- 1Texas A&M AgriLife Research (Texas A&M University System), Vernon, TX, United States
- 2United States Department of Agriculture - Agricultural Research Service, Cropping Systems Research Laboratory, Lubbock, TX, United States
- 3Department of Agricultural and Applied Economics, Texas Tech University, Lubbock, TX, United States
- 4United States Department of Agriculture - Agricultural Research Service, Conservation and Production Research Laboratory, Bushland, TX, United States
- 5Texas A&M AgriLife Research (Texas A&M University System), Plainview, TX, United States
In the Texas High Plains (THP), diminishing irrigation well-capacities, and increasing costs of energy and equipment associated with groundwater extraction and application are contributing factors to a transition from irrigated to dryland agriculture. The primary goal of this modeling exercise was to investigate whether and to what extent hypothetical changes in factors putatively associated with soil health would affect dryland cotton (Gossypium hirsutum L.) yields. The factors selected were drainage, surface runoff, soil water holding capacity, soil organic carbon (SOC) and albedo. As a first analysis to evaluate these factors, we used the CROPGRO-Cotton module within the Decision Support System for Agrotechnology Transfer (DSSAT) cropping system model. Specifically, we evaluated the effects of reduced surface runoff, increased soil water holding capacity, and SOC, doubling of the soil albedo through stubble mulching, and of soil drainage by enhancing infiltration with no-tillage/cover crops on yield by adjusting related soil properties. In our analysis, we used mean yields simulated with soil properties of a Pullman clay loam soil at Halfway, TX on the THP as baseline, which were compared to values obtained with the adjusted factors using weather data from 2005 to 2019. Simulated mean yield increased by 27% when the soil water holding capacity was increased by 25 mm, 7% when the runoff curve number was decreased from 73 to 60, 16% when soil albedo was increased from 0.2 to 0.4, and by 58% when the soil drainage factor (fraction day−1) was doubled from 0.2. No significant statistical change in simulated mean yield was calculated when SOC was increased by 1%. Further, effects of a 50 mm pre-plant irrigation were also assessed, simulating limited irrigation in the transition to dryland agriculture that resulted in a statistically insignificant 12% increase in seed-cotton yield. Simultaneous implementation of the four statistically significant individual scenarios (increased water holding capacity, infiltration, albedo, and drainage) resulted in the highest increase (93%) in mean seed-cotton yield. An economic and risk analysis of simulated yields under different scenarios indicated that these factors could reduce revenue risk for dryland cotton producers, with most of the effect from soil drainage improvements.
Introduction
The Texas High Plains (THP) is a warm semi-arid region (Köppen-Geiger class BSh) in the southwestern US (Peel et al., 2007). The THP is an agronomically important region capable of producing a fairly wide range of crops due, in part, to a relatively long growing season, mild winters, and high average terrestrial solar radiation levels (Lascano, 2000). While the environment is conducive to high crop yields, it also leads to high evapotranspiration (ET) rates. Water availability limits crop yields and constrains the types of crops that can be profitably grown in the THP. For example, crop production systems in the THP can be broadly divided into those dependent on irrigation and those typically grown without irrigation. Commercial production of corn, alfalfa, grapes, fruit and nut trees, and even pumpkins is almost exclusively dependent on irrigation. Other crops such as sorghum, sunflower, winter wheat, rapeseed (canola), and sesame are predominately, though not exclusively, grown without irrigation. Cotton (Gossypium hirsutum L.) is a unique crop in the THP and similar regions worldwide in that it has been, and continues to be, grown under a wide range of irrigation regimes ranging from “full” irrigation to exclusively dryland systems. Upland cotton is economically one of the most important crops in the THP. Texas cotton production represents about one third to nearly one half of cotton production in the US, the world's largest cotton producer (Raper et al., 2020).
Early in the twentieth century, cotton and sorghum in the THP were planted almost exclusively as dryland crops. Because rainfall is spatially and temporally stochastic, year-to-year yields, and profitability were variable (Sullivan, 1932). Though cotton was successfully grown under dryland systems, its yield was quite responsive to water availability, especially when compared to sorghum. This is due, in part to the indeterminate growth and development of cotton. Since cotton development and yield are both responsive to water availability, cotton was increasingly irrigated through the twentieth century as irrigation technology became more widely available. Irrigation of cotton led to both reduced year-to-year yield variability and increased profitability (Musick et al., 1990; Colaizzi et al., 2008). As irrigation technologies became increasingly available, higher value crops began to displace many of those well-adapted to dryland culture. In the case of cotton, the area under irrigated production displaced dryland production. However, as with sorghum and wheat, dryland cotton production was never entirely displaced (Musick et al., 1990).
In the THP, irrigation-pumping from the Ogallala Aquifer far exceeds a negligible recharge (Scanlon et al., 2012). As water has been excessively mined from the Ogallala Aquifer, the depth to the water level has increased (Chaudhuri and Ale, 2014), saturated aquifer thickness has decreased, and well-capacity has decreased. Irrigation water has therefore become more limited and more expensive to lift and to apply. It is generally accepted that irrigated land area in the THP will continue to decrease and the region is undergoing a transition from largely irrigated to dryland agricultural cropping systems. Similar trends in increasing water withdrawals for irrigation and associated reduction in irrigated areas are reported in other parts of the World (e.g., Valipour, 2016, 2017).
Crop water availability is the single most important managed environmental variable through irrigation. Irrigation is simply a replacement for timely rainfall. Since precipitation is an uncontrollable factor, as the THP transitions to dryland production, other management practices become more important to compensate for the vagaries of weather, especially the spatially, temporally, and stochastically distributed precipitation events. Hence, in an exclusively rain-fed system, managing crop performance is largely an exercise in managing the soil surface hydrologic and bulk physical properties because crop yield is dependent on those properties. For example, in a field study in east-central Mississippi, about 58–65% and 21–40% variability in dryland cotton yield was explained by soil properties and hydrologic attributes, respectively (Iqbal et al., 2005). Cultural practices such as no-tillage, cover crops, stubble mulching and crop rotation affect soil properties and thereby crop yield. In a long-term experiment in Queensland, Australia, Hulugalle et al. (2007) studied the effects of growing cereal and leguminous crops in rotation with dryland cotton on physical and chemical properties of a gray Vertisol and found that the cotton-wheat rotation reduced soil compaction, and improved soil structure and cotton lint yield when compared to cotton-sorghum rotation or continuous cotton. In another experiment on clay loam Stagnosols in Croatia, Bogunovic et al. (2017) found that the tillage practices influenced soil physical properties such as bulk density and penetration resistance and thereby affected crop yields. Using the APSIM-OZCOT cotton simulation model, Yeates and Poulton (2019) assessed the impacts of soil surface management on dryland cotton yields at four different sites in Australia and found that a legume mulch along with 100 kg ha−1 of N fertilizer maximized dryland cotton yield. Precipitation management is also very important in dryland agriculture, and several engineering and crop cultural approaches have been developed to enhance precipitation use efficiency. Most of these approaches focus on reducing runoff and increasing infiltration, such as landscape terracing, furrow diking, and altered planting geometries (Gerard et al., 1984; Colburn and Alexander, 1986; Hatfield et al., 2001; Lascano and Nelson, 2014).
Recently, management of “soil health,” a popularized extension of the soil quality concept as an integration of soil biotic and abiotic properties and processes (Harris and Bezdicek, 1994), has been suggested as an approach to maintain or even increase dryland crop yields by increasing precipitation use efficiency (Cano et al., 2018). While there is no direct measurement of soil health, several measurable physical properties putatively associated with health are used as indicators, proxy, of soil health. For example, it is frequently generalized that an increase of 1% (10 g/kg) in soil carbon will result in about 25 mm of additional soil water available to plants (Cano et al., 2018). However, based on a meta-analysis from 60 published studies and analysis of large global databases, Minasny and McBratney (2018) reported that the effect of an increase in soil organic matter (SOM) on the increase in amount of plant available water was overestimated. Further, whether it is feasible to attain 1% increase in carbon in THP soils is questionable, especially since only THP fields that have never been subjected to cultivation exhibit this level of organic carbon, which is restricted to the surface 0.2–0.3 m depth. Moreover, in the THP, wheat fields that were continuously stubble mulched since the 1940's did not achieve 1% carbon (Schwartz et al., 2015). Nevertheless, this begs the question: if soil water available to plants is increased by 25 mm, how would this affect dryland crop production yield? Also, how would other factors and soil physical properties associated with soil health affect cotton yield?
The overall goal of this study was to assess the potential long-term dryland cotton production response to hypothetical changes in selected factors, and soil physical and chemical properties associated with soil health. Further, the effect of pre-plant irrigation application on cotton yield, simulating a scenario in the transition to dryland agriculture, was also investigated. Our specific objective was to use the CROPGRO-Cotton module within the Decision Support System for Agrotechnology Transfer (DSSAT) cropping system model (CSM) (Jones et al., 2003; Hoogenboom et al., 2019) as a first analysis to evaluate the effects of reduced surface runoff, increased soil water holding capacity and soil organic carbon (SOC), doubling of the soil albedo (portion of the incident solar radiation reflected by the soil surface) through stubble mulching, and of soil drainage by enhancing infiltration with no-tillage/cover crops on seed-cotton yield by adjusting related soil properties. We recognize that in some instances, and for example, simply and only doubling a soil factor without further adjustments to other model calculations and/or parameters is an oversimplification. Nevertheless, our goal was to provide guidelines on what soil health related factors may be agronomically managed to achieve higher crop yields in the THP. Further, results from this study will be followed by similar comparison of simulated cotton lint yields but using a theoretical approach that links changes in a soil property to model functions and calculations of the water and energy balance of a dryland cotton system.
