of products). This was done because many production systems in Puerto Rico are already diversified, making it challenging to assign farmers to a specific category. Furthermore, even within some categories (e.g., fruit/vegetable farmer), systems can vary significantly from annual to perennial. Lastly, we had an overrepresentation of bona fide farmers-−53% in our study, while 24% overall in Puerto Rico as reported by past Secretary of Agriculture in 2019, Carlos Flores (Díaz Rolón, 2019)—, despite most other demographics consistent with census data. This may be the result of selection bias through Cooperative Extension, which may have stronger connections with bona fide farmer networks.
Conclusion
This study assessed how various determinants of adaptive capacity reflect on Puerto Rican farmers' actual and intended adoption of adaptation practices, in light of the obstacles they faced toward recovery after 2017's Hurricane Maria. Our results suggest that, in many cases, broader structures, such as systems of governance, farmers' social networks, and infrastructure, affect adaptive capacity more than individual perceptions or capacity assets. We find that experiencing a total loss, appears to provide a window of opportunity for reinventing agricultural systems, as evidenced by the fact that farmers who faced a total loss adopted the most actual adaptation practices. Importantly, farmers with higher education were also more likely to adopt more adaptation practices, suggesting that capacity to change farming systems after a total loss is related to human capital. These results suggest that catastrophic events like Hurricane Maria, while devastating, do provide opportunities for change and resilience; but being able to take advantage of those opportunities is related not only to the human capital of an individual farmer, and their social networks, but the institutional and infrastructure capacities that are in place for recovery. Absent either, agricultural resilience may be challenging to achieve, or slow at best. Thus, working to improve both individual and structural factors that affect adaptive capacity are both likely to lend themselves toward greater adoption of adaptation practices, which would, in turn, improve resilience of farm systems under future shocks. Lastly, our study shows that a mixed-methods approach into understanding adaptive capacity provides nuanced information that might not be captured in quantitative model assessments alone. Future studies should further integrate qualitative and quantitative data to improve our understanding on the role of broader structural components in individual adaptive capacity outcomes.
Data Availability Statement
The data sub-set used for this study can be available upon request to the corresponding author.
Ethics Statement
This study was approved on December 2017 by the Committees on Human Subjects Serving the University of Vermont and the UVM Medical Center at the Research Protections Office approved our study on 04-Dec- 2017. The study (CHRBSS: 18-0258) received Exemption Category 2. Consent was included in survey booklet, and was facilitated orally through enumerators. Written informed consent for participation was not required for this study in accordance with the national legislation and the institutional requirements.
Author Contributions
LR-C: wrote manuscript draft, conceptualized study, quantitative analysis, qualitative analysis, and project liaison. MM: reviewed and edited manuscript, conceptualized study, and qualitative analysis. MTN: reviewed and edited manuscript, conceptualized study, quantitative analysis, and funding allocation. All authors contributed to the article and approved the submitted version.
Funding
This research was internally funded through the College of Agriculture and Life Sciences and Food Systems Graduate program of the University of Vermont. MTN allocated funding.
Conflict of Interest
The authors declare that the research was conducted in the absence of any commercial or financial relationships that could be construed as a potential conflict of interest.
Acknowledgments
We acknowledge the farmers who answered the survey, and the agricultural agents that enumerated the survey. This study would not have been possible without the collaboration of the Extension Service of the University of Puerto Rico at Mayagüez and its personnel, and without the leadership of Aníbal II Ruiz-Lugo, Assistant Dean of the Extension Service, who assisted with the logistics on-site. We are grateful with Ms. Maritzabel Morales, who oversaw the digitalization of surveys, and with Ms. Olivia Peña, who assisted in data entry. Much thanks to the following colleagues for providing support in different steps of this study: Alissa White, Aura M. Alonso-Rodríguez, Amy Trubek, Carolyn Hricko, Catherine Horner, David Conner, Diana Hackenburg, Gabriela Buccini, Georges Félix, Guang Han, Jorge Ruiz-Arocho, Martha Caswell, Natalia Aristizábal, Nora Álvarez-Berríos, Ollin Langle-Chimal, V. Ernesto Méndez, Victor Izzo, Teresa Mares, and Vivian Carro-Figueroa. We also acknowledge the two reviewers that contributed in improving this paper, and the College of Agriculture and Life Sciences and the Food Systems Graduate Program of the University of Vermont for providing funding in a timely manner to carry out this study.
