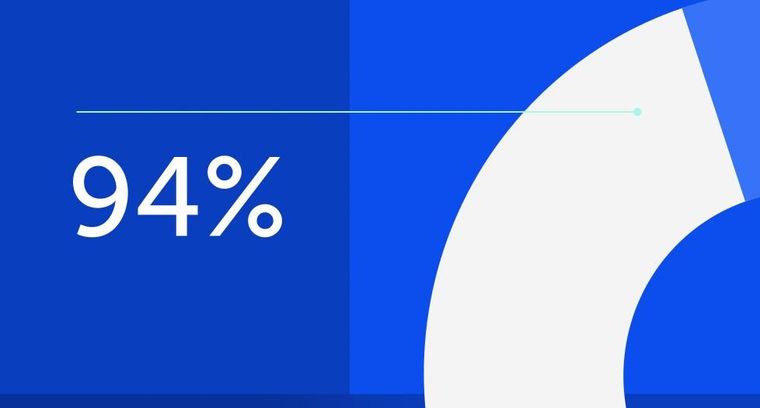
94% of researchers rate our articles as excellent or good
Learn more about the work of our research integrity team to safeguard the quality of each article we publish.
Find out more
ORIGINAL RESEARCH article
Front. Sustain. Food Syst., 25 November 2020
Sec. Agroecology and Ecosystem Services
Volume 4 - 2020 | https://doi.org/10.3389/fsufs.2020.604099
This article is part of the Research TopicIncreasing Resilience through Multi-Species Intercropping, Agroforestry and Mixed Grazing Crop-Livestock SystemsView all 10 articles
Integrated crop–livestock systems are a form of sustainable intensification of agriculture that rely on synergistic relationships between plant and animal system elements to bolster critical agroecosystem processes, with potential impacts on resilience to weather anomalies. We simulated productivity dynamics in an integrated cover crop grazing agroecosystem typical of southern Brazil to gain a better understanding of the impacts of livestock integration on system performance, including future productivity and resilience under climate change. Long-term historical simulations in APSIM showed that the integrated system resulted in greater system-wide productivity than a specialized control system in 77% of simulated years. Although soybean yields were typically lower in the integrated system, the additional forage and livestock production increased total system outputs. Under simulated future climate conditions [representative concentration pathway 8.5 (RCP8.5) scenario from 2020 to 2060], integrated system productivity exceeded specialized system productivity in 95% of years despite declines in average soybean yield and aboveground cover crop biomass production. While the integrated system provided a productivity buffer against chronic climate stress, its resilience to annual weather anomalies depended on disturbance type and timing. This study demonstrates the utility of process-based models for exploring biophysical proxies for resilience, as well as the potential advantages of livestock integration into cropland as a sustainable intensification strategy.
Sustainable intensification of agriculture aims to increase resource-use efficiency and limit expansion of agricultural land area in part by leveraging ecosystem services (e.g., soil structure, nutrient cycling, rangeland restoration) and harnessing ecosystem resilience mechanisms such as soil carbon accrual (Sanderson et al., 2013). System resilience is particularly important for agriculture's ability to cope with climate variability and change, and it is rooted in principles of adaptive capacity, self-organization, and the ability to maintain key ecosystem functions in the face of environmental stressors or disturbances (Walker, 2020). However, quantitative assessments of resilience in intensively managed agroecosystems remain elusive because of these systems' characteristic frequent disturbance regimes and the scarcity of the highly granular datasets for key biophysical functions (Peterson et al., 2018). Even where long-term datasets exist (e.g., Spiegal et al., 2018) for farm- and field-scale biophysical indicators, these remain poorly leveraged toward the examination of system resilience.
A number of recent studies have attempted to formulate proxies of resilience that characterize resistance toward stressful environmental conditions and/or increased capacity to respond to favorable conditions or recover after disturbance (e.g., Standish et al., 2014; Groot et al., 2016; Gil et al., 2017; Li et al., 2019). For example, Szymczak et al. (2020) used Ecological Network Analysis to assess the resilience of nitrogen and phosphorus flows to multiple disturbances (e.g., overgrazing, drought, soil acidification) in a diversified crop–livestock system in Brazil. Although farms and agricultural landscapes are coupled social–ecological systems that require holistic assessment of resilience at multiple scales and across domains, development of simplified, biophysical proxies of resilience and adaptive capacity (i.e., the ability to maintain function by changing in response to disturbance) represent crucial attempts to make the concept of resilience operational for intensive production systems. Furthermore, these proxies provide a bridge to better understanding the underlying mechanisms of resilience in these systems to guide planning and management.
Diversification of agroecosystems—whether biological, temporal, or spatial—has been identified as a crucial mechanism for resilience building through both maintenance of ecosystem services and cascading effects across scales (e.g., Lin, 2011; Renard and Tilman, 2019; Bowles et al., 2020). However, traditionally biodiverse integrated crop and livestock systems have followed a trend of increasing specialization over the last few decades (Garrett et al., 2020), improving productivity per unit land area and labor but exacerbating pollution, land degradation, vulnerability to climate extremes, and exposure to market fluctuations (Naylor et al., 2005; Gil et al., 2016). Recoupling of specialized crop and livestock operations as integrated crop–livestock systems (ICLSs) has shown promise as a sustainable intensification strategy (Peterson et al., 2020) as indicated by shifts in soil biological, physical, and chemical properties (Carvalho et al., 2018; Deiss et al., 2019), water and nutrient cycling (Assmann et al., 2015; Martins et al., 2016a), and plant–soil interactions (Peterson et al., 2019), typically without significantly affecting soybean grain productivity (Kunrath et al., 2015). ICLSs are also reported for foster climate change resilience via buffering mechanisms in both field-level biophysical processes, e.g., nutrient cycling (Szymczak et al., 2020) or improved crop productivity (Tracy and Zhang, 2008), and farm-level socioeconomics, e.g., economic risk mitigation and livelihood diversification (Bell et al., 2014).
However, implementing commercial-scale ICLS represents a complex challenge (Garrett et al., 2017), and the viability of ICLS as a sustainable intensification strategy is a pressing question in southern Brazil. Here, ICLSs are widely implemented in the form of an annual rotation of soybean followed by a grass cover crop grazed by beef cattle (finishing steers; Moraes et al., 2014). In the near-term future, annual precipitation in southern Brazil is projected to increase by 5 to 10%, along with an increase in the frequency of extreme rainfall events and increase in temperature from 1.5 to 4°C (Marengo et al., 2009; Chou et al., 2014). Together, these changes could impact agriculture through increased runoff and erosion risks, crop heat stress from higher daytime and nighttime temperatures, and decreased incident solar radiation due to cloud cover. Variability in precipitation is a particular concern for soybeans and is one of the primary causes of soybean yield gaps at our experimental site (Cecagno et al., 2016) and in southern Brazil more broadly (Sentelhas et al., 2015). However, it is uncertain how future conditions will impact beef–soybean ICLS given the shortage of long-term, data-intensive field experiments needed to understand the multiyear dynamics and resilience of essential agroecosystem structures and functions.
In this study, we use process-based model simulations as a tool to understand the potential impacts of climate change on beef–soybean ICLS productivity and its viability as a sustainable intensification strategy in southern Brazil. Modeling approaches are ideally suited for evaluating long-term productivity dynamics and addressing questions pertaining to resilience that are impractical to test in the field, such as predicting the impacts of novel chronic environmental conditions or quantifying response to stochastic stress events (Kahiluoto et al., 2014). Simulation approaches have been applied for some commercial-scale ICLS, for example, dual-purpose cropping systems and crop-pasture rotations in Australia and the United States (Zhang et al., 2008; Moore, 2009; Robertson et al., 2009; Lilley et al., 2015), silvopastoral systems in Europe (Balandier et al., 2003), and crop residue grazing in Australia (Thomas et al., 2010; Bell et al., 2011). However, cover crop grazing and annual crop–forage rotations, and especially their field-level response and resilience to climate change, have not been explored widely using process-based models.
We used the Agricultural Production Systems sIMulator (APSIM; Holzworth et al., 2014) to simulate long-term productivity outcomes and derive a simple proxy of resilience for an integrated soybean–beef cover crop grazing ICLS and a specialized (soybean-ungrazed cover crop) reference system in southern Brazil. We hypothesized that soybean yields in the integrated system would not differ from the specialized system in most years, but would (1) outperform the specialized system in extreme weather years (10th or 90th percentile of annual precipitation), (2) exhibit smaller production losses in low rainfall years, and (3) exhibit larger production gains in favorable/high rainfall years. Furthermore, the added production output from the grazing operation would increase field-level productivity of the integrated relative to the specialized system even under chronic adverse future climate conditions. Our results broaden the scope of process-based modeling tools for applications in ICLS and provide expanded insight into the resilience of cover crop grazing operations under future climate conditions.
A long-term experiment located at Espinilho Farm in Rio Grande do Sul, Brazil (28°56′14″S 54°20′52″W, 465-m elevation), has monitored cash crop, cover crop, and animal productivity and soil characteristics in a no-till, beef–soybean integrated system for over 18 years (since 2001; Carvalho et al., 2018). The climate is humid subtropical with an average annual temperature of 19°C and average annual precipitation of 1,850 mm, with a fairly even seasonal distribution. The soil is a deep, well-drained Oxisol (Rhodic Hapludox), acidic, with a surface soil (0–20 cm) texture of 54% clay, 27% silt, and 19% sand.
Details on experimental design and management protocols at the site are described in Peterson et al. (2019). Briefly, the integrated beef–soybean system involves a cover crop mixture of black oat (Avena strigosa Schreb.)/Italian ryegrass (Lolium multiflorum Lam.) grazed by yearling beef steers in winter, followed by a summer soybean (Glycine max [L.] Merr.) grain crop. Black oat is sown in early autumn (April–May), while Italian ryegrass establishes itself from the soil seed bank. The experiment examines the production and environmental impacts of the integrated system with four cover crop grazing intensities ranging from heavy to light (defined by sward heights of 10, 20, 30, and 40 cm) compared to an ungrazed, cover-crop-only control (specialized system). The specialized system utilizes the same black oat/Italian ryegrass cover crop mixture as the integrated system but it is left ungrazed, a typical management practice in this region for the purposes of soil protection during the winter and biomass production for no-till operations. Key ongoing experimental measures taken over the 18+ years of the experiment include (1) soil attributes such as bulk density and porosity (Cecagno et al., 2016), carbon and nitrogen fractions and pools (Assmann et al., 2015), pH (Martins et al., 2016b), moisture content (Martins et al., 2016a), and aggregation (Conte et al., 2011); (2) soybean crop attributes such as aboveground biomass accumulation (da Silva et al., 2014), residue (Assmann et al., 2015), leaf water potential (Martins et al., 2016a), and initial population density, yields, and yield components (Kunrath et al., 2015); (3) winter cover crop attributes such as spatial heterogeneity (Nunes et al., 2018), sward height, residue, forage allowance, and total aboveground biomass accumulation (Kunrath et al., 2020); and (4) livestock attributes such as forage intake (de Souza Filho et al., 2019), live weight (LW) gain (Carvalho et al., 2018), and manure distribution (da Silva et al., 2014).
