- 1Centre d'étude de la forêt, Centre Interdisciplinaire en Modélisation Mathématique de l'Université Laval, Québec, QC, Canada
- 2Department of Biology, Université Laval, Québec, QC, Canada
- 3School of Natural Resource Management, Nelson Mandela University, George Campus, George, South Africa
- 4Groupe Interdisciplinaire de Recherche en Éléments Finis, Centre Interdisciplinaire en Modélisation Mathématique de l'Université Laval, Centre d'étude de la forêt, Québec, QC, Canada
- 5Department of Mathematics and Statistics, Université Laval, Québec, QC, Canada
- 6REHABS International Research Laboratory, Centre national de la recherche scientifique-Université Lyon 1-Nelson Mandela University, George Campus, George, South Africa
- 7Department of Biology, University of Saskatchewan, Saskatoon, SK, Canada
- 8UMR CNRS 6554 LETG-Angers, UFR Sciences, University of Angers, Angers, France
Understanding, predicting and controlling animal movement is a fundamental problem of conservation and management ecology. The need to mitigate human-wildlife conflicts, such as crop raiding by large herbivores, is becoming increasingly urgent. Because of the substantial costs or the possibility of unsuitable outcomes on wildlife, managers are often encouraged to deploy interventions that can achieve their objective while minimizing the impact on animal populations. We propose an adaptive management framework that can identify cost-effective solutions to reduce human-wildlife conflicts, while also minimizing constraints on animal movement and distribution. We focus on conflicts involving animals for which conflict zones occupy only a portion of their home-range. The adaptive management approach includes four basic steps: define and spatialize conflict areas, predict animal distribution from functional connectivity and patch residency time, predict the impact of management actions on animal distribution, and test predictions and revise predictive models. Key to the process is development of a mathematical model that can predict how habitat-animal interactions shape animal movement dynamics within patch networks. In our model, networks consist of a set of high-quality patches connected by links (i.e., potential inter-patch movements). Inter-patch movement rules and determinants of patch residency time need to be determined empirically. These data then provide information to parameterize a reaction-advection-diffusion model that can predict animal distribution dynamics given habitat features and movement taxis toward (or against) conflict areas depending on management actions. Illustrative simulations demonstrate how quantitative predictions can be used to make spatial adjustments in management interventions (e.g., length of diversionary fences) with respect of conflict areas. Simulations also show that the impact of multiple interventions cannot be considered as simply having additive effect, and their relative impact on animal equilibrium distribution depends on how they are added and deployed across the network. Following the principles of adaptive, integrated landscape management, the predictive model should be revised as monitoring provides new information about the response of animals to the set of interventions. We contend that the proposed quantitative approach provides a robust framework to find cost-effective strategy toward sustainable human-wildlife conflicts.
Introduction
Increased rates of extinction of wildlife populations in association with human activity is the hallmark of the Anthropocene (Pereira et al., 2010; Richardson et al., 2020). Humans greatly impact wildlife by disrupting the distribution and behavior of animals globally (Gaynor et al., 2018; Tucker et al., 2018), and human footprint on the landscape is a key threat to wildlife across virtually all taxonomic groups (Turvey and Crees, 2019). The relation between wildlife and the available space is paramount for conservation (Rosenzweig and Ziv, 1999). Despite Aichi targets (CBD, 2018) aiming at increasing the amount of protected areas, a major the debate concerns how to allow biodiversity in human-dominated landscapes, such as land-sharing strategies (Green et al., 2005). Sharing space as a conservation model is contentious, however, as human-wildlife conflicts (HWC) are accelerating (Dickman, 2010). The International Union for the Conservation of Nature and the World Wildlife Fund consider HWC to be one of the main threats to biodiversity worldwide (IUCN, 2020; WWF, 2020).
These HWC, however, often stem from conflicts between humans about how to manage wildlife in shared landscapes (Peterson et al., 2010; Frank, 2016), and there is increasing recognition that the loss of species locally or strong spatial constraints on their movement can jeopardize management and conservation objectives, such as maintaining ecological integrity and ecosystem services (Ayantunde et al., 2011; Svenning et al., 2016; Wurtzebach and Schultz, 2016). There is growing interest in developing effective and efficient solutions toward sustainable HWC mitigation (Mumby and Plotnik, 2018), and integration HWC into landscape management will be key in this respect. This was well-coined two decades ago with the idea of reconciliation ecology, urging to rethink and design anthropogenic habitats so that their use is compatible with use by a broad array of other species (Rosenzweig, 2003).
While acknowledging that financial resources are often limited to carry out management actions (Richardson et al., 2020), the identification of cost-effective management interventions among a range of options can be difficult due to the multiple unknowns and uncertainties that characterize complex ecological systems (Ward et al., 2020). Understanding, predicting and controlling animal movement is key to designing anthropogenic landscapes that minimize the risk of negative interactions or maximize positive experience with wildlife. An additional challenge is to produce guidelines to assess the efficiency of management actions in the light of wildlife responses and human expectation. This is for instance the case with cross-boundary interactions that may occur at the interface between protected areas and their surroundings (Blanco et al., 2020) when animals move into (Piana and Marsden, 2014) and out of (Loveridge et al., 2017) protected areas to feed or migrate.
Here we propose an adaptive management framework (Walters and Hilborn, 1978; Richardson et al., 2020) that can be used to identify cost-effective solutions, while also minimizing constraints (e.g., avoid fencing off entire areas) on animal movement and distribution. We focus on conflicts involving animals for which conflict zones occupy only a portion of their home-range—a common situation for many species (e.g., Ripple et al., 2014; Soulsbury and White, 2015; Soliku and Schraml, 2018; Sigaud et al., 2020). The underlying idea is to use quantitative ecology to develop a species-specific predictive model of space-use dynamics, and then use this information to organize management actions over the landscape to divert animals away from conflict zones. The goal is not to completely constrain the movement of wildlife species but rather reduce human-wildlife interactions to socially acceptable levels. Our adaptive management approach includes four basic steps and a feedback loop (Figure 1): (A) define and spatialize HWC; (B) predict animal distribution from functional connectivity and patch residency time; (C) predict the impact of management actions on animal distribution; and (D) test predictions and revise the predictive model. Below we outline critical elements associated with each step of the management framework.
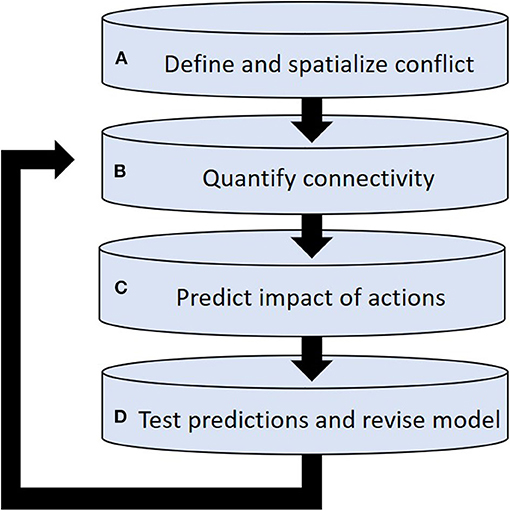
Figure 1. Conceptual framework of adaptive management proposed to mitigate human-wildlife conflicts based on four steps: (A) define and spatialize conflict areas by considering social tolerance and negative interactions between human and wildlife at local scale; (B) predict animal distribution from empirical models of functional connectivity and patch residency time; (C) use advection-diffusion equations developed from functional connectivity models to predict the impact of management actions on animal distribution; and (D) test predictions in the field and revise the model as more information become available.
Define and Spatialize Human-Wildlife Conflict
Human–wildlife conflict generally stems from negative interactions between humans and wildlife. Conflicts can involve a range of outcomes of human-animal interactions, such as human deaths (e.g., predation, wildlife vehicle collision), disease spread, impacts on vegetation, dissemination of exotic plants into protected areas, livestock depredation, crop-raiding, and property damage (Ujvári et al., 1998; Packer et al., 2005; Ripple et al., 2014; Hadidian, 2015; Sigaud et al., 2020; Simon and Fortin, 2020). For example, from 2005–2016, 21 727 cases of crop raiding, 6,768 of livestock depredation, and 1,152 of property damage were reported in Kenya (Long et al., 2020). Human-wildlife interactions can thus occur at high frequencies, with even multiple conflict types happening concurrently over a given area (Jordán and Báldi, 2013; Sigaud et al., 2020). HWC should be assessed while considering that people's degree of tolerance for wildlife can be fundamental to finding solutions to promote human coexistence with dangerous or damage-causing species (Treves and Bruskotter, 2014; Struebig et al., 2018). Hence, the level of HWC strongly depends on the tolerance of people toward wildlife (Ripple et al., 2014). This has led to the concept of social carrying capacity—the maximum wildlife population size that people are willing to tolerate (Cherry et al., 2019)—as well as to conflict-tolerance models (Kansky et al., 2016).