Supplementary Material
The Supplementary Material for this article can be found online at: https://www.frontiersin.org/articles/10.3389/fsufs.2021.662918/full#supplementary-material
References
Adger, W. N. (2006). Vulnerability. Glob. Environ. Change 16, 268–281. doi: 10.1016/j.gloenvcha.2006.02.006
Ajzen, I. (1991). The theory of planned behavior. Organ. Behav. Hum. Decis. Process. 50, 179–211. doi: 10.1016/0749-5978(91)90020-T
Akhter, A., and Erenstein, O. (2017). Assessing farmer use of climate change adaptation practices and impacts on food security and poverty in Pakistan | Elsevier Enhanced Reader. Clim. Risk Manag. 16, 183–194. doi: 10.1016/j.crm.2016.12.001
Álvarez-Berríos, N. L., Soto-Bayó, S., Holupchinski, E., Fain, S. J., and Gould, W. A. (2018). Correlating drought conservation practices and drought vulnerability in a tropical agricultural system. Renew. Agric. Food Syst. 33, 279–291. doi: 10.1017/S174217051800011X
Álvarez-Febles, N., and Félix, G. (2020). “Hurricane maría, agroecology, and climate change resiliency,” in Climate Justice and Community Renewal, eds B. Tokar and T. Gilbertson (London: Routledge), 131–146.
Anderzén, J., Guzmán Luna, A., Luna-González, D. V., Merrill, S. C., Caswell, M., Méndez, V. E., et al. (2020). Effects of on-farm diversification strategies on smallholder coffee farmer food security and income sufficiency in Chiapas, Mexico. J. Rural Stud. 77, 33–46. doi: 10.1016/j.jrurstud.2020.04.001
Bagagnan, A., Ouedraogo, I. M, Fonta, W., Sowe, M., and Wallis, A. (2019). Can protection motivation theory explain farmers' adaptation to climate change decision making in The Gambia? Climate 7:13. doi: 10.3390/cli7010013
Bang, H. N., Miles, L. S., and Gordon, R. D. (2019). Hurricane occurrence and seasonal activity: an analysis of the 2017 Atlantic hurricane season. Am. J. Clim. Change 08, 454–481. doi: 10.4236/ajcc.2019.84025
Barnes, M. L., Wang, P., Cinner, J. E., Graham, N. A. J., Guerrero, A. M., Jasny, L., et al. (2020). Social determinants of adaptive and transformative responses to climate change. Nat. Clim. Chang. 10, 823–828. doi: 10.1038/s41558-020-0871-4
Bonilla, Y. (2020). The coloniality of disaster: race, empire, and the temporal logics of emergency in Puerto Rico, USA. Polit. Geogr. 78:102181. doi: 10.1016/j.polgeo.2020.102181
Borges-Méndez, R., and Caron, C. (2019). Decolonizing resilience: the case of reconstructing the coffee region of puerto rico after Hurricanes Irma and Maria. J. Extreme Events 06:1940001. doi: 10.1142/S2345737619400013
Brooks, N., and Adger, W. N. (2005). “Assessing and enhancing adaptive capacity,” in Adaptation Policy Frameworks for Climate Change: Developing Strategies, Policies, and Measures, eds B. Lim, E. Spanger-Siegfried, I. Burton, E.L. Malone, and S. Huq (New York, NY: Cambridge University Press), 165–182.
Bueno, R. (2017). Puerto Rico, Climatic Extremes, and the Economics of Resilience. Middlesex County, MA: Global Development and Environmental Institute, Tufts University, 10. Available online at: https://sites.tufts.edu/gdae/files/2019/10/ClimatePolicyBrief6.pdf
Carro-Figueroa, V. (2002). Agricultural decline and food import dependency in puerto rico: a historical perspective on the outcomes of postwar farm and food policies. Caribb. Stud. 30, 77–107.
Castro Rivera, A., and López Marrero, T. d. M. Universidad de Puerto Rico Recinto de Mayagüez, Programa de Colegio Sea Grant, Centro Interdisciplinario de Estudios del Litoral (2018). Cartilla de los ciclones. Programa Sea Grant, Universidad de Puerto Rico.