In winter, paddocks are continuously stocked with beef steers after accumulating 1,500 to 1,600 kg ha−1 dry matter (24 cm sward height). Sward heights are maintained using the put-and-take method (Mott and Lucas, 1952), and the stocking period lasts an average of 120 days at stocking rates ranging from 300 to 1,400 kg LW ha−1 depending on grazing treatment (Kunrath et al., 2020). Urea N is applied in two doses during the winter, and potassium chloride and triple superphosphate are applied at cover crop sowing. At the end of the grazing period, paddocks are desiccated with glyphosate. Soybean is direct seeded into cover crop residues between 15 November and 15 December and harvested after ~120 days. Fungicides, insecticides, and foliar fertilizers are applied as needed throughout the growing season.
APSIM v7.9 is a biophysical modeling software framework designed to capture whole-system outcomes by combining a suite of interconnected crop, soil, and management modules with the ability to set management rules that emulate a variety of production systems and scenarios (Holzworth et al., 2014). A description of the APSIM software can be found in Holzworth et al. (2014) and online at https://www.apsim.info/. The following APSIM modules were used to represent the experimental site and treatments: Soybean, AusFarm Pasture, SoilWater, SoilN, Surface OM, Soil OM, and Fertilizer (Probert et al., 1998; Holzworth et al., 2014; Dalgliesh et al., 2016). APSIM-Soybean belongs to the PLANT family of crop modules, which simulates crop growth on a daily time-step per unit area in response to climate, soil water supply, and soil nitrogen (Robertson et al., 2002). The integrated AusFarm Pasture module recognizes four functional groups of forage plants—annuals, perennials, grasses, and forbs—and models phenological development according to daily environmental variables (Moore et al., 1997). Model configuration for soil and production parameters for the integrated system were based on the moderately grazed treatments, where sward heights were maintained between 20 and 30 cm, and from the specialized control system (Supplementary Tables 1, 2). Previous studies at the site have shown that the moderate grazing intensity treatments represent the best management practice for the integrated system based on optimization of soil health and animal production outcomes (Carvalho et al., 2018).
APSIM was configured for the integrated (grazed) and specialized (ungrazed) paddocks and modeled estimates were compared to published and unpublished datasets from the study site for soybean yield, cover crop biomass production (aboveground), animal LW production, and soil water content (Supplementary Table 1). Modeled estimates for the period 2002–2017 were graphically compared with observed data from the experimental site, and model performance was evaluated in terms of root mean square error (RMSE), relative root mean square error (RRMSE), and modeling efficiency (ME). A low RMSE/RRMSE indicates good agreement between simulated and observed values, while a high ME indicates good predictive ability of the model (Wallach et al., 2014). ME is a dimensionless statistic related to the coefficient of determination, but based on the proportion of variation explained by the 1:1 relationship between simulated and observed values (i.e., y = x). An ME of 1 indicates a perfectly fitted model. Acceptable levels of model error vary depending on study objectives. For a study such as this where treatment effects are the main objective, RMSE is considered very good if it is within 10% of observed mean values and acceptable if it is within 20% or if simulation error is no greater than experimental error (Ma et al., 2011). For ecological models where experimental uncertainty may be high, an ME of > 0.5 may be considered acceptable (e.g., Moriasi et al., 2007 for a watershed simulation). For crop and soil modules in APSIM, an ME of >0.7 has been used as an indicator of acceptable calibration (Archontoulis et al., 2014).
Management rules for the specialized system were set according to observed sowing, fertilizer, tillage (no-till), harvest, and crop termination operations at the experimental site. Model estimation of the timing of aboveground biomass accumulation in the cover crop was improved by setting planting depth to an artificially extreme 200 mm to recreate the effect of slower seedling emergence through layers of surface residual matter that can be 50 to 200 mm thick in this system (Caetano, 2017). Due to limitations in modeling mixed-species paddocks, cover crops were simulated using only ryegrass rather than the ryegrass/oat mixture used at the experimental site. This setting is representative of general practice in the greater Planalto region, where grazed or ungrazed pure ryegrass cover crops are common due to their high nutritive value as a winter forage as well as their ability to supply soil cover for direct-seeding operations (Conterato et al., 2016). To best represent the observed aboveground biomass accumulation of the ryegrass/oat mixture, simulated ryegrass planting was set to occur on 2 February every year at a seeding rate of 80 kg ha−1.
For the integrated system, a number of simplifications were made to accommodate the limitations of the model. Instead of simulating the livestock dynamics in a put-and-take grazing management system for the cover crop phase, we used a defoliation rule to approximate the total aboveground biomass removal observed experimentally. The defoliation rule made cuts of 80 mm height (~750 kg ha−1 of dry matter or ~50% of sward height) on a regular basis after the ryegrass exceeded a threshold of 1,500 kg ha−1 of available dry matter. This led to an approximate average of 2,500 kg ha−1 of available dry matter during the grazing window, or 20 to 30 cm of sward height, in agreement with target sward heights for the experimental site. Cover crop defoliation was implemented until the end of the grazing period (1 November) for all simulated years, after which the cover crop was killed. This rule was implemented to represent cattle grazing of the cover crop in amounts that approximate the maximum amount of dry matter intake per unit LW per day in beef steers. The latter is a function of the energy content of the feed and LW0.75 (NRC, 2016) as well as sward height, with sward height being particularly relevant in pasture-based systems (de Souza Filho et al., 2019). Total aboveground biomass production in the integrated (grazed) system was calculated as the sum of end-of-season standing shoot biomass and cumulative biomass removal by cattle annually, and in the specialized (ungrazed) system as the maximum standing shoot biomass. Simulations were N-unlimited for both ryegrass and soybean phases, so the contributions of dung and urine to N cycling were omitted for simplicity. Therefore, this simulation plays to the model's strengths of capturing the general effects of defoliation and aboveground biomass accumulation on soil water and C cycling and consequences for crop yield, rather than the more subtle effects of grazing animal activity on soil chemical (e.g., pH, cation exchange capacity, base saturation) and biological (e.g., root nodulation, microbial activity) parameters.
The soybean crop module was configured such that sowing was in accordance with observed dates, seeding rates (38 kg ha−1), and row spacings (450 mm). Two different soybean varieties have been planted during the experimental period, neither of which has been parameterized in the APSIM-soybean module. For this reason, we chose a cultivar (Hutcheson_5.0) that best approximated the phenology of sown crops and the photoperiod sensitivity and crop longevity of commonly grown cultivars in southern Brazil and Argentina (Conterato et al., 2016). Soybean was harvested when the growth stage reached mature/ripe, typically in late March/April in agreement with experimental observations. Volumetric water content was set to carry over from 1 year to the next, allowing the model to simulate the refilling and depletion of soil water over the cropping cycle.
Parameter values for plant available water capacity, soil texture, percent organic carbon, C:N ratio of soil organic matter, starting nitrogen concentrations, and additional soil chemical and physical properties were derived from direct measurements at the site and assigned as denoted in Supplemental Table 2. Direct measurements of soybean and ryegrass KL (fraction of plant available water extracted from a layer of soil per day) and XF (root exploration factor) were not available for this site, therefore these parameters were estimated so as to minimize error between model estimates and observed measurements. Sensitivity tests for these parameters were performed to ensure that model behavior was robust (e.g., Holzworth et al., 2011).
Parameter values for various soil physical properties differed between the integrated and specialized systems in accordance with observed differences at the field site. These differences include lower bulk density at depth, lower saturated soil water content, higher pH, and lower aluminum concentrations in soils in the integrated system. Many of these differences have been attributed to grazing effects, especially increased root litter deposition in grazed ryegrass (Wilson et al., 2018), increased soil macrofauna activity (Marchão et al., 2009), and altered nutrient cycling dynamics (da Silva et al., 2014; Assmann et al., 2015). For example, the combination of acidity and high aluminum tends to restrict root elongation in soybeans, which translates to the lower XF assigned to the specialized system (Silva et al., 2001; Supplementary Table 2).
Daily historical minimum and maximum temperatures, solar radiation, and precipitation for the calibration period (2002–2017) were obtained from the weather station at Cruz Alta, RS, 78 km from the experimental site. This weather station was chosen because it had the longest continuous dataset for the above variables and showed reasonable similarity to the 1 to 2 years of daily records available from an on-site weather station (R2 of 0.99 for the relationship between solar radiation records and 0.88 for temperature records between the two sites). Where gaps in the Cruz Alta temperature and solar radiation record occurred, missing values were interpolated with downscaled daily satellite observations from the LaRC POWER single point data access tool (Stackhouse, 2010). Missing precipitation values were infilled with data from the nearest available weather stations at São Luiz Gonzaga (81 km from site) and Julio de Castilhos (72 km from site).
In addition to model predictions of soybean yield and cover crop aboveground biomass accumulation, we developed a post-model prediction of beef production (Supplementary Figure 3) and system gross margins. All post-model analyses were conducted in R v3.5.2 statistical programming software (R Core Team, 2018). We estimated animal LW gain (kg ha−1) over the grazing period as a function of model-predicted forage removal (i.e., defoliated biomass) and a forage conversion ratio (FCR), that is, the ratio of dry matter intake to unit LW gain (Wilkinson, 2011). We opted for a conservative FCR of 14:1 based on experimental estimates of forage intake and animal weight gain (de Souza Filho et al., 2019). From this value we calculated end-of-season animal LW gain as:
We used gross margins as a proxy of field-level productivity, similar to total calorie or human digestible protein production, because it accounts for productivity originating from both the crop and livestock enterprises of the system. In this study, gross margins are not a complete economic treatment of the farm system, but rather a reflection of relative productivity between the integrated and specialized systems at the level of the field. To calculate gross margins for each simulated year, we estimated revenues using the average prices for soybean and beef LW from 2013 to 2017 (Food Agriculture Organization of the United Nations, 2018) multiplied by production predictions, and subtracted the typical production costs for each system. Prices were set as the 5 year (2013–2017) average in local currency units (LCU, Brazilian Reals) without considering long-term trends. As such, the analysis rests on the assumption that prices and input costs are constant and will remain fundamentally unchanged in the future (see Thamo et al., 2017 for a similar approach).
For the specialized system, fixed costs for the soybean enterprise and for the establishment and maintenance of the winter cover crop were obtained from a previous economic analysis of the experimental site (Oliveira et al., 2013). In this analysis, the authors calculated costs for the soybean enterprise as the sum of input costs, including soybean seed and desiccant, and operation costs, including sowing, fertilizing, pesticide application, and harvesting. For the integrated system, costs for the livestock enterprise were calculated as 90% of the expected revenues, which was representative of the typical margin for livestock sales (Oliveira et al., 2013) not including the cost of steer purchase. This assumption was the most representative of reality given that costs associated with the livestock enterprise are expected to vary in accordance with management decisions and the quality and supply of forage during the grazing season. We also tested a fixed cost approach and a variable cost approach derived from the linear model of the relationship between costs and length of the grazing window. The effects of these assumptions on the outcomes for gross margins are illustrated in Supplementary Figure 4.
