- 1A & L Canada Laboratories Inc., London, ON, Canada
- 2Department of Biology, The University of Western Ontario, London, ON, Canada
Soil bacteria and fungi are integral parts of healthy ecosystem functioning in production agriculture. The effects of fungal abundance and diversity on crop productivity is poorly understood. We sampled 10 corn farms at the V10 growth stage across southwestern Ontario, Canada, using aerial infrared imaging to identify zones of low and high productive corn plants. Roots and soils were sampled from low and high yield zones and soil physical and chemical properties were measured in conjunction with assessment of the root mycobiome communities using Illumina MiSeq sequencing of 4 rRNA amplicons. Higher crop yields were associated with sites having greater fungal phylogenetic diversity and Fisher's α diversity. Indicator species associated with high and low yield sites within a farm could be identified but there were no shared fungal indicators of productivity differences across farms. Communities largely varied across locations despite crop genetics, demonstrating a major influence of soil texture and chemistry in shaping the mycobiome in a site-specific manner. Across all 4 primers, roots from high-yielding sites shared 35 major OTUs including Penicillium spp., Trichoderma, Chalara fungorum, and Gibellulopsis. Low-yielding sites shared 31 OTUs including Fusarium spp., Pythium, Setophoma terrestris, and Neonectria. Soil physical and chemical parameters that contributed to broad scale differences in yield and mycobiome diversity included: %clay, %sand, %phosphorus saturation, cation exchange capacity, aluminum, pH, iron, potassium, %moisture, organic matter, and chlorine. The results show the importance of physicochemical balance in shaping the relationship between root mycobiome and plant productivity.
Introduction
Sustainable agricultural production can be achieved through the development and maintenance of high soil quality. One of the main biological factors related to soil quality is its fungal and bacterial microbiome abundance and diversity (Liebig and Doran, 1999). Microbial diversity can directly influence plant productivity by influencing plant growth and development, plant competition, nutrient and water uptake (Baliyarsingh et al., 2017), and indirectly through contributions to nutrient turnover and soil aggregation (Wilpiszeski et al., 2019). Soil type, cropping, and tillage systems substantially influence microbial structure and its function (Suzuki et al., 2005). Many of our current agricultural techniques and production practices result in the physicochemical and biological depletion of the soil environment due to intensive and extractive crop production, resulting in increased soil degradation including losses of nutrients and soil organic matter (Dregne, 2002; Rashid et al., 2016).
Maintaining microbiome-friendly agricultural practices in conjunction with the integration of naturally occurring beneficial microbiomes is an essential part of sustainable agriculture in order to ensure food security (Blaser, 2016). Root associated microbiota play important roles in agricultural ecosystems by helping to improve soil and plant health, thereby improving plant fitness in various production systems. In addition, microbes aid in improving yield while protecting the environment by reducing the need for chemical agricultural inputs such as fertilizers and pesticides (Rodriguez et al., 2009). Bacteria and fungi grow in the rhizosphere and rhizoplane, with some moving systemically to colonize aboveground plant parts and develop symbiotic relationships with host plants by improving beneficial plant functional traits (Hardoim et al., 2015). Petrini (1986) reviewed 100 years of research and suggested that almost all the plants in natural ecosystems have symbiotic relationships with mycorrhizal fungi and/or fungal endophytes.
Ecological factors and plant genetics that regulate plant-microbe interactions are variable, and understanding these relationships is essential for the appropriate integration of beneficial microbiota in crop production (Siddikee et al., 2016). Proper agricultural production practices can positively influence the soil system and its microbial diversity. Practices such as no-till farming (Islam et al., 2015), intercropping (Bargaz et al., 2017), and rotational cropping (Cook, 2007; Peralta et al., 2018) help to increase soil organic matter and to build and conserve microbial communities with specific functional traits to improve crop production. Increased plant productivity, plant health, enhanced photosynthetic rates, and grain yield have been linked with the beneficial functional activities of rhizosphere/root associated microorganisms with no-till fence-row farming of maize and soybeans with yearly rotation (Islam et al., 2015).
The development of high throughput single gene sequencing allows for the in-depth analyses of microbial communities (Fierer et al., 2007; Rousk et al., 2010; Caporaso et al., 2011). In order to maximize crop yields, we must combine the knowledge of the root-associated microbial communities, soil health, and chemical factors. Crop productivity has been linked to deficiencies or toxicities in nutrients including N, P, K, Mn, S, Zn, Fe, B, Cl, Si, and several heavy metals that may affect plant physiology, enhance or reduce biotic stress, or both (Astolfi et al., 2004; Dordas, 2008; Hänsch and Mendel, 2009; Veresoglou et al., 2013; Jacoby et al., 2017).
Providing a suitable environment for the establishment of beneficial microorganisms will increase the nutrient availability to plants and allow for the development of environmentally friendly, sustainable agricultural practices and technologies (Singh et al., 2011, 2017; Rashid et al., 2016). Plant root exudates promote rich microbial diversity around the root-zone, providing nutrition for bacteria and fungi in exchange for plant growth promotion through various mechanisms (Patel et al., 2015; Rashid et al., 2016; Singh et al., 2017). Beneficial microbes such as plant growth promoting rhizobacteria (PGPR) and plant growth promoting fungi (PGPF) are familiar targets for the development of agricultural technologies and practices that enhance plant productivity by reducing abiotic/biotic stress, providing pathogen protection, increasing nutrient solubilization (N and P), and producing bioactive compounds (Smith et al., 2003; Adesemoye et al., 2008; Behn, 2008; Gamalero et al., 2009; Nadeem et al., 2014; Singh et al., 2017; Bilal et al., 2018; Rocha et al., 2019), to list a few. Identifying potential PGPFs that have entomopathogenic, endophytic, and/or mycorrhizal (Bilal et al., 2018; Zhou L. et al., 2018) lifestyles for the development of inoculants or mycofertilizers (Hnatowich et al., 2012) is a major driver in increasing agricultural sustainability (Bashan, 1998; Berg, 2009).
The use of unmanned aerial vehicles (UAVs) with near-infrared imaging devices to construct a Normalized Difference Vegetation Index (NDVI) map allows researchers to identify areas of good or poor crop health (Mahajan and Bundel, 2017; Hassler and Baysal-Gurel, 2019; Shafi et al., 2019). Large variations in yield are common within a field, despite nearly identical levels of farm inputs (e.g., fertilizers, same genetic hybrid), and this variation can be related to interactions between plant, soil physical and/or chemical characteristics, and the interactions between soil- and plant- associated microbial communities. Our previous studies hypothesized that differences in plant productivity within a field are linked to the differences in abundance and diversity of microbial communities, and their impacts on plant growth and yield. However, we did not look for the contribution of soil physical and chemical characteristics on these interactions (Ali et al., 2020). A subsequent growth room study demonstrated the influence of key microbes on a soil's native microbial diversity and its plant productivity (Kandasamy et al., 2019). Disentangling, understanding, and manipulating these relationships may help to reduce unevenness in crop yields and to develop mycofertilizers.
Corn (Zea mays) is the major cereal crop grown in Ontario, and the province grows ~63% (7.5–9 million tons a year) of Canada's corn grain on 800,000 ha of farmland (OMAFRA: Field Crops, 2019). Corn production in Canada is predicted to be 14.1 million metric tons with the average yield of 10.33 t/ha (153.6 bu/ac) in 2020 (https://www150.statcan.gc.ca/n1/en/daily-quotidien/190912/dq190912b-eng.pdf). The basis for lower-than-average production and within field variability may be due to different soil nutritional and physical characteristics as well as biological components including fungi and bacteria. There are many studies that assess the association of bacterial communities on plant health and productivity, but fungal interactions are often harder to clarify. We hypothesized that root fungal communities would have strong correlations with crop productivity despite varying soil types and that there may be one or more indicator taxa present in high/low productive areas across all farms. In order to test the hypothesis: (1) we sampled soil and plants from low and high yielding sites within 10 corn fields across southwestern Ontario by using NDVI maps to identify sites with or without plant stress, (2) sequenced corn root-associated mycobiome to identify taxa that may be contributing to or reducing plant health and yield, and to identify the key soil physicochemical factors that associate with low or high productivity, and (3) assessed the underlying relationship of these fungal communities with soil nutritional and physical characteristics and their impact on yield.
Materials and Methods
Study Sites
Ten corn fields across southwestern Ontario, varying in cultivation practices but sharing similar eco-climatic factors, were sampled as a part of this study (Table 1). These regions represent various heat zones and fertility statuses. High and low yielding sites within each farm were determined by flying a remote sensing UAV to identify healthy and stressed corn plants, respectively (see Section UAV Data Collection and Construction of NDVI Maps; Figure 1). GPS coordinates of the stressed and healthy sites were then used to navigate to these locations during field sampling (Table 1).
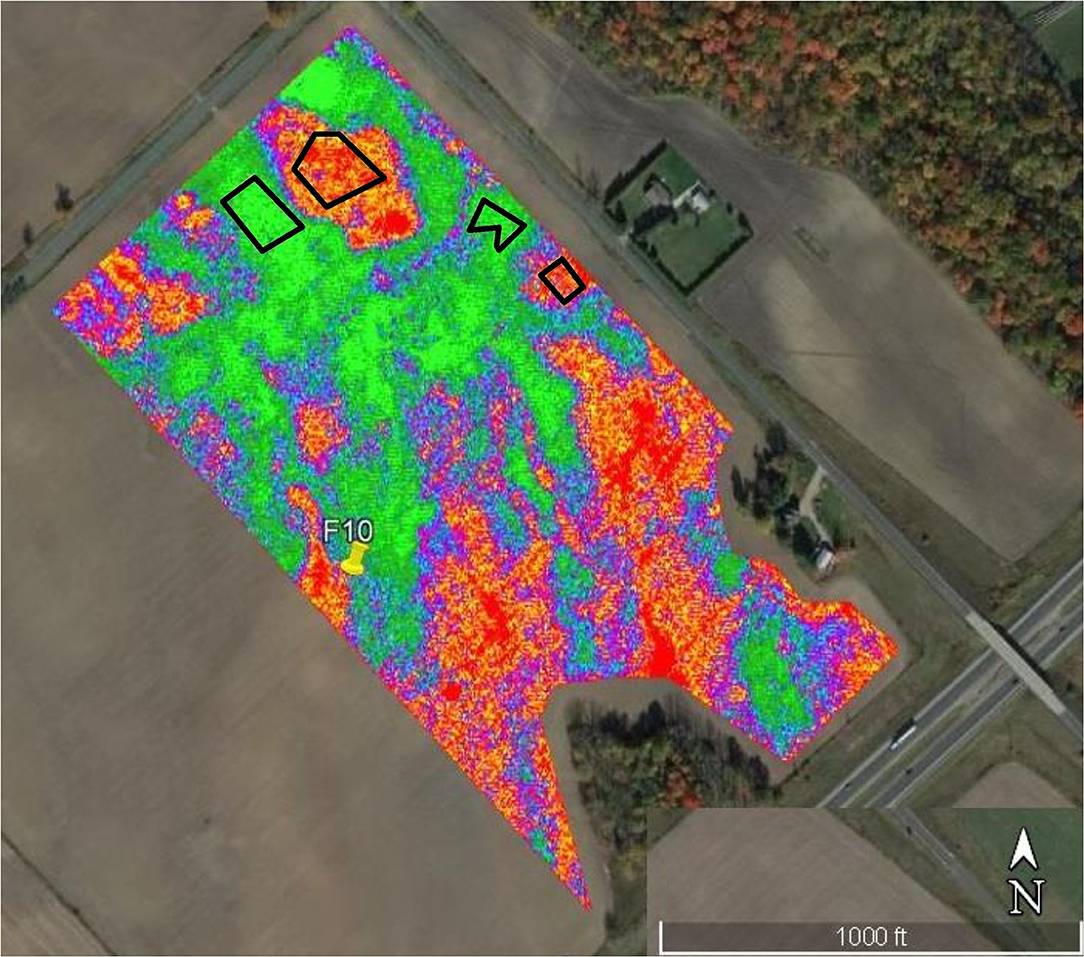
Figure 1. Infra-red aerial image taken by an Unmanned Aerial Vehicle (UAV) of corn at the V10 growth stage in Field 10. Black polygons outline stressed, low yield (red-orange) and healthy, high-yield (green-blue) corn.
UAV Data Collection and Construction of NDVI Maps
A remote sensing unmanned aerial vehicle (UAV) was flown over fields in each location at the V10 stage of the crop cycle (~80 days after planting) to create a visual representation of healthy and stressed sites across the field through the Normalized Difference Vegetation Index (NDVI). The UAV is ~2.1 m wide and 1.2 m long and contains two cameras mounted on each wing; one camera collects full color imagery while the other camera collects infrared imagery. NDVI is calculated based on contrasting intense chlorophyll pigment absorption in red (R) visible light against the high reflectance of plant materials in the near infrared (NIR) (Moriondo et al., 2007):
Sampling
Selected high and low producing sample sites were ~15 × 15 m each. Ten corn plants were sampled randomly from each site. Approximately 450 g of rhizosphere soil was collected from every plant by shaking the roots of corn plants into a bag after carefully removing the soil away from the roots. We also sampled ~500 g of bulk soil from non-root zones. Afterwards, roots were separated from the stalk, washed under running tap water, blotted dry, chopped into fine pieces, mixed, and 200 mg samples were weighed out for microbial DNA extraction.
Soil Chemical Analysis
All soil samples were sent to A&L Canada Inc. for chemical analysis which included general fertility index (GFI), organic matter (OM%), phosphorus (bicarbonate & Bray-P1; ppm), potassium (ppm), magnesium (ppm), calcium (ppm), sodium (ppm), sulfur (ppm), zinc (ppm), manganese (ppm), iron (ppm), copper (ppm), boron (ppm), aluminum (ppm), nitrate-nitrogen (NO3-N; ppm), ammoniacal-nitrogen (NH4-N; ppm), chloride (ppm), pH, cation exchange capacity (CEC meq/100 g), percent base saturations (K, Mg, Ca, H, Na), soluble salts (ms/cm), % phosphorus saturation, K/Mg ratio, estimated nitrogen release (ENR), % moisture, and microbially active carbon (%MAC). All the soil chemical, physical, and textural parameters were measured using the A&L Canada Laboratories accredited soil testing protocols.
Yield Measurements
Each sampling site was revisited in mid- to late- October for corn harvest. Fifty plants were randomly selected from high and low producing sampling sites, cobs were collected and threshed. The total kernel mass of the 50 plants was measured. Percent moisture was determined by drying a small subset of kernels from each site. We then calculated the yield of each site by adding 13% moisture to the kernel dry weight in order to balance the humidity difference across and between farms. The hand harvested yield was compared with the exact yield of sampling sites from a yield monitor attached to combine which can track yield to GPS coordinates of each sampling site.
Fungal Community DNA Extraction and Sequencing
Exactly 200 mg of root tissue was extracted for DNA extraction using the Soil DNA Isolation Kit (Norgen Biotek Corporation) as per the manufacturer's instructions with minor modifications. DNA samples were PCR-amplified with the following four sets of primer combinations, with primer-specific PCR conditions. Four sets of primers were chosen to target the amplification of fungal nuclear ribosomal small subunit to cover wider fungal communities. General fungi (minus Ascomycota) 28S LSUBG: LSU200-F (5′-AAC KGC GAG TGA AGM GGG A−3′) and LSU481-R (5′-TCT TTC CCT CAC GGT ACT TG−3′) (Asemaninejad et al., 2016); Ascomycota 28S LSUA: LSU200A-F (5′-AAC KGC GAG TGA AGC RGY A−3′) and LSU476A-R (5′-CSA TCA CTS TAC TTG TKC GC−3′) (Asemaninejad et al., 2016); Glomeromycota 18S V4AM: AMV4.5N-F (5′-AAC KGC GAG TGA AGC RGY A−3′) and AMDG-R (5′-CCC AAC TAT CCC TAT TAA TCA T−3′) (Sato et al., 2005); Fungi ITS2: 5.8S_Fun-F (5′-AAC TTT YRR CAA YGG ATC WCT−3′) and ITS4_Fun-R (5′-CCT CCG CTT ATT GAT ATG CTT AAR T−3′) (Taylor et al., 2016). The four fungal primers (LSUBG, LSUA, V4AM, ITS2) were tagged with Illumina MiSeq (MiSeq) adaptors, a four bp linker (NNNN), and eight nucleotide barcode sequences starting at the 5′ end of each forward and reverse primer (Gloor et al., 2010; Asemaninejad et al., 2016). Pooled amplicon libraries were sequenced through the MiSeq platform. Sample and sequence read data were deposited in the European Nucleotide Archive (https://www.ebi.ac.uk/ena/browser/home) with the project number PRJEB40800.