Caswell, M., Méndez, V. E., Hayden, J., Anderzén, J., Cruz, A., Merrit, P., et al. (2016). Assessing Resilience in Coffee Dependent Communities of Honduras, Nicaragua, and Haiti [Research Report]. Burlington: University of Vermont.
Cinner, J. E., Adger, W. N., Allison, E. H., Barnes, M. L., Brown, K., Cohen, P. J., et al. (2018). Building adaptive capacity to climate change in tropical coastal communities. Nat. Clim. Chang. 8, 117–123. doi: 10.1038/s41558-017-0065-x
Claassen, R., Langpap, C., and Wu, J. (2017). Impacts of federal crop insurance on land use and environmental quality. Am. J. Agric. Econ. 99, 592–613. doi: 10.1093/ajae/aaw075
Clay, L. A., Greer, A., and Kendra, J. (2018). Learning from historic disaster response: reviewing old lessons on disaster mental health. Risk Hazard. Crisis Public Policy 9, 303–331. doi: 10.1002/rhc3.12137
Comas-Pagán, M. (2014). Plan de sguridad alimentaria. San Juan: Puerto Rico Department of Agriculture, p. 40.
Creswell, J. W. (2014). Research Design: Qualitative, Quantitative, and Mixed Methods Approaches, 4th Edn. Thousand Oaks, CA: SAGE.
Creswell, J. W. (2016). Thirty Essential Skills for the Qualitative Researcher. Thousand Oaks, CA: Sage Publications, Inc.
Creswell, J. W., and Plano-Clark, V. (2018). Designing and Conducting Mixed Methods Research (Third). Thousand Oaks, CA: SAGE.
Dang, H. L., Li, E., Nuberg, I., and Bruwer, J. (2018). Vulnerability to climate change and the variations in factors affecting farmers' adaptation: a multi-group structural equation modelling study. Clim. Dev. 10, 509–519. doi: 10.1080/17565529.2017.1304885
Díaz Rolón, A. (2019). Aspira a que haya más agricultores bonafide. San Juan: El Vocero de Puerto Rico. Available online at: https://www.elvocero.com/gobierno/aspira-a-que-haya-m-s-agricultores-bonafide/article_017eaa76-a2b8-11e9-9255-8773684782d5.html (accessed June 12, 2020).
Díaz, E. L., Gould, W. A., Álvarez-Berríos, N., Aponte-Gonzalez, F., Archibald, W., Bowden, J. H., et al. (2018). Chapter 20: US Caribbean. Impacts, Risks, and Adaptation in the United States: The Fourth National Climate Assessment, Volume II. Washington, DC: U.S. Global Change Research Program.
Doran, E. M. B., Zia, A., Hurley, S. E., Tsai, Y., Koliba, C., Adair, C., et al. (2020). Social-psychological determinants of farmer intention to adopt nutrient best management practices: implications for resilient adaptation to climate change. J. Environ. Manage. 276:111304. doi: 10.1016/j.jenvman.2020.111304
FAO (2014). Food Security and Nutrition in Small Island Developing States (SIDS). Policy Paper 16. Rome: FAO.
Félix, G., and Holt-Giménez, E. (2017). Hurricane María: An Agroecological Turning Point for Puerto Rico? (Food First's Backgrounders) [Policy brief]. Food First/Institute for Food and Development Policy, 4. Available online at: https://foodfirst.org/wp-content/uploads/2017/11/PRv5.pdf
Fernandez, M., and Méndez, V. E. (2019). Subsistence under the canopy: agrobiodiversity's contributions to food and nutrition security amongst coffee communities in Chiapas, Mexico. Agroecol. Sustain. Food Syst. 43, 579–601. doi: 10.1080/21683565.2018.1530326
Foguesatto, C. R., Borges, J. A. R., and Machado, J. A. D. (2020). A review and some reflections on farmers' adoption of sustainable agricultural practices worldwide. Sci. Total Environ. 729:138831. doi: 10.1016/j.scitotenv.2020.138831
Gallopín, G. C. (2006). Linkages between vulnerability, resilience, and adaptive capacity. Glob. Environ. Change 16, 293–303. doi: 10.1016/j.gloenvcha.2006.02.004
Gould, W. A. (2015). Caribbean Regional Climate Sub Hub Assessment of Climate Change Vulnerability and Adaptation and Mitigation Strategies. San Juan: USDA Forest Service. International Institute of Tropical Forestry.