HWCs are unlikely to be uniformly distributed because landscape use by people and by wildlife generally displays spatial patterns at multiple scales. How often and where conflicts occur are partly determined by variation in environmental factors like resource distribution, agricultural practices, human occupation of land, and habitat connectivity (Mumby and Plotnik, 2018). Moreover, the presence a given behavior of animals may not be recognized and appreciated similarly by all people (Conforti and de Azevedo, 2003). Stakeholders may react differently to a given situation; for example, only some land owners give hunters access to their land (Simon and Fortin, 2019). Such differences exacerbate spatial heterogeneity in HWC. Defining HWC should therefore involve comprehensive assessment of the local situation, and locally acceptable levels should become management targets over local landscapes. A critical step in gaining insights into efficient organization of mitigation measures is therefore to spatialize conflict intensity, while considering patterns of negative interaction of wildlife with people and of social tolerance (Goswami et al., 2015; Kubasiewicz et al., 2016; Goswami and Vasudev, 2017).
Predict Animal Distribution From Functional Connectivity and Patch Residency Time
Where a conflict zone is only a portion of an individual's home range, managers are required to know what principles govern movement into conflict areas. They need this information to identify effective and efficient mitigation measures. Movement decisions are linked to landscape connectivity which involves structural and functional components. Structural connectivity relates to the spatial configuration of patches in the landscape, whereas functional connectivity relates to how animals move between patches (Baguette and Van Dyck, 2007). Functional connectivity is species-specific and is considered fundamental to landscape connectivity (Mimet et al., 2013).
Network theory can be used to determine the changes in landscape connectivity following a disturbance or a management practice. In spatial ecology, a network is comprised of nodes (high-quality patches) connected by links (inter-patch movements) (Fall et al., 2007). Many studies have emphasized the value of using a network-theory framework to assess landscape connectivity in the context of conservation and management planning (Minor and Urban, 2008; Urban et al., 2009). For example, the effects of disturbance of patches or links can vary among networks depending on topology (Urban and Keitt, 2001; Fortuna et al., 2006; Prima et al., 2019). Management actions may target different network components to achieve animal distributions that can reduce HWC.
In addition to network topology and associated functional connectivity, the time that animals spend in individual patches defines the spatial dynamics of animal distribution (Bastille-Rousseau et al., 2010; Stehfest et al., 2015). Non-random movements among network patches and residency time can be considered through the creation of weighted networks (Urban and Keitt, 2001; Prima et al., 2018, 2019), whereby each link in every direction is assigned a relative probability of being used, and each patch is given a residency time.
Weighted Network
Elements of spatial networks can be weighted based on field observations to reflect how long an animal will remain in a given patch and which patch will be visited next. Various methods have been used to quantify these elements in the field. For example, residency time has been determined by setting cameras in resource patches (Courant and Fortin, 2012), whereas, interpatch movements have been identified by mapping trail networks with GPS units (Dancose et al., 2011). Global Positioning System (GPS) radio-collars are now widely used to track wildlife with increasing accuracy and frequency of relocations. Such advancements in animal monitoring provides the opportunity to quantify both patch residency time and interpatch movement.
Residency Times
Residency times in individual patches is now commonly quantified by considering parts of the movement segments entering and leaving a patch, together with the numbers of successive locations inside the patch [see Figure 1 of Bastille-Rousseau et al. (2011) for an example of residency time calculation]. The time spent in different patches can then be related to habitat features to identify determinants of residency time. A statistical method called “Cox Proportional Hazards” model has been used to identify habitat features that can explain the relative risk that an animal leaves its current patch at any point in time (Bastille-Rousseau et al., 2011; Courbin et al., 2014). Not surprisingly, for example, bison (Bison bison) have a lower “risk” or probability of leaving a meadow at a given point in time when they have access to higher biomass of highly profitable vegetation (Courant and Fortin, 2012). Alternatively, Prima et al. (2018) used multiple linear regression to quantify the relationship between residency time in resource patches to patch size, and then considered this information as one of the inputs to model animal distribution.
Interpatch Movements
GPS tracking collars can also be used to determine the sequence of patches that are visited in a network (Dancose et al., 2011; Courbin et al., 2014; Merkle et al., 2015), and then identify factors influencing interpatch movements. Habitat properties that make it more likely that an animal moves to and selects a particular patch can be identified based on matched case-control design (Duchesne et al., 2010; Courbin et al., 2014; Merkle et al., 2014). The approach creates strata, with each stratum including a link (a potential interpatch movement) that was actually traveled by the collared animal (observed link, scored 1) and a set of links that could have been traveled from the same initial patch (random link, scored 0). Habitat features associated with traveled and available links are then contrasted based on conditional logistic regression (Compton et al., 2002). Patterned after step-selection functions (SSFs, Fortin et al., 2005) and in the context of HWC, the statistical model describing inter-patch movements can take the general form:
where ŵ is the model's score, βs are regression coefficients, LL is the link length [Euclidean or functional distance of the interpatch movement, Courbin et al. (2014) and Tardy et al. (2018)], ϕ is the angle difference between the direction of an observed or random link and that of the nearest conflict zone (Figure 2), D is the Euclidean distance to the conflict zone (Figure 2), and C is a parameter that allows the consideration of how the animal adjusts its movement with respect to the targeted patch (conflict zone) as a function of its distance to that patch. DC thus enables the model to consider that an animal may display stronger directional movement (cos[ϕ]) as it gets closer to the target (Bartoń et al., 2009). Covariates x1 to xp can represent a broad range of factors that influence movement decisions, such as movement taxis in response to various stimuli (Dancose et al., 2011; Latombe et al., 2014b; Nicosia et al., 2017) or selection of habitat features located either along the link leading to the next patch or associated directly with the location of that patch (Courbin et al., 2014; Merkle et al., 2014, 2015). For example, bison move toward canopy gaps when they travel in a forest, a behavior that has been revealed by estimating an SSF that included the cosine of the angle difference between the direction of observed or random interpatch movements and that of the nearest canopy gap (Dancose et al., 2011). Covariates can also include measurements such as the minimum distance to a particular habitat feature (e.g., nearest road), proportion of the link consisting of a particular land cover type (e.g., conifer forest, lake), expected energy requirement to reach the patch, and predation risk at the arrival patch (Fortin et al., 2005). Once parameters are estimated, Equation (1) can be used to assign weights to the links of the spatial network. The relative probability that the animal leaving patch j reaches patch i among the m patches that can be reached from its current location is given by:
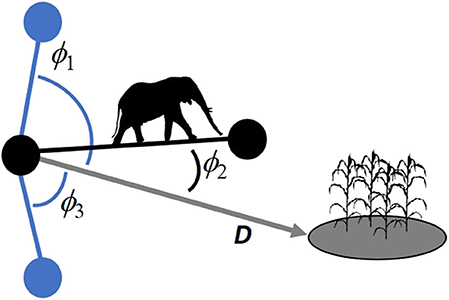
Figure 2. Example of observed (black to black patch) and potential (black to blue patch) interpatch movements, with associated measurements for the difference between the direction of an interpatch movement and the nearest conflict zone (ϕi, where ϕ1 and ϕ3 are associated with untraveled but available interpatch movements and ϕ2 is associated with the observed movement), and the Euclidean distance to the conflict zone (D).
Probabilities of all m potential interpatch movements thus sum up to 1 (see, e.g., m = 5 around each black patch in Figure 3). Available patches can be identified from field observations (O'Brien et al., 2006; Courbin et al., 2014; Prima et al., 2019).