Simulations were run using long-term observed meteorological data for the historical period, and using the bias-corrected predicted weather conditions for future climate scenarios. Daily historical minimum and maximum temperature, solar radiation, and precipitation for the years 1961–2002 were obtained from the Princeton Global Meteorological Forcing Dataset (Sheffield et al., 2006) and reanalyzed in the CCAFS GCM Data Portal (Navarro and Tarapues, 2015). Near-future meteorological conditions for representative concentration pathway (RCP) 8.5 were simulated for the years 2020–2060 (Riahi et al., 2011) with an ensemble of 6 CMIP5 Global Climate Models (GCMs) (Taylor et al., 2012): CMCC CMS, CSIRO Mk3.6.0, GFDL ESM2G, IPSL CM5A LR, MIROC5, and MPI ESM MR. This ensemble is a reasonable representation of regional climate projections for southern Brazil considering the availability of adequate data for downscaling. GCMs were calibrated with the Princeton dataset, and the RCP8.5 scenario was simulated in the CCAFS Data Portal. Raw GCM outputs were bias-corrected using the quantile mapping method in the qmap package of R (Gudmundsson, 2016), which corrects for GCM tendency to produce too many “drizzle days,” i.e., small-scale rainfall events (Gudmundsson et al., 2012).
Soybean sowing for the long-term simulations was modified to a conditional rule, whereupon the crop was sown within a specified window once minimum rainfall requirements of 30 mm for 3 consecutive days were met, or alternatively, the end of the sowing window (25 December) was reached. In addition to the Hutcheson_5.0 cultivar, a modified cultivar was tested that required 10% more thermal time for each growth stage to account for changes in season length under future climate conditions. All other soil and crop parameters and management rules remained the same as in the initial calibration.
In this study, we used the definition of resilience for managed agroecosystems proposed by Peterson et al. (2018), following Conway (1986) and Rist et al. (2014). This definition considers both the ability of a system to resist external disturbance (resistance or decreased downside) and to recover to usual levels of productivity after a disturbance (recovery or enhanced upside). This definition also places resilience in the context of the objectives and outcomes that human agents value in managed production systems. Accordingly, resilience can be either desirable or undesirable depending on whether it characterizes a system that is reliably performing needed functions to the needed degree (Standish et al., 2014; Peterson et al., 2018). For example, a system that is resistant toward drought but that always produces sub-optimal yields is exhibiting resilience, but not desirable resilience.
To describe resilience in our experimental systems, and in particular the downside and upside risks, we first calculated ranked Probability of Exceedance (POE) using the Weibull method (Makkonen, 2006). The POE represented the likelihood that soybean yield, forage cover crop aboveground biomass production, or field-level gross margins would benefit or be penalized by the inclusion of animal grazing in the system. Differences in the indicator variables between the integrated and specialized systems were ranked from most negative to most positive and then represented as a proportion of all observations as in Equation 2:
where r is the observation's rank and n is the number of observations.
We also calculated a proxy of resilience to abnormal annual precipitation for soybean, cover crop, and economic productivity outcomes, which we refer to here as the R-index. The R-index reflects the percent change in productivity metrics in response to a disturbance, in this case high (90th percentile) or low (10th percentile) precipitation years, in comparison to baseline average annual precipitation. Past research at this site has shown that the main driver in variability of soybean yields is variability in annual precipitation (Cecagno et al., 2016). APSIM stress indices also showed that soil water stress is an important driver of variability in both soybean yields and cover crop biomass production (Supplementary Figure 6 and Supplementary Table 3). The R-index was intended to capture both resistance to precipitation disturbance, indicated by a smaller negative percent change in unfavorable precipitation years, and capacity for recovery/enhanced upside, indicated by a larger positive percent change in favorable precipitation years. Smaller negative percent changes and larger positive percent changes indicated greater resilience in one system compared to the other. These within-system responses were then contextualized by the comparative magnitude of productivity of the given indicator to demonstrate whether or not resilience was a desirable system property in each scenario. For example, if soybean yields in one system exhibited higher resistance to a precipitation disturbance than the other but lower yields on average, this outcome would not reflect desirable resilience.
Model performance for soybean yield was acceptable, with RMSE of 598 kg ha−1, RRMSE of 21%, and ME of 0.69 for soybean yield predictions (Figure 1A). Generally, the model accurately predicted the yield of soybean in the integrated compared to the specialized system and correctly tracked year-to-year fluctuations in yield (Supplementary Figure 1). However, in a few cases it was less accurate, underpredicting soybean yields during highly productive years and overpredicting yields during extremely poor years, the latter of which tended to coincide with drought years at this site. Model predictions for cover crop aboveground biomass production were also acceptable, with RMSE of 1,210 kg DM ha−1, RRMSE of 37%, and ME of 0.58 (Figure 1B). Model predictions for aboveground biomass production in the specialized system agreed well with observed data but tended to simulate peak biomass later than observations (Supplementary Figure 2). For the integrated system, on the other hand, observed data were more variable than model simulations.
Figure 1. Relationship between observed and simulated (A) soybean yield and (B) cover crop aboveground biomass production for the calibration period in the integrated system (grazed cover crop; solid points) and the specialized control (ungrazed cover crop; open points). Model evaluation indices are root mean square error (RMSE), relative root mean square error (RRMSE), and modeling efficiency (ME).
The post-hoc model of animal gain, based on the cover crop biomass production and forage conversion ratio, poorly tracked observed year-to-year fluctuations in animal LW gain (RMSE = 82 kg ha−1, RRMSE = 19%, ME = −0.50; Supplementary Figure 3). However, other observed variables from 2002 to 2016 such as length of the grazing window and stocking rate explained less of the variability in model estimates than dry matter production. The model tended to underestimate animal LW gain in highly productive years and overestimate gain in poor production years. Model estimates were therefore conservative but produced reasonable estimates of long-term average animal gains such that they were sufficient for use in the subsequent analysis of gross margins.
Model outputs for soil water content agreed with observed data from 2016 to 2017 growing season and adequately predicted lower soil volumetric water content in the integrated system at both depths (Supplementary Figure 5). Model predictions for volumetric water content had a RMSE of 0.05 cm3 cm−3, RRMSE of 15%, and ME of 0.51. Magnitude and dynamics of soil water depletion and replenishment were comparable between observed and simulated volumetric water content values during the critical soybean grain-filling stages (January to mid-February) and deviations mostly occurred during the early and late stages of the soybean growing season. Deviations in estimates of soil water content during these stages generally did not affect the accuracy of predictions for soybean yields.
For 1961–2017, an overall mean soybean yield of 3,300 kg ha−1 was predicted across system types and years. A soybean yield penalty was predicted for the integrated system compared with the specialized system in 80% of years (Figure 2A). However, these yield penalties were minor, <400 kg ha−1 in over 75% of simulated years. Years in which integrated system yields were penalized relative to the specialized system typically coincided with years in which soil water stress occurred in the critical grain-filling growth stages. APSIM stress indices indicated that soil water stress had a strong negative relationship with soybean yield (Supplementary Figure 6 and Supplementary Table 3), whereas temperature stress and variation in net solar radiation had relatively minor impacts on yield.
Figure 2. Simulated difference in productivity indicators in the integrated system (grazed cover crop) compared with the specialized control (ungrazed cover crop). Values for the specialized system are set at 0, with points representing relative productive penalty or benefit for each simulated year in the integrated system. Productivity indicators are (A) soybean yield, (B) ryegrass cover crop aboveground biomass production, and (C) gross margins under historical (black points) or future (green triangles) climate conditions.
From 1961 to 2017, mean total cover crop aboveground biomass production (including biomass removed by cattle grazing) across systems was 7,500 kg DM ha−1. Grazed cover crops in the integrated system exceeded the aboveground biomass production of ungrazed cover crops in the specialized system in more than 90% of years (Figure 2B), with a forage yield benefit of over 1,000 kg DM ha−1 in over 60% of years. In a given year, an average of 4,900 kg DM ha−1 was removed by cattle in the integrated system. As a result, maximum standing aboveground biomass in the integrated system was typically less than maximum standing aboveground biomass in the specialized system at any given time point (3,900 kg DM ha−1 on average for the specialized and 2,200 kg DM ha−1 on average for the integrated system), but cumulative production (including removed biomass) was greater in the grazed, integrated system (7,060 kg DM ha−1 on average in the specialized vs. 7,890 kg DM ha−1 on average in the integrated system). As with soybean yields, cover crop aboveground biomass production typically decreased with increasing soil water stress and decreasing length of the grazing season (as indicated by the day of the first biomass cutting; Supplementary Figures 6, 7 and Supplementary Tables 3, 4).
Field-level productivity (as measured by gross margin from crop and livestock production) was on average 23% higher in the integrated system compared to the specialized system despite the yield penalties observed for soybeans (Figures 3A,B). From 1961 to 2017, mean gross margins were 3,177 LCU per hectare per year for the integrated system compared to 2,583 LCU ha−1 yr−1 in the specialized systems. The integrated system earned an average of 4,780 LCU ha−1 yr−1 in revenue from beef production and 3,661 LCU ha−1 yr−1 in revenue from soybean production with costs of 4,302 and 962 LCU ha−1 yr−1 for beef and soybean production, respectively (Figure 3). In contrast, the specialized system produced 3,908 LCU ha−1 yr−1 in revenue from soybean production and roughly 1,325 LCU ha−1 yr−1 in costs for cover crop maintenance and soybean production (Figure 3B). Under historical climate conditions, simulated annual gross margin in the integrated system exceeded gross margin in the specialized system in 77% of years (Figure 2C). These gross margin surpluses in the integrated system ranged from 22 LCU ha−1 yr−1 to more than 890 LCU ha−1 yr−1, while deficits ranged from 14 to 786 LCU ha−1 yr−1. Annual gross margin penalties were typically incurred in years with poor cover crop biomass production, when revenues from beef production were not sufficient to offset soybean yield penalties and operation costs in the integrated system.
Figure 3. Contributions of soybean and livestock revenues and production costs to gross margins for integrated (grazed cover crop; A,B) and specialized (ungrazed cover crop; C,D) system under historical (A,C; 1961–2017) and future (B,D; 2020–2060) climate scenarios.
Under the RCP8.5 future climate scenario for 2020–2060, the GCM ensemble projected a 23% increase in annual precipitation, from an average of 1,830 mm historically to 2,260 mm in the future simulation (Figures 4A,B). This change came mostly from a 52% increase in summer precipitation under the future scenario (from 915 to 1,389 mm), whereas precipitation during the winter cover crop phase remained relatively unchanged (916 mm yr−1 historically to 869 mm yr−1 in the future; Figure 4B). Average annual maximum temperature increased in both the soybean phase (Figure 4C) and the cover crop phase (Figure 4D), with an increase of 3°C predicted for both seasons. Inter-annual variance in precipitation accumulation did not differ between historical and future simulations for either the soybean or cover crop phase, with a coefficient of variation between 23 and 29% across seasons and years. Likewise, within-season variation was similar between the historical and future simulations with coefficients of variation of 23 and 20%, respectively. The average frequency of extreme rainfall events (daily precipitation of >3 standard deviations from the historical mean) was slightly higher in the future simulation, increasing from an average of 12 extreme events per year (SE = 0.78) to 16 extreme events per year (SE = 0.73).