Gould, W. A., Wadsworth, F. H., Quiñones, M., Fain, S. J., and Álvarez-Berríos, N. L. (2017). Land use, conservation, forestry, and agriculture in Puerto Rico. Forests 8:242. doi: 10.3390/f8070242
Graham, B. (2012). Profile of the Small-Scale Farming in the Caribbean. Rome: Food and Agriculture Organization, p. 62.
Haden, V. R., Niles, M. T., Lubell, M., Perlman, J., and Jackson, L. E. (2012). Global and local concerns: what attitudes and beliefs motivate farmers to mitigate and adapt to climate change? PLoS ONE 7:e52882. doi: 10.1371/journal.pone.0052882
Harvey, C. A., Saborio-Rodríguez, M., Martinez-Rodríguez, M. R., Viguera, B., Chain-Guadarrama, A., Vignola, R., et al. (2018). Climate change impacts and adaptation among smallholder farmers in Central America. Agric. Food Secur. 7:57. doi: 10.1186/s40066-018-0209-x
Holt-Giménez, E. (2002). Measuring farmers' agroecological resistance after Hurricane Mitch in Nicaragua: a case study in participatory, sustainable land management impact monitoring. Agric. Ecosyst. Envir. 93, 87–105. doi: 10.1016/S0167-8809(02)00006-3
IPCC (2014). The IPCC's Fifth Assessment Report: What's in It for Small Island Developing States? Climate and Development Knowledge Network.
Irizarry-Ruiz, C. (2016). Desafíos de la agricultura en Puerto Rico para garantizar la seguridad alimentaria. Retos Que Conlleva La Reutilización de Aguas Residuales En La Agricultura. San Juan: Reutilización de Aguas Residuales para la Agricultura.
Jezeer, R. E., Verweij, P. A., Boot, R. G. A., Junginger, M., and Santos, M. J. (2019). Influence of livelihood assets, experienced shocks, and perceived risks on smallholder coffee farming practices in Peru. J. Environ. Manage. 242, 496–506. doi: 10.1016/j.jenvman.2019.04.101
Kassie, M., Teklewold, H., Jaleta, M., Marenya, P., and Erenstein, O. (2015). Understanding the adoption of a portfolio of sustainable intensification practices in eastern and southern Africa. Land Use Policy 42, 400–411. doi: 10.1016/j.landusepol.2014.08.016
Kim, K., and Bui, L. (2019). Learning from Hurricane Maria: Island ports and supply chain resilience. Int. J. Disaster Risk Reduct. 39:101244. doi: 10.1016/j.ijdrr.2019.101244
Kuder, G. F., and Richardson, M. W. (1937). The theory of estimation of test reliability. Psychometrika 2, 151–160. doi: 10.1007/BF02288391
Lavrakas, P. J. (2008). Encyclopedia of Survey Research Methods, Vol. 1–1–10. Thousand Oaks, CA: Sage Publications, Inc.
López-Marrero, T. (2010). An integrative approach to study and promote natural hazards adaptive capacity: a case study of two flood-prone communities in Puerto Rico: an integrative approach to study and promote natural hazards adaptive capacity. Geogr. J. 176, 150–163. doi: 10.1111/j.1475-4959.2010.00353.x
López-Marrero, T., and Castro-Rivera, A. (2018). Actividad ciclónica en Puerto Rico y sus alrededores: 1867 al 2017. Centro Interdisciplinario de Estudios del Litoral. Mayagüez: University of Puerto Rico, Mayagüez Campus.