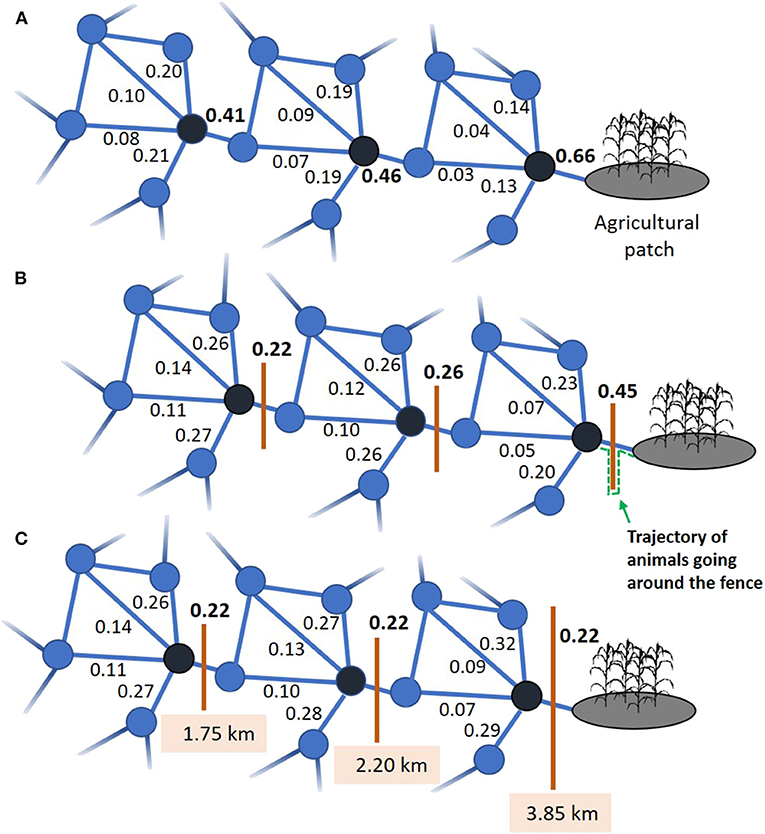
Figure 3. (A) Subset of a trail network showing the relative probability of traveling from the three black patches to each of the five connected patches, as estimated from Equation (3). (B) Outcome on the relative probability of traveling the different trails from the black nodes, following the erection of fences (orange lines). The 1.75-km fences intersect the edges between two patches that are 1.1 km apart, which imposes a 2.85-km path for an animal traveling between them while going around the fence, as illustrated by the dotted green line. (C) Fence lengths (1.75, 2.20, and 3.85 km) required to maintain a probability of 0.22 that the animal travels between the two patches by going around the fence, given that movement taxis intensifies with proximity to the agricultural patch.
Quantification of aji for the different links of a network can inform on the challenges and opportunities of reducing the risk of HWC. For example, consider a spatial network within which three patches have the same patterns of structural connectivity with respect to their adjacent patches (Figure 3A). We assume that the relative probability that an animal leaves one of the three patches to reach an adjacent patch follows:
Given this movement rule, the three patches display differences in functional connectivity despite having the same structural connectivity. This is because the attraction of animals toward the agricultural patch increases in intensity as they get closer (i.e., 1.2 D−0.5 cos[ϕ]). Such distance-dependent responses to habitat features have been reported for multiple species (McClintock et al., 2012; Preisler et al., 2013; Latombe et al., 2014a). To illustrate the calculation, let us consider the link between the nearest black node and the agricultural patch (Figure 3A). Following Equation (3), we can estimate ŵ = exp(−0.5 × 1.1 km + 1.2 × 1.1−0.5 × cos[10°]) = 1.78. After estimating ŵji for each of the other four connected patches, we can calculate a (Equation 2) for the link specifically leading to the agricultural patch as 1.78 / (0.08 + 0.11 + 0.37 + 0.33 + 1.78) = 0.66. More globally, aji values associated with the links leading the agricultural patch varied between 41 and 66% for all three hubs (black patches, Figure 3A).
Relative Use of Patch Network Given Movement Rules
Once the determinants of residency time and interpatch movement have been identified and their impact quantified, these elements of landscape connectivity can then be used to infer the relative distribution of individuals in the network. To do so, an advection-reaction-diffusion model can be used with predictions of animal distribution being proportional to relative intensity of space use (Prima et al., 2018). Consider the following reaction-diffusion model applied to a network with N patches:
where U(t) = [u1(t), u2(t),…, uN(t)]T is the vector of animal densities at time t in the N patches of the network, is the vector of instantaneous rate of change in U(t), is the vector of reaction functions in the N patches of the network and is the vector of diffusion functions in the N patches of the network (Kouvaris et al., 2012). The reaction term can be modeled as the density of individuals leaving patch i, based upon residency time in patch i:
where Ti is the average residency time in patch i. A higher residency time in patch i is reflected by a lower number of individuals leaving patch i. Advection properties can be modeled by assigning weights to the network's links, which then reflects an uneven movement of individuals between connected network patches. The advection process then can be implemented by modifying the diffusion term to:
where aji (Equation 2) which is proportional to the number of individuals arriving to patch i. Equation (4) becomes:
where I is the identity matrix, A is the weighted adjacency matrix of the network containing all weights aij, i, j ϵ {1, …, N}2, and T is a diagonal matrix of the residency times in the N nodes of the network. Predicted densities at any time of the simulation can be transformed to estimate relative intensity of space use:
where Ii(t) is the relative intensity of use for node i at time t.
To illustrate the relationship among functional connectivity, patch residency time and animal distribution dynamics, we created a fictive network of 50 natural resource patches and two human-related patches (agricultural patches 51 and 52) where HWC can occur (Figure 4A). We assume that animals display the same residency time (5 time units) in every patch, and inter-patch movements follows:
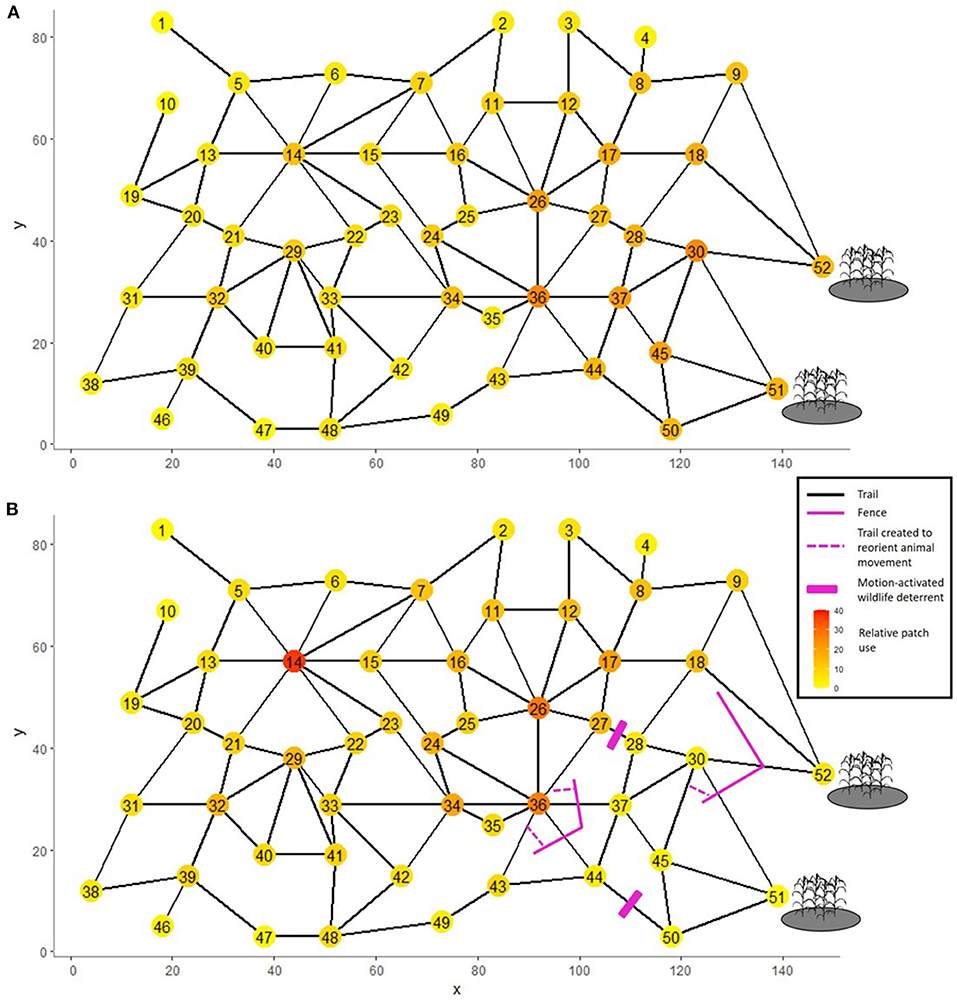
Figure 4. Hypothetical spatial network comprised of 52 patches—including two agricultural patches where human-wildlife conflicts occur—connected by links (trails) with relative probability of travel given by Equation (9). (A) Relative patch use before any management intervention. (B) Relative patch use following five types of interventions: erecting fences, crating trails to reorient animal traveling along a fence, deploying motion-activated wildlife deterrent, adding resources in patch 14, and disturbing of animals to reduce their residency times in patches 51 and 52.
Accordingly, animals are less likely to transit to a distant than nearby patch (βL < 0), and they are more likely to aim toward than away from the nearest agricultural patch (βD > 0), especially as they get closer to that patch (C < 0). We start the simulation with 10 individuals in each of the 52 patches and solve the system numerically to estimate the stable state solution, as described by Prima et al. (2018). In the context of adaptive management, transient distribution states might be of interest, which would simply require keeping track of how the system develops during its numerical resolution. In this case, initial conditions should impact how the system behave over time before reaching its steady state. An insightful approach could then be to set initial conditions to reflect animal distribution that creates human-wildlife conflicts. Here we simply focus on the steady-state solution.