Bioinformatic and Statistical Analyses
Raw FASTQ datasets were separately processed using a custom MiSeq data processing pipeline (https://github.com/ggloor/miseq_bin/tree/Jean). PANDAseq (https://github.com/neufeld/panda seq) (Masella et al., 2012) was used to overlap reads. Sequences containing ambiguous base calls (N) or mismatches to the primer sequence were removed, and barcodes and primers were trimmed prior to clustering. Individual reads were clustered into identical sequence units (ISUs) and chimera-checked using UCHIME (Edgar et al., 2011). ISUs were grouped into operational taxonomic units (OTUs) at 97% similarity around the most abundant centroid sequence using USEARCH v7.0.1090 (Edgar, 2010). A separate OTU table was made for each primer. Primary taxonomies were done using RDP Classifier and the Unite Fungal ITS trainset (07-04-2014) and the Fungal LSU training set 11 (Wang et al., 2007) for ITS2 and both LSU datasets, respectively. Taxonomic identifications were confirmed manually using BLASTn (Madden, 2002) for assignments with confidence scores lower than 70%. All VAM (Glomeromycota) reads were manually identified using the MaarjAM database (Öpik et al., 2010). Reference gene datasets were made for each primer set using the top BLASTn hits for each OTU, and phylogenetic trees were constructed using the reference sets and OTU sequences to confirm annotations by using Muscle v3.8.31 (Edgar et al., 2011) alignments and Neighbor Joining (NJ) and Maximum Likelihood (ML) in MEGA X v10.0.5 (Kumar et al., 2018).
The programs R v3.6.1 within RStudio 1.2.1335 were used for statistical analyses and visualization. The vegan package was used to make NMDS ordinations with rhizosphere metadata (Oksanen et al., 2019), the factoextra package was used to make biplots (Kassambara and Mundt, 2017), the corrplot package was used to do variable correlation analyses (Wei and Simko, 2017), a custom variance inflation factor (VIF) function was used to do a stepwise VIF analysis using the fsmb package (Beck, 2015; Nakazawa, 2018), and the metacoder package was used to make the taxonomy heatmap (Foster et al., 2017). The phyloseq package was used to calculate diversity indices and create stacked barplots (McMurdie and Holmes, 2013), alongside the mblm package that was used to do a Kendall-Theil Sen Siegel non-parametric linear regression on rarefied OTU data (n = 500 OTUs per sample, without replacement) (Theil, 1950; Sen, 1968; Siegel, 1982; Komsta, 2019), and btools to calculate Faith's Phylogenetic Distance (Battaglia, 2020). A PERMANOVA analysis was done using the “Adonis” function in R, starting with an analysis of variance using UniFrac distance matrices to highlight variables that fit linear models with yield and SIMPER was done to identify which taxonomic groups differed between yields and farms (vegan package) (Oksanen et al., 2019). The ALDEx2 package was used as an ANOVA-like univariate comparison tool incorporating the Bayesian estimate of taxon abundance into a compositional framework, useful for the differentiation of OTU abundances between two conditions to identify taxa that are significantly associated with each condition (Fernandes et al., 2013). The microbiomeSeq package was used to create a correlation matrix between ALDEx2 taxa and soil physicochemistry (Ssekagiri, 2020), and heatmaps were made with Complex Heatmap (Gu, 2016).
Results
Soil Physicochemical Analysis
The complete metadata set, including 52 soil variables from both bulk and rhizosphere, and yields for each high and low yielding site was analyzed using PCA to identify the top contributing soil factors. There was no significant difference in the factor contribution analysis between bulk and rhizosphere soil; therefore, we used rhizosphere soil for all analyses (n = 103). PCA components with variable contributions >10% in each dimension were retained. PC1 was separated by OM (19.6%), Al (19.0%), CEC (16.8%), moisture (16.3%) and yield (10.2%); PC2 by clay (28.1%), sand (18.7%), K (12.5%), %P saturation (12.0%), CEC (10.6%); and PC3 by NO3-N (22.6%), Cl (22.3%), (K, Fe, and %P saturation were also significant) (Figure 2).
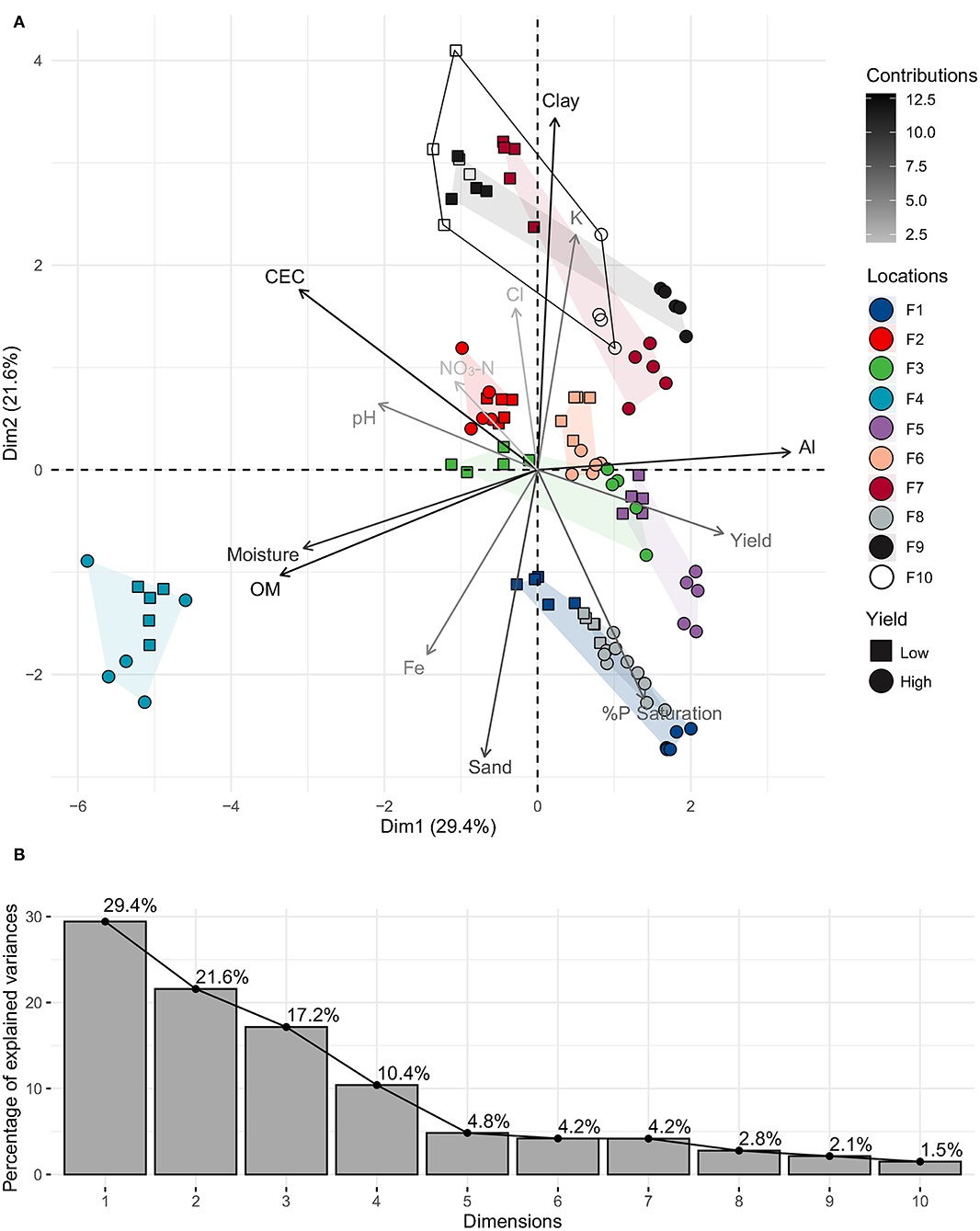
Figure 2. (A) PCA plot of key soil rhizosphere physicochemical factors from low and high yield sites on all farms (1–10). Variable vectors are colored by the strength of their contributions (cos2 value); (B) Scree plot of percentages of explained variances by PCA dimensions.
There were slightly larger between site differences in soil (linked to differences in OM/moisture, Al, CEC) that were seen along PC1 (29.4% explained variance), whereas within-site factors (Clay, sand, K, %P saturation, CEC) separated most high/low yield groups along PC2 (21.6% explained variance), and there was slight within-farm-yield separation seen in PC3 (17.2% explained variance) (influenced by K/Cl/NO3-N on sample replicates in farms 1, 4, and 10) (data not shown). The farms clustered into five groups (defined by overlapping polygons): group 1 containing farms 7, 9, and 10 had high clay, CEC, and K influencing low yield sites; group 2 containing farms 1 and 8 had high %P saturation and sand influencing high yield sites; group 3 containing farms 3, 5, and 6 had low CEC and high %P saturation influencing high yield sites; group 4 containing the outlier farm 4 was characterized by low soil Al and high moisture and OM (due to high hog manure amendments), and group 5 containing farm 2 that did not have differences in final yield measurements (186 vs. 169 bu/ac), resulting in no site separation. In general, higher yields were seen in soils that had high %P saturation (H: 16 vs. L: 11), high sand (49 vs. 35%), low clay (13 vs. 20%), low CEC (11 vs. 19 meq/100 g), low pH (6.6 vs. 7.3) low Ca (1,900 vs. 2,700 ppm), slightly higher levels of %K saturation (3.5 vs. 3.1), and NO3-N (59 vs. 51 ppm). This was true for high yield sites across all farms, except for farms 2 and 4, where there was no clear yield separation along PC1 or PC2.
A stepwise linear regression analysis with yield as the dependent variable and all twelve soil PCA vectors described a broad-scale linear model: yield ~ sand + pH + % moisture + OM + CEC + %P saturation (F(6, 96) = 17.62, p < 0.001, R2 = 0.52, Adj. R2 = 0.49; continuous predictors are mean-centered and scaled by 2 S.D.). A collinearity check showed that % moisture and OM were 81% collinear, and nothing was highly collinear with yield. Despite the high collinearity between OM and moisture, both are important factors that help to correctly predict yield, so we retained both in the model.
Separate PCA analyses were done within individual farms using 22 soil chemical parameters to identify common factors leading to yield differences (Figure 3). Nine out of ten farms (except farm 6), cleanly separated high and low sites along PC1 (data not shown), allowing us to deconstruct the individual factor loading scores (explained % variation along PC1). OM explained most of the PC1 variation (between H/L separated sites averaged across farms), followed closely by Mn, pH, Al, and CEC (Figure 3). The factors that least explained H/L separation across farms were C:N, NO3-N, %MAC, Cl, and sand. When considering only the farms with substantial yield differences >80 bu/ac (5.38 t/ha) (farms 10, 1, 9, 4, and 5), we see distinctions between low and high yield sites with: pH (L: 7.51, H: 6.20), OM (L: 7.64, H: 10.28), %Na (L: 0.28, H: 0.40), Mn (L: 42 ppm, H: 17.5 ppm), Al (L: 575 ppm, H: 700 ppm), and %P saturation (L: 6.21, H: 19.17) (data not shown).
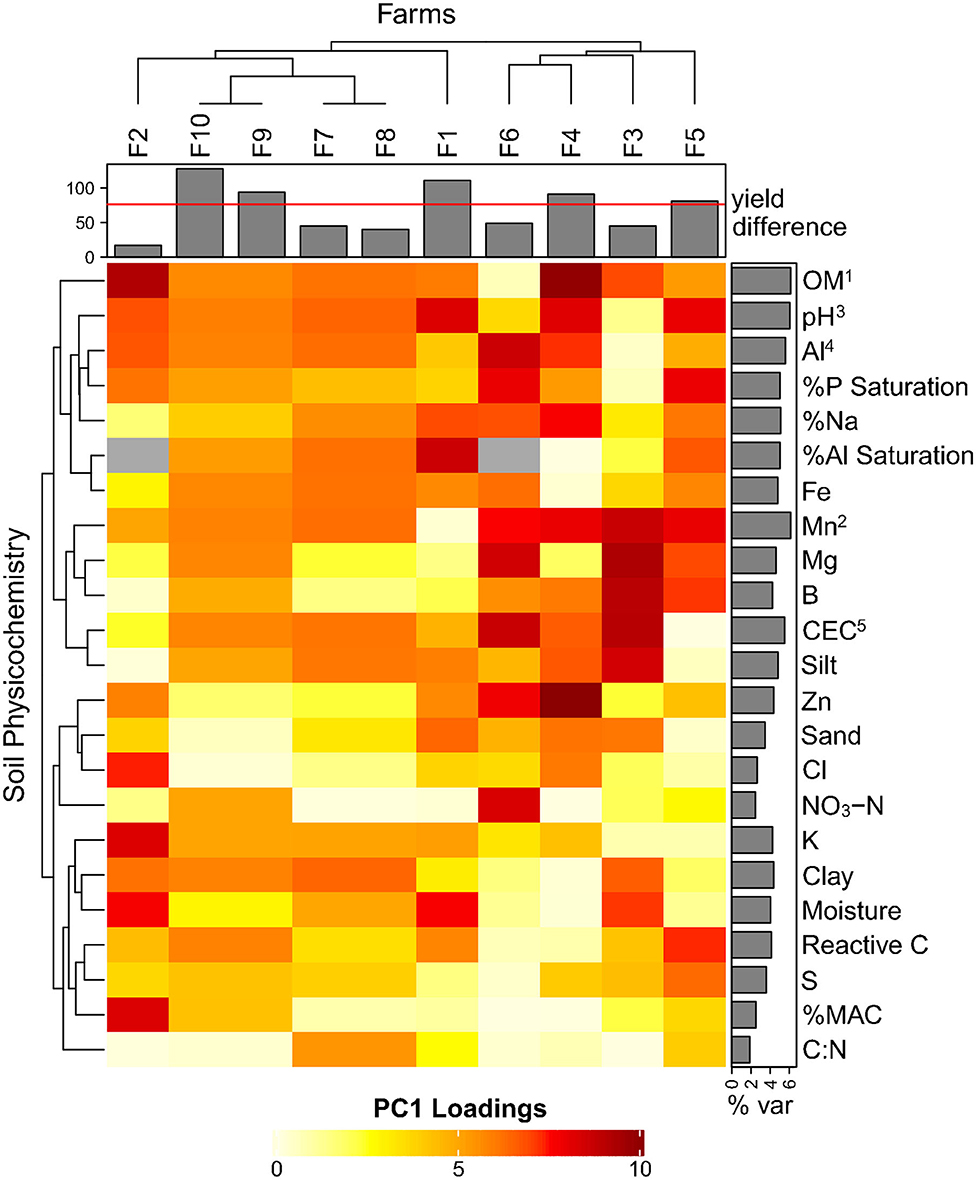
Figure 3. Heatmap of individual farms with PCA-derived loading variables (% explained variation in PC1) for soil physicochemical components (data not shown). The % var row barplot averages each component's explained variation along the PC1 axis, superscript numbers show the order of the top 5 contributing vectors. The column barplot shows the yield differences for each farm (high—low) with a cut-off line at 80 bu/ac (5.38 t/ha) identifying substantial yield differences. N/A values are in gray.
Soil texture, specifically clay content, shows a strong effect in separating study sites including farms 1, 4, 7, 9, and 10 (Figure 4). Low yield farm 1 soils were sandier than high yield, whereas higher yields in farm 4 showed the opposite trend, with low yield sites having a sand-loam or loam texture. Low yield sites in farms 7 and 10 had clay-loam or silt-clay-loam soils, high yield sites were in sand-loam/loam (farm 10) or silt-loam (farm 7) soils. High yield sites in farm 9 followed the opposite pattern, where high yield soils had more clay. Other farms (2, 3, 5 and 6) showed no clear textural distributions, with all of them evenly spread between loam and silt-loam categories, and both H/L sites in farms 2, 5 and 8 clustering closely (Figure 4).