López-Marrero, T., and Castro-Rivera, A. (2019). Let's not forget about non-land-falling cyclones: tendencies and impacts in Puerto Rico. Nat. Hazards 98, 809–815. doi: 10.1007/s11069-019-03716-y
López-Marrero, T., and Wisner, B. (2012). Not in the same boat: disasters and differential vulnerability in the insular Caribbean. Caribb. Stud. 40, 129–168. doi: 10.1353/crb.2012.0034
López-Marrero, T., and Yarnal, B. (2010). Putting adaptive capacity into the context of people's lives: a case study of two flood-prone communities in Puerto Rico. Nat. Hazards 52, 277–297. doi: 10.1007/s11069-009-9370-7
Lowitt, K., Hickey, G. M., Saint Ville, A., Raeburn, K., Thompson-Colón, T., Laszlo, S., et al. (2015). Factors affecting the innovation potential of smallholder farmers in the Caribbean Community. Reg. Environ. Change 15, 1367–1377. doi: 10.1007/s10113-015-0805-2
Luu, T. A., Nguyen, A. T., Trinh, Q. A., Pham, V. T., Le, B. B., Nguyen, D. T., et al. (2019). Farmers' intention to climate change adaptation in agriculture in the red river delta biosphere reserve (Vietnam): a combination of Structural Equation Modeling (SEM) and Protection Motivation Theory (PMT). Sustainability 11:2993. doi: 10.3390/su11102993
Mase, A. S., Gramig, B. M., and Prokopy, L. S. (2017). Climate change beliefs, risk perceptions, and adaptation behavior among Midwestern U.S. crop farmers. Clim. Risk Manag. 15, 8–17. doi: 10.1016/j.crm.2016.11.004
Masters, P., and Houser, trevor. (2017). America's Biggest Blackout. New York, NY: Rhodium Group. Available online at: https://rhg.com/research/americas-biggest-blackout-2/ (accessed January 29, 2021).
Moulton, A. A., and Machado, M. R. (2019). Bouncing forward after irma and maria: acknowledging colonialism, problematizing resilience, and thinking climate justice. J. Extreme Events 06:1940003. doi: 10.1142/S2345737619400037
Nichols, A., and Schaffer, M. (2007). “Clustered errors in Stata,” in United Kingdom Stata Users{Group Meeting, United Kingdom} (London).
Niles, M. T., Brown, M., and Dynes, R. (2016). Farmer's intended and actual adoption of climate change mitigation and adaptation strategies. Clim. Change 135, 277–295. doi: 10.1007/s10584-015-1558-0
Niles, M. T., Lubell, M., and Brown, M. (2015). How limiting factors drive agricultural adaptation to climate change. Agric. Ecosyst. Environ. 200, 178–185. doi: 10.1016/j.agee.2014.11.010
Niles, M. T., and Mueller, N. D. (2016). Farmer perceptions of climate change: associations with observed temperature and precipitation trends, irrigation, and climate beliefs. Glob. Environ. Change 39, 133–142. doi: 10.1016/j.gloenvcha.2016.05.002
Niles, M. T., and Salerno, J. D. (2018). A cross-country analysis of climate shocks and smallholder food insecurity. PLoS ONE 13:e0192928. doi: 10.1371/journal.pone.0192928
O'Connor, C., and Joffe, H. (2020). Intercoder reliability in qualitative research: debates and practical guidelines. Int. J. Qual. Methods 19:1609406919899220. doi: 10.1177/1609406919899220
Paul, J., Sierra, J., Causeret, F., Guindé, L., and Blazy, J.-M. (2017). Factors affecting the adoption of compost use by farmers in small tropical Caribbean islands. J. Clean. Prod. 142, 1387–1396. doi: 10.1016/j.jclepro.2016.11.168
Payne, P., Kaye-Blake, W., Kelsey, A., Brown, M., and Niles, M. (2021). Measuring rural community resilience: case studies in New Zealand and Vermont, USA. Ecol. Soc. 26:2. doi: 10.5751/ES-12026-260102
Perfecto, I., Hajian-Forooshani, Z., Iverson, A., Irizarry, A. D., Lugo-Perez, J., Medina, N., et al. (2019). Response of coffee farms to hurricane maria: resistance and resilience from an extreme climatic event. Sci. Rep. 9:15668. doi: 10.1038/s41598-019-51416-1
Phillips, C. A., Caldas, A., Cleetus, R., Dahl, K. A., Declet-Barreto, J., Licker, R., et al. (2020). Compound climate risks in the COVID-19 pandemic. Nat. Clim. Chang. 10, 586–588. doi: 10.1038/s41558-020-0804-2
Prokopy, L. S., Floress, K., Arbuckle, J. G., Church, S. P., Eanes, F. R., Gao, Y., et al. (2019). Adoption of agricultural conservation practices in the United States: evidence from 35 years of quantitative literature. J. Soil Water Conserv. 74, 520–534. doi: 10.2489/jswc.74.5.520
Quarantelli, E. L. (1992). The Importance of Thinking of Disasters as Social Phenomena. Newark, NJ: The Disaster Research Center of the University of Delaware 7.
