- 1Department of Agricultural Economics, School of Agriculture and Enterprise Development, Kenyatta University, Nairobi, Kenya
- 2Department of Land and Water Management, School of Agriculture, University of Embu, Embu, Kenya
- 3Department of Agricultural Science and Technology, School of Agriculture and Enterprise Development, Kenyatta University, Nairobi, Kenya
- 4National Agricultural Research Laboratories, Kenya Agricultural Livestock and Research Organization Nairobi, Nairobi, Kenya
- 5International Department, Research Institute of Organic Agriculture (FiBL), Frick, Switzerland
Soil fertility decline continues to be a major challenge limiting agricultural productivity globally. Despite the novelty of organic-based technologies in enhancing agricultural production in Kenya's central highlands, adoption is low. Therefore, we carried out a cross-sectional household survey of 300 randomly selected smallholder farmers to determine the specific organic-based practices by farmers; and the socioeconomic factors that influence the adoption intensity of selected organic-based technologies. We used descriptive statistics to summarize the data and the Tobit regression model to evaluate the socioeconomic determinants of adoption intensity of selected organic-based technologies. We identified nine organic-based technologies that had different adoption rates among the farmers. The majority of the farmers had adopted manure (97%) and manure combined with fertilizer (92%) in Murang'a and Tharaka-Nithi, respectively. Manure was applied to the largest land in Murang'a with 31% of the cultivated land. In comparison, manure combined with fertilizer had the highest adoption intensity in Tharaka-Nithi applied to about 25% of the cultivated land. Gender, age of the household head, level of education, household size, access to external labor, training, Tropical Livestock Unit, agriculture group membership, access to credit, land cultivated, and farming experience influenced the adoption intensity of organic-based technologies among smallholder farmers. Based on the smallholder farmers' adoption behavior, this study can be used to disaggregate the farming households better in order to tailor specific organic-based soil fertility technologies solutions that meet their unique needs. One group would be those households that face specific constraints, as reflected in their low adoption rates, women-headed households and older farmers, and thus require more targeted / intensive efforts to overcome these barriers. The other group would be those households that require less focus because, when confronted with the technologies, they are more likely to adopt them easily, for example, the male-headed households. Hence, the smallholder farmers' adoption behavior, can enable policymakers to form a base for designing appropriate policies that encourage the adoption of organic-based soil fertility technology by smallholder farmers.
Introduction
Land degradation is a primary agricultural production and socioeconomic challenge across the globe. Specifically, low soil fertility is a threat to smallholder farmers' livelihood in sub-Saharan Africa (SSA) (Nigussie et al., 2017). Over half of the SSA population are smallholder farmers relying wholly on subsistence agriculture for livelihood. Smallholder rain-fed dependent farming is highly susceptible to extreme weather events such as floods, droughts, and prolonged dry spells (Cordingley et al., 2015). The fast-growing population has also led to increased arable land fragmentation for settlement space and to meet growing food demand (Mulinge et al., 2016). A quick fix has been to open up more lands for cultivation (Teklewold et al., 2013). These new lands are still susceptible to degradation due to continuous cropping coupled with little or non-use of external soil inputs, resulting in low agricultural productivity and poverty (Teklewold et al., 2013). Central Highlands of Kenya face similar challenges of land degradation, low soil fertility, decreased crop production, and increasing population prompting various research studies on integrated (organic and inorganic inputs) soil fertility-based technologies (Mucheru-Muna et al., 2010; Ngetich et al., 2014; Kiboi et al., 2019). Despite governmental and non-governmental agencies' efforts to tackle the constraints by promoting organic-based technologies, these efforts do not match the adoption levels (Mugwe et al., 2009).