Figure 4. Change in (A,B) mean annual precipitation accumulation and (C,D) average annual maximum temperature in (A,C) the soybean phase and (B,D) ryegrass cover crop phase at the experimental site, as predicted by a six-model ensemble of CMIP5 GCMs under the RCP8.5 global emissions scenario.
Simulations under the RCP8.5 future climate scenario projected a 15% decline in mean soybean yield, from an average of 3,300 kg ha−1 under historical conditions to 2,900 kg ha−1 under future conditions (Table 1). Soybean yield reductions were partially related to soil water stress, though the response was less severe than in the historical simulation (Supplementary Figure 6). In the future simulation, soybean yield declines were also related to faster crop maturation times due to higher average temperatures during the growing season (Supplementary Figure 8 and Supplementary Table 5). The potential to adapt soybean genotypes to this altered climate was tested by re-running the future simulation with a cultivar with a 10% greater thermal time requirement for each phenological stage. Predicted mean yields using this adapted cultivar were 3,400 kg ha−1, equivalent to the yields predicted under the historical simulation and 15% higher than predicted future yields using the non-adapted cultivar (Supplementary Figure 8 and Supplementary Table 5).
Table 1. Predicted mean and change in mean soybean yield, ryegrass cover crop aboveground biomass production, and gross margins from historical (1961–2017) to future (2020–2060) simulation periods for integrated (grazed cover crop) and specialized (ungrazed cover crop) systems.
While overall soybean yields declined when using the non-adapted cultivar, soybean yields in the integrated system increased relative to the specialized system (Figure 2A and Table 1); the average deficit of 220 kg ha−1 under historical climate was reduced to 90 kg ha−1 under the future climate. Frequency of yield benefit from the integrated system increased from−20% of years in the historical simulation to 35% of years in the future simulation (Figure 2A). Yield differences between the integrated and specialized systems were smaller in the future simulation, with yield penalties of more than 400 kg ha−1 projected for <5% of years compared to 22% of years in the historical simulation (Figure 2A).
Mean cover crop aboveground biomass production declined by 23% under the future climate scenario (Table 1). Unlike soybean yields, this decline in cover crop biomass production was not driven by soil water stress, as higher summer rainfall accumulation and lower winter biomass production would result in both increased initial soil water content and decreased soil water consumption through transpiration. However, length of the winter grazing season (as indicated by the date of the last biomass cutting) decreased by 20%, from ~116 days in the historical simulation to 93 days in the future simulation (Supplementary Figure 7 and Supplementary Table 4). Temperature stress and reduced solar radiation did not impact aboveground biomass production in the future simulation. While overall cover crop aboveground biomass production declined, relative differences in biomass production between the integrated and specialized system remained the same (Figure 2B). Frequency of higher aboveground biomass production for the integrated system increased from 93 to 98% of years in the future climate compared to the historical climate scenarios (Figure 2B).
Future climate conditions were projected to have a negative impact on gross margins across systems (Table 1). In the integrated system, mean gross margins declined by 540 LCU ha−1 yr−1 in the future simulation, while in the specialized system mean gross margins declined by 581 LCU ha−1 yr−1. The difference in total field-level productivity between the systems was reduced in the future climate. Average future soybean revenues declined more in the specialized system (581 LCU ha−1 yr−1 less) than in the integrated system (434 LCU ha−1 yr−1 less). In the integrated system, the revenue loss from livestock production was almost twice as much as revenue loss from soybean production (1,061 LCU ha−1 yr−1 less). Despite this loss of income, gross margins in the integrated system exceeded gross margins in the specialized system in 95% of years (Figure 2C). These gross margin surpluses in the integrated relative to the specialized system ranged from 29 to 589 LCU ha−1 yr−1.
Under historical climate conditions, the R-index indicated greater resilience of soybean yields in the integrated system in high precipitation years and comparable resilience between the integrated and specialized systems in low precipitation years (Table 2). However, the specialized system typically had higher soybean yields on average, with a small average yield advantage of 150 to 200 kg ha−1. This relationship changed only slightly under the future climate simulation, with the integrated system showing better yield resilience in both high and low precipitation years but the two systems performing relatively equally in terms of overall mean soybean yield.
Table 2. R-index and productivity indicators for historical and future climate scenarios simulated in APSIM for a Brazilian soybean-beef integrated system (grazed cover crop) and a specialized control system (ungrazed cover crop).
For historical cover crop aboveground biomass production, on the other hand, the integrated system had a better R-index under both high and low rainfall scenarios along with higher overall biomass production (14%; Table 2). Under the future climate scenario, resilience was higher for the specialized system in low precipitation years, but relative aboveground biomass productivity remained greater in the integrated system in both high and low precipitation years (21 and 11% more biomass, respectively).
R-Indices for gross margins indicated more resilience in the integrated system under all precipitation scenarios except when high or low rainfall occurred in both the summer and winter seasons (Table 2). Similarly, the integrated system had better mean gross margins overall in all scenarios except when low rainfall occurred in both summer and winter, with gross margins up to 15% higher than the specialized system in the low summer precipitation scenario. Under the future climate scenario, the integrated system had a better R-index in most precipitation scenarios. R-index was equal between the two systems in the low winter precipitation scenario. Overall gross margins paralleled these relationships, with the integrated system performing better in all scenarios for which data were available.
We used long-term historical and future simulations to examine the dynamics of agroecosystem performance and resilience in a commercial, ICLS with a grazed forage cover crop. We found that soybean yields tended to be slightly lower in the integrated system relative to the specialized, ungrazed cover crop reference system due to more frequent soil water stress, but that gross margins were sufficient to compensate for these yield penalties because the integrated system generated revenue from both crop and livestock production. These trends were largely upheld under both historical and future climate scenarios, but the probability of gross margin benefits in integrated systems increased from 77% of all simulated years to 95% of years under future climate. Results for field-level resilience, as indicated by the R-index for resistance and/or recovery, were more favorable for the integrated system but depended on the disturbance in question (low or high precipitation year). These results have direct implications for the suitability of ICLS, and specifically cover crop grazing, as a sustainable intensification strategy for southern Brazilian row crop and pasture systems.
Cover crop grazing in the integrated system resulted in minor yield penalties relative to the specialized system for soybean cash crops grown in rotation under both historical and future climate conditions. These penalties, ranging from a yield difference of −1,000 kg ha−1 in 2 years to a yield difference of < -400 kg ha−1 in the majority of years, were partly attributable to the greater risk of soil water stress in the integrated system. Lower soil water content in grazed cover crops is caused by (1) greater winter water demand from cover crop transpiration—grazed biomass must continually regrow to compensate for defoliation, therefore remaining green and actively transpiring longer into the season than ungrazed biomass (e.g., Geremia et al., 2019)—and (2) greater evaporative loss from the soil surface due to less residue cover in grazed compared with ungrazed cover crops (Peterson et al., 2019). However, this dynamic may not fully explain yield penalties in the integrated system, and past studies at this site have shown that observed soybean yields do not differ statistically from the specialized system even during severe drought years (Martins et al., 2016a). Other soil properties that differ between the integrated and specialized systems include aggregate structure (Conte et al., 2011), total and particulate organic carbon and nitrogen (Assmann et al., 2014), and pH and aluminum toxicity (Martins et al., 2014), among others. The dynamics of these properties are not fully captured by the model but could contribute to observed relative yield responses in this system.
Average integrated and specialized system soybean yields declined by 15% under the future climate scenario. Expected trajectories for soybean yields in response to climate change tend to increase globally but decrease in parts of the tropics and subtropics, including Brazil (Deryng et al., 2014). Model simulations have shown future soybean yields in subtropical South America varying from −16 to +3% without accounting for the effect of elevated atmospheric CO2, and up to +50% when accounting for elevated CO2 (Travasso et al., 2009). When not accounting for CO2 fertilization, other studies have predicted similar declines of up to 20% in Brazilian soybean yields under the RCP8.5 climate change scenario (Deryng et al., 2014). Although this negative effect could be mitigated by soybean yield gains from CO2 fertilization, other limiting factors such as temperature stress, soil nutrients, drought, pests, and weeds would likely interact to alter the impact of elevated CO2. For example, while a large yield boost has been predicted for soybean in response to elevated CO2, this response can be significantly curtailed in tropical and subtropical regions where high temperatures and faster crop maturation rates limit photosynthesis and light interception (Lin et al., 2020). As in other simulation studies of crop response to future climate, we elected to examine potential productivity changes in the absence of CO2 fertilization because the ability of crop and pasture models to simulate the effects of elevated CO2 and its interactions with these other limiting factors is uncertain (Soussana et al., 2010; Durand et al., 2018).
Soybean yield declines under the future climate scenario were linked to faster maturation times due to the extreme increases in temperature—and thus acceleration of soybean growth rates—predicted for this region of Brazil. Modifying the degree-day requirements of the cultivar mitigated this effect of degree day accumulation, suggesting that adoption of longer-season varieties or similar cultivar adjustment may be an important consideration for adaptation of Brazilian soybean production to future changes in climate. Other adaptation options may include adjustment of sowing/harvesting dates to account for changing crop phenology (Travasso et al., 2006). Some authors have also suggested increasing the diversity of crop rotations in the region to reduce the vulnerability of single-crop monocultures and alleviate the nutrient imbalances that often accompany large-scale soybean operations (Costamilan et al., 2012). This option includes crop-pasture rotations such as the cover crop grazing system examined here, warranting continued examination of the possible tradeoffs noted for these systems.
The magnitude of soybean yield penalties in the integrated relative to the specialized system was reduced under future climate conditions, in part due to increased summer precipitation that mitigated soil water limitations. Increases in summer precipitation could negatively impact soybean productivity if excess precipitation causes flooding and anoxic soil conditions or increases the prevalence of disease (Urban et al., 2015). However, these kinds of threats were not captured in the model. Given the conservativeness of the model, both potential future losses from oversaturated soils and potential gains from decreased frequency of soil water stress might have been underestimated. In any case, precipitation increases meant that soil water reductions brought on by winter cover crop grazing did not become a limiting factor for crop maturation, such that the tradeoff between winter forage and crop water utilization became inconsequential in the integrated system. This dynamic raises important questions regarding the feasibility of cover crop grazing systems in more water-limited environments. In semi-arid climates, for example, the tradeoffs in soil water usage among rotation components might be too costly to warrant the risk in an integrated system (Wunsch et al., 2017), although careful grazing management or the addition of irrigation could potentially avoid such tradeoffs (Whish et al., 2009; Sulc and Franzluebbers, 2014).