Ranjan, P., Church, S. P., Floress, K., and Prokopy, L. S. (2019). Synthesizing conservation motivations and barriers: what have we learned from qualitative studies of farmers' behaviors in the United States? Soc. Nat. Resour. 32, 1171–1199. doi: 10.1080/08941920.2019.1648710
Raza, M. H., Abid, M., Yan, T., Ali Naqvi, S. A., Akhtar, S., and Faisal, M. (2019). Understanding farmers' intentions to adopt sustainable crop residue management practices: a structural equation modeling approach. J. Clean. Prod. 227, 613–623. doi: 10.1016/j.jclepro.2019.04.244
Reed, M. S., Podesta, G., Fazey, I., Geeson, N., Hessel, R., Hubacek, K., et al. (2013). Combining analytical frameworks to assess livelihood vulnerability to climate change and analyse adaptation options. Ecol. Econ. 94, 66–77. doi: 10.1016/j.ecolecon.2013.07.007
Reyes, J., Elias, E., Haacker, E., Kremen, A., Parker, L., and Rottler, C. (2020). Assessing agricultural risk management using historic crop insurance loss data over the ogallala aquifer. Agric. Water Manag. 232:106000. doi: 10.1016/j.agwat.2020.106000
Ribot, J. (2014). Cause and response: vulnerability and climate in the Anthropocene. J. Peasant Stud. 41, 667–705. doi: 10.1080/03066150.2014.894911
Rodríguez-Cruz, L. A., and Niles, M. T. (2020). Survey materials: Understanding Puerto Rican Farmers' Perceptions of Climate Change and Hurricane Maria, Vol. 1. Harvard Dataverse. doi: 10.7910/DVN/JZFWZZ
Rodríguez-Cruz, L. A., and Niles, M. T. (2021). Awareness of climate change's impacts and motivation to adapt are not enough to drive action: a look of Puerto Rican farmers after Hurricane Maria. PLoS ONE 16:e0244512. doi: 10.1371/journal.pone.0244512
Rosset, P. M., Sosa, B. M., Jaime, A. M. R., and Lozano, D. R. Á. (2011). The Campesino-to-Campesino agroecology movement of ANAP in Cuba: social process methodology in the construction of sustainable peasant agriculture and food sovereignty. J. Peasant Stud. 38, 161–191. doi: 10.1080/03066150.2010.538584
Saint Ville, A. S., Hickey, G. M., Locher, U., and Phillip, L. E. (2016). Exploring the role of social capital in influencing knowledge flows and innovation in smallholder farming communities in the Caribbean. Food Secur. 8, 535–549. doi: 10.1007/s12571-016-0581-y
Salerno, J., Konecky, B. L., Diem, J. E., and Hartter, J. (2019). Recent intensification of the seasonal rainfall cycle in equatorial Africa revealed by farmer perceptions, satellite-based estimates, and ground-based station measurements. Clim. Change 153, 123–139. doi: 10.1007/s10584-019-02370-4
Santos-Burgoa, C., Goldman, A., Andrade, E., Barret, N., Colon-Ramos, U., Edberg, M., et al (2018). Acertainment of the Estimated Excess Mortality from Hurricane Maria in Puerto Rico.pdf. Washington, DC: Milken Institute School of Public Heath, George Washington University. Available online at: https://publichealth.gwu.edu/sites/default/files/downloads/projects/PRstudy/Acertainment%20of%20the%20Estimated%20Excess%20Mortality%20from%20Hurricane%20Maria%20in%20Puerto%20Rico.pdf (accessed May 20, 2019).
Scobie, M. (2018). Accountability in climate change governance and Caribbean SIDS. Environ. Dev. Sustain. 20, 769–787. doi: 10.1007/s10668-017-9909-9
Shah, S. H., Wagner, C. H., Sanga, U., Park, H., Demange, L. H. M., de, L., et al. (2019). Does household capital mediate the uptake of agricultural land, crop, and livestock adaptations? evidence from the indo-gangetic plains (India). Front. Sustain. Food Syst. 3:1. doi: 10.3389/fsufs.2019.00001
Shinbrot, X. A., Jones, K. W., Rivera-Castañeda, A., López-Báez, W., and Ojima, D. S. (2019). Smallholder farmer adoption of climate-related adaptation strategies: the importance of vulnerability context, livelihood assets, and climate perceptions. Environ. Manage. 63, 583–595. doi: 10.1007/s00267-019-01152-z
Singh, A. S., Zwickle, A., Bruskotter, J. T., and Wilson, R. (2017). The perceived psychological distance of climate change impacts and its influence on support for adaptation policy. Environ. Sci. Policy 73, 93–99. doi: 10.1016/j.envsci.2017.04.011
Smit, B., and Wandel, J. (2006). Adaptation, adaptive capacity and vulnerability. Glob. Environ. Change 16, 282–292. doi: 10.1016/j.gloenvcha.2006.03.008
Spence, A., Poortinga, W., Butler, C., and Pidgeon, N. F. (2011). Perceptions of climate change and willingness to save energy related to flood experience. Nat. Clim. Chang. 1, 46–49. doi: 10.1038/nclimate,1059
US Census Bureau (2020). U.S. Census Bureau QuickFacts: Puerto Rico. Available online at: https://www.census.gov/quickfacts/PR (accessed May 27, 2021).