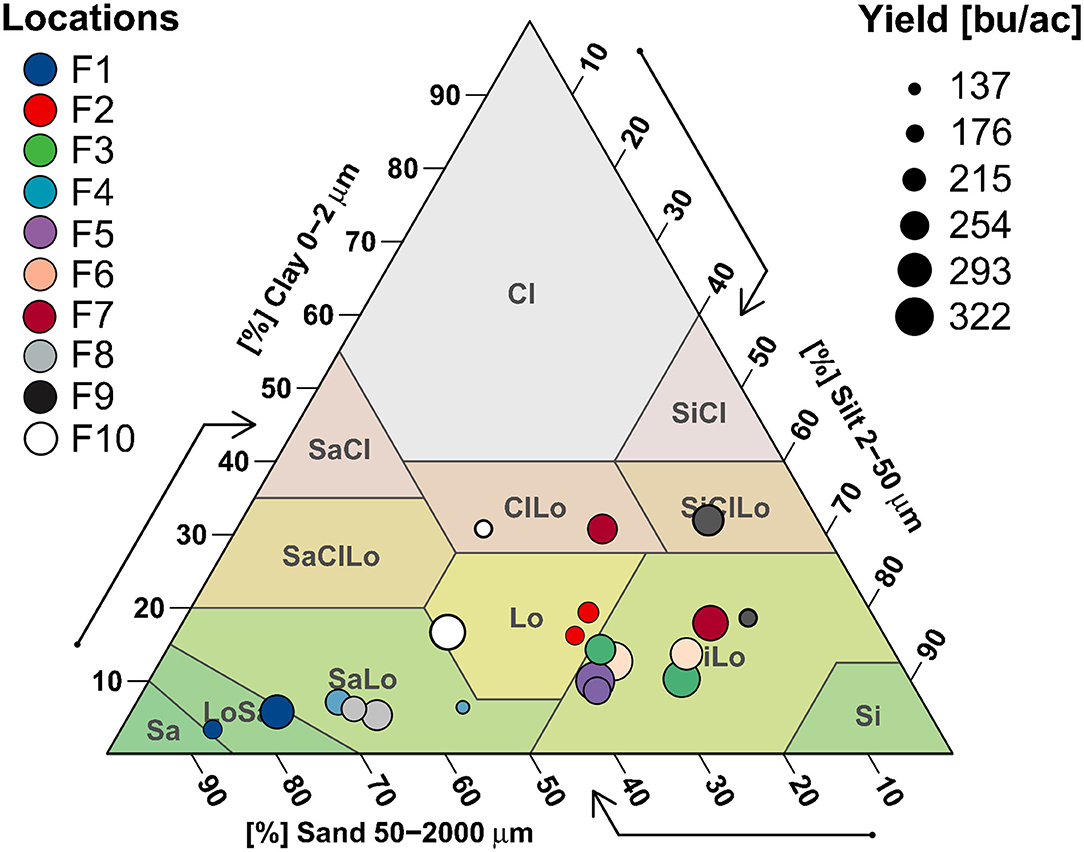
Figure 4. Average soil texture values are mapped onto a USDA Texture Triangle to visualize patterns of soil textural class in relation to farm and yield.
Root Mycobiome Analysis
MiSeq outputs from the four fungal primers are outlined in Table 2. Raw demultiplexed reads ranged from 346,071 to 9,065,818 reads depending on the primer, however, the number of on-target taxa obtained from each primer set was not always reflective of the proportion raw reads. LSUBG and V4AM primers both had a similar number of target taxa (42 and 22) despite ~240-fold difference in raw reads (8.4 million vs. 32,232). The majority of reads from the fungal LSU Basidiomycota/Glomeromycota primers were 9 OTUs from Zea mays (7,220,937 reads) and 5 OTUs from unknown Animalia (949,092 reads). Other non-target OTUs included: Cercozoa, Animalia, Amoebozoa, other Streptophyta, and Ascomycota. ITS2 universal fungal primers yielded 529 target fungi (584,380 reads), spanning with 373 Ascomycota (518,425 reads); 76 Basidiomycota (20,518 reads); 5 Chytridiomycota (2,256 reads); 9 Glomeromycota (194 reads); and Zygomycota (36,101 reads), with few non-target Viridiplantae (15,961 reads) (Figure 5A, Supplementary Table 1). V4AM primers yielded 22 target Glomeromycota (19,648 reads), 20 non-target Fungal OTUs (8,497 reads), Amoebozoa (2,404 reads), and a few others (Table 2). LSUA primers yielded 334 target Ascomycota (984,428 reads) (Figure 5B); 12 non-target Fungi (16,239 reads) including Basidiomycota, Chytridiomycota, Zygomycota, and Glomeromycota; Animalia (161,249 reads); Oomycota (6,621 reads; including Pythium and unknown Pythiales); Viridiplantae (200,247 reads of Zea mays); plus Protozoa (505 reads), Rhizaria, and others.
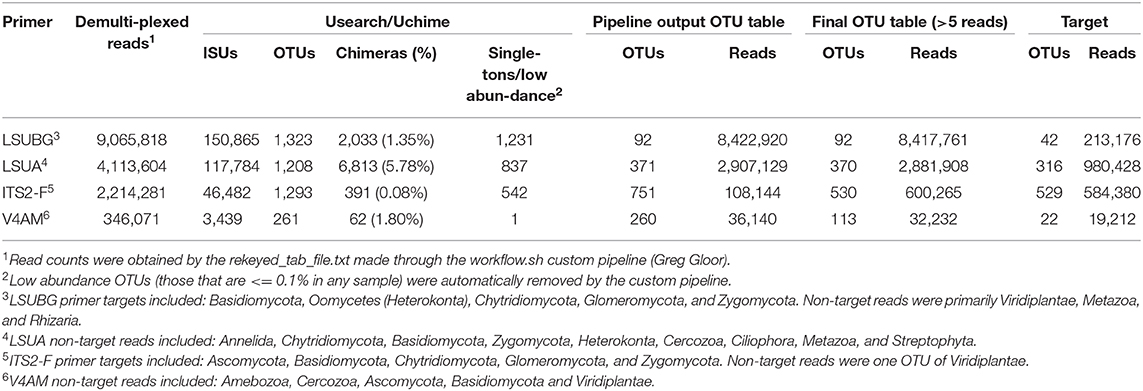
Table 2. Summary of demultiplexed Individual Sequence Units (ISUs) and clustered Operational Taxonomic Units (OTUs) from each of the 4 fungal primer sets used in this study.
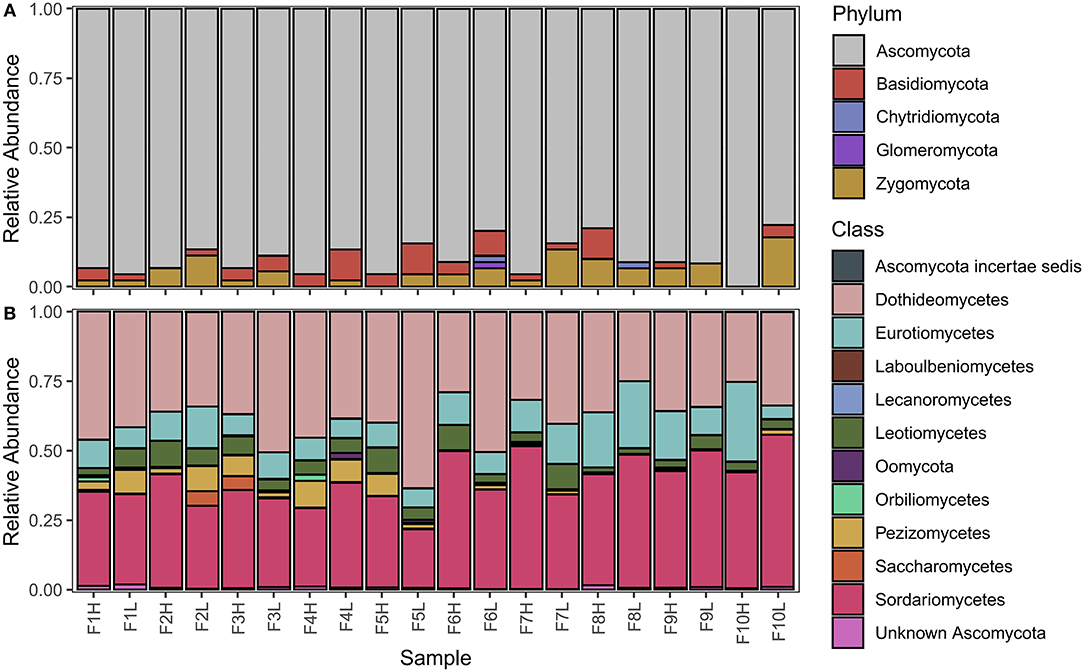
Figure 5. Average relative abundances of corn root fungi communities across all farms. Relative abundance of fungal communities at the (A) phylum level (ITS2 general fungal primer), and the (B) class level (LSU Ascomycota specific primer).
The ALDEx analysis is robust to noise in environmental metagenomic datasets. High (−1.0 ≤ x ≥ 1.0) and moderate (−0.8 > x > −1.0 and 0.8 < x < 1.0) effect sizes highlight important indicator taxa between high and low yield communities within farms. This within-site approach was necessitated due to the high degree of physicochemical variability between farms (and a possible batch effect) that did not allow the algorithm to identify any taxa of interest when considering all sites simultaneously. Instead, a narrowed within-farm analysis identified a total of 163 OTUs (including non-target taxa belonging to Amoebozoa and Platyhelminthes amplified by VAM and LSUBG primers; Supplementary Table 1), and those with relevant ecological strategies are visualized in a taxonomic heatmap (Figure 6).
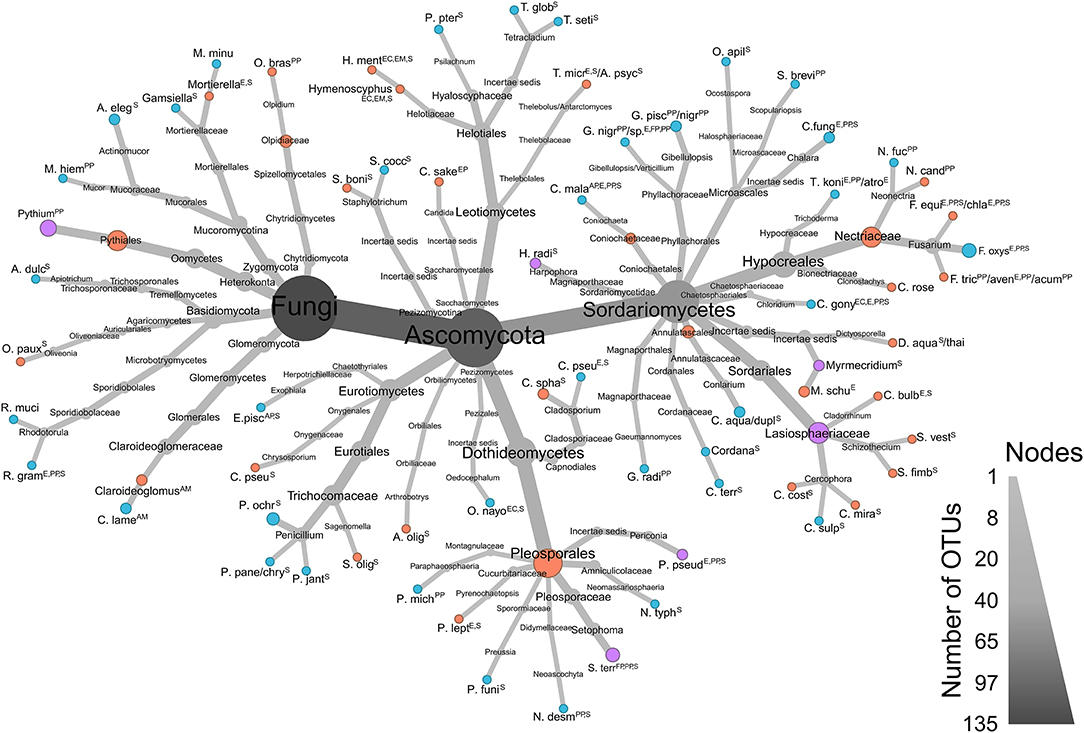
Figure 6. Taxonomy heatmap of significant ALDEx OTUs of ecological importance found in low (red) and high (blue) productivity sites, or in both (purple). Sizes of each node are proportionate to the number of OTUs in each branch. Data from all 4 primer sets (LSUA, LSUBG, ITS2-F, and V4AM) were combined. Ecological guilds for each taxon determined by FUNGuild (Nguyen et al., 2016): AM, Arbuscular mycorrhizal; AP, Animal pathogen; E, Endophyte; EC, Ectomycorrhizal; EM, Endomycorrhizal; EP, Epiphyte; IP, Insect parasite; FP, Fungal pathogen; PP, Plant pathogen; S, Saprotroph. Note: “animal pathogen” not kept for opportunistic animal pathogens; “lichen parasite” deleted; “bryophyte parasite” deleted; “ericoid mycorrhizal” deleted; “orchid mycorrhizal” deleted; “soil saprotroph,” “plant saprotroph” and “wood saprotroph” combined to “saprotroph”.
There are a number of OTUs significantly associated with both high and low yield sites: the saprotrophs Harpophora radicicola (= Phialophora radicicola) and Myrmecridium spp., the potential plant pathogen/endophyte/saprotroph Periconia pseudodigitata, and the arbuscular mycorrhizal fungus Claroideoglomus sp.—amplified by three primers in three low yield farms—and C. lamellosum—amplified twice by two primers in two high yield farms. The plant pathogen Pythium spp.—amplified by two primers—was also detected in three low yield and one high yield farm. In Setophoma terrestris, a fungal pathogen/plant pathogen/saprotroph, three OTUs were significantly associated with low yield sites in farm 6 and one OTU with high yield in farm 4. Plant pathogens Neonectria fuckeliana and N. candida were amplified in high and low yield sites, respectively. Fusarium was represented by three species: four OTUs of F. oxysporum significantly associated with only high yield sites, and one OTU each of F. equiseti/chlamydosporum and F. tricinctum/avenaceum/acuminatum that were significantly associated with only low yield sites. Penicillium species were significantly associated with high yield sites in farms 1, 6, 8, and 10, with a large proportion of significant OTUs found in farm 10. We also observed that farms 2, 4, 8 and 9 had few to no taxa differentially associated with high or low yield sites, although farms 2 and 8 had low yield differences between H and L sites, along with farms 3, 6 and 7 (Table 1, Figure 3). Farms 6 and 10 both had 37 unique significant taxa, farm 7 with 21, farm 5 with 19, and farm 1 with 17 (Supplementary Table 1).
Selected OTUs with ecological strategies of interest (pathogenic and/or beneficial) were visualized by their percent relative abundance within each farm (Figure 7). Relative abundances were typically preserved between OTUs identified as the same species using two separate primers. For example, Pythium sp. (OTUs 49 and 5) captured by LSUA and LSUBG primers had approximately the same range of relative abundances in each farm, although its differential abundance in LSUA-F7 was not reported as significant by ALDEx. In high-yield sites, Fusarium oxysporum, Chalara fungorum, Talaromyces sp., T. diversus, Penicillium ochrochloron, P. janthinellum, P. paneum/chrysogenum, Gibellulopsis spp., Neonectria fuckeliana, and Mucor hiemalis were consistently found, in 8 of 10 replicates in farm 6, 7 times in farm 10, and once in farm 1, 5 and 7. Pythium sp. was consistently found in low yield sites in farms 6 and 7, and Setophoma terrestris and Talaromyces diversus were seen in both high and low yield sites, depending on the location. A large percentage of reads in each farm belonged to S. terrestris or C. fungorum, with some having sites with >30% relative abundance. Penicillium ochrochloron was seen with >20% relative abundance in 6 low-yielding sites in farm 9, using both LSUA and ITS2 primers, and in low-yield sites in farm 8.