In contrast to the trend for soybean yields, cover crop aboveground biomass production in the integrated (grazed) system usually exceeded biomass production in the specialized (ungrazed) system under historical climate conditions. This result agrees with past observations at this site (Carvalho et al., 2018) and is consistent with compensatory growth behaviors of grazed ryegrass (Fulkerson and Donaghy, 2001). Relative forage production benefits persisted under future climate conditions and in both normal and anomalous (high or low) precipitation years.
The effects of climate change on tropical and subtropical pastures, annual forages, and grazing lands are highly uncertain, in part due to the complexity and diversity of these systems globally (Campbell and Stafford Smith, 2000). Our long-term simulations showed a considerable decline in average annual ryegrass productivity from the historical period to the near-future period (2020–2060), regardless of whether it was grazed. Similarly, Moore and Ghahramani (2013) demonstrated a 9% decline in annual net primary productivity of temperate grazing lands by 2030 using the GRAZPLAN model under the SRES A2 scenario in southern Australia. Similarly, we expect that the increases in winter temperatures projected for southern Brazil would be counterbalanced by increases in precipitation to mitigate cover crop water stress, but that faster maturation rates would result in an overall decline in cumulative end-of-season aboveground biomass production.
Elevated CO2 levels will likely further mitigate soil water stress by increasing water use efficiency, but other effects of elevated CO2 on annual forage production are uncertain. Interactions with temperature/rainfall variation, nutrient cycling, and shifts in growing season may limit many of the photosynthetic advantages of elevated CO2 (Ghahramani et al., 2019), while higher forage C:N ratios (Moore and Ghahramani, 2013) could decrease forage conversion efficiency, health, and productivity of grass-fed livestock (Ghahramani et al., 2019). Furthermore, animal LW gain is highly dependent on forage utilization, which is difficult to predict and can change in response to sward structure, composition, and palatability (Stejskalová and Hejcman, 2013). In the interest of minimizing confounding variables and simplifying our assessment of potential productivity outcomes in a complex system, we did not examine the effects of elevated CO2 levels on cover crop biomass accumulation and forage quality. Recognizing unknowns in forage utilization and grazing behavior, we instead used a conservative estimate of the forage conversion ratio to avoid overestimating animal production in the absence of elevated atmospheric CO2.
Average gross margins across systems declined by 18% under the future climate scenario, reflecting commensurate declines in both soybean and livestock revenue earning components. These results agree with some studies on the effects of global climate change on farm income and rural livelihoods, where models have predicted shrinking margins as a result of lower crop yields combined with increasing operation and input costs (Medellín-Azaura et al., 2011). In a simulation of an Australian mixed livestock-cropping system, Thamo et al. (2017) showed that most climate scenarios to 2050 resulted in decreased farm profitability, with profit margins more sensitive to climate conditions than crop or livestock productivity. Our simple field-level proxy suggests that an overall decline in farm profitability could also occur in subtropical beef–soybean systems, assuming constant prices and policy environments. However, favorable policy scenarios and price trends could result in improvements in farm livelihoods in other scenarios. It should be noted that our gross margins proxy is not intended to be a full bioeconomic assessment of farm-level incomes. Economic realities may differ from these proxy productivity indicators, as a poor yield could be offset by high prices in a given year or vice versa. In addition, this analysis reflects only the costs incurred at the field level without accounting for farm-wide expenses such as the purchase of steers for finishing—costs that are typically spread across multiple fields and seasons.
Nevertheless, the risk mitigation benefits of enterprise diversification would likely still hold true for integrated systems producing both livestock and crops (Thornton and Herrero, 2014). As Oliveira et al. (2013) found previously for this site, field-level productivity in the integrated system tended to exceed field-level productivity in the specialized system. The added animal production gleaned from winter grazing at this site tends to compensate for small losses in soybean yield and result in a more profitable system overall. ICLS have been shown to result in “income smoothing” for producers in both the small and large farm context—for example, even in extreme weather years when the crop component of the system fails outright, the livestock component may still produce revenues (Garrett et al., 2017). ICLS have also been shown to allow for more efficient allocation of resources and increased ability to manage spatial heterogeneity of the farm landscape (Bell and Moore, 2012), thus increasing adaptability in unfavorable years.
When livestock enterprise costs were held proportional to revenues, field-level gross margins in the integrated system were more likely to exceed gross margins in the specialized system under future climate conditions (77% of years under historical conditions, 95% of years under future conditions). Ryegrass appeared more sensitive to climate stress than soybeans, as gross margins for the livestock component of the integrated system declined twice as severely (28%) as profits for the soybean component (12–14%) in either system. Nevertheless, revenue from the livestock operation typically provided enough additional revenue both to compensate for small losses in soybean production and revenue and to outperform the specialized system, particularly under future climate conditions where both systems experienced declines in soybean yields.
Our post-hoc model of animal production is conservative with regard to forage dry matter conversion to LW, but does not account for potential changes in forage quality that might occur under future climatic conditions. In cool-season grasses, forage quality tends to decline with warming temperatures and increasing atmospheric CO2 concentrations (Izaurralde et al., 2011; Lee et al., 2017). Plant maturity is the major determining factor for nutritive quality, as more crude protein is provided by young green tissue (Nelson and Moser, 1994), so an increase in growth rates leading to faster maturation could also decrease nutritive value of forages through changes in structural composition of the pasture. These factors could negatively impact the productivity of the animal component of the integrated system and thus the outcome for field-level productivity.
Despite these potential risks for declining advantages from integrating livestock in the future, gross margins in the integrated system still exceeded gross margins in the specialized system in all but two of simulated years. When gross margins are interpreted as an indicator of field-level productivity, as intended here, this result indicates that the integrated system creates opportunities for more agricultural production from the same land area than the specialized system and provides a buffer from variability in soybean revenue. However, should adaptation measures be taken to offset the anticipated soybean productivity losses, the probability that the integrated system would outperform the specialized system would likely decrease. These results suggest that each component of an integrated system must be managed with care to obtain overall field-level benefits, underlining the complexity faced by managers of integrated systems.
The integrated system typically showed greater resilience than the specialized system in response to precipitation anomalies in the simulated historical and future weather. However, this was not true under all disturbance scenarios, and our approach reflects the value of accounting for context to identify cases where there is a tradeoff between greater resilience and field-level productivity or profitability. Greater resilience in either or all components of the system does not necessarily translate to greater productivity or maximum yield potential (Li et al., 2019) as seen under the high historical rainfall scenario, where soybean production in the integrated system was more resilient but the specialized system had a small relative yield advantage. Conversely, cover crop production in the integrated system was less resilient to low winter rainfall scenarios but still more productive on average than in the specialized system. These results illustrate the need to consider the objectives of a production agroecosystem—in this case, soybean and beef production—when appraising resilience. Because systems are not restricted to a single objective, environmental or cultural objectives could be held in equal weight to productivity, effectively reframing the assessment of resilience.
These results also reflect the challenge of directly characterizing resilience solely based on annual productivity trends, without fully considering how a system can rearrange or adapt its component parts when facing disturbances (Walker, 2020). These concepts have yet to be fully operationalized in agroecosystems research, especially when considering interactions that occur across system boundaries and temporal and spatial scales. Our approach provides a valuable quantitative assessment tool that can be complemented with other tools to develop more holistic assessments of social–ecological resilience.
For agricultural models in general, standards of model performance depend on the objectives of the study. For determining treatment effects, many authors consider models to be “acceptable” if estimates fall within 10% to 20% of observed means, or if model responses correctly track different trajectories among treatments (Ma et al., 2011). By either of these definitions, our model performance was acceptable for making estimates of soybean yield, cover crop aboveground biomass production, and for the soil-related functions for which the most reliable calibration data was available. The main limitation in the soybean simulation was the lack of a cultivar parameterization specific to cultivars grown in Brazil, which added to the uncertainty around this component of the simulation. However, the RMSE of ~600 kg ha−1 for soybean yield is comparable to errors predicted in previous simulation studies for a Brazilian soybean variety using APSIM and several other crop models (RMSEs of 535 to 650 kg ha−1; Battisti et al., 2017).
Model-estimated soil volumetric water content generally agreed with observed measurements from the 2016–2017 field season (Peterson et al., 2019), with some early- and late-season inaccuracies. Early-season disagreement in soil volumetric water content may have occurred due to the thick mats of residue present on the soil surface in this no-till system, especially in the specialized (ungrazed cover crop) system. While residue mats are captured by the model, potential related impacts such as lowered soil temperature and reduced evaporation from mats of this thickness may not have been. This aspect of the model could lead to underestimation of the time to emergence for seedlings and/or overestimation of the amount of soil water utilization by young plants or evaporation components of the water balance during these periods. Similarly, late-season disagreements may have occurred due to reduced reliability of field observations under dry soil conditions, when the granular matrix sensors used to detect soil water content tend to lose contact with the soil and cause observed readings to fluctuate.
Achieving a high level of agreement between observed and simulated cover crop and livestock production was more difficult due to the vagaries and uncertainties in both capturing all management dynamics and in the accurate measurement of such systems. Slight shifts in timing of simulated peak shoot biomass for the ungrazed cover crop and unrealistic precision of simulated biomass removal for the grazed cover crop decreased model performance, despite general agreement in magnitude and dynamics of aboveground biomass accumulation between observed and simulated time series. The greater variability of observed aboveground biomass quantities in the grazed cover crop can be attributed both to the finite intake capacity of livestock and to the put-and-take method of maintaining grass sward height. Biomass cutting rules implemented in the model precisely maintained sward heights of 20 to 30 cm (~2,500 kg DM ha−1), whereas some variability would be expected from sward height maintenance with put-and-take stocking. This idiosyncrasy, combined with the offset in timing of peak aboveground biomass in the specialized system, led to the higher RMSE and RRMSE for model predictions of cover crop aboveground biomass production.
APSIM performance for pastures and annual grasses is mixed, with outcomes dependent on initial soil water status, pasture type, region, and differences in transpiration and water use efficiency among cultivars (Pembleton et al., 2013; Ojeda et al., 2016). Lack of development for management scenarios such as annual forage-crop rotations and put-and-take stocking based on sward height targets limited our ability to exactly replicate the Brazilian soybean–beef integrated system. Such developments are ongoing for the APSIM AgPasture and AusFarm modules, with emphasis on grazing impacts such as dung and urine deposition and trampling on perennial mixed pasture productivity (Snow et al., 2014). Other simulation studies have modeled annual ryegrass growth dynamics in grazed pastures with reasonable accuracy (Cullen et al., 2008; Ojeda et al., 2016). However, there are few studies attempting model simulations of grazed annual ryegrass cover crops under climate change as we do here, especially in the subtropics (see Ghahramani and Moore, 2016 for an exception). In this study, calibration exercises with 15 years of observed data for the site indicated that the modeled values could account for more than 50% of the variation in observed cover crop forage production.