Under these rules and once the steady-state is reached, we observe a directed network with a general gradient of increasing use toward the two agricultural patches (Figure 4A). The most heavily used patches, however, are not the two agricultural ones, which demonstrates the role of structural connectivity such as the node's degree (number of links).
Predicting Impacts of Management Actions
Once the relative use of resource patches within the network can be anticipated from basic movement rules, the predictive model can be used to anticipate the outcome management actions on the risk of HWC. Conflicts can be mitigated using a large set of potential interventions (Miller et al., 2016; Nyhus, 2016; Ravenelle and Nyhus, 2017; König et al., 2020). For example, wildlife authorities may disturb and chase problem animals out of sensitive areas. Managers can even benefit from early warning by fitting individuals with collars that relay information over the mobile telephone network when collared-animals enter a sensitive zone (Graham et al., 2012). Managers may install olfactory (e.g., the use of Capsicum spp. as barrier plants), acoustic (e.g., drones, sirens, firecrackers) and visual (e.g., flashing light) deterrents, some of which can be activated by motion sensors (Blackwell et al., 2016; Miller et al., 2016; Enukwa, 2017). Beehive fences can prevent the use of some areas by African elephants (Loxodonta africana), while also providing farmers with financial benefit through honey production (Enukwa, 2017). Virtual fencing can be deployed with shock collars that are triggered when collared animals reach a virtual boundary (Miller et al., 2016; Campbell et al., 2019). Chacma baboons (Papio ursinus) cause damage to both crops and human infrastructure in South Africa, and virtual fences have been used with varying levels of success to redirect baboons away from human settlement (Kaplan and O'Riain, 2014). The use of biofences to control predators has had some success, with constant reapplication of urine and feces (Ausband et al., 2013). Although the use of fences has been questioned (Pfeifer et al., 2014; Woodroffe et al., 2014), their use remains a classic means to restrict movements toward areas prone to HWC. When an area is not entirely fenced, animals can circumvent fences (Meagher, 1989; Hoare, 2012). Even then, the longer travel distance required by going around a fence should decrease the probability of making this interpatch movement because inter-patch movements become less likely with increasing travel distances (Dancose et al., 2011; Courbin et al., 2014; Tardy et al., 2018).
To illustrate how management actions can be tailored to movement rules within the patch network, we can go back to the example displayed in Figure 3. In this case, the probability of interpatch movement not only depends on travel distance, but also on the level of attraction of the nearest agricultural patch which increases as individuals get closer to this target. Such distance-dependent, directional movement implies that a given management action becomes less likely to be effective as animals approach the conflict zone. Let us assume that 1.75-km fences are set to impede movement toward the agricultural patch. Individuals can go around the fence by traveling 2.85 km (i.e., [0.55 km to travel the first half the trail length] + [1.75 km to walk to the end of the fence and then to walk back to the trail on the other side of the fence] + [0.55 km to travel the second half of the trail length = 2.85 km], Figure 3B), a distance that would reduce the likelihood of making that step following Equation (3) (Figure 3B). Indeed, LLji in Equation (3) will take a value of 2.85 with the fence, instead of 1.1 km without a fence. On this basis, we can estimate that animals would be twice as likely to move around the fence located the nearest than the farthest from the conflict zone (Equation 3). In fact, to maintain the same probability of traveling for all three focal nodes (black patches) the fence would need to gradually increase from 1.75 to 3.85 km (Figure 3C).
We now consider the more complex example displayed in Figure 4. Let us assume that we deploy four types of intervention in the patch network, each with different expected consequences on the response of large herbivores. This would be the case of bison, for example, living in a forest environment (e.g., landscape is displayed in Babin et al., 2011) adjacent to agricultural lands and traveling within a trail network (see illustrations in Dancose et al., 2011). The spatial distribution of management interventions can be decided based on various indices of landscape connectivity (e.g., betweenness centrality, Perry et al., 2017). Here we orient our decision from a visual inspection of network structure, as identified from greedy optimization of modularity (Clauset et al., 2004). We detected five network communities (sensu Cai et al., 2020) for which potential movements involve stronger interconnection among patches within than between communities. On this basis, we reduced movement toward the community involving the agricultural patches, while promoting movement out of that community. Specifically, we assume that a fence was erected with a design that prevents movements from 36 to 37 and 44, but not from 37 or 44 to 36 (Figure 4B). Another (virtual) fence was placed to prevent movement from 30 to 51 and 52. The fence configuration is expected, however, to reduce movement from 51 to 30 by decreasing ŵ51,30 by 50% (i.e., exp[−3 LL + 0.1 D−0.1 cos(ϕF)] × 0.5), and to increase movement from 52 to 18 by ŵ52,18 × 125%. We assume that a motion-activated wildlife deterrent (e.g., radio speaker) was installed along trails pointing away from patches 28 and 50 (Figure 4B), such that animals do not travel from 27 to 28 or from 44 to 50, but can still travel from 28 to 27 and from 50 to 44. We further assume that the addition of resources (food and/or water) double residency time in patch 14, whereas the disturbance of individuals (e.g., hazing) reduce their residency times in patches 51 and 52 from 5 to 4 time units. To assess the impact of these interventions on expected animal distribution, we start the simulation with 10 individuals in each of the 52 patches and solve the system numerically to estimate the stable state solution given these local changes in movement rules.
Before these interventions the two agricultural patches (51, 52) had 30.6 individuals (5.9% of the population) at equilibrium, which declined to 7.3 (1.4%) after the application of all these interventions (Figure 4B—remember that animal number is proportional to relative intensity of space use). If we remove a single intervention, we find that increasing residency time in patch 14 by adding resources had the least impact on the use of agricultural patches, whereas removing the fence between 36 and 37 and 44 had the largest impact (Figure 5). By contrast, if we implement a single intervention, we find that increasing residency time in patch 14 resulted in the lowest decrease in the use of the two agricultural patches, whereas erecting the fence between 30 and 51 and 52 had the largest impact. The lack of symmetry between the impact of implementing and removing a single intervention (Figure 5) illustrates that the effect of interventions cannot be expected to be simply additive to one another. A holistic assessment of management plans should therefore be carried out to identify the most effective strategy. As we show, the proposed analytical approach can provide such global assessment of multiple interventions altogether.
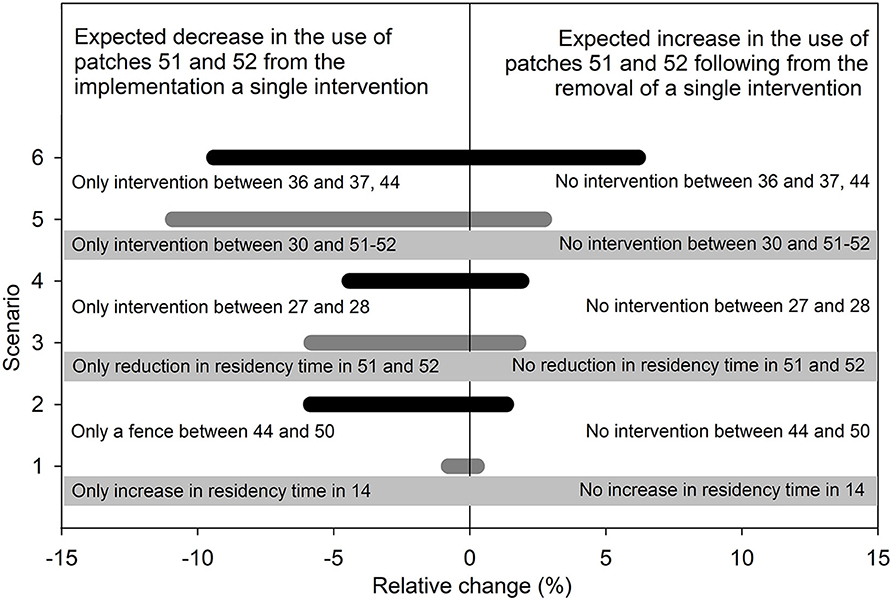
Figure 5. Predicted impact of implementing a single intervention among those displayed in Figure 4B on the combined use of the two agricultural patches displayed in Figure 4A, together with predicted impact of removing a single intervention among those displayed in Figure 4B on the combined use of the two agricultural patches displayed in Figure 4B. The text on gray background refers to the gray bars, where the text on the white background refers to the black bars.