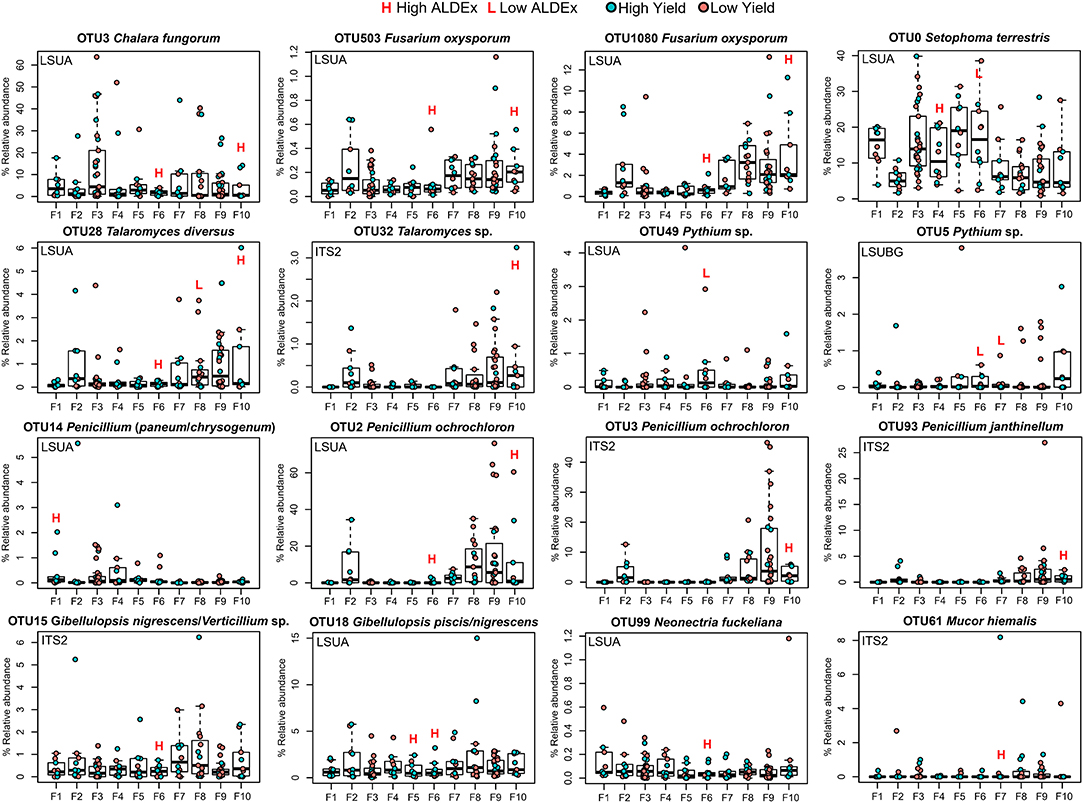
Figure 7. Relative abundance (%) boxplots of significant ALDEx OTUs. Points are colored by site yield (low or high). Outliers are shown beside y-axis values. ALDEx significance (large effect sizes; −1.0 > x > 1) is shown using L/H lettering above corresponding farms.
Based on the results of rarefied (n = 500 OTUs in each sample) LSUA and ITS2 primers, there were significant trends in selected diversity and evenness indices. Non-parametric Kendall–Theil Sen regressions modeled significant linear relationships in both LSUA and ITS2 data between yield and observed OTU richness, Fisher's α (Fisher), and Phylogenetic Diversity (PD; Faith's PD-index) (Faith, 1992) (Figures 8A,B). No significance was seen in either Shannon's or Simpson's diversity indices, and the Abundance-based Coverage Estimator (ACE) was found significant for LSUA but not ITS2 data (data not shown). A strong batch effect was detected in each OTU dataset between the two NGS runs, which may have a stronger effect on mycobiome distribution than location, genetics, soil chemistry, or any other tested factors and partially confound OTU differences between the productivity sites.
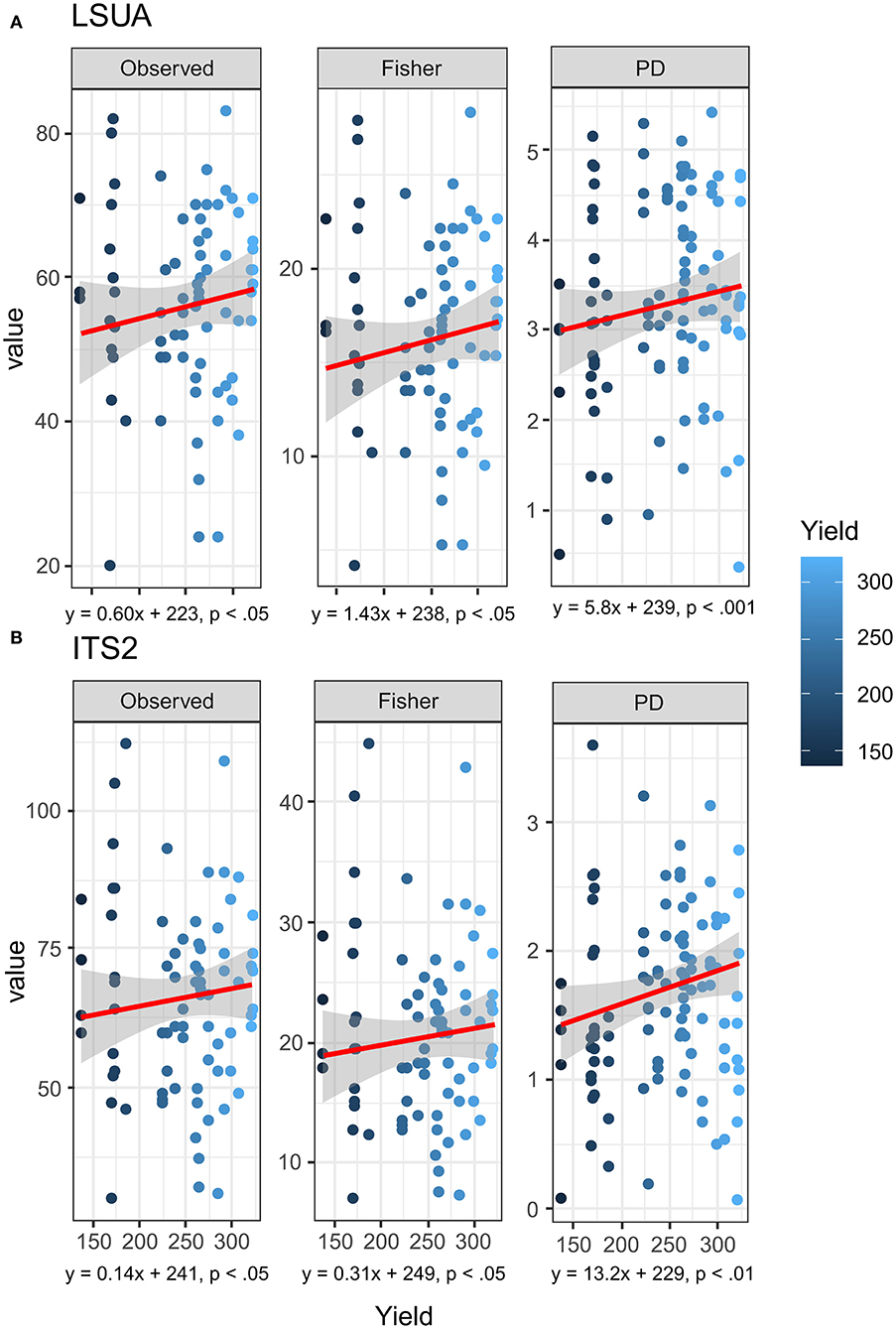
Figure 8. Kendall-Theil Sen Siegel non-parametric linear regressions on rarefied (n = 500 OTU counts) for (A) LSUA and (B) ITS2 primers using multiple diversity and evenness indices: OTU richness (Observed), Fisher's alpha (Fisher) diversity, and Faith's Phylogenetic Diversity (PD) index.
Key Soil Physicochemical and Fungal Factors Related to Yield
A correlation analysis between key fungi, soil physicochemical factors and yield identified three taxa that significantly correlate with soil chemistry (after a Benjamin–Hotchberg p-value adjustment) (Figure 9). Rhodotorula sp., a potential plant pathogen/saprobe/endophyte, was significantly associated with high yield soils in farm 10 (ALDEx analysis) with a significant positive correlation (p < 0.05) with high sand, Fe, and %P saturation. An unknown Hymenoscyphus sp.—endophyte/ectomycorrhizal/saprobe seen in low yield farm 1 soil—was significantly (p < 0.05) positively correlated with high moisture. Both Hymenoscyphus menthae and Setophoma terrestris OTUs were associated with high yield sites, but correlated negatively with moisture (p < 0.05, p < 0.01, respectively). Hymenoscyphus menthae potentially plays a mycorrhizal/saprobic role in soil, and S. terrestris is identified as a potential fungal pathogen/plant pathogen/saprobe. Other non-significant trends can be seen within row clusters, where positive correlations to soil factors on the left side of the heatmap (moisture, OM, sand, Fe, %P saturation, Al and yield) are visible with the topmost OTU cluster, and the opposite trend is seen with taxa in the bottom cluster (a negative correlation with moisture, OM, sand, Fe). OTUs of Clavicipitaceae, Dendryphion, and one F. oxysporum found in high yield soils were positively but not significantly correlated with yield.
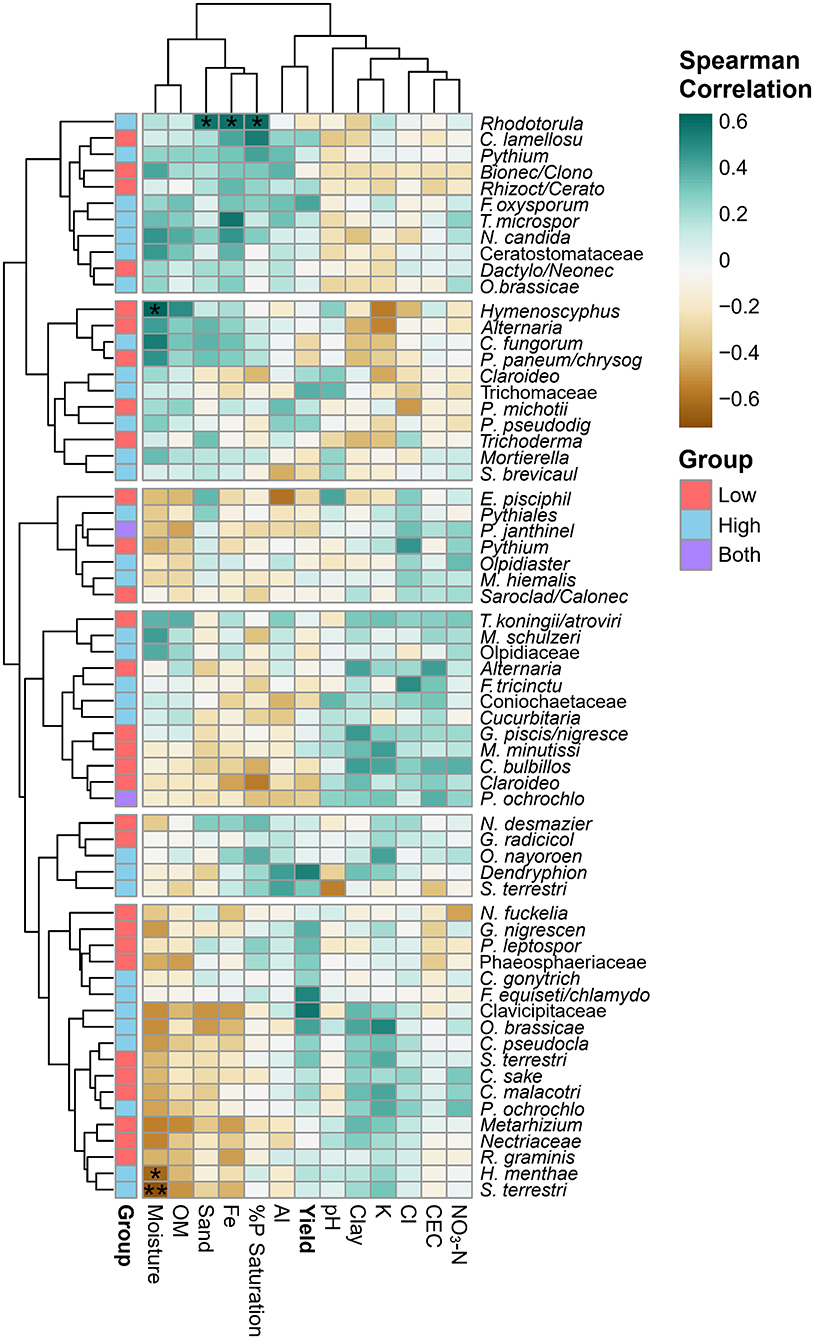
Figure 9. Clustered heatmap of Spearman correlation coefficients between ecologically important indicator taxa (ALDEx) and key soil variables found in this study. Significant Benjamini–Hochberg adjusted p-values are shown with asterisks: *p < 0.05 and **p < 0.01. Top (soil physicochemistry) and left (ALDEx taxa) dendrograms cluster similar factors using the complete linkage method.
A Pearson correlation plot combining yield, soil physical/chemical factors, and select diversity indices (ITS2 Fisher's alpha diversity, LSUA ACE richness estimate, LSUA Phylogenetic Diversity) was used to visualize overall trends in the data (Figure 10). Diversity and evenness indices were trimmed to remove duplicate patterns (i.e., ITS2 and LSUA PD indices correlated identically with other factors, so only one was retained). No single soil physicochemical component or diversity/evenness index highly (>0.75 or < -0.75) correlated with yield, but pH had a strong negative correlation (−0.5 < x < -0.75), confirming that higher yields were typically seen in soils with pH slightly below neutral. Other partially correlated factors included CEC, moisture, OM and Al. The three fungal diversity and evenness indices are also partially correlated with Fe and moisture. Neither Shannon's H′ or Simpson's D were significantly correlated with any other factor (data not shown), confirming the non-parametric nature of the OTU abundances found in these datasets. We intended to develop a structural equation model (SEM) to determine the interaction effects of each variable on the dependent variable (yield). The SEM was weakly correlated, which may be due to underlying complex interactions of numerous soil and other environmental factors that are different in different sites and across farms, as well as the aforementioned batch effect between the two NGS runs.
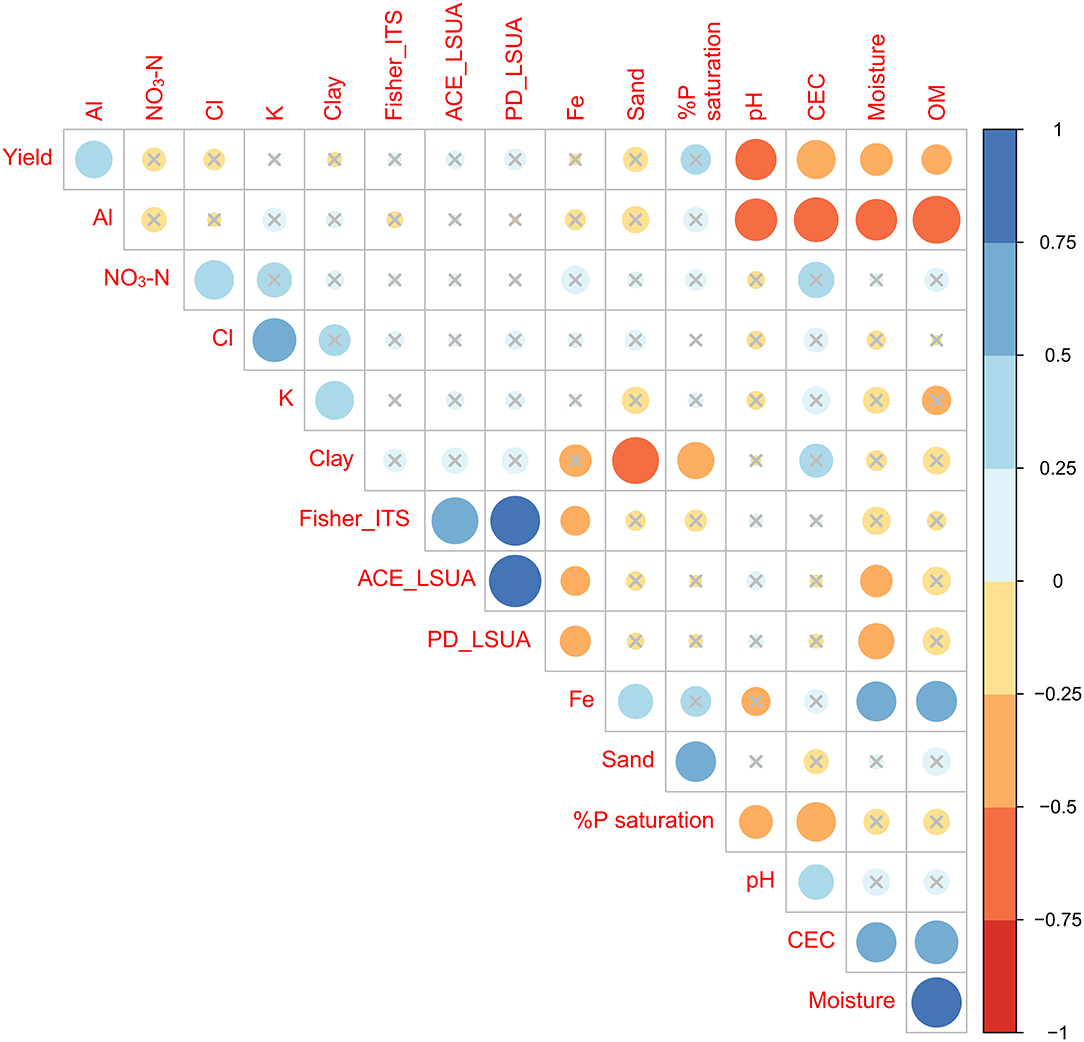
Figure 10. Clustered correlation matrix of key soil factors, significant diversity indices, and yield. Non-significant (p > 0.05) correlation coefficients are marked by gray ×'s.