Using process-based model simulations to represent an annually grazed cover crop rotation with soybeans typical of southern Brazil, we showed that livestock integration with best management practices resulted in higher field-level productivity and resilience to chronic climate stress compared to a similar specialized system (no livestock integration). Winter grazing often resulted in yield penalties (up to 1,200 kg ha−1) for soybean in rotation, but this penalty was typically outweighed by the additional benefit generated by animal production. Field-level productivity (including income from both crop and livestock enterprises) was higher in the integrated system in 77% of years under historical climate conditions and in 95% of years under future climate conditions. While in many cases the multifunctionality of the integrated system was reflected in superior resilience to weather anomalies and to chronic climate stress under future conditions, outcomes were dependent on disturbance type. Nevertheless, these results highlight the importance of ICLS as an approach for sustainable intensification and agricultural adaptation to climate change. Future research should continue to calibrate and customize agricultural systems models for other types of integrated and diversified systems to determine if these results can be generalized to different environments and different management and adaptation scenarios. Further work to develop quantitative proxies for assessing biophysical resilience in agroecosystems would also increase our understanding of field-level outcomes and resilience building potential of ICLSs under future climate change.
The datasets presented in this study can be found in online repositories. The names of the repository/repositories and accession number(s) can be found at: https://doi.org/10.25338/B81918.
CP, LB, and AG contributed to the conception and design of the study. PC contributed to the experimental database. PC and LB contributed to interpretation of the results. CP performed the simulation analyses and wrote the first draft of the manuscript. All authors contributed to manuscript revision and read and approved of the final manuscript.
Funding for this work was provided by the NSF Graduate Research Fellowship Program fellowship and NSF-CAPES Graduate Research Opportunities Worldwide fellowship to CP (#1650042, P. Mohapatra), the UC Davis Graduate Group in Ecology Henry A. Jastro Research Fellowship to CP (#36327), the Australian Academy of Sciences Australia-Americas Ph.D. Research Internship to CP, and the USDA National Institute of Food and Agriculture Agricultural Experiment Station Hatch Projects to AG (project CA-D-PLS-2332-352H).
The authors declare that the research was conducted in the absence of any commercial or financial relationships that could be construed as a potential conflict of interest.
The authors wish to thank the team at the Agricultural Production Systems Research Unit of CSIRO in Toowoomba, QLD, for their support with the APSIM software framework, A. Moore and D. Thomas (CSIRO) for their valuable feedback on the manuscript draft, as well as the members of the Grazing Ecology Research Group at the Federal University of Rio Grande do Sul for valuable assistance in the field and laboratory.
The Supplementary Material for this article can be found online at: https://www.frontiersin.org/articles/10.3389/fsufs.2020.604099/full#supplementary-material
Archontoulis, S. V., Miguez, F. E., and Moore, K. J. (2014). Evaluating APSIM maize, soil water, soil nitrogen, manure, and soil temperature modules in the Midwestern United States. Agron. J. 106, 1025–1040. doi: 10.2134/agronj2013.0421
Assmann, J. M., Anghinoni, I., Martins, A. P., Costa, S. E. V. G. A., Cecagno, D., Carlos, F. S., et al. (2014). Soil carbon and nitrogen stocks and fractions in a long-term integrated crop-livestock system under no-tillage in southern Brazil. Agric. Ecosyst. Environ. 190, 52–59. doi: 10.1016/j.agee.2013.12.003
Assmann, J. M., Anghinoni, I., Martins, A. P., Costa, S. E. V. G. A., Kunrath, T. R., Bayer, C., et al. (2015). Carbon and nitrogen cycling in an integrated soybean-beef cattle production system under different grazing intensities. Pesqui. Agropecu. Bras. 50, 967–978. doi: 10.1590/S0100-204X2015001000013
Balandier, P., Bergez, J., and Etienne, M. (2003). Use of the management-oriented silvopastoral model ALWAYS: calibration and evaluation. Agrofor. Syst. 57, 159–172 doi: 10.1023/A:1024863408559
Battisti, R., Sentelhas, P. C., and Boote, K. J. (2017). Inter-comparison of performance of soybean crop simulation models and their ensemble in southern Brazil. F Crop Res. 200, 28–37. doi: 10.1016/j.fcr.2016.10.004
Bell, L. W., Kirkegaard, J. A., Swan, A., Hunt, J. R., Huth, N. I., and Fettell, N. A. (2011). Impacts of soil damage by grazing livestock on crop productivity. Soil Tillage Res. 113, 19–29. doi: 10.1016/j.still.2011.02.003
Bell, L. W., and Moore, A. D. (2012). Livestock systems in Australian agriculture: trends, drivers and implications. Agric. Syst. 111, 1–12. doi: 10.1016/j.agsy.2012.04.003
Bell, L. W., Moore, A. D., and Kirkegaard, J. A. (2014). Evolution in crop-livestock integration systems that improve farm productivity and environmental performance in Australia. Eur. J. Agron. 57, 10–20. doi: 10.1016/j.eja.2013.04.007
Bowles, T. M., Mooshammer, M., Socolar, Y., Calderón, F., Cavigelli, M. A., Culman, S. W., et al. (2020). Long-term evidence shows that crop-rotation diversification increases agricultural resilience to adverse growing conditions in North America. One Earth 2, 284–293. doi: 10.1016/j.oneear.2020.02.007
Caetano, L. A. M. (2017). Impacto da intensidade de pastejo na produtividade da soja em integração com bovinos de corte (Master's thesis). Universidade Federal do Rio Grande do Sul, Porto Alegre, Brazil.
Campbell, B. D., and Stafford Smith, D. M. (2000). A synthesis of recent global change research on pasture and rangeland production: reduced uncertainties and their management implications. Agric. Ecosyst. Environ. 82, 39–55 doi: 10.1016/S0167-8809(00)00215-2
Carvalho, PCF, Peterson, C. A., Nunes, P. A. A., Martins, A. P., de Souza Filho, W., Bertolazi, V. T., et al. (2018). Animal production and soil characteristics from integrated crop-livestock systems: toward sustainable intensification. J. Anim. Sci. 96, 3513–3525. doi: 10.1093/jas/sky085
Cecagno, D., Costa, S. E. V. G. A., Anghinoni, I., Kunrath, T. R., Martins, A. P., Reichert, J. M., et al. (2016). Least limiting water range and soybean yield in a long-term, no-till, integrated crop-livestock system under different grazing intensities. Soil Tillage Res. 156, 54–62. doi: 10.1016/j.still.2015.10.005
Chou, S. C., Lyra, A., Mourão, C., Dereczynski, C., Pilotto, I., Gomes, J., et al. (2014). Assessment of climate change over South America under RCP 4.5 and 8.5 downscaling scenarios. Am. J. Clim. Chang. 3, 512–527. doi: 10.4236/ajcc.2014.35043
Conte, O., Flores, J. P. C., Cassol, L. C., Anghinoni, I., Carvalho, P. C. F, Levien, R., et al. (2011). Evolução de atributos físicos de solo em sistema de integração lavoura-pecuária [Evolution of soil physical attributes in an integrated crop-livestock system]. Pesqui. Agropecu. Bras. 46, 1301–1309. doi: 10.1590/S0100-204X2011001000026
Conterato, I. F., David, D. B., Da Trindade, J. K., Maldaner, J., and Bremm, C. (2016). Comportamento agronômico de azevém anual (Lolium multiflorum L.) no estado do Rio Grande do Sul. Bol. Indústria Anim. 73, 198–205. doi: 10.17523/bia.v73n3p198
Conway, G. R. (1986). Agroecosystem Analysis for Research and Development. Bangkok: Winrock International.
Costamilan, L. M., Carrão-Panizzi, M. C., Strieder, M. L., and Bertagnolli, P. F. (2012). Indicações Técnicas Para a Cultura da soja no Rio Grande do Sul e em Santa Catarina, Safras 2012/2013 e 2013/2014. Passo Fundo: Embrapa Trigo.
Cullen, B. R., Eckard, R. J., Callow, M. N., Johnson, I. R., Chapman, D. F., Rawnsley, R. P., et al. (2008). Simulating pasture growth rates in Australian and New Zealand grazing systems. Aust. J. Agric. Res. 59, 761–768. doi: 10.1071/AR07371
da Silva, F. D., Amado, T. J. C., Bredemeier, C., Bremm, C., Anghinoni, I., and Carvalho, P. C. F. (2014). Pasture grazing intensity and presence or absence of cattle dung input and its relationships to soybean nutrition and yield in integrated crop-livestock systems under no-till. Eur. J. Agron. 57, 84–91. doi: 10.1016/j.eja.2013.10.009
Dalgliesh, N., Hochman, Z., Huth, N., and Holzworth, D. (2016). Field Protocol to APSoil Characterisations. CSIRO.
de Souza Filho, W., Nunes, P. A. A., Barro, R. S., Kunrath, T. R., Menezes de Almeida, G., Genro, T. C. M., et al. (2019). Mitigation of enteric methane emissions through pasture management in integrated crop-livestock systems: trade-offs between animal performance and environmental impacts. J. Clean. Prod. 213, 968–975. doi: 10.1016/j.jclepro.2018.12.245
Deiss, L., Kleina, G. B., Moraes, A., Franzluebbers, A. J., Motta, A. C. V., Dieckow, J., et al. (2019). Soil chemical properties under no-tillage as affected by agricultural trophic complexity. Eur. J. Soil Sci. 2019, 1–16. doi: 10.1111/ejss.12869
Deryng, D., Conway, D., Ramankutty, N., Price, J., and Warren, R. (2014). Global crop yield response to extreme heat stress under multiple climate change futures. Environ. Res. Lett. 9:034011. doi: 10.1088/1748-9326/9/3/034011
Durand, J. L., Delusca, K., Boote, K., Lizaso, J., Manderscheid, R., Weigel, H. J., et al. (2018). How accurately do maize crop models simulate the interactions of atmospheric CO2 concentration levels with limited water supply on water use and yield? Eur. J. Agron. 100, 67–75. doi: 10.1016/j.eja.2017.01.002
Food and Agriculture Organization of the United Nations (2018). FAOSTAT Statistical Database. Rome: FAO.