Materials and Methods
Study Site and Measured Data for Model Evaluation
The study focused on Halfway (34° 10′ N, 101° 56′ W; elevation 1,075 m) in Hale County, TX in the THP where the Texas A&M AgriLife Research Station is located. Measured data from a cotton irrigation water use efficiency (IWUE) experiment conducted at this site with a center pivot irrigation system over four growing seasons from 2010 to 2013 (Bordovsky et al., 2015) were used for model evaluation. The soil at the study site is classified in the Pullman clay loam series (fine, mixed, super active, thermic Torrertic Paleustolls). The average (1977–2018) annual and growing season (from 1 May to 31 October) rainfall was 463 and 344 mm, respectively (Himanshu et al., 2019). A total of 27 irrigation treatments were implemented in the IWUE field experiment with combinations of three levels of daily irrigation [0 mm day−1 (Low), 3.2 mm day−1 (Medium), and 6.4 mm day−1 (High)] applied during the vegetative, reproductive, and maturation growth stages. Conventional tillage was adopted at the study site and pre-plant irrigations, if needed, to elevate the top 0.6 m of the soil profile to field capacity, were applied uniformly on all plots. Additional details about irrigation, fertilizer, and chemical applications in the IWUE field experiment are given by Bordovsky et al. (2015) and used for model parameterization. Measured seed-cotton yield data from the IWUE experiment were used for model evaluation. Daily weather data for the period from 2005 to 2019 were obtained from the on-site weather station for scenario analysis.
DSSAT CSM CROPGRO-Cotton Model
The DSSAT is a platform that integrates soil, weather, crop, and experimental data management programs with crop models and different application programs (Jones et al., 2003; Hoogenboom et al., 2019). The DSSAT CSM can calculate crop growth, development, water use and yield, soil water, and C and N processes under combined effects of weather patterns, soil properties, cultivar characteristics, and management practices on a daily interval. The latest 4.7.5 version of the DSSAT model has 42 crop modules including CROPGRO-Cotton (Hoogenboom et al., 2019). The minimum weather data required to run the model include daily precipitation, maximum and minimum air temperature, and solar irradiance, while wind speed and relative humidity are optional input parameters. The model requires several soil parameters including texture, color, bulk density, slope, albedo, soil water constants, hydraulic conductivity, drainage, organic carbon content, and total soil nitrogen. The management data required by the model include dates of planting, harvest, and tillage; planting depth and density; row spacing; cultivar; and details about crop residue management, and fertilizer, chemical and irrigation applications, as applicable. More details about the DSSAT model inputs used in this study can be found in Adhikari et al. (2016).
A tipping bucket approach is used for soil water balance simulation in DSSAT with three key soil moisture variables: Saturated Water Content (SAT), Drained Upper Limit (DUL), and the Lower Limit (LL) of plant extractable water (Hoogenboom et al., 2019). The DUL and LL are equivalent to field capacity and wilting point soil water contents, respectively. In DSSAT, soil water balance is calculated on a daily basis by adding irrigation and rainfall and subtracting surface runoff, drainage, and ET. Rainfall is partitioned to infiltration and surface runoff using the Soil Conservation Service (SCS) curve number approach (Ritchie, 1998). Downward movement of water within the soil depends on a soil drainage factor (fraction day−1), which is limited by saturated hydraulic conductivity of soil layers (Hoogenboom et al., 2019). There are two options for estimating ET; the Priestley-Taylor method (Priestley and Taylor, 1972), and the FAO-56 method (Allen et al., 1998). For computation of SOM dynamics, either the original CERES-based module (Godwin and Singh, 1998) or the CENTURY-based module (Gijsman et al., 2002) can be used. The main difference between these two modules is that the surface fresh organic matter and three pools of SOM are included in the CENTURY model, and it allows more control over initialization of stable carbon pools and hence overall decomposition dynamics (Hoogenboom et al., 2019).
Model Evaluation
The DSSAT CSM CROPGRO-Cotton model was previously evaluated for Halfway, TX by Adhikari et al. (2016) using measured data from Bordovsky et al. (2015) IWUE experiment by creating a DSSAT experiment project. In an experiment project, the initial conditions (e.g., soil water, crop residue) are initialized at the beginning of each simulation year and this was appropriate for the Adhikari et al. (2016) study, which followed the field experiment in which pre-plant irrigation was applied when necessary based on the soil water status. However, as the goal of the current study was to evaluate the long-term effects of changes in drainage, surface runoff and soil properties on dryland crop production, a re-evaluation of the Adhikari et al. (2016) model by creating a DSSAT sequential project was necessary. In a sequential project, long-term simulations are conducted continuously (without initializing the initial conditions each year) and hence it represents a cotton-fallow cropping system more realistically and ensures that the water and nutrient balances during the fallow periods between cotton growing seasons are simulated accurately.
During the sequential evaluation, as was done in Adhikari et al. (2016) study, the DSSAT CSM CROPGRO-Cotton model was calibrated using the measured data over four growing seasons (2010–2013) from four high irrigation treatments with negligible/no water stress (HHH, HHM, MHH, and MHM) in the Bordovsky et al. (2015) experiment. The calibrated model was then evaluated using measured data from the remaining 23 treatments over the four growing seasons. More details about the model setup for the study area and model inputs can be found in Adhikari et al. (2016). Model calibration was carried out manually by slightly adjusting Adhikari et al. (2016) parameters until simulated and measured seed-cotton yields matched. The model performance during the calibration and evaluation was assessed both graphically (by constructing scatter plots) and statistically. For consistency, four model performance statistics used by Adhikari et al. (2016), including the coefficient of determination (r2) (Legates and McCabe, 1999), index of agreement (d) (Willmott et al., 1985), root mean square error (RMSE), and the percent error (PE), were used in this study. The r2 and d-values range between 0 (indicating no fit between simulated and measured values) and 1 (perfect fit). The RMSE values closer to 0 indicate a better agreement between the simulated and measured values. The PE varies between −100 and ∞, with smaller absolute values closer to 0 indicating a better agreement. The model calibration was continued until the performance statistics were comparable to those achieved in the Adhikari et al. (2016) study.
Evaluation of the Effects of Changes in Selected Factors and Soil Properties on Dryland Cotton Production
Long-term simulations were run with the evaluated model for the period from 2005 to 2019 and this scenario was considered as the “baseline” scenario (hereafter referred to as the S0 scenario). In the long-term simulations, a common planting date of May 9 was assumed each year based on the actual dates implemented in the field experiment. A conventional tillage (with a field cultivator and rotary hoe tillage) was implemented in each year of simulation as per the standard practice followed at the study site. Potential dryland cotton yield increases from changes in runoff, drainage, and soil properties associated with soil health were then assessed by running five hypothetical scenarios: (1) increasing DUL by 25 mm to reflect the potential increase in soil water holding capacity due to adoption of soil health improving practices such as cover crops (S1 scenario); (2) decreasing curve number from 73 to 60 to reduce surface runoff and thereby simulate potential increase in infiltration/soil water holding capacity due to adoption of soil health promoting practices such as no-tillage (S2); (3) increasing soil albedo from 0.2 to 0.4 to reflect potential increase in reflected solar radiation due to placement of crop residue on the surface (S3); (4) increasing the soil drainage factor from 0.2 to 0.4 to reflect potential increase in infiltration and downward movement of soil water from practices such as cover crops and deep tillage (S4); and (5) increasing SOC by 1% through adoption of practices such as no-tillage and cover crops (S5). Additionally, we ran a sixth scenario (S6) with a 50 mm pre-plant irrigation (25 mm each, applied on 28 April and 2 May in each year) to reflect a practice that some of the producers in the THP region adopt. These producers convert a portion of the center pivot area into dryland production due to reduced irrigation capacities, and apply pre-plant irrigations on dryland cotton in late April/early May when groundwater levels are relatively shallower (compared to summer) and crop water demand is negligible (unless cover crops are grown).
A statistical analysis was done using a student's t-test (Gosset, 1908) to determine if the seed-cotton yields under simulated scenarios were significantly different from those under the baseline scenario. A paired two-tailed t-test (considering the same population twice, with- and without changes to soil properties/pre-plant irrigation) was performed using the T.TEST command in Microsoft Excel to test statistical significance at 95% level (p = 0.05). A “combination” scenario (Sc1) was then run by simultaneously implementing all statistically significant scenarios to predict the “potential maximum dryland seed-cotton yield” with the adoption of multiple soil health promoting practices. Additionally, in view of mixed reports on the effect of increase in SOC on plant available water (Cano et al., 2018; Minasny and McBratney, 2018), an additional combination scenario (Sc2) was run by excluding S1 scenario from the Sc1 scenario. The effect of climate variability on simulated results was finally assessed by dividing the simulation years into dry, normal, and wet years based on the growing season precipitation from 1 April to 31 October (Figure 1). After sorting the years based on the growing season precipitation, the 5 years that received the lowest precipitation were classified as the dry years, and the 5 years that received the highest precipitation were classified as the wet years. The remaining 5 years were classified as the normal years.
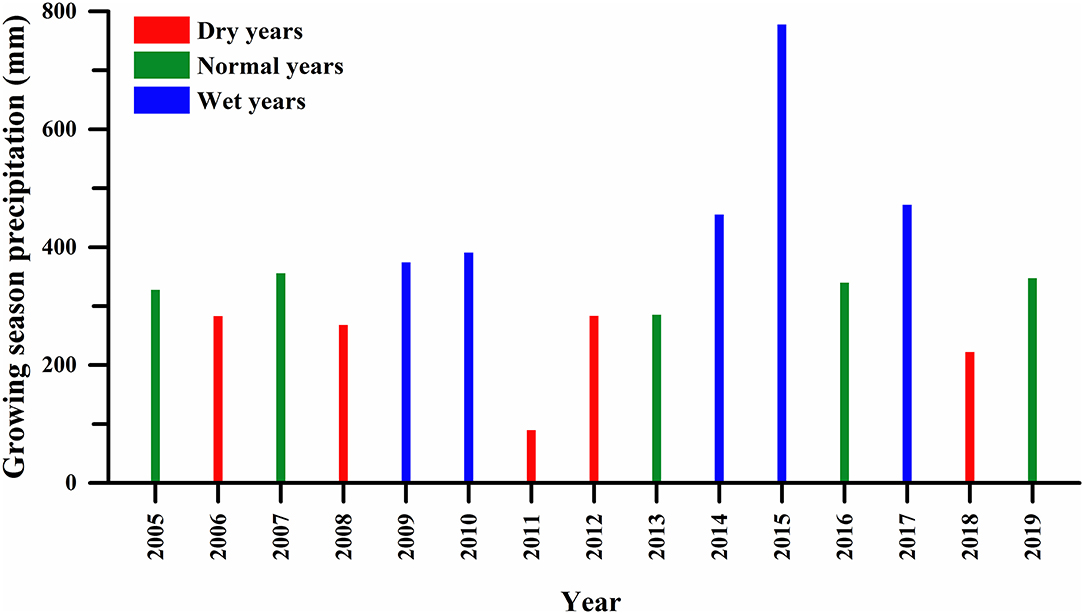
Figure 1. Classification of the simulation period (2005–2019) into dry, normal, and wet years based on the accumulated precipitation during the growing season (from 1 April to 31 October).