When combining all the trends in soil chemistry, yield, ad the corn root mycobiome, we see unique patterns within individual farms (Figure 3, Supplementary Table 1). In farm 1, chemical differences in %Al saturation, pH, %Na, Zn, Fe had no relationship with overall differences in corn mycobiomes (non-significant NMDS separation of high and low yield, data not shown), but some interesting indicator taxa, including Claroideoglomus sp., Neomassariosphaeria typhicola, Pythium spp., and Penicillium sp. were observed. In farm 2, no significant relationship between soil physicochemical parameters, yield, or root mycobiomes were observed, most likely due to the negligible difference in yield between sites. Farms 3, 8, and 9 had few differential taxa between high and low yield soils with no significant separation between the total microbial profile of H/L yielding sites. However, noticeable chemical differences including pH, Mn, Al, clay, and Reactive C were observed in farm 9, with a 6.32 t/ha (94 bu/ac) difference in yield between productivity sites. Farm 8 had 2.69 t/ha (40 bu/ac) difference in yield, with moderate differences in chemical factors including pH, Mn, CEC, Mg, and %P saturation. Farm 3, with a 3.03 t/ha (45 bu/ac) difference showed variation in physicochemical factors such as Mn, CEC, Mg, Silt, and B. In these three cases, we can surmise that the mycobiome had little relation to yield when compared to the influence of soil physicochemical parameters, since there were no clear mycobiome separations in NMDS analyses. In farm 4, similar to other high yielding sites, physicochemical factors specifically pH, Mn, OM, %Na, and Zn, seemed to overwhelmingly drive the 6.12 t/ha (91 bu/ac) yield difference, with OM and Zn primarily separating sites. This farm also had a significantly high occurrence of Setophoma terrestris in both high and low sites, and unknown Pythiales in low sites. Farm 7, with a moderate 3.03 t/ha (45 bu/ac) difference in yield, had different pH, Mn, %Al saturation, Al, and clay in soils, with a unique presence of some taxa including beneficial Claroideoglomus spp., pathogenic Olpidiaster sp., Pythium sp., and Murispora sp. Farm 10 had different pH, Mn, %Na, %P saturation, %Al saturation and several distinct fungi, including the pathogens Conlarium sp., Psilachnum pteridii, beneficial fungi including Claroideoglomus, Exophiala pisciphila, Fusarium oxysporum, Penicillium spp. (5 OTUs), and Rhodotorula mucilaginosa between low/high yield sites. Farm 6 was unique in having a clear separation of H/L sites in PCA or NMDS ordinations but having physicochemical factors orthogonal to the plane of site separation, with only high Fe significantly associated with high yield sites (data not shown). Despite this, the yield difference was 3.36 t/ha (50 bu/ac), leading us to presume that there was more stochastic physicochemical variability in low yield sites, and heterogeneous microbial factors potentially affecting to plant health and crop productivity, alongside other unmeasured factors. In farm 6, we identified several fungal species including the potential plant pathogens Pythium sp., Pseudohalonectria sp., Myrmecridium sp., Setophoma sp., Bionectria/Clonostachys sp., and Cladorrhinum bulbillosum in low yield sites. Additionally, there were two Penicillium sp., Conlarium aquaticum/duplumascospora, Chalara fungorum, Gibellulopsis sp., and Trichoderma sp. that were significantly associated with high yield sites. In contrast to farm 6, farm 10 had a clear separation of productive sites in both PCA and NMDS analyses (data not shown) with some fungi species such as Penicillium ochrochloron, P. janthinellum, Claroideoglomus spp., Exophiala pisciphila, and Conlarium aquaticum/duplumascospora present only in high yield sites. Notably, farm 10 also had the largest difference in yield of all sampled sites, 8.61 t/ha (128 bu/ac), and a significant presence of Penicillium in high yield sites. However, it is farm 5 where we saw the highest yield differences–21.52 t/ha (320 bu/ac) vs. 16.07 t/ha (239 bu/ac) in high and low yield sites, respectively. This farm had obvious chemical differences such as pH, Mn, %P saturation, B, and Reactive C, with some unique fungal taxa such as Claroideoglomus sp., Exophiala pisciphila, and Oedocephalum nayoroense, one potentially pathogenic and two beneficial fungi. However, with no clear NMDS/PCA separation based on abundance data, it would suggest that the soil chemistry in farm 5 was a driving factor in the higher overall yield and yield differences.
Discussion
This study focused on associating key fungal species and soil physiochemistry with high or low farm productivity within and across corn fields in southwestern Ontario. Despite the general differences in the 51 soil physicochemical parameters assessed across all of the 10 farms included in this study, factors such as pH, CEC, organic matter, % moisture, % phosphorus saturation, sand, clay, nitrate-nitrogen, potassium, iron, chloride and aluminum linked to site specific productivity differences and the corn root mycobiome. Soil factors, ranked as pH > OM > %Na > Mn > Al, were primarily involved in site separation in farms with the highest yield differences (>3.36 t/ha or 50 bu/ac) compared to the fields with minimal productivity differences. The least influential factors, including %MAC, NO3-N, Cl, and C:N were not correlated with productivity differences. Interestingly, Mn, %Al saturation, Reactive C, B, and Zn—important contributors in individual locations—were not part of the overall yield model, which instead included Sand, pH, % moisture, OM, CEC, %P saturation. Significant positive trends between yield and observed OTU, Fisher's alpha and Phylogenetic Distance were seen, with higher yields having higher richness and diversities in primers amplifying general fungi and Ascomycetes.
It was unsurprising that soil texture (sand and clay) was significant in modeling differences between low and high yield corn in adjacent sites, and across all the farms in the study region. Soil texture classification systems that measure and categorize the percent silt, sand, and clay, allow soil scientists to determine water and nutrient retention/release, both of which control microbial access to soil organic matter (SOM). High clay soils, in particular, limit decomposition by reducing microbial access to SOM or by protecting organic matter from enzyme attack (Baldock and Skjemstad, 2000). Despite management practices that attempt to increase uniformity (tillage, fertilization, digital field mapping), soil heterogeneity still occurs at the field level, and this clearly still has a strong effect on plant productivity, and the incidence of pests and pathogen loads (Dordas, 2008; Patzold et al., 2008; Hbirkou et al., 2011; Veresoglou et al., 2013).
Differences in abiotic and biotic factors in soils as well as stochastic processes are major contributors to productivity differences within sites having the same cropping history, crop genotype, and management practices (Chang et al., 2017). There were 8 different hybrid corn varieties among the 10 sites (Table 1), so it was difficult to correlate yield or mycobiome differences with corn variety. Many researchers claim that plant genotypes are responsible for the composition of root exudates that influence the microbial recruitment in the rhizosphere (Patel et al., 2015), and we can assume that a portion of the overall unexplained variation was also due to genotypic variations between cultivars. Soil characteristics, types of fertilization, and crop management practices can all strongly influence soil properties, rhizosphere and root microbial abundance, diversity, and resilience (Xu et al., 2009; Antoninka et al., 2015; Tkacz and Poole, 2015), as well as crop and soil productivity in changing environments, climatic conditions, and increased anthropogenic activities (Stockmann et al., 2015; Pii et al., 2016). There was no clear, singular mechanism linking productivity differences to the mycobiome in our study, which might be due to multiple soil and climatic factors including but not limited to soil physicochemical parameters such as moisture content, water holding capacity, particle size differences, difference in the cropping regimes of individual farms, geographic location of the farm, stage and time of sampling, underlying differences in pathogen pressure depending on farm-specific rotation practices, and differences in functional community abundance and diversity driven by years of repeated farming practices. Physicochemical parameters such as pH, %P saturation, CEC, sand, and clay showed a strong relationship with corn productivity in most of the fields sampled in this study. Tedersoo et al. (2014) found the association of edaphic factors such as mean annual precipitation, pH and the concentrations of P and Ca with fungal diversity. Fungal communities may have different response patterns to fertilization and crop rotation than bacteria. In a study by Ai et al. (2018), soil fungi were more affected by crop rotation type (wheat-maize and wheat-soybean), indicating that plant-fungal communities were more tightly linked to one another than bacterial-fungal or bacterial-plant communities in arable soils (Cassman et al., 2016).
Chen et al. (2020) demonstrated that a balanced fertilization regime of P and K increased yield by enhancing the activity of PO4 solubilizing microbes, and fertilization using only N and K did not increase yield, indicating that in addition to balancing fertilization, other approaches are required for quickly improving crop yield according to the nature of the soil. Our results corroborate this finding, asserting that the right chemical balance is essential for promoting the establishment of beneficial mycobiomes that are key to enhancing plant productivity. Despite the data suggesting a strong link between crop productivity and selected soil factors, we also see different mycobiomes between healthy and unhealthy sites within farms. There were numerous known antagonistic or beneficial fungi identified in the ITS2 and LSUA datasets, alongside others that did not have any immediately clear ecological roles. In some cases, fungi that are commonly regarded as pathogenic were found in high yield soils in significantly higher abundances than in low yield soils, while the opposite was true in other cases. This could indicate the possibility of non-pathogenic strains of known fungal pathogens (Chulze et al., 2014) or alternative ecological roles that have yet to be revealed.
Corn producing higher yields had higher root-associated fungal phylogenetic diversity and OTU richness than low yield corn in this study (considering general fungi as well as only ascomycetes). However, because the ITS2 primer preferentially amplifies the shorter Ascomycota over the longer Basidiomycota amplicons (see Technical Shortfalls), it is likely that this significant result in both primers (ITS2 and LSUA) is mostly driven by the overrepresentation of the Ascomycota in our datasets. Soil structure and stability has been linked to higher bacterial (Awasthi et al., 2014; Tardy et al., 2014; Maron et al., 2018) and fungal (Yang et al., 2017) functional and taxonomic diversity. In this case, high root-associated fungal diversity may be linked to corn variety-specific root exudates that are released in order to recruit healthy mycorrhizospheres (Rillig and Mummey, 2006), that in turn support plant growth through pathogen protection, nutrient acquisition, and other mechanisms (Shiomi et al., 2006; Ikram et al., 2020). Unfortunately, there was an inadequate number of corn hybrid replicates across farms to test this interaction. Higher soil and root-associated fungal diversity and richness may also be attributed to management differences including cover cropping or specific crop rotations that promote the colonization of the soil rhizosphere (Lehman et al., 2012; Njeru et al., 2014; Borrell et al., 2017). Lehman et al. (2012) demonstrated that a fall cover cropping mixture (oats–pea) had significantly higher AMF propagules g−1 than no-crop. Lower diversity (and potentially lower yield) can also be the result of degradative management practices including long-term fertilization (Beauregard et al., 2010; Zhou et al., 2016), or tillage (Schmidt et al., 2019).
Soil chemical and/or mycobiome profiles are fairly distinct in all the sampled locations, except for farm 2 and 4. No obvious differences were seen in soil physicochemical parameters, the corn root mycobiome, or yield in farm 2, which may be due to environmental impact on the quality of NDVI imaging (Johnson et al., 2018). Otherwise, it is possible that sources of plant stress during the imaging stage was subsequently corrected through natural or mediated farming practices, resulting in narrowing yield differences at the time of sampling. Extremely high levels of OM in farm 4 likely masked the influence of other factors on productivity and the mycobiome. As reported by Sul et al. (2013), soil organic matter was the most important factor explaining differences in the microbial community structure in agricultural soils. Despite farm 10 having the largest yield difference between stressed and healthy plants, it was farm 5 that exemplified an ideal harvest situation, where the healthy corn plants had the highest yield of all measured sites (21.52 t/ha; 320 bu/ac), not to mention a substantially higher yield in stressed, low yield plots (16.07 t/ha; 239 bu/ac) than average Ontario values (10.33 t/ha; 153.6 bu/ac). This may be due to synergistic effects between optimal soil health conditions, the mycobiome, crop genetics, management practices, and other unmeasured variables (see below). Farm 5 also has a silt-loam soil type, one that is typically the best suited for agricultural purposes (Haraldsen et al., 2000).
The mycobiome species identified in this study may be affected by differences in crop genetics, cropping regimes, farm management, stochastic soil processes, heterogeneous microbial distributions, on top of any other indiscernible effects of soil physiochemistry and abiotic/biotic factors. Some of these unmeasured variables potentially affecting our study include biotic factors such as nematodes, earthworms, and rhizosphere/bulk soil bacteria, as well as abiotic factors such as water table height, growing season temperature, and monthly precipitation. Plant-parasitic nematodes (e.g., root-knot and root-lesion nematodes) have long since been implicated in corn, soybean, wheat, tomato, and other crop losses in North America (McSorley and Gallaher, 1993; Grabau and Chen, 2016; Simon et al., 2018), but were not measured or considered in this paper. Bacterial populations are also an essential component of corn-soil-rhizosphere interactions (Song et al., 2007; Li et al., 2018), but their influence on biotic and abiotic factors in our study sites were not within the scope of this paper. Other unmeasured abiotic factors in this study include groundwater, precipitation, and temperature. Water in all ten farm sites may be an important but incompletely measured variable since soil moisture data was taken only at the time of sample collection. In Ontario, there is generally not enough groundwater available to replenish corn agricultural water use and evaporation. Corn requires ~50 cm (20 in.) of water to produce high yields and this can be supplied over the growing season from a combination of stored water in the soil, rainfall or irrigation (OMAFRA, 2017). In 2017, the average total rainfall levels across sites was 34 cm (https://climate.weather.gc.ca), which necessitated spite specific watering by farmers to prevent drought conditions. Management practices such as differences in planting date, planting density, irrigation (timing of), fertilizer applications, weed management techniques (including cover and companion cropping), etc. (Scharf et al., 2002; Bruns and Abbas, 2006; Kucharik, 2006; Van Roekel and Coulter, 2011; Yeganehpoor et al., 2015) are difficult to control for in studies comparing yield differences between farms. In addition to this, the development of various hybrid varieties further introduces sources of variation between locations since each variety is developed for different soil physicochemical and environmental systems (Troyer, 2009; OMAFRA, 2017). These and many other components may have increased the proportion of unexplained variation in the mycorrhizosphere in our study.
Farms 6 and 10 had more potentially influential taxa than all other farms in this study. Two OTUs identified as Fusarium oxysporum were significantly associated with high yield sites in farms 6 and 10, but also in high relative abundances in farms 2, 7, and 9. Fusarium equiseti/chlamydosporum and F. tricinctum/avenaceum/acuminatumi OTUs were also significantly associated with low yield sites within farms 7 and 10. In agriculture, pathogenic Fusarium species, including F. oxysporum and F. equiseti, among others, are known to primarily cause corn ear and kernel rot (Wall and Mortimore, 1965). However, F. oxysporum is also considered a common member of the plant rhizosphere as a saprotroph (Gordon and Martyn, 1997), and all strains can grow and survive for long periods of time on plant matter in the soil and rhizosphere (Garrett, 1970). Pathogenic species of Fusarium produce a wide range of mycotoxins (Rep and Kistler, 2010), have a high level of host specificity, and cause root rot, although others have been found in roots without any disease symptoms (Palmer and Kommedahl, 1969; Olivain and Alabouvette, 1997); all of this depending on the plant species or cultivar. Fusarium oxysporum was found as one of the predominant species (at 12.1% isolation frequency) from rotted maize ears and kernels in Chongquing (Zhou D. et al., 2018), and 76% of soil isolates and 35% of stem isolates in New Zealand (Harrow et al., 2010), whereas F. equiseti was found at 3.4% isolation frequency (Zhou D. et al., 2018). This species is considered ubiquitous in cropping soils and cereal stem bases (Harrow et al., 2010) and was seen in our study as well. It is therefore unsurprising that F. oxysporum was found in both low and high-yield sites in our study. The F. avenaceum/acuminatum/tricinctum species complex (OTU469 in low yield farm 10 sites) is one that has been linked to Fusarium head blight. In temperate regions, Fusarium avenaceum is similar to F. tricinctum, which is often considered to be a relatively weak pathogen of cereals (Harrow et al., 2010), and morphologically similar to F. acuminatum, identified as a causative agent in crown and root rot of wheat (Rep and Kistler, 2010). Harrow et al. (2010) found that F. avenaceum was most commonly found in stems but in lower abundances in the soil, indicating their ability to survive for short periods of time.