Test Predictions and Revise Model
The implementation of management plans needs to be monitored for several reasons. For example, multiple species may be simultaneously impacted by local interventions. Monitoring is required to ensure that mitigation measures aimed at reducing a given HWC does not jeopardize other conservation or management objectives (Jordán and Báldi, 2013; Sigaud et al., 2020). Also, wildlife management is often driven by the immediate need to solve a problem (Blackwell et al., 2016), such actions may have to precede the development of a mitigation plan based on an exhaustive understanding the behaviors resulting in HWC. The choice of mitigation measures thus may be based on observations conducted on other species, on few observations of the system or an educated guess. Even highly detailed observations collected over short-term period are unlikely fully to capture all behavioral decisions causing HWC. This can be due, for example, to seasonal variations in animal-habitat relationships. In Canada bison typically only leave the safety of a national park (i.e., Prince Albert National Park) between mid-summer and mid-fall, when highly profitable vegetation is abundant in agricultural fields (Sigaud et al., 2017, 2020). Wild boar (Sus scrofa) in Alta Murgia National Park, Italy, thrive on natural vegetation during much of the year, and only switch to cultivated crops during summer (Ficetola et al., 2014). In Zimbabwe, African elephants switched to crop raiding toward the end of the wet season when grass quality in protected areas begin to decrease (Osborn, 2004). Both plant-herbivore and predator-prey interactions vary dynamically during the course of the year (Babin et al., 2011; Simon et al., 2019), such that movement decisions and related functional connectivity can also change.
The effectiveness of mitigation measures may also change over time. For biofencing to work, for example, urine and feces need to be frequently reapplied (Ausband et al., 2013). Monitoring is thus needed to determine how long each application maintains its effectiveness given the species involved and local environmental conditions. Management techniques also differ in how long they remain effective. Acoustic deterrents appear to impact carnivores/omnivores for only a few days, whereas shock collars can maintain their effectiveness for over a year (Miller et al., 2016). Given uncertainty in the effectiveness of actions, management plans can be improved over time by using an adaptive approach (Walters and Hilborn, 1978; Richardson et al., 2020). Information gathered by monitoring the response of animals to the management actions should thus be used to refine the predictive model (Figure 1), and adjust the mitigation plan over time to improve or maintain its efficiency and effectiveness.
Discussion
The need for effective HWC mitigation is more important now than ever, as across the globe humans and wildlife increasingly compete for space and resources. We present an adaptive management framework that involves the use of quantitative ecology to strategically alter the movement and distribution of animals in a way that reduces HWC. A fundamental principle is that animal movement can be characterized across the landscape, including along the main paths used to reach areas prone to HWC, and this information is then used to identify the most effective HWC mitigation strategies.
Although we specify that our examples could reflect a situation where bison might travel among well-delineated meadow patches in a forest matrix, the proposed framework is more broadly applicable. Our framework is suitable when animal movements can be predicted within a patch network. For example, a network approach was used to clarify the link between habitat changes and landscape connectivity for species ranging from small frogs (Schivo et al., 2020) to African elephants (Bastille-Rousseau and Wittemyer, 2020). As with these studies, our framework involves knowledge of how animals adjust their interpatch movements in response to landscape changes; however, here we suggest to actively manipulate landscape features to alter inter-patch functional connectivity in a way that results in suitable management or conservation outcomes.
Relevant spatial networks may be developed based on various patch types. Past studies have built network while considering, for instance, that nodes (patches) were water holes (Heintzman and McIntyre, 2019), discrete meadows (Prima et al., 2018), stands of deciduous vegetation (Courbin et al., 2014), or large stands of conifer vegetation (Prima et al., 2019). A patch is often a resource-rich area or a landcover type that is selectively used by the animal. The network of two species may thus be organized around different patch types, even if both species are established in the same landscape (Courbin et al., 2014). When habitat patches are difficult to circumscribe (e.g., less discrete systems), habitat selection analysis can provide guidance. O'Brien et al. (2006), for example, analyse habitat selection by woodland caribou (Rangifer tarandus caribou), and used the results to develop a spatial network for which high-quality patches were comprised of mature jack pine stands and sparsely treed rock. Movement among those patches then becomes the basis to define structural landscape connectivity.
Managers develop strategies for conservation while considering that the intensity and spatial patterns of HWC can be highly dynamic. For example, information sharing among animals can lead to a steep increase in HWC, with problematic behaviors becoming the norm within a few years (Sigaud et al., 2017). Also, the presence of animals on private lands may be undesirable only during a portion of the year, such as when wildlife might interact with domestic animals or when damage to property is most likely. Management may then involve interventions deployed specifically where and when the level of social tolerance toward wildlife is exceeded. In this context, public outreach programs and monetary compensation for wildlife damage may be used to reduce HWC by increasing social tolerance (Ravenelle and Nyhus, 2017). Conservation agencies may also purchase lands with high HWC or where managers can attract wildlife to lessen HWC elsewhere (Curran et al., 2016; Sijtsma et al., 2020).
Management strategies should be developed while considering that interventions can have consequences well-beyond the target population or the conflict areas (Osipova et al., 2018; Sigaud et al., 2020). Efforts to protect a given population may even conflict with the conservation objectives of other populations (Williams et al., 2011; Baynham-Herd et al., 2018). Increasing social tolerance can certainly reduce HWC with minimal impact on wildlife species and their habitat. Here we propose to make management plans based on strategically placed interventions, so that managers can pinpoint where HWC mitigation measures would be most effective with the least impact on animals not involved in conflicts. Accordingly, assessing the interplay between animal movement and landscape features should be done while considering that different population members may use different movement tactics. For example, males and females of a given species do not use the landscape similarly (e.g., Bjorneraas et al., 2012; Marchand et al., 2015; Paton et al., 2017), and in the case of the African elephant, males are more frequently involved in HWC (Cook et al., 2015; Orrick, 2018). Even individuals of the same sex of may display different tactics (Dussault et al., 2012; Losier et al., 2015), with some being more likely to trigger HWC (Sigaud et al., 2017). To be most effective and minimize the impact on non-problem animals, functional connectivity can be quantified concurrently for population members with different movement tactics and for other species (e.g., Courbin et al., 2014); the expected impact of management can then be assessed broadly for community members based on the proposed framework.
Our study demonstrates how quantitative ecology can help understand spatio-temporal patterns of animal distribution, and provide a valuable basis to for the development of effective and efficient management strategies to mitigate HWC. By modeling HWC managers and conservationists can benefit from testing different scenarios before implementation, especially where non-target species are involved. We provide a method for “out of the box thinking” (Shivik, 2006; Blackwell et al., 2016) in line with the notion that fencing broad areas is not a panacea of HWC solving. As more options become available, our framework can provide guidance for the deployment of management actions to reduce conflicts to socially acceptable levels.
Data Availability Statement
The original contributions presented in the study are included in the article/supplementary materials, further inquiries can be directed to the corresponding author/s.
Author Contributions
DF: conceptualization, formal analysis, funding acquisition, methodology, wrote—original draft, wrote—review and editing. CB, PL, HF, PM, and OP: wrote—review and editing. All authors contributed to the article and approved the submitted version.
Funding
Funding for this research was provided by Université Laval, Natural Sciences and Engineering Research Council of Canada, and by Canada First Research Excellence Fund through the Sentinel North's research program.
Conflict of Interest
The authors declare that the research was conducted in the absence of any commercial or financial relationships that could be construed as a potential conflict of interest.
References
Ausband, D. E., Mitchell, M. S., Bassing, S. B., and White, C. (2013). No trespassing: using a biofence to manipulate wolf movements. Wildl. Res. 40, 207–216. doi: 10.1071/WR12176
Ayantunde, A. A., de Leeuw, J., Turner, M. D., and Said, M. (2011). Challenges of assessing the sustainability of (agro)-pastoral systems. Livest. Sci. 139, 30–43. doi: 10.1016/j.livsci.2011.03.019
Babin, J.-S., Fortin, D., Wilmshurst, J. F., and Fortin, M.-E. (2011). Energy gains predict the distribution of plains bison across populations and ecosystems. Ecology 92, 240–252. doi: 10.1890/10-0252.1
Baguette, M., and Van Dyck, H. (2007). Landscape connectivity and animal behavior: functional grain as a key determinant for dispersal. Landsc. Ecol. 22, 1117–1129. doi: 10.1007/s10980-007-9108-4
Bartoń, K. A., Phillips, B. L., Morales, J. M., and Travis, J. M. J. (2009). The evolution of an 'intelligent' dispersal strategy: biased, correlated random walks in patchy landscapes. Oikos 118, 309–319. doi: 10.1111/j.1600-0706.2008.16936.x
Bastille-Rousseau, G., Fortin, D., and Dussault, C. (2010). Inference from habitat-selection analysis depends on foraging strategies. J. Anim. Ecol. 79, 1157–1163. doi: 10.1111/j.1365-2656.2010.01737.x
Bastille-Rousseau, G., Fortin, D., Dussault, C., Courtois, R., and Ouellet, J.-P. (2011). Foraging strategies by omnivores: are black bears actively searching for ungulate neonates or are they simply opportunistic predators? Ecography 34, 588–596. doi: 10.1111/j.1600-0587.2010.06517.x
Bastille-Rousseau, G., and Wittemyer, G. (2020). Characterizing the landscape of movement to identify critical wildlife habitat and corridors. Conserv. Biol. doi: 10.1111/cobi.13519. [Epub ahead of print].