Application of external soil inputs (organic and inorganics fertilizers) can enhance soil fertility in rain-fed smallholder farming systems, thus increasing crop production (Diwani et al., 2013; Kiboi et al., 2019). However, the use of inorganic fertilizers by the smallholder farmers has been low due to their high price and untimely accessibility (Mugwe et al., 2009; Ngetich et al., 2012; Liverpool-Tasie et al., 2017). Hence, locally available and more affordable soil organic inputs is an alternative remedy for declining soil fertility. Organic inputs play a crucial role in organic matter build-up and sustainability; thus, improving agricultural productivity (Omenda et al., 2019). Animal manure, green manure (Tithonia diversifolia, Calliandra Calothyrsus, Lantana camara), mulching, and agroforestry are some of the readily available organic inputs, while organic practices in the study area included crop rotation and intercropping (Mugendi et al., 1999; Mucheru-Muna et al., 2014; Kiboi et al., 2018). Several researchers have recommended using these organic inputs or integrating the organics with inorganic inputs (organic-based technologies) to enhance agricultural productivity (Place et al., 2003; Nganga et al., 2020). The use of sole organics or integration with inorganic resources bridges the gap between high-cost external inputs and the farmers' resources related challenges (Mutegi et al., 2012). Studies by Kimani et al. (2004) and Macharia et al. (2014) found that about 80% of the CHK households used animal manure and attributed its use to cattle ownership and financial constraints that hinder the accessibility of inorganic fertilizer. The organic and inorganic resources are not perfect substitutes, but both are fundamental for sustainable crop production (Odendo et al., 2011; Bargaz et al., 2018). There is a need to improve the understanding of why the intensity of adoption of organic-based technologies has been low despite the prolonged demonstrations and the reported salient benefits of their use.
The adoption of new technologies seems to offer opportunities to alleviate poverty in developing counties, thus attracting scientists to research their adoption levels by the smallholder farmers (De Graaff et al., 2008). Since the value of agricultural research innovations is realized when end users take them up, the identification of the determinants of factors that influence the adoption of improved technologies will help in promoting the effectiveness of research and extension services as well as the agricultural policy to increase agricultural productivity of the smallholder farmers (Alene et al., 2000). There have been few studies carried out to determine the rate of adoption of improved agricultural technologies in Kenya and specifically the central highlands of Kenya (Marenya and Barrett, 2007). However, very limited analysis has been done focusing on the factors influencing the intensity or extent of adoption of the technologies once they are introduced (Salasya et al., 2007). The existing literature does not provide sufficient empirical explanation as to why smallholder farmers in the Central Highlands of Kenya fail to adopt the desired level of recommended technologies.
The farmer's decision-making process on technology adoption and adoption level is understood by inquiring about the crucial factors a farmer considers in their adoption decision and the extent of adoption. However, smallholder farmers' adoption intensity of technology differs from one to another (Rauniyar and Goode, 1992). Thus, it is vital to explore the intensity of adoption of the organic-based technologies in the study area. Also, most of the studies on the adoption of organic-based technologies by smallholder farmers use a static binary framework model, which is not sufficient to explain the socioeconomics determinates of adoption intensity (Amsalu and De Graaff, 2007; Ali et al., 2012; Erb et al., 2013; Finger and El Benni, 2013). The use of the static binary framework model would lead to loss of important information (Lynne et al., 1988). Therefore, there is a need to obtain a clear understanding of smallholder farmer characteristics, farm, and institutional factors associated with the intensity of adoption (Olwande et al., 2009).
Previous studies on adoption conducted in Kenya have shown that the adoption of new agricultural technologies, which includes the adoption of low soil fertility correction practices among the smallholder farmers, has been behind the scientific and technological advancement which occasions low agricultural productivity (Okuro et al., 2002; Mugwe et al., 2009; Macharia et al., 2014). The principal reason for low adoption and low adoption intensity has been inadequate knowledge of farmers' adoption behavior toward the new technologies. Determining factors that influence the adoption intensity is essential in crafting new technologies and disseminating them as it would yield answers to questions regarding the adoption intensity and which factors condition the intensity of adoption. The analysis of the factors that determine the extent of the smallholder farmers' adoption decision to apply the low soil fertility replenishing technologies that maintain sustainable agricultural production while conserving the natural resource base is essential, realistic, sustainable, and improves crop productivity. Therefore, the purpose of this study was to identify the specific organic -based technologies applied by farmers and determine the socioeconomic factors that influence the adoption intensity of selected organic-based technologies in the CHK.