A fungus identified as Chalara fungorum was amplified by the Ascomycete-specific primers with high levels of occurrence across many farms; it was significantly associated with high yield sites in farms 6 and 10 and found in high relative abundance in farm 3. The anamorphic genus Chalara represents a polyphyletic assemblage of species with similar morphologies (Koukol, 2011), and the true identity of this OTU remains to be determined. The genus contains some aggressive primary plant pathogens responsible for oak-wilt (conidial stage Chalara quercina) (Raj and Kendrick, 2006), the causal agent of narrow-leaved ash dieback (C. fraxinea) (Kowalski and Holdenrieder, 2009), myrtle beech dieback in Australia (C. australis) (Kile and Walker, 1987), and on Pseudocyphellaria “speckle belly” lichens (C. pseudocyphellariae) (Etayo and Sancho, 2008; Koukol, 2011). However, most C. fungorum observations are as saprobic dematiaceous anamorphs on plant litter—coniferous needles, cones, wood or bark, in temperate and tropical regions, with several recurring on particular substrates (Koukol, 2011). It may also be fungicolous as it was originally described on an old fruiting body of a Hydnellum (Saccardo, 1886). Its significant association with high yield sites might be due to its role as a saprobe, but it has the possibility of being a beneficial root-associated partner.
Penicillium ochrochloron, P. janthinellum and P. paneum/chrysogenum were all significantly associated with high yield sites in farms 1, 6 and 10. Penicillium species are famous for their production of antibiotics and secondary metabolites with interesting biological activity, and so their significant occurrences in high yield sites lead us to categorize their roles as beneficial fungi in this study. Species such as P. ochrochloron show activity against Gram positive and negative bacteria, as well as Candida albicans (Rančić et al., 2006), and chitinase extracts from this species have shown to affect the growth of larvae of Helicoverpa armigera, a common worldwide crop insect pest (Patil and Jadhav, 2015). Additionally, P. ochrochloron has been known to solubilize phosphate (Sánchez-de Prager and Cisneros-Rojas, 2017) through the production of citric and malic acid (Coutinho et al., 2012). It is therefore possible that recruitment or proliferation of Penicillium in corn roots may have contributed to yield increases in our high yield sites.
Two Trichoderma OTUs were significantly associated with high yield sites in farm 6. Trichoderma species (T. hamatum and T. koningii) can induce biofertilization of crops and can increase production up to 300% (Benítez et al., 2004). Trichoderma is also considered a bio-parasite, and has been proposed as a biocontrol agent for phytopathogenic fungi such as Bipolaris oryzae, Fusarium spp., Rhizoctonia solani, Sclerotium rolfsii, Phytophthora cactorum, Sclerotinia sclerotiorum, B. cinerea, and Pythium spp. (Benítez et al., 2004; Anuar et al., 2019; Arifin and Ilham, 2019; Sanchez et al., 2019). Inoculation of Trichoderma sp. in soil fertilized by tricalcium phosphate showed an increase in plant biomass (dry weight and root) of chickpea plants (Kapri and Tewari, 2010).
Despite farming practices that attempt to increase homogeneity, a field's topography, water table, soil erosion patterns, stochastic processes and other factors (Girvan et al., 2003) continue to create pockets of low productivity within average-to-high producing fields. To sustain demanding agricultural practices, inclusive soil health assessments such as physicochemical balances and biological indicators of soil quality are needed (Doran and Zeiss, 2000). Unraveling the association of the mycobiome with its contribution to rhizosphere health and soil quality will identify which fungi are recruited to or naturally proliferate within high or low productive soil environments, and further uncover the roles of fungi in agricultural systems. Our results suggest that the associations between soil edaphic and biotic factors, the mycobiome, and productivity are complex and that no single fungus or set of a few fungi drive yield in positive or negative ways.
Technical Shortfalls
The general fungal LSU primers did not recover an adequate number of fungal reads to be a reliable choice for plant tissues due to the large proportion of Zea mays reads from root DNA (85.7% of all reads). These primers were originally developed for use in soil samples (Asemaninejad et al., 2016), in which they understandably have better performance than with root-associated DNAs. Unfortunately, this leaves the Basidiomycota poorly represented since the LSUA primers have multiple mismatches to members of Basidiomycota, and Illumina reads of ITS2 amplicons will favor the shorter (and thus more abundant) amplicons of Ascomycota rather than Basidiomycota, which are 30–190 bp longer (Bellemain et al., 2010).
Batch effects were also a confounding factor in our ordination analyses and none of the batch removal tools employed through R resulted in any datasets with visible patterns or clusters. This lead us to believe that (a) the sampling effect differences between runs were strong, (b) it may be difficult to truly generalize clustering patterns between low and high yield sites across farms with different corn varieties, geographic locations, farming/amendment practices, and soil types, and (c) future studies with similar designs will require more randomization of samples between runs to help reduce batch effects.
Future Research
An immediate next step will be expanding our research to further confirm the key and stable fungal taxa associated with high/low productivity sites by involving structural equation models (SEM), which may help understand stochastic and deterministic ecosystem processes (Antoninka et al., 2009; Pascual-García and Bell, 2020). We hope that a better understanding of the ecological roles of relatively abundant taxa associated with farm-specific productivity will enable the development of biological products that help to improve under-productive sites. Our preliminary list of fungal root-associated taxa in this study is a starting point for this selection. Further exploring mechanisms to balance key soil physicochemical factors will help to establish and sustain a healthy, beneficial mycobiome. Alongside corn, wheat and soybeans are common rotational crops in Ontario that will also benefit from similar in-depth analyses into root mycobiomes and how they may vary across soil types, farming practices, and overall yields. Analyses like this may help to improve soil productivity regardless of crops grown and improve the nutrient use efficiency as well as reduce the overuse of chemical fertilizer. Excessive agrochemicals, such as N fertilizer, tip nutrient balances and are often not completely consumed by plants (Magdoff, 1991). This is not an economically sustainable practice and results in large-scale environmental issues such as terrestrial and aquatic eutrophication, acidification, atmosphere pollution, and the creation of large hypoxic zones in freshwater and marine water bodies (Good and Beatty, 2011). There will continue to be an incentive to replace short-term fertilization for immediate yield gains with long term sustainable practices to keep up with predicted high future demand for cereal production resulting from increases to the global population and subsequent dietary shifts, especially in underdeveloped regions (FAO, 2009). Developed countries have already nearly reached the maximum biological yield potentials of crops using fertilizers, but we cannot sustain these levels of production without serious consequences (Good and Beatty, 2011). By looking into site-specific farming practices that incorporate biological-fertilizers and natural mechanisms for plant growth promotion, we can reduce the need for damaging long-term abiotic amendments. There is a need to explore the dynamics of stress-tolerant microbes in relation to host species in various habitats and geographical locations to reduce sources of crop stress, the need for excessive farm inputs, and crop production heterogeneity.
Conclusion
Understanding the deterministic process involved in shaping the biogeography of microbial communities and its association with plant productivity remains a mystery, but our research has taken a step to provide some insights through large scale field-based research. In this study we identified soil physicochemical factors and corn root associated fungi within and between low and high producing farms in southwestern Ontario. When modeling for yield differences across farms with varying soil chemical factors, pH, moisture, %P saturation, sand, clay CEC, and OM levels helped to predict corn yield. When looking at individual farms, low yielding sites were often correlated with less than ideal levels of one or more of soil physicochemical factors such as pH, Mn, OM, %Na, CEC and Mg. Associated indicator root fungi were distinct in most of the high or low yielding soils within farms but could not be generalized across farms, perhaps because of variation in soil physicochemical parameters. However, higher yield sites had greater fungal diversity (Fisher's α and phylogenetic diversity) than lower yield sites. Locations with large differences in yield (>80 bu/ac or 5.38 t/ha) had substantial differences in soil chemistry, structure, and/or fungal indicator taxa. This confirms previous studies that claim an imbalance in soil physicochemistry not only directly affects crop productivity but may also indirectly influence productivity by modifying the abundance and diversity of soil myco- and microbiomes. Different combinations of fungi and soil features, in addition to other edaphic and biotic components, lead to differences in site-specific plant productivity that are unique to each farm location, which further emphasizes the importance of personalized farm prescriptions to improve pockets of under-productive farm locations. A better understanding of the identities and ecological roles of fungi constituting the soil mycobiome will be needed to bring prescriptive management for sustainable agriculture.
Data Availability Statement
The datasets presented in this study can be found in online repositories. The names of the repository/repositories and accession number(s) can be found below: https://www.ebi.ac.uk/ena, PRJEB40800.
Author Contributions
SK, GL, and RGT contributed to the conception and design of the study. SK sampled the fields and did all the meta data analysis. SK and SA took part in sample processing at the lab. SK and GS prepared NGS libraries. NW performed bioinformatic analysis of NGS data. SK, NW, RGT, GL, and GP participated in data interpretation. NW and SK wrote the manuscript. SK, NW, RGT, and GL reviewed and edited the manuscript. SK, NW, RGT, and GL contributed to manuscript revision, and all coauthors read and approved the final version.
Funding
Funding provided by NSERC, AAFC growing forward 2, Ontario Center of Excellence (OCE) VIP II and ECO Canada programs was crucial to the successful completion of this project.
Conflict of Interest
SK, NW, GS, GP, SA, and GL were employed by the company A & L Canada Laboratories Inc.
The remaining author declares that the research was conducted in the absence of any commercial or financial relationships that could be construed as a potential conflict of interest.
Acknowledgments
We thank and appreciate the assistance of all the participating farmers for allowing us to sample in their fields. We would also like to thank S. Saldias and other A&L Biologicals staff and co-op students for their immeasurable help on this project.
Supplementary Material
The Supplementary Material for this article can be found online at: https://www.frontiersin.org/articles/10.3389/fsufs.2021.617332/full#supplementary-material
References
Adesemoye, A. O., Torbert, H. A., and Kloepper, J. W. (2008). Enhanced plant nutrient use efficiency with PGPR and AMF in an integrated nutrient management system. Can. J. Microbiol. 54, 876–886. doi: 10.1139/W08-081
Ai, C., Zhang, S., Zhang, X., Guo, D., Zhou, W., and Huang, S. (2018). Distinct responses of soil bacterial and fungal communities to changes in fertilization regime and crop rotation. Geoderma 319, 156–166. doi: 10.1016/j.geoderma.2018.01.010
Ali, S., Saldias, S., Weerasuriya, N., Delaney, K., Kandasamy, S., and Lazarovits, G. (2020). Corn microbial diversity and its relationship to yield. Can. J. Microbiol. 66, 457–473. doi: 10.1139/cjm-2020-0002
Antoninka, A. J., Ritchie, M. E., and Johnson, N. C. (2015). The hidden Serengeti – mycorrhizal fungi respond to environmental gradients. Pedobiol. J. Soil Ecol. 58, 165–176. doi: 10.1016/j.pedobi.2015.08.001
Antoninka, A. J., Wolf, J. E., Bowker, M., Classen, A. T., and Johnson, N. C. (2009). Linking above- and belowground responses to global change at community and ecosystem scales. Glob. Chang. Biol. 15, 914–929. doi: 10.1111/j.1365-2486.2008.01760.x
Anuar, I. S. M., Gani, H. A., Safi'n, N. N., Samsudin, K. A., and Wahab, M. Z. (2019). Alginate encapsulation of Trichoderma harzianum as biocontrol agent against brown spot disease on rice (Oryza sativa) in vitro assays. Int. J. Adv. Sci. Eng. Inf. Technol. 9, 461–466. doi: 10.18517/ijaseit.9.2.3226
Arifin, S., and Ilham, M. (2019). “The biocontrol ability test of Trichoderma harzianum toward damping off desease on soybean seedlings,” in Journal of Physics: Conference Series (Bristol: IOP Publishing), 12045. doi: 10.1088/1742-6596/1232/1/012045
Asemaninejad, A., Weerasuriya, N., Gloor, G. B., Lindo, Z., and Thorn, G. (2016). New primers for discovering fungal diversity using nuclear large ribosomal DNA. PLoS ONE 11:e0159043. doi: 10.1371/journal.pone.0159043
Astolfi, S., Zuchi, S., Cesco, S., Varanini, Z., and Pinton, R. (2004). Influence of iron nutrition on sulphur uptake and metabolism in maize (Zea mays L.) roots. Soil Sci. plant Nutr. 50, 1079–1083. doi: 10.1080/00380768.2004.10408577
Awasthi, A., Singh, M., Soni, S. K., Singh, R., and Kalra, A. (2014). Biodiversity acts as insurance of productivity of bacterial communities under abiotic perturbations. ISME J. 8, 2445–2452. doi: 10.1038/ismej.2014.91
Baldock, J. A., and Skjemstad, J. O. (2000). Role of the soil matrix and minerals in protecting natural organic materials against biological attack. Org. Geochem. 31, 697–710. doi: 10.1016/S0146-6380(00)00049-8
Baliyarsingh, B., Nayak, S. K., and Mishra, B. B. (2017). “Soil microbial diversity: an ecophysiological study and role in plant productivity,” in Advances in Soil Microbiology: Recent Trends and Future Prospects (Singapore: Springer), 1–17. doi: 10.1007/978-981-10-7380-9_1
Bargaz, A., Noyce, G. L., Fulthorpe, R., Carlsson, G., Furze, J. R., Jensen, E. S., et al. (2017). Species interactions enhance root allocation, microbial diversity and P acquisition in intercropped wheat and soybean under P deficiency. Appl. Soil Ecol. 120, 179–188. doi: 10.1016/j.apsoil.2017.08.011
Bashan, Y. (1998). Inoculants of plant growth-promoting bacteria for use in agriculture. Biotechnol. Adv. 16, 729–770. doi: 10.1016/S0734-9750(98)00003-2
Battaglia, T. (2020). btools: A suite of R function for all types of microbial diversity analyses. R package version 0.0.1. Available online at: https://CRAN.R-project.org/package=btools
Beauregard, M. S., Hamel, C., and St-Arnaud, M. (2010). Long-term phosphorus fertilization impacts soil fungal and bacterial diversity but not AM fungal community in alfalfa. Microb. Ecol. 59, 379–389. doi: 10.1007/s00248-009-9583-z
Beck, M. W. (2015). Collinearity and stepwise VIF selection. R-bloggers. Available online at: https://gist.github.com/fawda123/4717702#file-vif_fun-r (accessed October 30, 2019).
Behn, O. (2008). Influence of Pseudomonas fluorescens and arbuscular mycorrhiza on the growth, yield, quality and resistance of wheat infected with Gaeumannomyces graminis. J. Plant Dis. Prot. 115, 4–8. doi: 10.1007/BF03356232
Bellemain, E., Carlsen, T., Brochmann, C., Coissac, E., Taberlet, P., and Kauserud, H. (2010). ITS as an environmental DNA barcode for fungi: an in silico approach reveals potential PCR biases. BMC Microbiol. 10:189. doi: 10.1186/1471-2180-10-189
Benítez, T., Rincón, A. M., Limón, M. C., and Codón, A. C. (2004). Biocontrol mechanisms of Trichoderma strains. Int. Microbiol. 7, 249–260.