USDA ERS (2020). USDA ERS–Farm Household Income Estimates. Available online at: https://www.ers.usda.gov/topics/farm-economy/farm-household-well-being/farm-household-income-estimates/ (accessed May 27, 2021).
USDA NASS (2020). 2017 Census of Agriculture—Puerto Rico Data (p. 117) [Census]. Available online at: https://www.nass.usda.gov/Publications/AgCensus/2017/index.php (accessed May 27, 2021).
Wang, Y., Liang, J., Yang, J., Ma, X., Li, X., Wu, J., et al. (2019). Analysis of the environmental behavior of farmers for non-point source pollution control and management: an integration of the theory of planned behavior and the protection motivation theory. J. Environ. Manag. 237, 15–23. doi: 10.1016/j.jenvman.2019.02.070
Weis, T. (2007). Small farming and radical imaginations in the Caribbean today. Race Class 49, 112–117. doi: 10.1177/03063968070490020607
White, A., Faulkner, J., Sims, S., Tucker, P., and Weatherhogg, K. (2018). Report of the 2017-2018 New England Adaptation Survey for Vegetable and Fruit Growers. University of Vermont.
Wilson, R. S., Herziger, A., Hamilton, M., and Brooks, J. S. (2020). From incremental to transformative adaptation in individual responses to climate-exacerbated hazards. Nat. Clim. Chang. 10, 200–208. doi: 10.1038/s41558-020-0691-6
Wisner, B., Blaikie, P., Cannon, T., and Davis, I. (2004). At Risk: Natural Hazards, People's Vulnerability and Disasters, 2nd Edn. London: Routledge.
Wisner, B., Gaillard, J., and Kelman, I. (2012). “Framing disaster: theories and stories seeking to understand hazards, vulnerability and risk,” in The Routledge Handbook of Hazards and Disaster Risk Reduction, eds B. Wisner, J. C. Gaillard, and I. Kelman (London; New York, NY: Routledge), 18–33.
Zhang, J. A., Dunion, J. P., and Nolan, D. S. (2020). In situ observations of the diurnal variation in the boundary layer of mature hurricanes. Geophys. Res. Lett. 47:2019GL086206. doi: 10.1029/2019GL086206
Keywords: climate change, islands and archipelagos, food systems, farmers' decision-making, disaster and climate risk reduction
Citation: Rodríguez-Cruz LA, Moore M and Niles MT (2021) Puerto Rican Farmers' Obstacles Toward Recovery and Adaptation Strategies After Hurricane Maria: A Mixed-Methods Approach to Understanding Adaptive Capacity. Front. Sustain. Food Syst. 5:662918. doi: 10.3389/fsufs.2021.662918
Received: 01 February 2021; Accepted: 02 June 2021;
Published: 05 July 2021.
Edited by:
Margiana Petersen-Rockney, University of California, Berkeley, United StatesReviewed by:
Anita Wreford, Lincoln University, New ZealandTimothy Bowles, University of California, Berkeley, United States
Copyright © 2021 Rodríguez-Cruz, Moore and Niles. This is an open-access article distributed under the terms of the Creative Commons Attribution License (CC BY). The use, distribution or reproduction in other forums is permitted, provided the original author(s) and the copyright owner(s) are credited and that the original publication in this journal is cited, in accordance with accepted academic practice. No use, distribution or reproduction is permitted which does not comply with these terms.
*Correspondence: Luis Alexis Rodríguez-Cruz, lrodrig2@uvm.edu