Economic and Risk Analysis
In dryland systems, increasing average yield is important. However, producers are risk averse (Sandmo, 1971) and would therefore be willing to give up some revenue to reduce exposure to risk. The impacts of different soil health improvements must also consider the impacts on variance (risk) of returns as well. Farm revenue was simulated using a Monte Carlo method combining the simulated yields in this analysis with a sample of actual cotton market prices drawn from the US Department of Agriculture (USDA) database on farm prices (USDA-ERS, 2019). A beta distribution was fit to the simulated yields because it allows for the skewness inherent in yields censored at zero and has been used in many applications of evaluating yields for insurance modeling purposes (Luitel et al., 2018). A log-normal distribution, which is the most commonly assumed distribution for prices, was fit to cotton prices. Prices and yields are often (at least minimally) negatively correlated, thus Monte Carlo simulations must account for that correlation when modeling farm revenue (price × yield). The procedure outlined by Anderson et al. (2009) was used to generate a simulated distribution of farm revenue assuming a price/yield correlation of r = −0.092 as was used by Luitel et al. (2018) for Texas cotton farms.
In our analysis, only farm revenue was considered (no costs of production and no costs of obtaining the soil health improvements). The analysis aimed to identify two key outcomes. First, a risk averse producer would be willing to accept some level of revenue lower than the expected (mean) revenue to avoid the risk of producing. This value is called the “certainty equivalent” (CE) and represents the amount of revenue required for the producer to avoid taking the risk. Again, following Luitel et al. (2018), this analysis used a constant relative risk aversion (CRRA) coefficient of two, which is associated with a moderately risk averse producer. This value was arbitrary but was held constant across all scenarios for comparability. The CE was interpreted in relation to the base value (S0). Higher CE values indicated that the producer would prefer that scenario to the base and vice versa. Second, an important outcome of soil health improvements must be to reduce the risk of low revenue years if the producer is to be at all interested in pursuing those outcomes. If the soil health improvement does not reduce risk of catastrophic losses relative to the status quo, improvements in average revenue may be ignored in preference for “safer” alternatives. To evaluate this risk, a value of 40% of the expected (mean) revenue was arbitrarily considered to be a catastrophic loss. Again, the value chosen was arbitrary but was held fixed across alternatives to insure comparability. Revenue distributions were found to be best fit by log-normal distributions, so this comparison was conducted by calculating the probability of observing 40% of the mean revenue in the base case, or $69 ha−1 in each of the scenarios.
Results and Discussion
Model Calibration and Validation
Several of the calibrated parameters of Adhikari et al. (2016) were adjusted and some of their initial conditions were modified during the sequential calibration of the DSSAT CSM CROPGRO-Cotton model (Table 1). The values of previous crop (cotton) residue and root weight, which were not considered by Adhikari et al. (2016), were estimated in this study, and input to the model. The N and P contents of residue were obtained from the literature (Gemtos and Tsiricoglou, 1999; Wanjura et al., 2014). In addition, the initial soil water content was changed from 0.18 to 0.24 m3 m−3 during the sequential calibration (Table 1). Some of the methods used by Adhikari et al. (2016) were also modified to get a good match between the simulated and measured seed-cotton yields (Table 1). Most importantly, the FAO-56 ET method (Allen et al., 1998), which was used by Adhikari et al. (2016), calculated consistently lower seed-cotton yields for the sequential project and hence Priestly-Taylor method was used in this study. A majority of other DSSAT cotton modeling studies in the literature have also used the Priestley-Taylor method (e.g., Guerra et al., 2007; Garcia et al., 2008; Gérardeaux et al., 2018). The SOM simulation method was also changed from the CERES (Godwin and Singh, 1998) to the CENTURY (Gijsman et al., 2002) method as the latter method simulates soil carbon and nitrogen dynamics more efficiently than the former method.
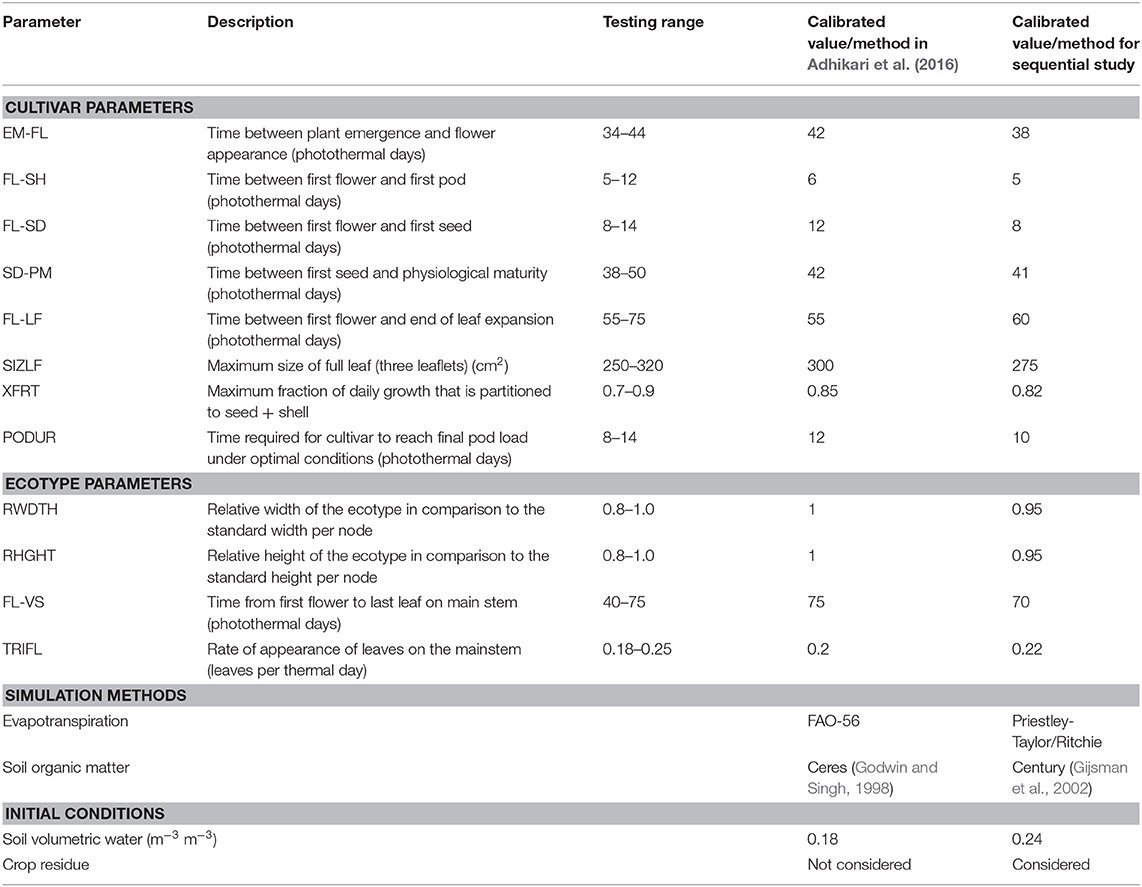
Table 1. Parameter values and simulation methods changed during the Decision Support System for Agrotechnology Transfer (DSSAT) cropping system model (CSM) CROPGRO-Cotton model sequential calibration.
The simulated seed-cotton yield matched well with the measured yield during both calibration and evaluation as shown in Figure 2, and also as indicated by model performance statistics (Table 2). The performance statistics achieved during calibration (r2 = 0.82, d = 0.9, RMSE = 18%, and PE = 10%) and evaluation (r2 = 0.84, d = 0.95, RMSE = 29%, and PE = −0.3%) were comparable to those obtained by Adhikari et al. (2016). In general, the model predictions of seed-cotton yield were better in case of high irrigation (calibration) treatments as compared to those for low/medium irrigation (validation) treatments as indicated by lower % RMSE values during the model calibration (18 vs. 29%). These trends are in agreement with previous studies that reported unsatisfactory performance of DSSAT CSM under dry conditions (Thorp et al., 2014; Modala et al., 2015; Adhikari et al., 2016; Kothari et al., 2019). The model performance during the year 2012 was not satisfactory, mainly due to the influence of extreme dry conditions in the preceding year. Overall, the evaluated DSSAT CSM CROPGRO-Cotton sequential model demonstrated potential in effectively simulating seed-cotton yield under 27 treatments in Bordovsky et al. (2015) experiment with widely variable irrigation applications over four growing seasons (2010 to 2013) with contrasting weather conditions.