Conceptual Framework
Determinants of the decision to adopt and the extent of adoption of agricultural technologies are described as a complex process that initially includes establishing whether a farmer has adopted or not and the extent of the adoption (Meijer et al., 2015). Seminal work on adoption by Gunawan (1998) and Rogers (1995) put forward a theoretical framework that showed that farmers become aware of the new technology, which forms the first stage in the adoption process, referred to as innovation-diffusion. In the innovation-diffusion stage, farmers form an attitude about technology before deciding whether to adopt or not (Rogers, 1995). Additionally, according to Rogers (1995), new technology information is transferred from its source to the farmers through a medium such as field extension officers and media. The technology diffusion to potential adopters relies on the attributes of the individual user. The adoption behavioral framework has been used in several studies to examine the adoption of various technologies by farmers (Neupane et al., 2002; Adnan et al., 2017) and was adopted for this study, as presented in Figure 1. The influence of farmers' awareness and attitude formation is unobservable. However, what is observable is the farmer adoption behavior, whether they adopt or not, and the extent of adopting the new technology. AlthoughWhile the decision whether to adopt or not is dichotomous, the extent of adoption is a continuous variable (Choudhury and Goswami, 2013). Therefore, we assume that; (1) the farmer decideds whether to adopt or not adopt, (2) upon the decision to adopt, the farmer decides to what extent the new technology should be applied. The extent of the adoption decision will be dependent on the farmer characteristic, defined by the four dimensions Socio-economic, HH Demographics, Farm and Institutions (see Figure 1). The conceptual framework is to build a Tobit regression model that predicts how a particular farmer with given household and farm attributes decides the extent of technology adoption (Waithaka et al., 2007; Mazvimavi and Twomlow, 2009; Mugwe et al., 2009).
This study assumes that the farmer's behavior of making choices is rational. Therefore, the decision of the ith farmer to adopt an introduced technology will be motivated by the expected benefit derived from that technology. An ith farmer decides to adopt a technology that will deliver maximum utility. This theory of utility maximization is derived from the Von Neumann-Morgenstern utility function. The utility function suggested that, if a ith farmer is presented with two options (i.e., a conventional and new technologies), the ith a farmer will evaluate the expected utility of the new technology (ΣUni) in comparison to the expected utility of the conventional technology (ΣUti). When the farmer selects the new technology over conventional technology it shows a preference which is a proxy that indicate maximum utility is derived from the new technology compared to the conventional technology. This can be expressed mathematically as;
Materials and Methods
Study Area
We conducted the study in Murang'a and Tharaka-Nithi counties located in the Central Highlands of Kenya. The two counties experience a bimodal rainfall pattern that presents two cropping seasons; long rains (from March to June) and short rains (October, November, December). The predominant soil type in the two study areas is humic Nitisols (Kiboi et al., 2018). The soils are deep, well-drained, and of moderate to high fertility, but due to continuous cropping, it has lost fertility over time (Jaetzold et al., 2007). The two counties' main economic activity is rainfed subsistence agriculture characterized by mixed farming systems with low productivity (Kisaka et al., 2016). The dominant cash crops are tea (Camellia sinensis) and coffee (Coffea arabica), while annual crops which are mainly under mixed cropping system are Maize (Zea mays), beans (Phaseolus vulgaris), kales (Brassica oleracea), cowpeas (Vigna unguiculata), among others.
Murang'a County lies between Lower Highland zone one to three (LH1-3) and Upper Midland Zone one to two (UM1-2) agro-ecological zones (AEZ) on the eastern slope of the Aberdare Range with an elevation of about 400 to 1,600 m above the sea level (Jaetzold et al., 2007). The annual bimodal rainfall ranges from 850 mm to 1,500 mm, while the yearly average temperature is 23°C (Jaetzold et al., 2007). The county has an estimated population size of 1,056,640 people with an average household size of 3.3 and a population density of 419 per square kilometer [Kenya National Bureau of Statistics (KNBS), 2019].
Tharaka-Nithi County is on the eastern slopes of Mt. Kenya lying between Upper Midland Zone two (UM2) and Upper Midland Zone three (UM3) AEZ, at an altitude of 500 to 1,500 m above sea level (Jaetzold et al., 2007). The area has an annual mean temperature of 20°C with an annual bimodal rainfall ranging between 500 to 1,400 mm (Jaetzold et al., 2007). Tharaka-Nithi County has a population size of 393,177 people with an average household size of 3.6 and a population density of 153 per square kilometer [Kenya National Bureau of Statistics (KNBS), 2019].