Berg, G. (2009). Plant–microbe interactions promoting plant growth and health: perspectives for controlled use of microorganisms in agriculture. Appl. Mcrobiology Biotechnol. 84, 11–18. doi: 10.1007/s00253-009-2092-7
Bilal, L., Asaf, S., Hamayun, M., Gul, H., Iqbal, A., Ullah, I., et al. (2018). Plant growth promoting endophytic fungi Aspergillus fumigatus TS1 and Fusarium proliferatum BRL1 produce gibberellins and regulates plant endogenous hormones. Symbiosis 76, 117–127. doi: 10.1007/s13199-018-0545-4
Blaser, M. J. (2016). Antibiotic use and its consequences for the normal microbiome. Science 352, 544–545. doi: 10.1126/science.aad9358
Borrell, A. N., Shi, Y., Gan, Y., Bainard, L. D., Germida, J. J., and Hamel, C. (2017). Fungal diversity associated with pulses and its influence on the subsequent wheat crop in the Canadian prairies. Plant Soil. 414, 13–31. doi: 10.1007/s11104-016-3075-y
Bruns, H. A., and Abbas, H. K. (2006). Planting date effects on Bt and non-Bt corn in the Mid-South USA. Agron. J. 98, 100–106. doi: 10.2134/agronj2005.0143
Caporaso, J. G., Lauber, C. L., Walters, W. A., Berg-Lyons, D., Lozupone, C. A., Turnbaugh, P. J., et al. (2011). Global patterns of 16S rRNA diversity at a depth of millions of sequences per sample. PNAS 108(Suppl. 1), 4516–4522. doi: 10.1073/pnas.1000080107
Cassman, N. A., Leite, M. F. A., Pan, Y., De Hollander, M., Van Veen, J. A., and Kuramae, E. E. (2016). Plant and soil fungal but not soil bacterial communities are linked in long-term fertilized grassland. Sci. Rep. 6, 1–11. doi: 10.1038/srep23680
Chang, H.-X., Haudenshield, J. S., Bowen, C. R., and Hartman, G. L. (2017). Metagenome-wide association study and machine learning prediction of bulk soil microbiome and crop productivity. Front. Microbiol. 8:519. doi: 10.3389/fmicb.2017.00519
Chen, M., Xu, J., Li, Z., Zhao, B., and Zhang, J. (2020). Soil physicochemical properties and bacterial community composition jointly affect crop yield. Agron. J. 112:4358–4372. doi: 10.1002/agj2.20358
Chulze, S. N., Palazzini, J. M., Torres, A. M., Barros, G., Ponsone, M. L., Geisen, R., et al. (2014). Biological control as a strategy to reduce the impact of mycotoxins in peanuts, grapes and cereals in Argentina. Food Addit. Contam. Part A 32, 471–479. doi: 10.1080/19440049.2014.984245
Cook, R. J. (2007). “Take-all decline: model system in the science of biological control and clue to the success of intensive cropping”, in Biological Control a Global Perspective: Case Studies from Around the World, eds. C. Vincent, M. Goettel, and G. Lazarovits (Wallingford: CAB International), 399–414. doi: 10.1079/9781845932657.0399
Coutinho, F. P., Felix, W. P., and Yano-Melo, A. M. (2012). Solubilization of phosphates in vitro by Aspergillus spp. and Penicillium spp. Ecol. Eng. 42, 85–89. doi: 10.1016/j.ecoleng.2012.02.002
Doran, J. W., and Zeiss, M. R. (2000). Soil health and sustainability: managing the biotic component of soil quality. Appl. Soil Ecol. 15, 3–11. doi: 10.1016/S0929-1393(00)00067-6
Dordas, C. (2008). Role of nutrients in controlling plant diseases in sustainable agriculture. A review. Agron. Sustain. Dev. 28, 33–46. doi: 10.1051/agro:2007051
Dregne, H. E. (2002). Land degradation in the drylands. Arid L. Res. Manag. 16, 99–132. doi: 10.1080/153249802317304422
Edgar, R. C. (2010). Search and clustering orders of magnitude faster than BLAST. Bioinformatics 26, 2460–2461. doi: 10.1093/bioinformatics/btq461
Edgar, R. C., Haas, B. J., Clemente, J. C., Quince, C., and Knight, R. (2011). UCHIME improves sensitivity and speed of chimera detection. Bioinformatics 27, 2194–2200. doi: 10.1093/bioinformatics/btr381
Etayo, J., and Sancho, L. G. (2008). Hongos Liquenícolas del sur de Sudamérica, Especialmente de Isla Navarino (Chile). Berlin: J. Cramer Verlag.
Faith, D. P. (1992). Conservation evaluation and phylogenetic diversity. Biol. Conserv. 61, 1–10. doi: 10.1016/0006-3207(92)91201-3
FAO (2009). How to feed the World in 2050. Food Agric. Organ. United Nations. Available online at: http://www.fao.org/fileadmin/templates/wsfs/docs/expert_paper/How_to_Feed_the_World_in_2050.pdf (accessed December 16, 2019).
Fernandes, A. D., Macklaim, J. M., Linn, T. G., Reid, G., and Gloor, G. B. (2013). ANOVA-like differential expression (ALDEx) analysis for mixed population RNA-Seq. PLoS ONE 8:e67019. doi: 10.1371/journal.pone.0067019
Fierer, N., Breitbart, M., Nulton, J., Salamon, P., Lozupone, C., Jones, R., et al. (2007). Metagenomic and small-subunit rRNA analyses reveal the genetic diversity of bacteria, archaea, fungi, and viruses in soil. Appl. Env. Microbiol. 73, 7059–7066. doi: 10.1128/AEM.00358-07
Foster, Z. S. L., Sharpton, T. J., and Grünwald, N. J. (2017). Metacoder: An R package for visualization and manipulation of community taxonomic diversity data. PLOS Comput. Biol. 13:e1005404. doi: 10.1371/journal.pcbi.1005404
Gamalero, E., Lingua, G., Berta, G., and Glick, B. R. (2009). Beneficial role of plant growth promoting bacteria and arbuscular mycorrhizal fungi on plant responses to heavy metal stress. Can. J. Microbiol. 55, 501–514. doi: 10.1139/W09-010
Girvan, M. S., Bullimore, J., Pretty, J. N., Osborn, A. M., and Ball, A. S. (2003). Soil type is the primary determinant of the composition of the total and active bacterial communities in arable soils. Appl. Environ. Microbiol. 69, 1800–1809. doi: 10.1128/AEM.69.3.1800-1809.2003
Gloor, G. B., Hummelen, R., Macklaim, J. M., Dickson, R. J., Fernandes, A. D., MacPhee, R., et al. (2010). Microbiome profiling by Illumina sequencing of combinatorial sequence-tagged PCR Products. PLoS ONE 5:e15406. doi: 10.1371/journal.pone.0015406
Good, A. G., and Beatty, P. H. (2011). Fertilizing nature: a tragedy of excess in the commons. PLoS Biol. 9:e1001124. doi: 10.1371/journal.pbio.1001124
Gordon, T. R., and Martyn, R. D. (1997). The evolutionary biology of Fusarium oxysporum. Annu. Rev. Phytopathol. 35, 111–128. doi: 10.1146/annurev.phyto.35.1.111
Grabau, Z. J., and Chen, S. (2016). Determining the role of plant–parasitic nematodes in the corn–soybean crop rotation yield effect using nematicide application: I. Corn. Agronomy J. 108, 782–793. doi: 10.2134/agronj2015.0431
Gu, Z. (2016). Complex heatmaps reveal patterns and correlations in multidimensional genomic data. Bioinformatics. Available online at: http://bioconductor.org/packages/release/bioc/html/ComplexHeatmap.html
Hänsch, R., and Mendel, R. R. (2009). Physiological functions of mineral micronutrients (Cu, Zn, Mn, Fe, Ni, Mo, B, Cl). Curr. Opin. Plant Biol. 12, 259–266. doi: 10.1016/j.pbi.2009.05.006
Haraldsen, T. K., Asdal, Å., Grasdalen, C., Nesheim, L., and Ugland, T. N. (2000). Nutrient balances and yields during conversion from conventional to organic cropping systems on silt loam and clay soils in Norway. Biol. Agric. Hortic. 17, 229–246. doi: 10.1080/01448765.2000.9754844
Hardoim, P. R., van Overbeek, L. S., Berg, G., Pirttilä, A. M., Compant, S., Campisano, A., et al. (2015). The hidden world within plants: ecological and evolutionary considerations for defining functioning of microbial endophytes. Microbiol. Mol. Biol. Rev. 79, 293–320. doi: 10.1128/MMBR.00050-14
Harrow, S. A., Farrokhi-Nejad, R., Pitman, A. R., Scott, I. A. W., Bentley, A., Hide, C., et al. (2010). Characterisation of New Zealand Fusarium populations using a polyphasic approach differentiates the F. avenaceum/F. acuminatum/F. tricinctum species complex in cereal and grassland systems. Fungal Biol. 114, 293–311. doi: 10.1016/j.funbio.2010.01.005
Hassler, S. C., and Baysal-Gurel, F. (2019). Unmanned Aircraft System (UAS) technology and applications in agriculture. Agronomy 9:618. doi: 10.3390/agronomy9100618
Hbirkou, C., Welp, G., Rehbein, K., Hillnhütter, C., Daub, M., Oliver, M. A., et al. (2011). The effect of soil heterogeneity on the spatial distribution of Heterodera schachtii within sugar beet fields. Appl. Soil Ecol. 51, 25–34. doi: 10.1016/j.apsoil.2011.08.008
Hnatowich, G. L., Steckler, S. J., Leggett, M. E., and Priest, K. L. (2012). Methods and Compositions for Increasing the Amounts of Phosphorus Available for Plant Uptake From Soils. U.S. Patent No. US 8,278,247 B2. Washington, DC: U.S. Patent and Trademark Office.
Ikram, M., Ali, N., Jan, G., Jan, F. G., and Khan, N. (2020). Endophytic fungal diversity and their interaction with plants for agriculture sustainability under stressful condition. Recent Pat. Food Nutr. Agric. 11, 115–123. doi: 10.2174/2212798410666190612130139
Islam, R., Glenney, D. C., and Lazarovits, G. (2015). No-till strip row farming using yearly maize-soybean rotation increases yield of maize by 75%. Agron. Sustain. Dev. 35, 837–846. doi: 10.1007/s13593-015-0289-y
Jacoby, R., Peukert, M., Succurro, A., Koprivova, A., and Kopriva, S. (2017). The role of soil microorganisms in plant mineral nutrition—current knowledge and future directions. Front. Plant Sci. 8:1617. doi: 10.3389/fpls.2017.01617
Johnson, H. E., Gustine, D. D., Golden, T. S., Adams, L. G., Parrett, L. S., Lenart, E. A., et al. (2018). NDVI exhibits mixed success in predicting spatiotemporal variation in caribou summer forage quality and quantity. Ecosphere 9:e02461. doi: 10.1002/ecs2.2461
Kandasamy, S., Liu, E. Y. R., Patterson, G., Saldias, S., Ali, S., and Lazarovits, G. (2019). Introducing key microbes from high productive soil transforms native soil microbial community of low productive soil. Microbiologyopen 8:e895. doi: 10.1002/mbo3.895
Kapri, A., and Tewari, L. (2010). Phosphate solubilization potential and phosphatase activity of rhizospheric Trichoderma spp. Brazilian J. Microbiol. 41, 787–795. doi: 10.1590/S1517-83822010005000001
Kassambara, A., and Mundt, F. (2017). factoextra: extract and visualize the results of multivariate data analyses. Available online at: https://cran.r-project.org/package=factoextra
Kile, G. A., and Walker, J. (1987). Chalara australis sp nov (Hyphomycetes), a vascular pathogen of Nothofagus cunninghamii (Fagaceae) in Australia and its relationship to other Chalara species. Aust. J. Bot. 35, 1–32. doi: 10.1071/BT9870001
Komsta, L. (2019). mblm: Median-based linear models. R package version 0.12.1. Available online at: https://CRAN.R-project.org/package=mblm
Koukol, O. (2011). New species of Chalara occupying coniferous needles. Fungal Divers. 49, 75–91. doi: 10.1007/s13225-011-0092-2
Kowalski, T., and Holdenrieder, O. (2009). Pathogenicity of Chalara fraxinea. For. Pathol. 39, 1–7. doi: 10.1111/j.1439-0329.2008.00565.x
Kucharik, C. J. (2006). A multidecadal trend of earlier corn planting in the Central USA. Agron. J. 98, 1544–1550. doi: 10.2134/agronj2006.0156
Kumar, S., Stecher, G., Li, M., Knyaz, C., and Tamura, K. (2018). MEGA X: molecular Evolutionary genetics analysis across computing platforms. Mol. Biol. Evol. 35, 1547–1549. doi: 10.1093/molbev/msy096
Lehman, R. M., Taheri, W. I., Osborne, S. L., Buyer, J. S., and Douds, D. D. Jr. (2012). Fall cover cropping can increase arbuscular mycorrhizae in soils supporting intensive agricultural production. Appl. Soil Ecol. 61, 300–304. doi: 10.1016/j.apsoil.2011.11.008
Li, Q., Chen, J., Wu, L., Luo, X., Li, N., Arafat, Y., et al. (2018). Belowground interactions impact the soil bacterial community, soil fertility, and crop yield in maize/peanut intercropping systems. Int. J. Molec. Sci. 19:622. doi: 10.3390/ijms19020622
Liebig, M. A., and Doran, J. W. (1999). Impact of organic production practices on soil quality indicators. J. Environ. Qual. 28, 1601–1609. doi: 10.2134/jeq1999.00472425002800050026x
Madden, T. (2002). Chapter 16 The BLAST Sequence Analysis Tool. Natl. Cent. Biotechnol. Inf. USA. Available online at: https://unmc.edu/bsbc/docs/NCBI_blast.pdf (accessed January 15, 2020).
Magdoff, F. (1991). Managing nitrogen for sustainable corn systems: problems and possibilities. Am. J. Altern. Agric. 6, 3–8. doi: 10.1017/S0889189300003738
Mahajan, U., and Bundel, B. R. (2017). “Drones for Normalized Difference Vegetation Index (NDVI), to estimate crop health for precision agriculture: a cheaper alternative for spatial satellite sensors,” in International Conference on Innovative Research in Agriculture, Food Science, Forestry, Horticulture, Aquaculture, Animal Sciences, Biodiversity, Ecological Sciences and Climate Change (AFHABEC-2016) (New Delhi: Jawaharlal Nehru University).
Maron, P. A., Sarr, A., Kaisermann, A., Lévêque, J., Mathieu, O., Guigue, J., et al. (2018). High microbial diversity promotes soil ecosystem functioning. Appl. Environ. Microbiol. 84, e02738–e02717. doi: 10.1128/AEM.02738-17
Masella, A. P., Bartram, A. K., Truszkowski, J. M., Brown, D. G., and Neufeld, J. D. (2012). PANDAseq: paired-end assembler for illumina sequences. BMC Bioinformatics 13:31. doi: 10.1186/1471-2105-13-31
McMurdie, P. J., and Holmes, S. (2013). phyloseq: an R package for reproducible interactive analysis and graphics of microbiome census data. PLoS ONE 8:e61217. doi: 10.1371/journal.pone.0061217
McSorley, R., and Gallaher, R. N. (1993). Population dynamics of plant-parasitic nematodes on cover crops of corn and sorghum. J. Nematol. 25, 446–453.
Moriondo, M., Maselli, F., and Bindi, M. (2007). A simple model of regional wheat yield based on NDVI data. Eur. J. Agron. 26, 266–274. doi: 10.1016/j.eja.2006.10.007
Nadeem, S. M., Ahmad, M., Zahir, Z. A., Javaid, A., and Ashraf, M. (2014). The role of mycorrhizae and plant growth promoting rhizobacteria (PGPR) in improving crop productivity under stressful environments. Biotechnol. Adv. 32, 429–448. doi: 10.1016/j.biotechadv.2013.12.005
Nakazawa, M. (2018). fmsb: Functions for Medical Statistics Book with some Demographic Data. R package version 0.6.3. Available online at: https://cran.r-project.org/package=fmsb
Nguyen, N. H., Song, Z., Bates, S. T., Branco, S., Tedersoo, L., Menke, J., Schilling, J. S., and Kennedy, P. G. (2016). FUNGuild: an open annotation tool for parsing fungal community datasets by ecological guild. Fungal Ecol. 20, 241–248. doi: 10.1016/j.funeco.2015.06.006
Njeru, E. M., Avio, L., Sbrana, C., Turrini, A., Bocci, G., Bàrberi, P., et al. (2014). First evidence for a major cover crop effect on arbuscular mycorrhizal fungi and organic maize growth. Agron. Sustain. Dev. 6, 841–848. doi: 10.1007/s13593-013-0197-y
Oksanen, J., Blanchet, F. G., Kindt, R., Legendre, P., Minchin, P., O'Hara, R. B., et al. (2019). vegan: Community Ecology Package. R package version 2.5-5. Available online at: https://cran.r-project.org/package=vegan
Olivain, C., and Alabouvette, C. (1997). Colonization of tomato root by a non-pathogenic strain of Fusarium oxysporum. New Phytol. 137, 481–494. doi: 10.1046/j.1469-8137.1997.00855.x
OMAFRA (2017). 1. Corn in Agronomy Guide for Field Crops – Publication 811. eds. C. Brown. (Toronto, Canada: Ministry of Agriculture, Food and Rural Affairs). Available online at: http://www.omafra.gov.on.ca/english/crops/pub811/pub811.pdf
OMAFRA: Field Crops (2019). Ontario Minist. Agric. Food Rural Aff. Available online at: http://www.omafra.gov.on.ca/english/stats/crops/index.html (accessed December 12, 2019).