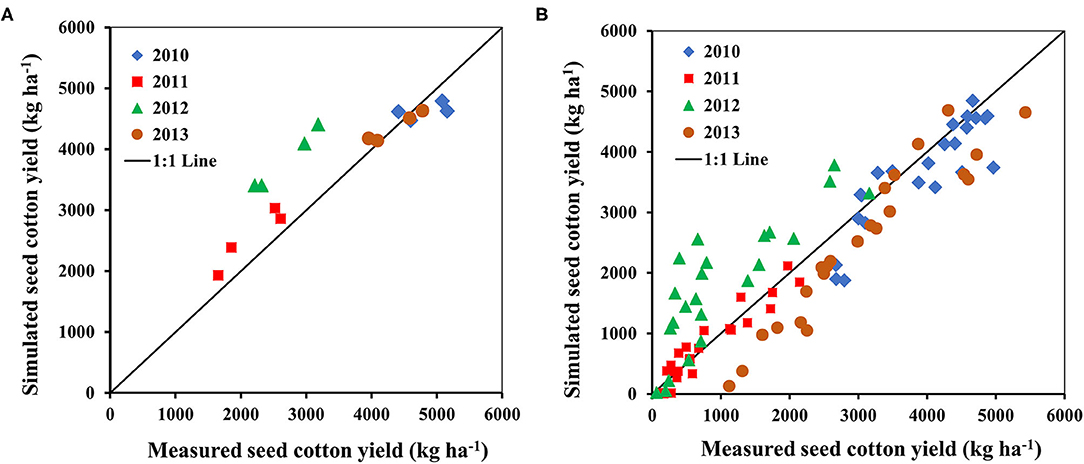
Figure 2. Comparison of simulated and measured seed-cotton yield during the Decision Support System for Agrotechnology Transfer (DSSAT) cropping system model (CSM) CROPGRO-Cotton model (A) calibration and (B) evaluation.
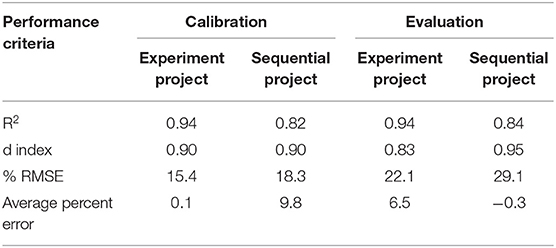
Table 2. Comparison of model performance statistics between the experiment evaluation (Adhikari et al., 2016) and the sequential evaluation (this study).
Effect of Changes in Selected Factors, Soil Properties, and Pre-plant Irrigation on Simulated Dryland Seed-Cotton Yield
Among the simulated scenarios, the soil drainage rate increase scenario (S4) showed the greatest increase in simulated seed-cotton yield followed by DUL increase (S1) and soil albedo increase (S3) scenarios as compared to the baseline scenario (Figure 3). While the mean seed-cotton yield increased by 27, 7, 16, 58, and 12% under S1, S2, S3, S4, and S6 scenarios, respectively, when compared to the baseline scenario, it decreased slightly by about 1% under S5 scenario (increased SOC) (Figure 3). There was high variability in simulated seed-cotton yield under S4 and S1 scenarios. The calculated p-values from the student's t-test for S1, S2, S3, S4, S5, and S6 scenarios were 0.022, 0.026, 0.002, 0.0008, 0.162, and 0.090, respectively. These p-values indicated that the simulated seed-cotton yield changed significantly (p < 0.05) under S1 to S4 scenarios, while it did not change significantly (p > 0.05) under the remaining two (S5 and S6) scenarios (p > 0.05). Although the differences in mean seed-cotton yield between the base scenario and the alternate scenarios were substantial, the differences in median seed-cotton yield were small, except in the case of S4 scenario. This was because simulated seed-cotton yield values were low in majority of simulation years. However, long-term simulations were run for only 15 years in this study, and in general, median values present more realistic trends when the simulation period is much longer (~40 years or when the sample size is larger). The effect of changes in selected factors, soil properties, and pre-plant irrigation on seed-cotton yield is discussed in more detail in the subsequent sections.
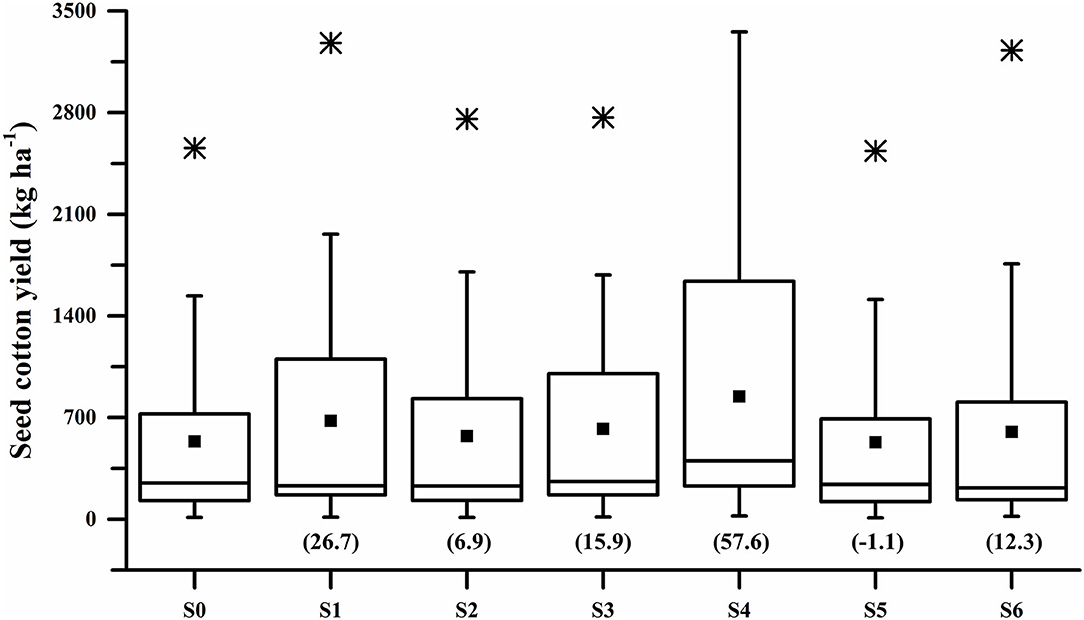
Figure 3. Effect of changes in selected factors and soil properties on simulated dryland seed-cotton yield (2005–2019). Scenario acronyms: S0, baseline; S1, drained upper limit increased by 25 mm; S2, curve number reduced from 73 to 60; S3, soil albedo increased from 0.2 to 0.4; S4, soil drainage factor increased from 0.2 to 0.4; S5, soil organic carbon increased by 1%; S6, pre-plant irrigation of 50 mm. The percent changes in mean seed-cotton yield under different scenarios relative to S0 are shown in parentheses at the bottom of respective boxes. The horizontal line and solid square inside the box indicate the median and mean, respectively. The ends of boxes indicate the 25th and 75th percentiles. Asterisks outside the boxes represent outliers or values >1.5 interquartile ranges away from the 25th or 75th percentiles.
Effect of Increasing Drainage Upper Limit (S1 Scenario) on Seed-Cotton Yield
The increase in DUL by 25 mm (S1 scenario) increased plant available water (the difference between field capacity and permanent wilting point soil water contents), which contributed to a 27% increase in mean seed-cotton yield (Figure 3). The effect of increase in DUL on mean seed-cotton yield was the highest in normal years (34%) and lowest in the dry years (4%) (Figure 4). In the dry years, the average growing season precipitation was about 31% lower than that in the normal years, and hence the benefits of increase in DUL could not be fully realized due to insufficient rainfall. These results are consistent with published studies (Wang et al., 2009; He and Wang, 2019). Using an agricultural production systems model (APSIM), Wang et al. (2009) found that the soils with higher soil plant available water holding capacity (PAWC) enabled more rainfall to be used by crops. In a follow-up study, He and Wang (2019) noted that the long-term averaged dryland wheat biomass and yield increased with an increase in PAWC until a critical value was reached. The increase in DUL also increased soil water evaporation from the surface layer and decreased deep drainage, and therefore the increase in mean seed-cotton yield with the increase in DUL was lower compared to the increase in soil drainage rate [S4 scenario; section Effect of Increasing Soil Drainage Rate (S4 Scenario) on Seed-Cotton Yield].
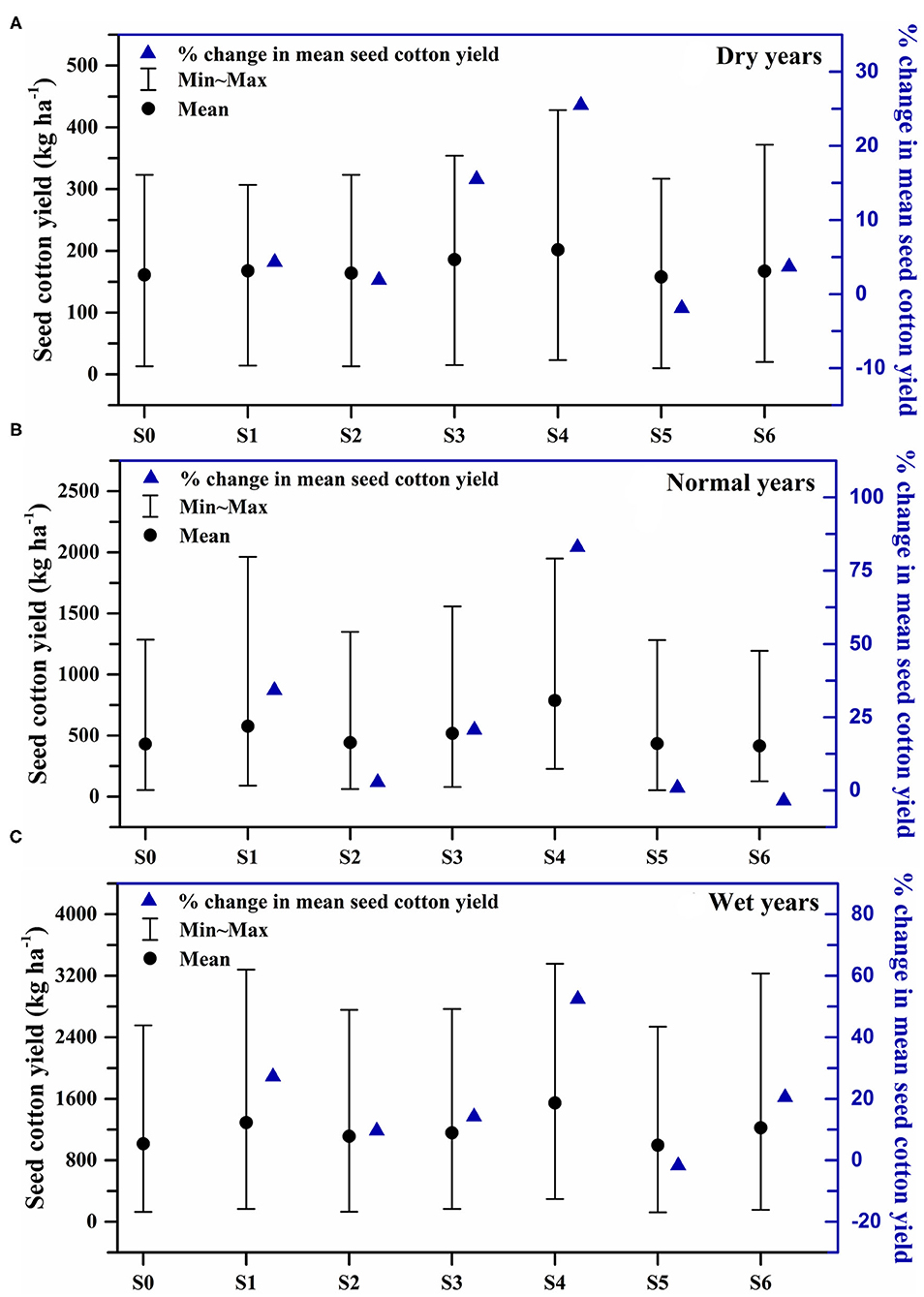
Figure 4. Simulated changes in dryland seed-cotton yield due to changes in selected factors and soil properties in: (A) dry, (B) normal, and (C) wet years. Scenario acronyms: S0, baseline; S1, drained upper limit increased by 25 mm; S2, curve number reduced from 73 to 60; S3, soil albedo increased from 0.2 to 0.4; S4, soil drainage factor increased from 0.2 to 0.4; S5, soil organic carbon increased by 1%; S6, pre-plant irrigation of 50 mm.