Fulkerson, W. J., and Donaghy, D. J. (2001). Plant-soluble carbohydrate reserves and senescence - key criteria for developing an effective grazing management system for ryegrass-based pastures: a review. Aust J Exp Agric 41, 261–275. doi: 10.1071/EA00062
Garrett, R. D., Niles, M. T., Gil, J. D. B., Gaudin, A., Chaplin-Kramer, R., Assmann, A., et al. (2017). Social and ecological analysis of commercial integrated crop livestock systems: current knowledge and remaining uncertainty. Agric. Syst. 155, 136–146. doi: 10.1016/j.agsy.2017.05.003
Garrett, R. D., Ryschawy, J., Bell, L. W., Cortner, O., Ferreira, J., Garik, A. V. N., et al. (2020). Drivers of decoupling and recoupling of crop and livestock systems at farm and territorial scales. Ecol. Soc. 25:24. doi: 10.5751/ES-11412-250124
Geremia, C., Merkle, J. A., Eacker, D. R., Wallen, R. L., White, P. J., Hebblewhite, M., et al. (2019). Migrating bison engineer the green wave. Proc. Natl. Acad. Sci. U.S.A. 116, 25707–25713. doi: 10.1073/pnas.1913783116
Ghahramani, A., Howden, S. M., del Prado, A., Thomas, D. T., Moore, A. D., Ji, B., et al. (2019). Climate change impact, adaptation, and mitigation in temperate grazing systems: a review. Sustain 11, 1–30. doi: 10.3390/su11247224
Ghahramani, A., and Moore, A. D. (2016). Impact of climate changes on existing crop-livestock farming systems. Agric. Syst. 146, 142–155. doi: 10.1016/j.agsy.2016.05.011
Gil, J., Garrett, R., and Berger, T. (2016). Determinants of crop-livestock integration in Brazil: evidence from the household and regional levels. Land Use Policy 59, 557–568. doi: 10.1016/j.landusepol.2016.09.022
Gil, J. D. B., Cohn, A. S., Duncan, J., Newton, P., and Vermeulen, S. (2017). The resilience of integrated agricultural systems to climate change. Wiley Interdiscip. Rev. Clim. Chang. 8:e461. doi: 10.1002/wcc.461
Groot, J. C. J., Cortez-Arriola, J., Rossing, W. A. H., Massiotti, R. D. A., and Tittonell, P. (2016). Capturing agroecosystem vulnerability and resilience. Sustain 8:1206. doi: 10.3390/su8111206
Gudmundsson, L. (2016). qmap: Statistical Transformations for Post-Processing Climate Model Output. R package version 1.0–4
Gudmundsson, L., Bremnes, J. B., Haugen, J. E., and Engen-Skaugen, T. (2012). Technical note: downscaling RCM precipitation to the station scale using statistical transformations - a comparison of methods. Hydrol. Earth Syst. Sci. 16, 3383–3390. doi: 10.5194/hess-16-3383-2012
Holzworth, D. P., Huth, N. I., and DeVoil, P. G. (2011). Simple software processes and tests improve the reliability and usefulness of a model. Environ. Model Softw. 26, 510–516. doi: 10.1016/j.envsoft.2010.10.014
Holzworth, D. P., Huth, N. I., deVoil, P. G., Zurcher, E. J., Herrmann, N. I., McLean, G., et al. (2014). APSIM - evolution towards a new generation of agricultural systems simulation. Environ. Model Softw. 62, 327–350. doi: 10.1016/j.envsoft.2014.07.009
Izaurralde, R. C., Thomson, A. M., Morgan, J. A., Fay, P. A., Polley, W., and Hatfield, J. L. (2011). Climate impacts on agriculture: implications for forage and rangeland production. Agron. J. 103, 371–381. doi: 10.2134/agronj2010.0304
Kahiluoto, H., Rötter, R., Webber, H., and Ewert, F. (2014). “The role of modelling in adapting and building the climate resilience of cropping systems,” in Climate Change Impact and Adaptation in Agricultural Systems, eds J. Fuhrer and P. J. Gregory (Wallingford, CT: CAB International), 204–215.
Kunrath, T. R., Carvalho PC de, F., Cadenazzi, M., Bredemeier, C., and Anghinoni, I. (2015). Grazing management in an integrated crop-livestock system: soybean development and grain yield. Rev. Cienc. Agron. 46, 645–653. doi: 10.5935/1806-6690.20150049
Kunrath, T. R., Nunes, P. A. A., de Souza Filho, W., Cadenazzi, M., Bremm, C., Martins, A. P., et al. (2020). Sward height determines pasture production and animal performance in a long-term soybean-beef cattle integrated system. Agric. Syst. 177:102716. doi: 10.1016/j.agsy.2019.102716
Lee, M. A., Davis, A. P., Chagunda, M. G. G., and Manning, P. (2017). Forage quality declines with rising temperatures, with implications for livestock production and methane emissions. Biogeosciences 14, 1403–1417. doi: 10.5194/bg-14-1403-2017
Li, M., Peterson, C. A., Tautges, N. E., Scow, K. M., and Gaudin, A. C. M. (2019). Yields and resilience outcomes of organic, cover crop, and conventional practices in a Mediterranean climate. Sci. Rep 9, 1–11. doi: 10.1038/s41598-019-48747-4
Lilley, J. M., Bell, L. W., and Kirkegaard, J. A. (2015). Optimising grain yield and grazing potential of crops across Australia's high-rainfall zone: a simulation analysis. Canola. Crop Pasture Sci. 66, 349–364. doi: 10.1071/CP14240
Lin, B. B. (2011). Resilience in agriculture through crop diversification: adaptive management for environmental change. Bioscience 61, 183–193. doi: 10.1525/bio.2011.61.3.4
Lin, T.-S., Song, Y., Jain, A. K., Lawrence, P., and Kheshgi, H. S. (2020) Effects of environmental management factors on worldwide maize soybean yields over the 20th 21st centuries, Biogeosciences Discuss. (in review). doi: 10.5194/bg-2020-68
Ma, L., Ahuja, L. R., Saseendran, S. A., Malone, R. W., Green, T. R., Nolan, B. T., et al. (2011). “A protocol for parameterization and calibration of RZWQM2 in field research,” in Methods of Introducing System Models Into Agricultural Research, eds L. Ahuja, L. Ma (Madison, WI: ASA, CSSA, SSSA). p.1–64.
Makkonen, L. (2006). Plotting positions in extreme value analysis. J. Appl. Meteorol. Climatol. 45, 334–340. doi: 10.1175/JAM2349.1
Marchão, R. L., Lavelle, P., Celini, L., Balbino, L. C., Vilela, L., and Becquer, T. (2009). Soil macrofauna under integrated crop livestock systems in a Brazilian Cerrado Ferralsol. Pesqui Agropecuár. Bras. 44, 1011–1020 doi: 10.1590/S0100-204X2009000800033
Marengo, J. A., Jones, R., Alves, L. M., and Valverde, M. C. (2009). Future change of temperature and precipitation extremes in South America as derived from the PRECIS regional climate modeling systems. Int. J. Climatol. 29, 2241–2255. doi: 10.1002/joc.1863
Martins, A. P., Cecagno, D., Borin, J. B. M., Arnuti, F., Lochmann, S. H., Anghinoni, I., et al. (2016b). Long-, medium- and short-term dynamics of soil acidity in an integrated crop–livestock system under different grazing intensities. Nutr. Cycl. Agroecosyst. 104, 67–77. doi: 10.1007/s10705-015-9759-5
Martins, A. P., Costa, S. E. V. G. A., Anghinoni, I., Kunrath, T. R., Balerini, F., Cecagno, D., et al. (2014). Soil acidification and basic cation use efficiency in an integrated no-till crop-livestock system under different grazing intensities. Agric. Ecosyst. Environ. 195, 18–28. doi: 10.1016/j.agee.2014.05.012
Martins, A. P., Costa, S. E. V. G. A., Anghinoni, I., Kunrath, T. R., Cecagno, D., Reichert, J. M., et al. (2016a). Soil moisture and soybean physiology affected by drought in an integrated crop-livestock system. Pesqui. Agropecu. Bras. 51, 978–989. doi: 10.1590/S0100-204X2016000800010
Medellín-Azaura, J., Howitt, R. E., MacEwan, D. J., and Lund, J. (2011). Economic impacts of climate-related changes to California agriculture. Clim. Change. 109, S387–S405. doi: 10.1007/s10584-011-0314-3
Moore, A. D. (2009). Opportunities and trade-offs in dual-purpose cereals across the southern Australian mixed-farming zone: a modelling study. Anim. Prod. Sci. 49, 759–768. doi: 10.1071/AN09006
Moore, A. D., Donnelly, J. R., and Freer, M. (1997). GRAZPLAN: decision support systems for Australian grazing enterprises. III. Pasture growth and soil moisture submodels, and the GrassGro DSS. Agric. Syst. 55, 535–582. doi: 10.1016/S0308-521X(97)00023-1
Moore, A. D., and Ghahramani, A. (2013). Climate change and broadacre livestock production across southern Australia. 1. Impacts of climate change on pasture and livestock productivity, and on sustainable levels of profitability. Glob. Chang. Biol. 19, 1440–1455. doi: 10.1111/gcb.12150
Moraes, A., de Carvalho, PC de F., Anghinoni, I., Lustosa, S. B. C., Costa, S. E. V. G. A., and Kunrath, T. R. (2014). Integrated crop–livestock systems in the Brazilian subtropics. Eur. J. Agron. 57, 4–9. doi: 10.1016/j.eja.2013.10.004
Moriasi, D. N., Van Liew, M. W., Bingner, R. L., Harmel, R. D., and Veith, T. L. (2007). Model evaluation guidelines for systematic quantification of accuracy in watershed simulations. Trans ASABE 50, 885–900 doi: 10.13031/2013.23153
Mott, G. O., and Lucas, H. L. (1952). “The design, conduct, and interpretation of grazing trials on cultivated and improved pastures,” in Proceedings of the 6th International Grassland Congress (State College, PA), 1380–1385.
Navarro, C. E., and Tarapues, J. E. (2015). Bias-Correction in the CCAFS-Climate Portal: A Description of Methodologies. Cali: CCAFS.
Naylor, R., Steinfeld, H., Falcon, W., Galloway, J., Smil, V., Bradford, E., et al. (2005). Losing the links between livestock and land. Science 310, 1621–1622. doi: 10.1126/science.1117856
Nelson, C. J., and Moser, L. E. (1994). “Plant factors affecting forage quality,” in Forage Quality, Evaluation, and Utilization, ed G. C. Fahey (Madison, WI: American Society of Agronomy, Crop Science Society of America, Soil Science Society of America), 115–154.
NRC (2016). Nutrient Requirements of Beef Cattle, 8th revision. Washington, DC: The National Academy Press.