Öpik, M., Vanatoa, A., Vanatoa, E., Moora, M., Davison, J., Kalwij, J. M., et al. (2010). The online database MaarjAM reveals global and ecosystemic distribution patterns in arbuscular mycorrhizal fungi (Glomeromycota). New Phytol. 188, 223–241. doi: 10.1111/j.1469-8137.2010.03334.x
Palmer, L. T., and Kommedahl, T. (1969). Root-infecting Fusarium species in relation to rootworm infestations in corn. Phytopathology 59, 1613–1617.
Pascual-García, A., and Bell, T. (2020). Community-level signatures of ecological succession in natural bacterial communities. Nature Comm. 11, 1–11. doi: 10.1038/s41467-020-16011-3
Patel, J. S., Singh, A., Singh, H. B., and Sarma, B. K. (2015). Plant genotype, microbial recruitment and nutritional security. Front. Plant Sci. 6:608. doi: 10.3389/fpls.2015.00608
Patil, N. S., and Jadhav, J. P. (2015). Significance of Penicillium ochrochloron chitinase as a biocontrol agent against pest Helicoverpa armigera. Chemosphere 128, 231–235. doi: 10.1016/j.chemosphere.2015.01.038
Patzold, S., Mertens, F. M., Bornemann, L., Koleczek, B., Franke, J., Feilhauer, H., et al. (2008). Soil heterogeneity at the field scale: a challenge for precision crop protection. Precis. Agric. 9, 367–390. doi: 10.1007/s11119-008-9077-x
Peralta, A. L., Sun, Y., McDaniel, M. D., and Lennon, J. T. (2018). Crop rotational diversity increases disease suppressive capacity of soil microbiomes. Ecosphere 9:e02235. doi: 10.1002/ecs2.2235
Petrini, O. (1986). “Taxonomy of endophytic fungi of aerial plant tissues”, in Microbiology of the Phyllosphere, eds. N. Fokkema and J. van den Heuvel (Cambridge: Cambridge University Press), 175–187.
Pii, Y., Borruso, L., Brusetti, L., Crecchio, C., Cesco, S., and Mimmo, T. (2016). The interaction between iron nutrition, plant species and soil type shapes the rhizosphere microbiome. Plant Physiol. Biochem. 99, 39–48. doi: 10.1016/j.plaphy.2015.12.002
Raj, T. R. N., and Kendrick, B. (2006). A Monograph of Chalara and Allied Genera. Waterloo, ON: Wilfrid Laurier Univ. Press.
Rančić, A., Soković, M., Karioti, A., Vukojević, J., and Skaltsa, H. (2006). Isolation and structural elucidation of two secondary metabolites from the filamentous fungus Penicillium ochrochloron with antimicrobial activity. Environ. Toxicol. Pharmacol. 22, 80–84. doi: 10.1016/j.etap.2005.12.003
Rashid, M. I., Mujawar, L. H., Shahzad, T., Almeelbi, T., Ismail, I. M. I., and Oves, M. (2016). Bacteria and fungi can contribute to nutrients bioavailability and aggregate formation in degraded soils. Microbiol. Res. 183, 26–41doi: 10.1016/j.micres.2015.11.007
Rep, M., and Kistler, H. C. (2010). The genomic organization of plant pathogenicity in Fusarium species. Curr. Opin. Plant Biol. 13, 420–426. doi: 10.1016/j.pbi.2010.04.004
Rillig, M. C., and Mummey, D. L. (2006). Mycorrhizas and soil structure. New Phytol. 171, 41–53. doi: 10.1111/j.1469-8137.2006.01750.x
Rocha, I., Ma, Y., Carvalho, M. F., Magalhães, C., Janoušková, M., Vosátka, M., et al. (2019). Seed coating with inocula of arbuscular mycorrhizal fungi and plant growth promoting rhizobacteria for nutritional enhancement of maize under different fertilisation regimes. Arch. Agron. Soil Sci. 65, 31–43. doi: 10.1080/03650340.2018.1479061
Rodriguez, R. J., White, J. F. Jr, Arnold, A. E., and Redman, R. S. (2009). Fungal endophytes: diversity and functional roles. New Phytol. 182, 314–330. doi: 10.1111/j.1469-8137.2009.02773.x
Rousk, J., Bååth, E., Brookes, P. C., Lauber, C. L., Lozupone, C., Caporaso, J. G., et al. (2010). Soil bacterial and fungal communities across a pH gradient in an arable soil. ISME J. 4, 1340–1351. doi: 10.1038/ismej.2010.58
Saccardo, P. A. (1886). Sylloge fungorum: ominum hucusque cognitorum. Ann Arbor, Michigan: Edwards Brothers, Inc. Available online at: http://www.librifungorum.org/Image.asp?Nav=yes&FirstPage=4839&LastPage=5650&NextPage=4839 (accessed December 11, 2019).
Sanchez, A. D., Ousset, M. J., and Sosa, M. C. (2019). Biological control of Phytophthora collar rot of pear using regional Trichoderma strains with multiple mechanisms. Biol. Control 135, 124–134. doi: 10.1016/j.biocontrol.2019.05.012
Sánchez-de Prager, M., and Cisneros-Rojas, C. A. (2017). Organic acids production by rhizosphere microorganisms isolated from a Typic Melanudands and its effects on the inorganic phosphates solubilization. Acta Agronómica 66, 241–247. doi: 10.15446/acag.v66n2.56148
Sato, K., Suyama, Y., Saito, M., and Sugawara, K. (2005). A new primer for discrimination of arbuscular mycorrhizal fungi with polymerase chain reaction-denature gradient gel electrophoresis. Grassl. Sci. 51, 179–181. doi: 10.1111/j.1744-697X.2005.00023.x
Scharf, P. C., Wiebold, W. J., and Lory, J. A. (2002). Corn yield response to nitrogen fertilizer timing and deficiency level. Agron. J. 94, 435–441. doi: 10.2134/agronj2002.4350
Schmidt, R., Mitchell, J., and Scow, K. (2019). Cover cropping and no-till increase diversity and symbiotroph: saprotroph ratios of soil fungal communities. Soil Biol. Biochem. 129, 99–109. doi: 10.1016/j.soilbio.2018.11.010
Sen, P. K. (1968). Estimates of regression coefficient based on Kendall's tau. J. Am. Stat. Ass. 63, 1379–1389. doi: 10.1080/01621459.1968.10480934
Shafi, U., Mumtaz, R., García-Nieto, J., Hassan, S. A., Zaidi, S. A. R., and Iqbal, N. (2019). Precision agriculture techniques and practices: from considerations to applications. Sensors 19:3796. doi: 10.3390/s19173796
Shiomi, H. F., Silva, H. S. A., Melo, I. S. D., Nunes, F. V., and Bettiol, W. (2006). Bio-prospecting endophytic bacteria for biological control of coffee leaf rust. Sci. Agri. 63, 32–39. doi: 10.1590/S0103-90162006000100006
Siddikee, M. A., Zereen, M. I., Li, C.-F., and Dai, C.-C. (2016). Endophytic fungus Phomopsis liquidambari and different doses of N-fertilizer alter microbial community structure and function in rhizosphere of rice. Sci. Rep. 6:32270. doi: 10.1038/srep32270
Siegel, A. F. (1982). Robust regression using repeated medians. Biometrika, 69, 242–244. doi: 10.1093/biomet/69.1.242
Simon, A. C., Lopez-Nicora, H. D., Niblack, T. L., Dayton, E. A., Tomashefski, D., and Paul, P. A. (2018). Cropping practices and soil properties associated with plant-parasitic nematodes in corn fields in Ohio. Plant Disease 102, 2519–2530. doi: 10.1094/PDIS-03-18-0471-RE
Singh, J. S., Singh, D. P., and Pandey, V. C. (2011). Efficient soil microorganisms: a new dimension for sustainable agriculture and environmental development. Agric. Ecosyst. Environ. 140, 339–353. doi: 10.1016/j.agee.2011.01.017
Singh, S. R., Vimal, J. S., Singh, N. K., and Arora, S. (2017). Soil-plant-microbe interactions in stressed agriculture management: a review. Pedosphere 27, 177–192. doi: 10.1016/S1002-0160(17)60309-6
Smith, S. E., Smith, F. A., and Jakobsen, I. (2003). Mycorrhizal fungi can dominate phosphate supply to plants irrespective of growth responses. Plant Physiol. 133, 16–20. doi: 10.1104/pp.103.024380
Song, Y. N., Zhang, F. S., Marschner, P., Fan, F. L., Gao, H. M., Bao, X. G., et al. (2007). Effect of intercropping on crop yield and chemical and microbiological properties in rhizosphere of wheat (Triticum aestivum L.), maize (Zea mays L.), and faba bean (Vicia faba L.). Biol. Fertility Soils 43, 565–574. doi: 10.1007/s00374-006-0139-9
Ssekagiri, A. (2020). microbiomeSeq: Microbial community analysis in an environmental context. R package, version 0.1. Available online at: http://www.github.com/umerijaz/microbiomeSeq
Stockmann, U., Padarian, J., McBratney, A., Minasny, B., de Brogniez, D., Montanarella, L., et al. (2015). Global soil organic carbon assessment. Glob. Food Sec. 6, 9–16. doi: 10.1016/j.gfs.2015.07.001
Sul, W. J., Asuming-Brempong, S., Wang, Q., Tourlousse, D. M., Penton, C. R., Deng, Y., et al. (2013). Tropical agricultural land management influences on soil microbial communities through its effect on soil organic carbon. Soil Biol. Biochem. 65, 33–38. doi: 10.1016/j.soilbio.2013.05.007
Suzuki, C., Kunito, T., Aono, T., Liu, C.-T., and Oyaizu, H. (2005). Microbial indices of soil fertility. J. Appl. Microbiol. 98, 1062–1074. doi: 10.1111/j.1365-2672.2004.02529.x
Tardy, V., Mathieu, O., Lévêque, J., Terrat, S., Chabbi, A., Lemanceau, P., et al. (2014). Stability of soil microbial structure and activity depends on microbial diversity. Environ. Microbiol. Rep. 6, 173–183. doi: 10.1111/1758-2229.12126
Taylor, D. L., Walters, W. A., Lennon, N. J., Bochicchio, J., Krohn, A., Caporaso, J. G., et al. (2016). Accurate estimation of fungal diversity and abundance through improved lineage-specific primers optimized for Illumina amplicon sequencing. Appl. Environ. Microbiol. 82, 7217–7226. doi: 10.1128/AEM.02576-16
Tedersoo, L., Bahram, M., Põlme, S., Kõljalg, U., Yorou, N. S., Wijesundera, R., et al. (2014). Global diversity and geography of soil fungi. Science 346, 1256688. doi: 10.1126/science.1256688
Theil, H. (1950). A rank invariant method for linear and polynomial regression analysis. Nederl. Akad. Wetensch. Proc. Ser. A 53, 386–392 (Part I), 521–525 (Part II), 1397–1412 (Part III).
Tkacz, A., and Poole, P. (2015). Role of root microbiota in plant productivity. J. Exp. Bot. 66, 2167–2175. doi: 10.1093/jxb/erv157
Troyer, A. F. (2009). “Development of hybrid corn and the seed corn industry,” in Handbook of maize (New York, NY: Springer), 87–114. doi: 10.1007/978-0-387-77863-1_5
Van Roekel, R. J., and Coulter, J. A. (2011). Agronomic responses of corn to planting date and plant density. Agron. J. 103, 1414–1422. doi: 10.2134/agronj2011.0071
Veresoglou, S. D., Barto, E. K., Menexes, G., and Rillig, M. C. (2013). Fertilization affects severity of disease caused by fungal plant pathogens. Plant Pathol. 62, 961–969. doi: 10.1111/ppa.12014
Wall, R. E., and Mortimore, C. G. (1965). The growth pattern of corn in relation to resistance to root and stalk rot. Can. J. Bot. 43, 1277–1283. doi: 10.1139/b65-134
Wang, Q., Garrity, G. M., Tiedje, J. M., and Cole, J. R. (2007). Naive Bayesian classifier for rapid assignment of rRNA sequences into the new bacterial taxonomy. Appl. Environ. Microbiol. 73, 5261–5267. doi: 10.1128/AEM.00062-07
Wei, T., and Simko, V. (2017). R package “corrplot”: Visualization of a correlation matrix (Version 0.84). Available online at: https://github.com/taiyun/corrplot
Wilpiszeski, R. L., Aufrecht, J. A., Retterer, S. T., Sullivan, M. B., Graham, D. E., Pierce, E. M., et al. (2019). Soil aggregate microbial communities: towards understanding microbiome interactions at biologically relevant scales. Appl. Environ. Microbiol. 85, e00324–e00319. doi: 10.1128/AEM.00324-19
Xu, Y., Wang, G., Jin, J., Liu, J., Zhang, Q., and Liu, X. (2009). Bacterial communities in soybean rhizosphere in response to soil type, soybean genotype, and their growth stage. Soil Biol. Biochem. 41, 919–925. doi: 10.1016/j.soilbio.2008.10.027
Yang, Y., Dou, Y., Huang, Y., and An, S. (2017). Links between soil fungal diversity and plant and soil properties on the Loess Plateau. Front. Microbiol. 8:2198. doi: 10.3389/fmicb.2017.02198
Yeganehpoor, F., Salmasi, S. Z., Abedi, G., Samadiyan, F., and Beyginiya, V. (2015). Effects of cover crops and weed management on corn yield. J. Saudi Soc. Agric. Sci. 14, 178–181. doi: 10.1016/j.jssas.2014.02.001
Zhou, D., Wang, X., Chen, G., Sun, S., Yang, Y., Zhu, Z., et al. (2018). The major Fusarium species causing maize ear and kernel rot and their toxigenicity in Chongqing, China. Toxins 10:90. doi: 10.3390/toxins1002009
Zhou, J., Jiang, X., Zhou, B., Zhao, B., Ma, M., Guan, D., et al. (2016). Thirty four years of nitrogen fertilization decreases fungal diversity and alters fungal community composition in black soil in northeast China. Soil Biol. Biochem. 95, 135–143. doi: 10.1186/s13568-018-0587-2
Keywords: root mycobiome, soil quality, soil productivity, soil chemistry, sustainable agriculture, high throughput sequencing, rhizosphere
Citation: Kandasamy S, Weerasuriya N, Subramanian G, Thorn RG, Patterson G, Ali S and Lazarovits G (2021) Disentangling the Association of Corn Root Mycobiome With Plant Productivity and the Importance of Soil Physicochemical Balance in Shaping Their Relationship. Front. Sustain. Food Syst. 5:617332. doi: 10.3389/fsufs.2021.617332
Received: 14 October 2020; Accepted: 13 January 2021;
Published: 16 February 2021.
Edited by:
Maryke T. Labuschagne, University of the Free State, South AfricaReviewed by:
Nancy Collins Johnson, Northern Arizona University, United StatesYonglong Wang, Baotou Teachers' College, China
Copyright © 2021 Kandasamy, Weerasuriya, Subramanian, Thorn, Patterson, Ali and Lazarovits. This is an open-access article distributed under the terms of the Creative Commons Attribution License (CC BY). The use, distribution or reproduction in other forums is permitted, provided the original author(s) and the copyright owner(s) are credited and that the original publication in this journal is cited, in accordance with accepted academic practice. No use, distribution or reproduction is permitted which does not comply with these terms.
*Correspondence: Saveetha Kandasamy, c2F2ZWV0aGFAYWxjYW5hZGEuY29t