Effect of Decreasing Runoff Curve Number (S2 Scenario) on Seed-Cotton Yield
The reduction of 18% in curve number from 73 to 60 decreased surface runoff and increased infiltration, and thereby plant available soil water, which contributed to a 7% increase in mean seed-cotton yield (Figure 3). As expected, the effect of decreased surface runoff was the highest in wet years (10%; Figure 4), which received, on an average, about 49 and 115% higher growing season precipitation compared to normal and dry years, respectively (Figure 1). Adimassu et al. (2019) and Gharibdousti et al. (2019) have also reported that conservation practices such as contour farming, zero/no-tillage, conservation tillage with sufficient crop residue increased water infiltration to the soil, which resulted in a reduction in surface runoff and enhanced wheat crop yield. In another field study in Croatia, Bogunovic et al. (2018) observed a significantly lower average annual surface runoff and soil loss under no-tillage and deep tillage than under conventional tillage. However, no-tillage had lower grain yields compared to conventional and deep tillage, but higher yields in dry years due to high capacity for water retention.
Effect of Increasing Soil Albedo (S3 Scenario) on Seed-Cotton Yield
The increase in soil albedo from 0.2 to 0.4 reduced heat flux into the soil, and water vapor flux from the soil (Lascano and Baumhardt, 1996; Bogunovic et al., 2018), resulting in an overall reduction in soil water evaporation and an increase in plant available water, and consequently a 16% increase in mean seed-cotton yield (Figure 3). The effect of increasing soil albedo was relatively higher in the normal years (21%) as compared to dry years (16%) and wet years (14%) (Figure 4). However, the effect of changing soil albedo depends on the average growing season temperature in addition to precipitation. Using a numerical model, Lascano and Baumhardt (1996) also found that the cumulative soil water evaporation was 50% of the seasonal ET in conventional cotton and 31% of the seasonal ET in the wheat-stubble cotton while the seasonable ET was similar in both systems.
Effect of Increasing Soil Drainage Rate (S4 Scenario) on Seed-Cotton Yield
The DSSAT crop models simulate only a one-dimensional water balance with vertical flow, and the downward movement of water within the soil depends on the soil drainage rate (Hoogenboom et al., 2019). Soil drainage rate directly affects the amount of plant available water, and hence accurate calculation of crop yield (Suleiman and Ritchie, 2004). While a higher soil drainage rate may result in a significant deep percolation loss and thereby reduce the plant available water, lower soil drainage rate can cause excess water stress in the cases of extended saturation periods. Saturation resulting from slow soil drainage rate can also cause surface ponding/runoff (Vanderborght et al., 1997; Suleiman and Ritchie, 2004). A soil drainage rate of 0.4 (fraction day−1) is recommended for moderately drained soils (Romero et al., 2012). In this study, an increase in soil drainage factor from 0.2 (baseline) to 0.4 (S4 scenario) enhanced downward movement of water to deeper soil layers and resulted in a reduced soil water evaporation and increased availability of soil water for plant transpiration requirements, and thereby contributing to the highest increase (58%) in simulated mean seed-cotton yield (Figure 3). The effect of increasing soil drainage rate on seed-cotton yield was the highest in normal years (83%) followed by wet years (52%) and dry years (26%) (Figure 4) indicating that the increase in soil drainage rate led to higher percolation losses and hence reduction in plant available water in wet years, compared to normal years.
Effect of Increasing Soil Organic Carbon (S5 Scenario) on Seed-Cotton Yield
A statistically insignificant and slightly declining trend (1% decrease) in simulated mean seed-cotton yield was found with a 1% increase in SOC (Figure 3) and this was contrary to many published results in the literature. For example, Lal (2006, 2020), Qiu et al. (2009), and Baumhardt et al. (2013) report a substantial improvement in crop yield with an increase in SOC. However, large productivity gains with the increase in SOC are associated with the implementation of a combination of practices including judicious application of fertilizers, irrigation, and other amendments. Baumhardt et al. (2013) reported that cotton irrigated at 2.5- and 5.0-mm day−1 averaged 55 kg ha−1 lint for every 25 mm of water used. Increasing soil available water content (AWC) was attributed to greater SOM associated with improved soil health (Cano et al., 2018); that is, a 1% SOM increase in the surface 150 mm of soil expanded AWC by >252.5 m3 ha−1 (NRCS, 2013a). However, increasing the SOC by 1% did not result in any statistically significant increase in our simulated cotton yields compared with simulations for unadjusted soil conditions. The matched increases of 1% SOM and 252.5 m3 ha−1 AWC appearing in an NRCS graphic media (NRCS, 2013b) identified a source article by Mengel (2012) that summarizes results from Australia (Emerson, 1995). In that work, the AWC of loams and fine sandy loams determined between 10-and 1,500-kPa increased 3% by volume with every 1% increase in SOC and was also reported by Hudson (1994) using 1,500-kPa for permanent wilt and 10–33 kPa for field capacity. The NRCS (2019) stipulates AWC is determined at pressures of 33 and 1,500 kPa. By contrast, the near saturated 10 kPa condition may exaggerate the effect of SOM on AWC due to gravity drainage to 33 kPa that in turn reduces potential water for crop use.
Effect of Applying Pre-plant Irrigation (S6 Scenario) on Seed-Cotton Yield
Application of 50 mm of pre-plant irrigation increased mean seed-cotton yield by 12% (Figure 3). Interestingly, the effect of pre-plant irrigation was the highest (21%) under wet years followed by dry years (4%) (Figure 4). In normal years, pre-plant irrigation reduced seed-cotton yield, on an average, by 4%. Water requirement of cotton varies with the growth stages (Himanshu et al., 2019) and hence, in addition to the total amount of rain received during the growing season, its distribution during the growing season is equally important and affects seed-cotton yield. These percent changes in seed-cotton yield with pre-plant irrigation application were statistically not significant (p > 0.05). These trends are consistent with those reported by Bordovsky (2020) from a field experiment near the study site. Bordovsky (2020) found that the commonly applied pre-plant irrigations and larger irrigation applications during the vegetative period did not significantly increase seed-cotton yield due to high evaporative losses during the late spring and early summer in the semi-arid environment prevalent at the experimental site. Evett et al. (2019) have also reported high evaporative losses from sprinkler-irrigated corn fields at Bushland, TX in the THP region.
Effect of Combined Changes in Soil Properties on Seed-Cotton Yield
Combined implementation of changes in four factors, i.e., increase in DUL, soil albedo, and soil drainage rate, and decrease in runoff curve number, significantly affected seed-cotton yield (scenario Sc1) and resulted in a 93% increase in mean (2005–2019) seed-cotton yield (Figure 5). When only soil albedo, soil drainage rate, and runoff curve number were changed (scenario Sc2), the simulated mean seed-cotton yield increased by 86%. Letey (1958) also suggested considering multiple soil properties while assessing productivity potential of a soil because soil properties affect water use potential, oxygen diffusion rate, and mechanical resistance, which have a direct relationship with production.