Baynham-Herd, Z., Redpath, S., Bunnefeld, N., Molony, T., and Keane, A. (2018). Conservation conflicts: behavioural threats, frames, and intervention recommendations. Biol. Conserv. 222, 180–188. doi: 10.1016/j.biocon.2018.04.012
Bjorneraas, K., Herfindal, I., Solberg, E. J., Sther, B. E., van Moorter, B., and Rolandsen, C. M. (2012). Habitat quality influences population distribution, individual space use and functional responses in habitat selection by a large herbivore. Oecologia 168, 231–243. doi: 10.1007/s00442-011-2072-3
Blackwell, B. F., DeVault, T. L., Fernandez-Juricic, E., Gese, E. M., Gilbert-Norton, L., and Breck, S. W. (2016). No single solution: application of behavioural principles in mitigating human-wildlife conflict. Anim. Behav. 120, 245–254. doi: 10.1016/j.anbehav.2016.07.013
Blanco, J., Bellon, B., Fabricius, C., Roque, F. D., Pays, O., Laurent, F., et al. (2020). Interface processes between protected and unprotected areas: a global review and ways forward. Glob. Chang. Biol. 26, 1138–1154. doi: 10.1111/gcb.14865
Cai, B., Wang, Y. P., Zeng, L. N., Hu, Y. M., and Li, H. J. (2020). Edge classification based on convolutional neural networks for community detection in complex network. Phys. Stat. Mech. Appl. 556:124826. doi: 10.1016/j.physa.2020.124826
Campbell, D. L. M., Lea, J. M., Keshavarzi, H., and Lee, C. (2019). Virtual fencing is comparable to electric tape fencing for cattle behavior and welfare. Front. Vet. Sci. 6:445. doi: 10.3389/fvets.2019.00445
CBD [Secretariat of the Convention on Biological Diversity] (2018). Aichi Biodiversity Targets. Available online at: https://www.cbd.int/sp/targets/ (accessed October 8, 2020).
Cherry, S. G., Merkle, J. A., Sigaud, M., Fortin, D., and Wilson, G. A. (2019). Managing genetic diversity and extinction risk for a rare plains bison (Bison bison bison) population. Environ. Manage. 64, 553–563. doi: 10.1007/s00267-019-01206-2
Clauset, A., Newman, M. E. J., and Moore, C. (2004). Finding community structure in very large networks. Phys. Rev. E Stat. Nonlin. Soft. Matter. Phys. 70:066111. doi: 10.1103/PhysRevE.70.066111
Compton, B. W., Rhymer, J. M., and McCollough, M. (2002). Habitat selection by wood turtles (Clemmys Insculpta): an application of paired logistic regression. Ecology 83, 833–843. doi: 10.2307/3071885
Conforti, V. A., and de Azevedo, F. C. C. (2003). Local perceptions of jaguars (Panthera onca) and pumas (Puma concolor) in the Iguaçu National park area, south Brazil. Biol. Conserv. 111, 215–221. doi: 10.1016/S0006-3207(02)00277-X
Cook, R. M., Henley, M. D., and Parrini, F. (2015). Elephant movement patterns in relation to human inhabitants in and around the Great Limpopo transfrontier park. Koedoe 57:a1298. doi: 10.4102/koedoe.v57i1.1298
Courant, S., and Fortin, D. (2012). Time allocation of bison in meadow patches driven by potential energy gains and group size dynamics. Oikos 121, 1163–1173. doi: 10.1111/j.1600-0706.2011.19994.x
Courbin, N., Fortin, D., Dussault, C., and Courtois, R. (2014). Logging-induced changes in habitat network connectivity shape behavioral interactions in the wolf-caribou-moose system. Ecol. Monogr. 84, 265–285. doi: 10.1890/12-2118.1
Curran, M., Kiteme, B., Wunscher, T., Koellner, T., and Hellweg, S. (2016). Pay the farmer, or buy the land?-Cost-effectiveness of payments for ecosystem services versus land purchases or easements in Central Kenya. Ecol. Econ. 127, 59–67. doi: 10.1016/j.ecolecon.2016.03.016
Dancose, K., Fortin, D., and Guo, X. L. (2011). Mechanisms of functional connectivity: the case of free-ranging bison in a forest landscape. Ecol. Appl. 21, 1871–1885. doi: 10.1890/10-0779.1
Dickman, A. J. (2010). Complexities of conflict: the importance of considering social factors for effectively resolving human-wildlife conflict. Anim. Conserv. 13, 458–466. doi: 10.1111/j.1469-1795.2010.00368.x
Duchesne, T., Fortin, D., and Courbin, N. (2010). Mixed conditional logistic regression for habitat selection studies. J. Anim. Ecol. 79, 548–555. doi: 10.1111/j.1365-2656.2010.01670.x
Dussault, C., Pinard, V., Ouellet, J. P., Courtois, R., and Fortin, D. (2012). Avoidance of roads and selection for recent cutovers by threatened caribou: fitness-rewarding or maladaptive behaviour? Proc. R Soc. B 279, 4481–4488. doi: 10.1098/rspb.2012.1700
Enukwa, E. H. (2017). Human-elephant confilict mitigation methods: a review of effectiveness and sustainability. J. Wildl. Biodivers. 1, 69–78. Available online at: http://www.wildlife-biodiversity.com/article_28260_18d2b0113a7db22e0688f9080209e6f5.pdf
Fall, A., Fortin, M. J., Manseau, M., and O'Brien, D. (2007). Spatial graphs: principles and applications for habitat connectivity. Ecosystems 10, 448–461. doi: 10.1007/s10021-007-9038-7
Ficetola, G. F., Bonardi, A., Mairota, P., Leronni, V., and Padoa-Schioppa, E. (2014). Predicting wild boar damages to croplands in a mosaic of agricultural and natural areas. Curr. Zool. 60, 170–179. doi: 10.1093/czoolo/60.2.170
Fortin, D., Beyer, H. L., Boyce, M. S., Smith, D. W., Duchesne, T., and Mao, J. S. (2005). Wolves influence elk movements: behavior shapes a trophic cascade in Yellowstone National park. Ecology 86, 1320–1330. doi: 10.1890/04-0953
Fortuna, M. A., Gomez-Rodriguez, C., and Bascompte, J. (2006). Spatial network structure and amphibian persistence in stochastic environments. Proc. R. Soc. B Biol. Sci. 273, 1429–1434. doi: 10.1098/rspb.2005.3448
Frank, B. (2016). Human-wildlife conflicts and the need to include tolerance and coexistence: an Introductory comment. Soc. Nat. Resour. 29, 738–743. doi: 10.1080/08941920.2015.1103388
Gaynor, K. M., Hojnowski, C. E., Carter, N. H., and Brashares, J. S. (2018). The influence of human disturbance on wildlife nocturnality. Science 360, 1232–1235. doi: 10.1126/science.aar7121
Goswami, V. R., Medhi, K., Nichols, J. D., and Oli, M. K. (2015). Mechanistic understanding of human-wildlife conflict through a novel application of dynamic occupancy models. Conserv. Biol. 29, 1100–1110. doi: 10.1111/cobi.12475
Goswami, V. R., and Vasudev, D. (2017). Triage of conservation needs: the juxtaposition of conflict mitigation and connectivity considerations in heterogeneous, human-dominated landscapes. Front. Ecol. Evol. 4:144. doi: 10.3389/fevo.2016.00144
Graham, M. D., Adams, W. M., and Kahiro, G. N. (2012). Mobile phone communication in effective human-elephant conflict management in Laikipia County, Kenya. Oryx 46, 137–144. doi: 10.1017/S0030605311001104
Green, R. E., Cornell, S. J., Scharlemann, J. P. W., and Balmford, A. (2005). Farming and the fate of wild nature. Science 307, 550–555. doi: 10.1126/science.1106049
Hadidian, J. (2015). Wildlife in US cities: managing unwanted animals. Animals 5, 1092–1113. doi: 10.3390/ani5040401
Heintzman, L. J., and McIntyre, N. E. (2019). Quantifying the effects of projected urban growth on connectivity among wetlands in the Great Plains (USA). Landsc. Urban Plan 186, 1–12. doi: 10.1016/j.landurbplan.2019.02.007
Hoare, R. (2012). Lessons from 15 years of human–elephant conflict mitigation: Management considerations involving biological, physical and governance issues in Africa. Pachyderm 51 60–74. Available online at: https://pachydermjournal.org/index.php/pachyderm/article/view/291/248
IUCN (2020). IUCNSSC Human-Wildlife Conflict Task Force. Available online at: http://www.hwctf.org (accessed April 20, 2020).