Sampling Procedure and Data Collection
We employed a multistage sampling approach involving purposive and random sampling procedures in respondents' selection. In the first stage, we purposively selected Murang'a and Tharaka-Nithi counties, the justification being that the selected organic-based technologies have been promoted in the two sites (Kangai, 2004). In the second stage, we purposively selected Gatanga (Murang'a) and Meru South (Tharaka-Nithi) sub-counties. In contrast, in the third stage, we applied a simple random sampling technique to choose half the number of locations (administrative units) from each sub-county. From each sampled location, we randomly selected two sub-locations for the household survey.
Given that the population size varied in the sampled sub-locations, we determined the number of households interviewed proportionate to the number of households in each sub-location. We calculated the total sample size based on a 95% confidence level and a 9.8% confidence interval, leading to a total sample size of 300 smallholder farmers. For each sub-county, the sample size was 150 households. We collected the data by administering a semi-structured interview schedule to the household heads using the Open Data Kit (ODK) collect app.
Statistical Analysis
We managed the data using Microsoft excel before analyzing using STATA version 13. To characterize the smallholder farmers' households, we did the descriptive statistical analysis using measures of central tendencies, percentages, and frequency distribution. For inferential statistics, we used the Tobit regression model to estimate the socioeconomic variables that influenced the adoption intensity of organic-based technologies in the Central Highlands of Kenya.
Empirical Models
Probit and Logit regression models have been used widely to study factors that influence the adoption of different organic-based technologies in the region (e.g., Mugwe et al., 2009; Odendo et al., 2011). The two regression models are suitable when assessing the decision to adopt a technology whereby the decision to adopt is measured as a dummy variable. However, when evaluating the adoption intensity, Probit, and Logit are not appropriate econometric models because they use categorical measures to estimate adoption (Jingchao et al., 2019). To address this, a Tobit regression model was used to determine socioeconomic factors that influence the intensity of using organic-based technologies. This was motivated by Mazvimavi and Twomlow (2009) who applied Tobit in the evaluation of socioeconomic and institutional factors influencing adoption of conservation farming by vulnerable households in Zimbabwe, Choudhury and Goswami (2013) who used the same in determining the factors that influenced the area under jatropha plantation in North East India and Jingchao et al. (2019) who applied the same in the evaluation of the of coal quality-based household energy choices in rural Beijing. Unlike Probit and Logit, a Tobit regression model uses a dependent variable that is continuous, as in the case of this study. The area under the selected organic-based technologies was used as the dependent variable. Therefore, the area under each selected technology represented a censored distribution as farmers who had not adopted any organic -based soil fertility technologies assumed zero value. This means that there was a cluster of households with zero area of land without any technology at the limit. The tobit regression model is applicable in such cases because it uses data at the limit as well as the data above the limit to estimate the coefficients (McDonald and Moffitt, 1980). The empirical model of the influence of a set of explanatory variables on the use of selected organic-based technologies was specified using Equation (1).
Where Y is the % land fraction under a selected technology compared to the total cultivated; β0 is the intercept, β1, β2…βn, are the regression coefficients of independent variables (X1, X2, Xn, respectively) and εi is the error term.
Description of Variables
Dependent Variables
To measure the selected organic-based technologies' adoption intensity, we initially considered nine technologies that formed the dependent variables. Below are the details/definitions of the technologies:
(i) Agroforestry: the planting of leguminous trees and applying the leaves or other materials harvested from such trees to enrich the soil. Mercer and Pattanayak (2003) argued that the use of agroforestry is important in protecting the ecological capital, such as replenishing low fertile soils, among other environmental benefits.
(ii) Compost: decomposing organic materials before applying them in the soil. The use of composts is considered as an option for recycling organic wastes available on the farm (Paul et al., 2017). The advantage of using compost is that it improves soil structure and aeration and increases its water-holding capacity (Twarog, 2006).
(iii) Residue incorporation: burying Maize stalks in the soil after harvesting Maize. Residue incorporation is reported to increases soil organic matter content crop yield and soil aggregate stability (Lehtinen et al., 2014; Spiegel et al., 2015).
(iv) Cover cropping: the planting of legumes to cover the soil rather than for the harvest. Application of cover crop protects the soil from runoff and improves the physical and biological properties of soils and increased biodiversity (Celette et al., 2008).
(v) Crop rotation: planting crops (cereal and legume) in rotation to ensure the best-balanced nutrient supply. The inclusion of leguminous crops in the rotation potentially contributed to the nitrogen in the soil for the subsequent crops grown (Lundy et al., 2015).