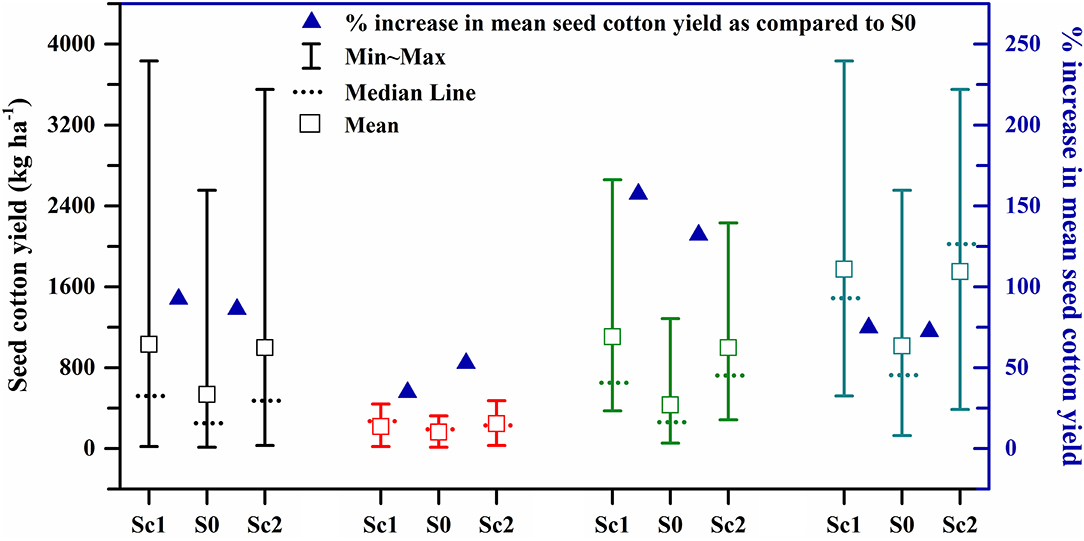
Figure 5. Potential dryland seed-cotton yield increases from combined management of soil properties. Scenario acronyms: S0, baseline; Sc1, combined management of soil properties S1, S2, S3, and S4; Sc2, combined management of soil properties S2, S3, and S4; S1, drained upper limit increased by 25 mm; S2, curve number reduced from 73 to 60; S3, soil albedo increased from 0.2 to 0.4; S4, soil drainage factor increased from 0.2 to 0.4. Black lines represent values for all years combined, while red, green, and cyan lines represent dry, normal, and wet years.
Changing a single soil physical property that is linked with other properties and ignoring their relation may lead to results that do not reflect the interaction that takes place in the soil system. For example, an increase in SOM may change the soil structure, which may lead to changes in bulk density and the porosity of the soil. More importantly, what is ultimately affected is the soil pore distribution, which in turn affects the soil moisture release curve and thus transport of water in the profile. The complexity of capturing the interaction of basic processes with a modification of the state of the system is a key challenge in modeling soil processes (Vereecken et al., 2016). This is the subject of a forthcoming paper where we explore the combined effect of changing soil properties and how they affect the simulated cotton lint yield. This exploration is crucial to provide realistic and practical recommendations on management options to dryland producers in the THP.
The effect of changing multiple soil properties together was the highest under normal years (157 and 132% increase under Sc1 and Sc2, respectively) followed by wet years (75% increase under Sc1 and 73% increase under Sc2). Potential reason for a significantly lower increase in mean seed-cotton yield under wet years as compared to normal years could be development of excess water stress due to excessive precipitation in critical growth stages in some years (Himanshu et al., 2019). Overall, based on these simulations, if a combination of soil health promoting practices could be successfully implemented in dryland cotton production systems in the semi-arid regions, and if these practices resulted in the modeled soil characteristics, then seed-cotton yield could be improved substantially by as high as about 86–93% as compared to the baseline scenario. However, successful implementation of soil health promoting practices in dryland cotton production in the THP region would be challenging. For example, no-till practice results in blowing soil (sand) following a rain event, and hence could potentially destroy an early plant stand. Cover crops could potentially reduce soil water availability for establishing dryland cotton in dry years (Adhikari et al., 2017; Lewis et al., 2018). Moreover, there is no empirical evidence, nor is it suggested, that a simple increase of SOC leads to DUL increases on the order of those modeled herein. It should be borne in mind that the purpose of using a 1% increase in SOC or of an additional 25 mm DUL was to examine the effects of two separate parameters associated with soil health upon potential crop yields.
The Effect of Simulated Scenarios on Expected Mean Revenue and Probability of Large Revenue Losses
Results of the CE, expected (mean) revenue, the probability that a value of $68.81/ha (40% of the mean revenue under the base scenario) or less would be observed in each scenario, and a Kruskal–Wallis test of equivalence of median revenues (compared to the base) are shown in Table 3. In the base case (S0), the CE is equal to $156/ha, the mean revenue is $172/ha, and the probability of observing a revenue ≤$68.81 is 0.34. By comparison, the CE for S1 (DUL) is $242/ha, indicating that producers would prefer the risk adjusted returns for S1 compared to S0, which is reflected in the higher expected revenue per hectare. The increase in DUL does reduce the risk of lower returns to 0.30, or a 4-percentage point decrease in probability. Note that across scenarios, S2 and S5 generate higher mean returns ($237 and $223, respectively) but the Kruskal–Wallis test [low Chi-squared statistic values of 1.29 (S2) and 2.73 (S5)] indicates that the median returns from those scenarios is not statistically different from the base case.
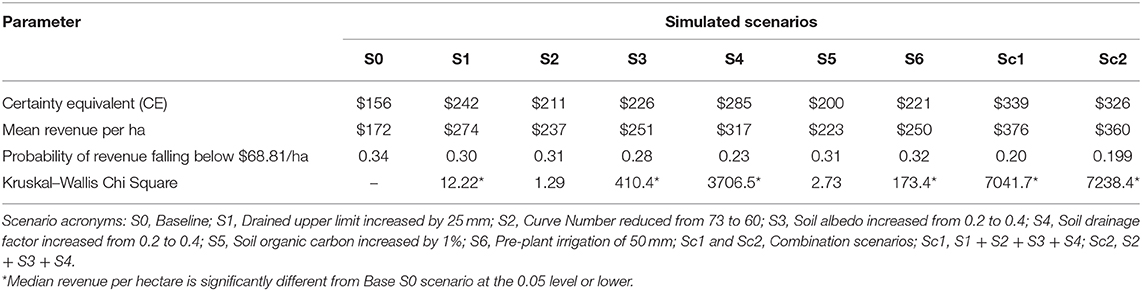
Table 3. Simulated mean revenue, certainty equivalent (CE), and probability of large revenue losses for alternative soil health changes for a risk averse Texas dryland cotton producer.
The single factors that we used in our analysis and that had the largest impact on producer returns is soil drainage rate (S4), with larger CE ($285) and mean returns ($317) while reducing overall risk of revenue shortfall to 0.23 vs. 0.34 in the base case. The combined soil health improvements (Sc1 and Sc2 scenarios) do reduce risk and raise both CE ($339 and $326, respectively) and mean returns [$376 (Sc1) and $360 (Sc2)] relative to all other scenarios and the base (both CE and mean return are over twice the base case). Thus, the results indicated that a combined suite of soil health improvements does reduce revenue risk for producers with most of that impact coming from soil drainage rate improvements. This result, however, does not include the cost (or feasibility) of those improvements and thus impacts profitability.
Conclusions
The potential dryland cotton yield increases from altering soil drainage, albedo, plant available water, SOC, and surface runoff, which are associated with soil health, and pre-plant irrigation were assessed using the DSSAT CSM CROPGRO-Cotton model. Among the six scenarios considered, increasing soil drainage rate scenario (S4) resulted in the highest increase (58%) in simulated mean (2005–2019) seed-cotton yield followed by the increase in DUL scenario (S1; with 27% increase in mean seed-cotton yield) and increase in soil albedo (S3; with 16% increase in mean seed-cotton yield). The increase in SOC (S5) and pre-plant irrigation application (S6) scenarios did not significantly affect mean seed-cotton yield. Potential maximal increases in seed-cotton yield of 93 and 86% were predicted with a combination of four (S1, S2, S3, and S4) and of three scenarios (S2, S3, and S4), respectively. Improvements to soil drainage and combined soil health improvements have also reduced risk to producers and increased mean returns from dryland cotton production relative to all other scenarios including the baseline scenario. These results indicate that the adoption of soil health promoting practices such as leaving crop residue on the surface, no-till, deep/conservation tillage, and growing of cover crops, which potentially improve the soil properties (that were tested in this study), could increase dryland seed-cotton yield within a range of 7–93%, if such practices actually resulted in such soil properties. The contention that a 1% increase in soil carbon would lead to a DUL increase of 25 mm seems specious. Moreover, such increases in seed-cotton yield with changes in soil properties depend on several factors such as soil type, weather, management practices adopted on the farms, and the duration of implementation of soil health promoting practices. Similar assessments at multiple locations in the THP region would be needed to provide useful recommendations for improving dryland cotton production across the study region, and our future efforts will focus on these assessments. We recognize that these results did not consider the synergistic effect that changing a soil physical property might have on other properties and processes. However, these results represent a first analysis and are encouraging to pursue further investigations using a mechanistic approach. Nevertheless, regardless of the approach used, field experiments at the appropriate temporal and spatial are needed to verify results obtained by simulation models. Models are tools that require field experiments to validate the results obtained.
Data Availability Statement
The original contributions presented in the study are included in the article, further inquiries can be directed to the corresponding author/s.
Author Contributions
SA and SH designed the DSSAT model calibration and built the scenarios. SA and DG developed the first draft, which was improved by RL. SH ran the model simulations, analyzed the results, and prepared the figures and tables. SM, DH, TG, RB, DB, RL, and DG assisted in conceptualizing the study and designing model scenarios. DH and BL conducted the economic and risk analyses. JB conducted the field experiment and provided the data for model evaluation. All authors assisted in improving the manuscript.
Funding
This research was provided by the Ogallala Aquifer Program, a consortium between USDA Agricultural Research Service, Kansas State University, Texas AgriLife Research, Texas AgriLife Extension Service, Texas Tech University, and West Texas A&M University.
Disclaimer
The mention of trade or manufacturer names is made for information only and does not imply an endorsement, recommendation, or exclusion by the USDA-Agricultural Research Service. The USDA is an equal opportunity provider and employer. The U.S. Department of Agriculture (USDA) prohibits discrimination in all its programs and activities on the basis of race, color, national origin, age, disability, and where applicable, sex, marital status, familial status, parental status, religion, sexual orientation, genetic information, political beliefs, reprisal, or because all or part of an individual's income is derived from any public assistance program (not all prohibited bases apply to all programs). Persons with disabilities who require alternative means for communication of program information (Braille, large print, audiotape, etc.) should contact USDA's TARGET Center at (202) 720-2600 (voice and TDD). To file a complaint of discrimination, write to USDA, Director, Office of Civil Rights, 1400 Independence Avenue, S.W., Washington, DC, 20250-9410, or call (800) 795-3272 (voice) or (202) 720-6382 (TDD). USDA is an equal opportunity provider and employer.