Jordán, F., and Báldi, A. (2013). Short communication: systems-based conservation and conflicts between species protection programs. Web Ecol. 13 85–89. doi: 10.5194/we-13-85-2013
Kansky, R., Kidd, M., and Knight, A. T. (2016). A wildlife tolerance model and case study for understanding human wildlife conflicts. Biol. Conserv. 201, 137–145. doi: 10.1016/j.biocon.2016.07.002
Kaplan, B. S., and O'Riain, J. M. (2014). Shedding light on reflective prisms as potential baboon (Papio ursinus) deterrents in the Cape Peninsula, South Africa. African J. Wildl. Res. 45, 117–121. doi: 10.3957/056.045.0108
König, H. J., Kiffner, C., Kramer-Schadt, S., Fürst, C., Keuling, O., and Ford, A. T. (2020). Human-wildlife coexistence in a changing world. Conserv. Biol. 34, 786–794. doi: 10.1111/cobi.13513
Kouvaris, N. E., Kori, H., and Mikhailov, A. S. (2012). Traveling and pinned fronts in bistable reaction-diffusion systems on networks. PLoS ONE 7:e45029. doi: 10.1371/journal.pone.0045029
Kubasiewicz, L. M., Bunnefeld, N., Tulloch, A. I. T., Quine, C. P., and Park, K. J. (2016). Diversionary feeding: an effective management strategy for conservation conflict? Biodivers. Conserv. 25, 1–22. doi: 10.1007/s10531-015-1026-1
Latombe, G., Fortin, D., and Parrott, L. (2014a). Spatio-temporal dynamics in the response of woodland caribou and moose to the passage of grey wolf. J. Anim. Ecol. 83, 185–198. doi: 10.1111/1365-2656.12108
Latombe, G., Parrott, L., Basille, M., and Fortin, D. (2014b). Uniting statistical and individual-based approaches for animal movement modelling. PLoS ONE 9:e99938. doi: 10.1371/journal.pone.0099938
Long, H. P., Mojo, D., Fu, C., Wang, G. Q., Kanga, E., Oduor, A. M. O., et al. (2020). Patterns of human-wildlife conflict and management implications in Kenya: a national perspective. Hum. Dimens Wildlife 25, 121–135. doi: 10.1080/10871209.2019.1695984
Losier, C. L., Couturier, S., St-Laurent, M. H., Drapeau, P., Dussault, C., Rudolph, T., et al. (2015). Adjustments in habitat selection to changing availability induce fitness costs for a threatened ungulate. J. Appl. Ecol. 52, 496–504. doi: 10.1111/1365-2664.12400
Loveridge, A. J., Valeix, M., Elliot, N. B., and Macdonald, D. W. (2017). The landscape of anthropogenic mortality: how African lions respond to spatial variation in risk. J. Appl. Ecol. 54, 815–825. doi: 10.1111/1365-2664.12794
Marchand, P., Garel, M., Bourgoin, G., Dubray, D., Maillard, D., and Loison, A. (2015). Coupling scale-specific habitat selection and activity reveals sex-specific food/cover trade-offs in a large herbivore. Anim. Behav. 102, 169–187. doi: 10.1016/j.anbehav.2015.01.011
McClintock, B. T., King, R., Thomas, L., Matthiopoulos, J., McConnell, B. J., and Morales, J. M. (2012). A general discrete-time modeling framework for animal movement using multistate random walks. Ecol. Monogr. 82, 335–349. doi: 10.1890/11-0326.1
Meagher, M. (1989). Evaluation of boundary control for bison of Yellowstone National park. Wildl. Soc. Bull. 17, 15–19.
Merkle, J. A., Fortin, D., and Morales, J. M. (2014). A memory-based foraging tactic reveals an adaptive mechanism for restricted space use. Ecol. Lett. 17, 924–931. doi: 10.1111/ele.12294
Merkle, J. A., Sigaud, M., and Fortin, D. (2015). To follow or not? How animals in fusion-fission societies handle conflicting information during group decision-making. Ecol. Lett. 18, 799–806. doi: 10.1111/ele.12457
Miller, J. R. B., Stoner, K. J., Cejtin, M. R., Meyer, T. K., Middleton, A. D., and Schmitz, O. J. (2016). Effectiveness of contemporary techniques for reducing livestock depredations by large carnivores. Wildl. Soc. Bull. 40, 806–815. doi: 10.1002/wsb.720
Mimet, A., Houet, T., Julliard, R., and Simon, L. (2013). Assessing functional connectivity: a landscape approach for handling multiple ecological requirements. Methods Ecol. Evol. 4, 453–463. doi: 10.1111/2041-210x.12024
Minor, E. S., and Urban, D. L. (2008). A graph-theory frarmework for evaluating landscape connectivity and conservation planning. Conserv. Biol. 22, 297–307. doi: 10.1111/j.1523-1739.2007.00871.x
Mumby, H. S., and Plotnik, J. M. (2018). Taking the elephants' perspective: remembering elephant behavior, cognition and ecology in human-elephant conflict mitigation. Front. Ecol. Evol. 6:122. doi: 10.3389/fevo.2018.00122
Nicosia, A., Duchesne, T., Rivest, L. P., and Fortin, D. (2017). A multi-state conditional logistic regression model for the analysis of animal movement. Ann. Appl. Stat. 11, 1537–1560. doi: 10.1214/17-AOAS1045
Nyhus, P. J. (2016). Human-wildlife conflict and coexistence. Annu. Rev. Environ. Resour. 41, 143–171. doi: 10.1146/annurev-environ-110615-085634
O'Brien, D., Manseau, M., Fall, A., and Fortin, M. J. (2006). Testing the importance of spatial configuration of winter habitat for woodland caribou: an application of graph theory. Biol. Conserv. 130, 70–83. doi: 10.1016/j.biocon.2005.12.014
Orrick, K. D. (2018). Range size and drivers of African elephant (Loxodonta africana) space use on Karongwe Private game reserve, South Africa. Afr. J. Ecol. 56, 572–581. doi: 10.1111/aje.12500
Osborn, F. V. (2004). Seasonal variation of feeding patterns and food selection by crop-raiding elephants in Zimbabwe. Afr. J. Ecol. 42, 322–327. doi: 10.1111/j.1365-2028.2004.00531.x
Osipova, L., Okello, M. M., Njumbi, S. J., Ngene, S., Western, D., Hayward, M. W., et al. (2018). Fencing solves human-wildlife conflict locally but shifts problems elsewhere: A case study using functional connectivity modelling of the African elephant. J. Appl. Ecol. 55, 2673–2684. doi: 10.1111/1365-2664.13246
Packer, C., Ikanda, D., Kissui, B., and Kushnir, H. (2005). Lion attacks on humans in Tanzania-understanding the timing and distribution of attacks on rural communities will help to prevent them. Nature 436, 927–928. doi: 10.1038/436927a
Paton, D. G., Ciuti, S., Quinn, M., and Boyce, M. S. (2017). Hunting exacerbates the response to human disturbance in large herbivores while migrating through a road network. Ecosphere 8, e01841. doi: 10.1002/ecs2.1841
Pereira, H. M., Leadley, P. W., Proenca, V., Alkemade, R., Scharlemann, J. P. W., Fernandez-Manjarres, J. F., et al. (2010). Scenarios for global biodiversity in the 21st Century. Science 330, 1496–1501. doi: 10.1126/science.1196624
Perry, G. L. W., Moloney, K. A., and Etherington, T. R. (2017). Using network connectivity to prioritise sites for the control of invasive species. J. Appl. Ecol. 54, 1238–1250. doi: 10.1111/1365-2664.12827
Peterson, M. N., Birckhead, J. L., Leong, K., Peterson, M. J., and Peterson, T. R. (2010). Rearticulating the myth of human-wildlife conflict. Conserv. Lett. 3, 74–82. doi: 10.1111/j.1755-263X.2010.00099.x
Pfeifer, M., Packer, C., Burton, A. C., Garnett, S. T., Loveridge, A. J., MacNulty, D., et al. (2014). In defense of fences. Science 345, 389–389. doi: 10.1126/science.345.6195.389-a
Piana, R. P., and Marsden, S. J. (2014). Impacts of cattle grazing on forest structure and raptor distribution within a neotropical protected area. Biodivers. Conserv. 23, 559–572. doi: 10.1007/s10531-013-0616-z
Preisler, H. K., Ager, A. A., and Wisdom, M. J. (2013). Analyzing animal movement patterns using potential functions. Ecosphere 4:art32. doi: 10.1890/ES12-00286.1
Prima, M. C., Duchesne, T., Fortin, A., Rivest, L. P., Drapeau, P., St-Laurent, M. H., et al. (2019). A landscape experiment of spatial network robustness and space-use reorganization following habitat fragmentation. Funct. Ecol. 33, 1663–1673. doi: 10.1111/1365-2435.13380
Prima, M. C., Duchesne, T., Fortin, A., Rivest, L. P., and Fortin, D. (2018). Combining network theory and reaction-advection-diffusion modelling for predicting animal distribution in dynamic environments. Methods Ecol. Evol. 9, 1221–1231. doi: 10.1111/2041-210X.12997