Nunes, P. A. A., Bredemeier, C., Bremm, C., Caetano, L. A. M., Menezes de Almeida, G., de Souza Filho, W., et al. (2018). Grazing intensity determines pasture spatial heterogeneity and productivity in an integrated crop-livestock system. Grassl. Sci. 2018, 1–11. doi: 10.1111/grs.12209
Ojeda, J. J., Pembleton, K. G., Islam, M. R., Agnusdei, M. G., and Garcia, S. C. (2016). Evaluation of the agricultural production systems simulator simulating Lucerne and annual ryegrass dry matter yield in the Argentine Pampas and south-eastern Australia. Agric. Syst. 143, 61–75. doi: 10.1016/j.agsy.2015.12.005
Oliveira, C. A., Bremm, C., Anghinoni, I., de Moraes, A., Kunrath, T. R., and Carvalho, P. C. F. (2013). Comparison of an integrated crop–livestock system with soybean only: Economic and production responses in southern Brazil. Renew. Agric. Food Syst. 28, 1–9. doi: 10.1017/S1742170513000410
Pembleton, K. G., Rawnsley, R. P., Jacobs, J. L., Mickan, F. J., O'Brien, G. N., Cullen, B. R., et al. (2013). Evaluating the accuracy of the Agricultural Production Systems Simulator (APSIM) simulating growth, development, and herbage nutritive characteristics of forage crops grown in the south-eastern dairy regions of Australia. Crop Pasture Sci. 64, 147–164. doi: 10.1071/CP12372
Peterson, C. A., Deiss, L., and Gaudin, A. C. M. (2020). Integrated Crop-Livestock Systems Achieve Comparable Crop Yields to Specialized Systems: A Meta-Analysis. PLoS ONE 15:e0231840. doi: 10.1371/journal.pone.0231840
Peterson, C. A., Eviner, V. T., and Gaudin, A. C. M. (2018). Ways forward for resilience research in agroecosystems. Agric. Syst. 162, 19–27. doi: 10.1016/j.agsy.2018.01.011
Peterson, C. A., Nunes, P. A. A., Martins, A. P., Bergamaschi, H., Anghinoni, I., Carvalho, P. C. F., et al. (2019). Winter grazing does not affect soybean yield despite lower soil water content in a subtropical crop-livestock system. Agron. Sustain. Dev. 39:26. doi: 10.1007/s13593-019-0573-3
Probert, M. E., Dimes, J. P., Keating, B. A., Dalal, R. C., and Strong, W. M. (1998). APSIM's water and nitrogen modules and simulation of the dynamics of water and nitrogen in fallow systems. Agric. Syst. 56, 1–28. doi: 10.1016/S0308-521X(97)00028-0
R Core Team (2018). R: A Language and Environment for Statistical Computing. R Foundation for Statistical Computing, Vienna.
Renard, D., and Tilman, D. (2019). National food production stabilized by crop diversity. Nature 571, 257–260. doi: 10.1038/s41586-019-1316-y
Riahi, K., Rao, S., Krey, V., Cho, C., Chirkov, V., Fischer, G., et al. (2011). RCP 8.5 - a scenario of comparatively high greenhouse gas emissions. Clim. Change 109, 33–57. doi: 10.1007/s10584-011-0149-y
Rist, L., Felton, A., Nyström, M., and Troell, M. (2014). Applying resilience thinking to production ecosystems. Ecosphere 5, 1–11. doi: 10.1890/ES13-00330.1
Robertson, M., Bathgate, A., Moore, A., Lawes, R., and Lilley, J. (2009). Seeking simultaneous improvements in farm profit and natural resource indicators: a modelling analysis. Anim. Prod. Sci. 49, 826–836. doi: 10.1071/AN09008
Robertson, M. J., Carberry, P. S., Huth, N. I., Turpin, J. E., Probert, M. E., Poulton, P. L., et al. (2002). Simulation of growth and development of diverse legume species in APSIM. Aust. J. Agric. Resour. Econ. 53, 429–446. doi: 10.1071/AR01106
Sanderson, M. A., Archer, D., Hendrickson, J., Kronberg, S., Liebig, M., Nichols, K., et al. (2013). Diversification and ecosystem services for conservation agriculture: outcomes from pastures and integrated crop–livestock systems. Renew. Agric. Food Syst. 28, 129–144. doi: 10.1017/S1742170512000312
Sentelhas, P. C., Battisti, R., Câmara, G. M. S., Farias, J. R. B., Hampf, A. C., and Nendel, C. (2015). The soybean yield gap in Brazil - magnitude, causes and possible solutions for sustainable production. J. Agric. Sci. 153, 1394–1411. doi: 10.1017/S0021859615000313
Sheffield, J., Goteti, G., and Wood, E. F. (2006). Global meteorological forcing dataset for land surface modeling. J. Clim. 19, 3088–3111 doi: 10.1175/JCLI3790.1
Silva, I. R., Smyth, T. J., Raper, C. D., Carter, T. E., and Rufty, T. W. (2001). Differential aluminum tolerance in soybean: an evaluation of the role of organic acids. Physiol. Plant 112, 200–210. doi: 10.1034/j.1399-3054.2001.1120208.x
Snow, V. O., Rotz, C. A., Moore, A. D., Martin-Clouaire, R., Johnson, I. R., Hutchings, N. J., et al. (2014). The challenges - and some solutions - to process-based modelling of grazed agricultural systems. Environ. Model Softw. 62, 420–436. doi: 10.1016/j.envsoft.2014.03.009
Soussana, J. F., Graux, A. I., and Tubiello, F. N. (2010). Improving the use of modelling for projections of climate change impacts on crops and pastures. J. Exp. Bot. 61, 2217–2228. doi: 10.1093/jxb/erq100
Spiegal, S., Bestelmeyer, B. T., Archer, D. W., Augustine, D. J., Boughton, E. H., Boughton, R. K., et al. (2018). Evaluating strategies for sustainable intensification of US agriculture through the Long-Term Agroecosystem Research network. Environ. Res. Lett. 13:034031. doi: 10.1088/1748-9326/aaa779
Standish, R. J., Hobbs, R. J., Mayfield, M. M., Bestelmeyer, B. T., Suding, K. N., Battaglia, L. L., et al. (2014). Resilience in ecology: abstraction, distraction, or where the action is? Biol. Conserv. 177, 43–51. doi: 10.1016/j.biocon.2014.06.008
Stejskalová, M, Hejcmanová, P, Pavlu, V, and Hejcman, M. (2013). Grazing behavior and performance of beef cattle as a function of sward structure and herbage quality under rotational and continuous stocking on species-rich upland pasture. Anim. Sci. J. 84, 622–629. doi: 10.1111/asj.12048
Sulc, R. M., and Franzluebbers, A. J. (2014). Exploring integrated crop-livestock systems in different ecoregions of the United States. Eur. J. Agron. 57, 21–30. doi: 10.1016/j.eja.2013.10.007
Szymczak, L. S., Carvalho PC de, F., Lurette, A., de Moraes, A., Nunes, P. A. A, Martins, A. P., et al. (2020). System diversification and grazing management as resilience-enhancing agricultural practices: the case of crop-livestock integration. Agric. Syst. 184:102904. doi: 10.1016/j.agsy.2020.102904
Taylor, K. E., Stouffer, R. J., and Meehl, G. A. (2012). An overview of CMIP5 and experimental design. Bull. Am. Meteorol. Soc. 93, 485–498. doi: 10.1175/BAMS-D-11-00094.1
Thamo, T., Addai, D., Pannell, D. J., Robertson, M. J., Thomas, D. T., and Young, J. M. (2017). Climate change impacts and farm-level adaptation: economic analysis of a mixed cropping–livestock system. Agric. Syst. 150, 99–108. doi: 10.1016/j.agsy.2016.10.013
Thomas, D. T., Finlayson, J., Moore, A. D., and Robertson, M. J. (2010). Profitability of grazing crop stubbles may be overestimated by using the metabolisable energy intake from the stubble. Anim. Prod. Sci. 50, 699–704. doi: 10.1071/AN09213
Thornton, P. K., and Herrero, M. (2014). Climate change adaptation in mixed crop-livestock systems in developing countries. Glob. Food Sec. 3, 99–107. doi: 10.1016/j.gfs.2014.02.002
Tracy, B. F., and Zhang, Y. (2008). Soil compaction, corn yield response, and soil nutrient pool dynamics within an integrated crop – livestock system in Illinois. Crop Sci. 48, 1211–1218. doi: 10.2135/cropsci2007.07.0390
Travasso, M. I., Magrin, G., Rodríguez, G., and López, G. (2009). Potential impacts of climate change on soybean yields in the Argentinean pampas and adaptation measures for future sustainable production. IOP Conf. Ser. Earth Environ. Sci. 6:372045. doi: 10.1088/1755-1307/6/37/372045
Travasso, M. I., Magrin, G. O., Baethgen, W. E., Castaño, J. E., Rodriguez, G. R., Pires, J. L., et al. (2006). Adaptation Measures for Maize and Soybean in Southeastern South America. Washington, DC: AIACC.
Urban, D. W., Roberts, M. J., Schlenker, W., and Lobell, D. B. (2015). The effects of extremely wet planting conditions on maize and soybean yields. Clim. Change 130, 247–260. doi: 10.1007/s10584-015-1362-x
Walker, B. H. (2020). Resilience: what it is and is not. Ecol. Soc. 25, 25–27. doi: 10.5751/ES-11647-250211
Wallach, D., Makowski, D., Jones, J. W., and Brun, F. (eds.). (2014). “Model evaluation,” in Working With Dynamic Crop Models, eds D. Wallach, D. Makowski, J. W. Jones, and F. Brun (Amsterdam: Elsevier), 345–406.
Whish, J. P. M., Price, L., and Castor, P. A. (2009). Do spring cover crops rob water and so reduce wheat yields in the northern grain zone of eastern Australia? Crop Pasture Sci. 60, 517–525. doi: 10.1071/CP08397
Wilkinson, J. M. (2011). Re-defining efficiency of feed use by livestock. Animal 5, 1014–1022. doi: 10.1017/S175173111100005X
Wilson, C. H., Strickland, M. S., Hutchings, J. A., Bianchi, T. S., and Flory, S. L. (2018). Grazing enhances belowground carbon allocation, microbial biomass, and soil carbon in a subtropical grassland. Glob. Chang. Biol. 24, 2997–3009. doi: 10.1111/gcb.14070
Wunsch, E. M., Bell, L. W., and Bell, M. J. (2017). Can legumes provide greater benefits than millet as a spring cover crop in southern Queensland farming systems? Crop Pasture Sci. 68:746. doi: 10.1071/CP17223
Keywords: cropping systems, resilience, climate change, cover crop grazing, APSIM, soybean, pasture
Citation: Peterson CA, Bell LW, Carvalho PCdF and Gaudin ACM (2020) Resilience of an Integrated Crop–Livestock System to Climate Change: A Simulation Analysis of Cover Crop Grazing in Southern Brazil. Front. Sustain. Food Syst. 4:604099. doi: 10.3389/fsufs.2020.604099
Received: 08 September 2020; Accepted: 16 October 2020;
Published: 25 November 2020.
Edited by:
Johann G. Zaller, University of Natural Resources and Life Sciences Vienna, AustriaReviewed by:
Edward James Raynor, United States Department of Agriculture, United StatesCopyright © 2020 Peterson, Bell, Carvalho and Gaudin. This is an open-access article distributed under the terms of the Creative Commons Attribution License (CC BY). The use, distribution or reproduction in other forums is permitted, provided the original author(s) and the copyright owner(s) are credited and that the original publication in this journal is cited, in accordance with accepted academic practice. No use, distribution or reproduction is permitted which does not comply with these terms.
*Correspondence: Amélie C. M. Gaudin, YWdhdWRpbkB1Y2RhdmlzLmVkdQ==
Disclaimer: All claims expressed in this article are solely those of the authors and do not necessarily represent those of their affiliated organizations, or those of the publisher, the editors and the reviewers. Any product that may be evaluated in this article or claim that may be made by its manufacturer is not guaranteed or endorsed by the publisher.
Research integrity at Frontiers
Learn more about the work of our research integrity team to safeguard the quality of each article we publish.