Conflict of Interest
The authors declare that the research was conducted in the absence of any commercial or financial relationships that could be construed as a potential conflict of interest.
References
Adhikari, P., Ale, S., Bordovsky, J. P., Thorp, K. R., Modala, N. R., Rajan, N., et al. (2016). Simulating future climate change impacts on seed-cotton yield in the Texas High Plains using the CSM-CROPGRO-Cotton model. Agric. Water Manage. 164, 317–330. doi: 10.1016/j.agwat.2015.10.011
Adhikari, P., Omani, N., Ale, S., DeLaune, P. B., Thorp, K. R., Barnes, E. M., et al. (2017). Simulated effects of winter wheat cover crop on cotton production systems of the Texas Rolling Plains. Trans. ASABE 60, 2083–2096. doi: 10.13031/trans.12272
Adimassu, Z., Alemu, G., and Tamene, L. (2019). Effects of tillage and crop residue management on runoff, soil loss and crop yield in the Humid Highlands of Ethiopia. Agr. Syst. 168, 11–18. doi: 10.1016/j.agsy.2018.10.007
Allen, R. G., Pereira, L. S., Raes, D., and Smith, M. (1998). Crop Evapotranspiration. Guidelines for Computing Crop Water Requirements. Rome: FAO Irrigation and drainage paper No. 56. FAO. Available online at: http://www.climasouth.eu/sites/default/files/FAO%2056.pdf (acessed September 19, 2020).
Anderson, J., Harri, A., and Coble, K. (2009). Techniques for multivariate simulation from mixed marginal distributions with application to whole-farm revenue simulation. J Agric. Resour Econ. 34, 53–67. doi: 10.22004/ag.econ.50076
Baumhardt, R. L., Schwartz, R. C., Howell, T. A., Evett, S. R., and Colaizzi, P. D. (2013). Residue management effects on water use and yield of deficit irrigated cotton. Agron. J. 105, 1026–1034. doi: 10.2134/agronj2012.0361
Bogunovic, I., Bilandzija, D., Andabaka, Z., Stupic, D., Comino, J. R., Cacic, M., et al. (2017). Soil compaction under different management practices in a Croatian vineyard. Arab J. Geosci. 10:340. doi: 10.1007/s12517-017-3105-y
Bogunovic, I., Pereira, P., Kisic, I., Sajko, K., and Sraka, M. (2018). Tillage management impacts on soil compaction, erosion and crop yield in Stagnosols (Croatia). Catena 160, 376–384. doi: 10.1016/j.catena.2017.10.009
Bordovsky, J. P. (2020). Preplant and early-season cotton irrigation timing with deficit amounts using subsurface drip (SDI) systems in the Texas High Plains. Irrigation Sci. 38, 485–499. doi: 10.1007/s00271-019-00661-3
Bordovsky, J. P., Mustian, J. T., Ritchie, G. L., and Lewis, K. L. (2015). Cotton irrigation timing with variable seasonal irrigation capacities in the Texas south plains. Appl. Eng. Agric. 31, 883–897. doi: 10.13031/aea.31.10953
Cano, A., Núñez, A., Acosta-Martinez, V., Schipanski, M., Ghimire, R., Rice, C., et al. (2018). Current knowledge and future research directions to link soil health and water conservation in the Ogallala Aquifer region. Geoderma 328, 109–118. doi: 10.1016/j.geoderma.2018.04.027
Chaudhuri, S., and Ale, S. (2014). Long-term (1930–2010) trends in groundwater levels in Texas: influences of soils, landcover and water use. Sci. Total Environ. 490, 379–390. doi: 10.1016/j.scitotenv.2014.05.013
Colaizzi, P. D., Gowda, P. H., Marek, T. H., and Porter, D. O. (2008). Irrigation in the Texas High Plains: a brief history and potential reductions in demand. Irrig. Drain. 58, 257–274. doi: 10.1002/ird.418
Colburn, A. E., and Alexander, U. U. (1986). Furrow Diking in Texas. Texas Agricultural Extension Service Bulletin, B-1539, 7. Availavle online at: https://oaktrust.library.tamu.edu/bitstream/handle/1969.1/129146/Bull1539a.pdf (accessed September 08, 2020).
Emerson, W. W. (1995). Water-retention, organic-C and soil texture. Soil Res. 33, 241–251. doi: 10.1071/sr9950241
Evett, S. R., Marek, G. W., Colaizzi, P. D., Brauer, D. K., and O'Shaughnessy, S. A. (2019). Corn and Sorghum ET, E, Yield, and CWP as affected by irrigation application method: SDI versus mid-elevation spray irrigation. Trans. ASABE 62, 1377–1393. doi: 10.13031/trans.13314
Garcia, A. G., Guerra, L. C., and Hoogenboom, G. (2008). Impact of generated solar radiation on simulated crop growth and yield. Eco. Model. 210, 312–326. doi: 10.1016/j.ecolmodel.2007.08.003
Gemtos, T. A., and Tsiricoglou, T. (1999). Harvesting of cotton residue for energy production. Biomass Bioenerg. 16, 51–59. doi: 10.1016/s0961-9534(98)00065-8
Gerard, C. J., Sexton, P. D., and Conover, D. M. (1984). Effect of furrow diking, subsoiling, and slope position on crop yields 1. Agron. J. 76, 945–950. doi: 10.2134/agronj1984.00021962007600060019x
Gérardeaux, E., Loison, R., Palaï, O., and Sultan, B. (2018). Adaptation strategies to climate change using cotton (Gossypium hirsutum L.) ideotypes in rainfed tropical cropping systems in Sub-Saharan Africa. A modeling approach. Field Crops Res. 226, 38–47. doi: 10.1016/j.fcr.2018.07.007
Gharibdousti, S. R., Kharel, G., and Stoecker, A. (2019). Modeling the impacts of agricultural best management practices on runoff, sediment, and crop yield in an agriculture-pasture intensive watershed. PeerJ 7:e7093. doi: 10.7717/peerj.7093
Gijsman, A. J., Hoogenboom, G., Parton, W. J., and Kerridge, P. C. (2002). Modifying DSSAT crop models for low-input agricultural systems using a soil organic matter-residue module from CENTURY. Agron. J. 94, 462–74. doi: 10.2134/agronj2002.0462
Godwin, D. C., and Singh, U. (1998). “Nitrogen balance and crop response to nitrogen in upland and lowland cropping systems,” in Understanding Options for Agricultural Production, eds G. Y. Tsuji, G. Hoogenboom, and P. K. Thornton (Dordrecht: Kluwer Academic Publishers), 55–77. doi: 10.1007/978-94-017-3624-4_4
Guerra, L. C., Garcia, A. G., Hook, J. E., Harrison, K. A., Thomas, D. L., Stooksbury, D. E., et al. (2007). Irrigation water use estimates based on crop simulation models and kriging. Agric. Water Manage. 89, 199–207. doi: 10.1016/j.agwat.2007.01.010
Harris, R. F., and Bezdicek, D. F. (1994). “Chapter 2: Descriptive aspects of soil Quality/Health,” in Defining Soil Quality for a Sustainable Environment. Vol. 35,eds J. W. Doran, D. C. Coleman, D. F. Bezdicek, and B. A. Stewart (Madison, WI: SSSA Special Publications), 23–35. doi: 10.2136/sssaspecpub35.c2
Hatfield, J. L., Sauer, T. J., and Prueger, J. H. (2001). Managing soils to achieve greater water use efficiency: a review. Agron. J. 93, 271–280 doi: 10.2134/agronj2001.932271x
He, D., and Wang, E. (2019). On the relation between soil water holding capacity and dryland crop productivity. Geoderma 353, 11–24. doi: 10.1016/j.geoderma.2019.06.022
Himanshu, S. K., Ale, S., Bordovsky, J., and Darapuneni, M. (2019). Evaluation of crop-growth-stage-based deficit irrigation strategies for cotton production in the Southern High Plains. Agric. Water Manage. 225:105782. doi: 10.1016/j.agwat.2019.105782
Hoogenboom, G., Porter, C. H., Boote, K. J., Shelia, V., Wilkens, P. W., Singh, U., et al. (2019). “The DSSAT crop modeling ecosystem,” in Advances in Crop Modelling for a Sustainable Agriculture, ed K. J. Boote (Cambridge: Burleigh Dodds Science Publishing), 173–216.
Hudson, B. D. (1994). Soil organic matter and available water capacity. J. Soil Water Conserv. 49, 189–194.
Hulugalle, N. R., Weaver, T. B., Finlay, L. A., Hare, J., and Entwistle, P. C. (2007). Soil properties and crop yields in a dryland Vertisol sown with cotton-based crop rotations. Soil Tillage Res. 93, 356–369. doi: 10.1016/j.still.2006.05.008
Iqbal, J., Read, J. J., Thomasson, A. J., and Jenkins, J. N. (2005). Relationships between soil–landscape and dryland cotton lint yield. Soil Sci. Soc. Am. J. 69, 872–882. doi: 10.2136/sssaj2004.0178
Jones, J. W., Hoogenboom, G., Porter, C. H., Boote, K. J., Batchelor, W. D., Hunt, L. A., et al. (2003). The DSSAT cropping system model. Eur. J. Agron. 18, 235–265. doi: 10.1016/S1161-0301(02)00107-7
Kothari, K., Ale, S., Bordovsky, J. P., Thorp, K. R., Porter, D. O., and Munster, C. L. (2019). Simulation of efficient irrigation management strategies for grain sorghum production over different climate variability classes. Agric. Syst. 170, 49–62. doi: 10.1016/j.agsy.2018.12.011
Lal, R. (2006). Enhancing crop yields in the developing countries through restoration of the soil organic carbon pool in agricultural lands. Land Degrad. Dev. 17, 197–209. doi: 10.1002/ldr.696
Lal, R. (2020). Soil organic matter content and crop yield. J. Soil Water Conserv. 75, 27A–32A. doi: 10.2489/jswc.75.2.27A
Lascano, R. J. (2000). A general system to measure and calculate daily crop water use. Agron. J. 92, 821–832. doi: 10.2134/agronj2000.925821x
Lascano, R. J., and Baumhardt, R. L. (1996). Effects of crop residue on soil and plant water evaporation in a dryland cotton system. Theor. Appl. Climatol. 54, 69–84. doi: 10.1007/bf00863560
Lascano, R. J., and Nelson, J. R. (2014). “Circular planting to enhance rainfall capture in dryland cropping systems at a landscape scale: measurement and simulation,” in Practical Applications of Agricultural System Models to Optimize the Use of Limited Water Advanced Agriculture System, Vol. 5., eds L. R. Ahuja, L. Ma, and R. J. Lascano (Madison, WI: ASA, CSSA, and SSSA), 85–112.