Ravenelle, J., and Nyhus, P. J. (2017). Global patterns and trends in human-wildlife conflict compensation. Conserv. Biol. 31, 1247–1256. doi: 10.1111/cobi.12948
Richardson, S., Mill, A. C., Davis, D., Jam, D., and Ward, A. I. (2020). A systematic review of adaptive wildlife management for the control of invasive, non-native mammals, and other human-wildlife conflicts. Mamm. Rev. 50, 147–156. doi: 10.1111/mam.12182
Ripple, W. J., Estes, J. A., Beschta, R. L., Wilmers, C. C., Ritchie, E. G., Hebblewhite, M., et al. (2014). Status and ecological effects of the world's largest carnivores. Science 343:151. doi: 10.1126/science.1241484
Rosenzweig, M. L. (2003). Reconciliation ecology and the future of species diversity. Oryx 37, 194–205. doi: 10.1017/S0030605303000371
Rosenzweig, M. L., and Ziv, Y. (1999). The echo pattern of species diversity: pattern and processes. Ecography 22, 614–628. doi: 10.1111/j.1600-0587.1999.tb00510.x
Schivo, F., Mateo-Sanchez, M. C., Bauni, V., and Quintana, R. D. (2020). Influence of land-use/land-cover change on landscape connectivity for an endemic threatened amphibian ( Argenteohyla siemersi pederseni, Anura: Hylidae). Landsc. Ecol. 35, 1481–1494. doi: 10.1007/s10980-020-01031-7
Shivik, J. A. (2006). Tools for the edge: what's new for conserving carnivores. Bioscience 56, 253–259. doi: 10.1641/0006-3568(2006)056[0253:TFTEWN]2.0.CO;2
Sigaud, M., Mason, T. H. E., Barnier, F., Cherry, S. G., and Fortin, D. (2020). Emerging conflict between conservation programmes: when a threatened vertebrate facilitates the dispersal of exotic species in a rare plant community. Anim. Conserv. doi: 10.1111/acv.12579
Sigaud, M., Merkle, J. A., Cherry, S. G., Fryxell, J. M., Berdahl, A., and Fortin, D. (2017). Collective decision-making promotes fitness loss in a fusion-fission society. Ecol. Lett. 20, 33–40. doi: 10.1111/ele.12698
Sijtsma, F. J., van der Veen, E., van Hinsberg, A., Pouwels, R., Bekker, R., van Dijk, R. E., et al. (2020). Ecological impact and cost-effectiveness of wildlife crossings in a highly fragmented landscape: a multi-method approach. Landsc. Ecol. 35, 1701–1720. doi: 10.1007/s10980-020-01047-z
Simon, R. N., Cherry, S. G., and Fortin, D. (2019). Complex tactics in a dynamic large herbivore-carnivore spatiotemporal game. Oikos 128, 1318–1328. doi: 10.1111/oik.06166
Simon, R. N., and Fortin, D. (2019). Linking habitat use to mortality and population viability to disarm an ecological trap. Biol. Conserv. 236, 366–374. doi: 10.1016/j.biocon.2019.05.001
Simon, R. N., and Fortin, D. (2020). Crop raiders in an ecological trap: optimal foraging individual-based modeling quantifies the effect of alternate crops. Ecol. Appl. 30:e02111. doi: 10.1002/eap.2111
Soliku, O., and Schraml, U. (2018). Making sense of protected area conflicts and management approaches: A review of causes, contexts and conflict management strategies. Biol. Conserv. 222, 136–145. doi: 10.1016/j.biocon.2018.04.011
Soulsbury, C. D., and White, P. C. L. (2015). Human-wildlife interactions in urban areas: a review of conflicts, benefits and opportunities. Wildl. Res. 42, 541–553. doi: 10.1071/WR14229
Stehfest, K. M., Patterson, T. A., Barnett, A., and Semmens, J. M. (2015). Markov models and network analysis reveal sex-specific differences in the space-use of a coastal apex predator. Oikos 124, 307–318. doi: 10.1111/oik.01429
Struebig, M. J., Linkie, M., Deere, N. J., Martyr, D. J., Millyanawati, B., Faulkner, S. C., et al. (2018). Addressing human-tiger conflict using socio-ecological information on tolerance and risk. Nat. Commun. 9:3455. doi: 10.1038/s41467-018-05983-y
Svenning, J. C., Pedersen, P. B. M., Donlan, C. J., Ejrnaes, R., Faurby, S., Galetti, M., et al. (2016). Science for a wilder Anthropocene: synthesis and future directions for trophic rewilding research. Proc. Natl. Acad. Sci. U.S.A. 113, 898–906. doi: 10.1073/pnas.1502556112
Tardy, O., Masse, A., Pelletier, F., and Fortin, D. (2018). Interplay between contact risk, conspecific density, and landscape connectivity: an individual-based modeling framework. Ecol. Modell. 373, 25–38. doi: 10.1016/j.ecolmodel.2018.02.003
Treves, A., and Bruskotter, J. (2014). Tolerance for predatory wildlife. Science 344, 475–476. doi: 10.1126/science.1252690
Tucker, M. A., Bohning-Gaese, K., Fagan, W. F., Fryxell, J. M., Van Moorter, B., Alberts, S. C., et al. (2018). Moving in the anthropocene: global reductions in terrestrial mammalian movements. Science 359, 466–469. doi: 10.1126/science.aam9712
Turvey, S. T., and Crees, J. J. (2019). Extinction in the anthropocene. Curr. Biol. 29, R982–R986. doi: 10.1016/j.cub.2019.07.040
Ujvári, M., Baagøe, H. J., and Madsen, A. B. (1998). Effectiveness of wildlife warning reflectors in reducing deer-vehicle collisions: a behavioral study. J. Wildl. Manage. 62, 1094–1099. doi: 10.2307/3802562
Urban, D., and Keitt, T. (2001). Landscape connectivity: a graph-theoretic perspective. Ecology 82, 1205–1218. doi: 10.1890/0012-9658(2001)082[1205:LCAGTP]2.0.CO;2
Urban, D. L., Minor, E. S., Treml, E. A., and Schick, R. S. (2009). Graph models of habitat mosaics. Ecol. Lett. 12, 260–273. doi: 10.1111/j.1461-0248.2008.01271.x
Walters, C. J., and Hilborn, R. (1978). Ecological optimization and adaptive management. Annu. Rev. Ecol. Syst. 9, 157–188. doi: 10.1146/annurev.es.09.110178.001105
Ward, A. I., Richardson, S., Macarthur, R., and Mill, A. C. (2020). Using and communicating uncertainty for the effective control of invasive non-native species. Mamm. Rev. 50, 211–220. doi: 10.1111/mam.12188
Williams, R., Krkosek, M., Ashe, E., Branch, T. A., Clark, S., Hammond, P. S., et al. (2011). Competing conservation objectives for predators and prey: estimating killer whale prey requirements for Chinook salmon. PLoS ONE 6:e26738. doi: 10.1371/journal.pone.0026738
Woodroffe, R., Hedges, S., and Durant, S. (2014). In defense of fences-response. Science 345, 389–390. doi: 10.1126/science.345.6195.389-b
Wurtzebach, Z., and Schultz, C. (2016). Measuring ecological integrity: history, practical applications, and research opportunities. Bioscience 66, 446–457. doi: 10.1093/biosci/biw037
WWF (2020). WWF - Human-Wildlife Conflict. Available online at: https://wwf.panda.org/our_work/wildlife/problems/human_animal_conflict (accessed April 20, 2020).
Keywords: adaptive management, functional connectivity, human-wildlife conflict (HWC), movement ecology, patch network, quantitative ecology
Citation: Fortin D, Brooke CF, Lamirande P, Fritz H, McLoughlin PD and Pays O (2020) Quantitative Spatial Ecology to Promote Human-Wildlife Coexistence: A Tool for Integrated Landscape Management. Front. Sustain. Food Syst. 4:600363. doi: 10.3389/fsufs.2020.600363
Received: 29 August 2020; Accepted: 21 October 2020;
Published: 17 November 2020.
Edited by:
Pablo Gregorini, Lincoln University, New ZealandReviewed by:
Emilio Andres Laca, University of California, Davis, United StatesNicholas Tyler, Arctic University of Norway, Norway
Copyright © 2020 Fortin, Brooke, Lamirande, Fritz, McLoughlin and Pays. This is an open-access article distributed under the terms of the Creative Commons Attribution License (CC BY). The use, distribution or reproduction in other forums is permitted, provided the original author(s) and the copyright owner(s) are credited and that the original publication in this journal is cited, in accordance with accepted academic practice. No use, distribution or reproduction is permitted which does not comply with these terms.
*Correspondence: Daniel Fortin, daniel.fortin@bio.ulaval.ca