Legates, D. R., and McCabe, G. J. Jr. (1999). Evaluating the use of “goodness-of-fit” measures in hydrologic and hydroclimatic model validation. Water Resour. Res. 35, 233–241. doi: 10.1029/1998WR900018
Letey, J. (1958). “Relationship between soil physical properties and crop production,” in Advances in Soil Science, ed B. A. Stewart (New York, NY: Springer), 277–294. doi: 10.1007/978-1-4612-5046-3_8
Lewis, K. L., Burke, J. A., Keeling, W. S., McCallister, D. M., DeLaune, P. B., and Keeling, J. W. (2018). Soil benefits and yield limitations of cover crop use in Texas High Plains cotton. Agron. J. 110, 1616–1623. doi: 10.2134/agronj2018.02.0092
Luitel, K., Hudson, D., and Knight, T. (2018). Implications and evaluation of crop insurance choices for cotton farmers under the 2014 farm bill. J. Agric. Appl. Econ. 50, 526–543. doi: 10.1017/aae.2018.15
Mengel, D. (2012). “The value of soil organic matter,” in Agronomy e—Updates Number 357. ed S. Watson (Manhattan, KS: K-State Extension Agronomy), 1–2.
Minasny, B., and McBratney, A. B. (2018). Limited effect of organic matter on soil available water capacity. Euro. J. Soil Sci. 69, 39–47. doi: 10.1111/ejss.12475
Modala, N. R., Ale, S., Rajan, N., Munster, C. L., DeLaune, P. B., Thorp, K. R., et al. (2015). Evaluation of the CSM-CROPGRO-Cotton model for the Texas rolling plains region and simulation of deficit irrigation strategies for increasing water use efficiency. Trans. ASABE 58, 685–696. doi: 10.13031/trans.58.10833
Musick, J. T., Pringle, F. B., Harman, W. L., and Stewart, B. A. (1990). Long-term irrigation trends - Texas High Plains. App. Eng. Agric. 6, 717–724 doi: 10.13031/2013.26454
NRCS (2013a). Natural Resources Conservation Service, Soil Health Key Points. Retrieved from: https://www.nrcs.usda.gov/Internet/FSE_DOCUMENTS/stelprdb1082147.pdf (accessed September 08, 2020).
NRCS (2013b). Natural Resources Conservation Service, Soil Health Infographic Series #002: What's Underneath. Retrieved from: https://www.nrcs.usda.gov/Internet/FSE_DOCUMENTS/stelprdb1186089.pdf (accessed September 08, 2020)
NRCS (2019). “Natural resources conservation service, soil properties and qualities: available water capacity,” in National Soil Survey Handbook, Title 430-VI. Available online at: http://www.nrcs.usda.gov/wps/portal/nrcs/detail/soils/ref/?cid=nrcs142p2_054242 (accessed September 08, 2020).
Peel, M. C., Finlayson, B. L., and McMahon, T. A. (2007). “Updated world map of the Köppen-Geiger climate classification,” in Hydrology and Earth System Sciences, Vol. 4 (Discussions, European Geosciences Union), 439–473. Available online at: https://hal.archives-ouvertes.fr/hal-00298818 (accessed October 01, 2020).
Priestley, C. H. B., and Taylor, R. J. (1972). On the assessment of surface heat flux and evaporation using large-scale parameters. Mon. Weather Rev. 100, 81–92. doi: 10.1175/1520-0493(1972)100<0081:otaosh>2.3.co;2
Qiu, J. J., Wang, L. G., Hu, L., Tang, H. J., Li, C. S., and Van Ranst, E. (2009). Modeling the impacts of soil organic carbon content of croplands on crop yields in China. Agric. Sci. China 8, 464–471. doi: 10.1016/s1671-2927(08)60233-3
Raper, T. B., Pilon, C., Singh, V., Snider, J., Stewart, S., and Byrd, S. (2020). “Cotton production in the United States of America: an overview,” in Cotton Production, 1st Edn, eds K. Jabran and B. S. Chauhan (Chichester, UK: John Wiley and Sons Ltd.), 217–247. doi: 10.1002/9781119385523.ch11
Ritchie, J. T. (1998). “Soil water balance and plant water stress,” in Understanding Options for Agricultural Production, eds G. Y. Tsuji, G. Hoogenboom, and P. K. Thornton (Dordrecht: Kluwer Academic Publishers), 41–54. doi: 10.1007/978-94-017-3624-4_3
Romero, C. C., Hoogenboom, G., Baigorria, G. A., Koo, J., Gijsman, A. J., and Wood, S. (2012). Reanalysis of a global soil database for crop and environmental modeling. Env. Mod. Soft. 35, 163–170. doi: 10.1016/j.envsoft.2012.02.018
Sandmo, A. (1971). On the theory of the competitive firm under price uncertainty. Am. Econ. Rev. 61, 65–73.
Scanlon, B. R., Faunt, C. C., Longuevergne, L., Reedy, R. C., Alley, W. M., McGuire, V. L., et al. (2012). Groundwater depletion and sustainability of irrigation in the US High Plains and Central Valley. Proc. Natl. Acad. Sci. U.S.A. 109, 9320–9325. doi: 10.1073/pnas.1200311109
Schwartz, R. C., Baumhardt, R. L., Scanlon, B. R., Bell, J. M., Davis, R. G., Ibragimov, N., et al. (2015). Long-term changes in soil organic carbon and nitrogen under semiarid tillage and cropping practices. Soil Sci. Soc. Am. J. 79, 1771–1781. doi: 10.2136/sssaj2015.06.0241
Suleiman, A. A., and Ritchie, J. T. (2004). Modifications to the DSSAT vertical drainage model for more accurate soil water dynamics estimation. Soil Sci. 169, 745–757. doi: 10.1097/01.ss.0000148740.90616.fd
Sullivan, J. G. (1932). Agriculture of the Southern High Plains. Econ. Geog. 8, 245–261. doi: 10.2307/140435
Thorp, K. R., Ale, S., Bange, M. P., Barnes, E. M., Hoogenboom, G., Lascano, R. J., et al. (2014). Development and application of process-based simulation models for cotton production: A review of past, present, and future directions. J. Cotton Sci. 18, 10–47.
USDA-ERS (2019). United States Department of Agriculture Economic Research Service. Available online at: www.ers.usda.gov (accessed September 8, 2020).
Valipour, M. (2016). How do different factors impact agricultural water management? Open Agric. 1, 89–111. doi: 10.1515/opag-2016-0014
Valipour, M. (2017). Global experience on irrigation management under different scenarios. J. Water Land Develop. 32, 95–102. doi: 10.1515/jwld-2017-0011
Vanderborght, J., Vanclooster, M., Mallants, D., Feyen, J., and Gonzalez, C. (1997). Effects of soil type and water flux on solute transport. Soil Sci. Soc. Am. J. 61, 372–389. doi: 10.2136/sssaj1997.03615995006100020004x
Vereecken, H., Schnepf, A., Hopmans, J. W., Jaxaux, M., Or., D., and Roose, T.. (2016). Modeling soil processes: review, key challenges, and new perspectives. Vadose Zone J. 15, 1–57. doi: 10.2136/vzj2015.09.0131
Wang, E., Cresswell, H., Xu, J., and Jiang, Q. (2009). Capacity of soils to buffer impact of climate variability and value of seasonal forecasts. Agric. Forest. Meteorol. 149, 38–50. doi: 10.1016/j.agrformet.2008.07.001
Wanjura, J. D., Barnes, E. M., Kelley, M. S., Holt, G. A., and Pelletier, M. G. (2014). Quantification and characterization of cotton crop biomass residue. Ind. Crop Prod. 56, 94–104. doi: 10.1016/j.indcrop.2014.02.019
Keywords: DSSAT, CROPGRO-Cotton, pre-plant irrigation, soil management, soil albedo, soil drainage, soil organic carbon, soil water content
Citation: Ale S, Himanshu SK, Mauget SA, Hudson D, Goebel TS, Liu B, Baumhardt RL, Bordovsky JP, Brauer DK, Lascano RJ and Gitz DC III (2021) Simulated Dryland Cotton Yield Response to Selected Scenario Factors Associated With Soil Health. Front. Sustain. Food Syst. 4:617509. doi: 10.3389/fsufs.2020.617509
Received: 14 October 2020; Accepted: 22 December 2020;
Published: 09 February 2021.
Edited by:
Mathias Neumann Andersen, Aarhus University, DenmarkReviewed by:
Yaosheng Wang, Chinese Academy of Agricultural Sciences, ChinaMohammad Valipour, Payame Noor University, Iran
Copyright © 2021 Ale, Himanshu, Mauget, Hudson, Goebel, Liu, Baumhardt, Bordovsky, Brauer, Lascano and Gitz III. This is an open-access article distributed under the terms of the Creative Commons Attribution License (CC BY). The use, distribution or reproduction in other forums is permitted, provided the original author(s) and the copyright owner(s) are credited and that the original publication in this journal is cited, in accordance with accepted academic practice. No use, distribution or reproduction is permitted which does not comply with these terms.
*Correspondence: Srinivasulu Ale, c3JpbmlhbGVAYWcudGFtdS5lZHU=; c3Jpbml2YXN1bHUuYWxlQGdtYWlsLmNvbQ==