(vi) Mulching: application of organic materials on the soil such that the soil has a 30% cover. Mulching enriches and protects the soil. Also, it helps provide a better growing environment for crops (Akinola and Owombo, 2012).
(vii) Intercropping defined as planting cereals with legumes. The legumes have a unique role in sustaining soil fertility through symbiotic biological N fixation (Sauer et al., 2018).
(viii) Manure: application of well-cured manure before planting. Manure is a major component of the organic-based technologies with the potential benefits of long-term improvement of low soil fertility, organic matter, and supply of nutrients, especially nitrogen (N), phosphorus (P), and potassium (K) macronutrients (Kassie et al., 2013).
(ix) Combination of manure and fertilizer: the application of a combination of well-cured manure and fertilizer in the soil. The combined use of manure and fertilizer inputs leads to positive synergistic effects and hence replenishes the soil (Mucheru-Muna et al., 2014).
The nine dependent variables were first analyzed to determine the average per cent of land under organic-based technologies compared to total cultivated land, after which, the technologies that had over 10% threshold were considered for further analysis. We defined the adoption intensity as the proportion of total cultivated land that is under a given organic-based technology; therefore, the dependent variable is bounded by the [0,1] interval.
Independent Variables
Martey et al. (2014) asserted that the efficient promotion of organic-based technologies needs the provision of information on the factors that can accelerate or decelerate uptake. Ajayi et al. (2007) insist that adoption and intensity of adoption of technologies by farmers is a process that starts with the dissemination of information, testing, and eventual adoption or continued use of the technology. Given that adoption is a dynamic process, this study hypothesized several factors influence the intensity of adoption by the ith farmer, which were based on the economic theory and empirical literature on the adoption of organic-based technologies and integration of organic technologies inorganic inputs (Ervin and Ervin, 1982; Doss and Morris, 2000; Marenya and Barrett, 2007; Muzira et al., 2011; Martey et al., 2014). These independent variables are discussed as follows;
• GENDER refers to the gender of the household head. It has been observed that gender disparity exists in access to resources, information, and access to agricultural inputs (Peterman et al., 2014). Gender disparity is important in determining adoption and/or adoption intensity. Female farmers are less likely to adopt agricultural technologies, which may be an indicator of the existence of absolute socioeconomic inequalities and barriers (Ndiritu et al., 2014).
• AGE is the age (in years) of the head of the household. Empirical studies show that compared to younger farmers, the likelihood of adoption and adoption intensity of soil replenishing technologies is lower among older farmers because they have a shorter planning range (Ervin and Ervin, 1982; Chianu et al., 2004). Hence, this study hypothesizes a negative relationship between age and the intensity of adopting organic-based technologies.
• OCCUPATION relates to the primary source of livelihood of the household. It was articulated using code one (1) for farming, two (2) for off-farm business, three (3) for employed, and four (4) for retired. Farming is the main source of livelihood that may imply that the households may be willing to invest more to conserve their soil fertility using organic-based technologies (Ogunlana, 2004). Farming as a primary occupation was hypothesized to have a positive relationship between adoption intensity and farming as the household's main occupation.
• EDUCATION measured the level of education of the head of the household. It was captured using code one (1) for no formal education, two (2) for primary education, three (3) for secondary education, and four (4) for tertiary education. Education is paramount in enhancing human capital, farm management skills and improves the ability to comprehend and adopt new agricultural technologies (Bezuayehu et al., 2002; O'Donoghue and Heanue, 2018). It is expected that farmers that have attained a higher level of education are more likely to adopt, to a great extent, organic-based technologies than less educated farmers.
• HHSIZE refers to the size of the farmer's household. Household members are the main source of farm labor in the Central Highlands of Kenya and, by extension, sub-Saharan Africa. It has been reported that labor constraints impend a farmers' ability to adopt technology to great extent technologies with a high labor requirement (Kpadonou et al., 2017; Krah et al., 2019). Application of organic-based technologies has a high labor requirement. Larger households have a higher probability of adopting organic-based technologies intensively than the small sized households. This is explained by the likelihood of larger households to have adequate labor to apply organic-based technologies.
• HIRING LABOR is the hiring of labor. Hiring labor was measured as a dummy variable where one (1) was yes and zero (0) otherwise. Hired labor is a supplementation to the already existing household labor. Mugwe et al. (2009) reported that labor positively influenced the adoption of soil infertility-correcting technology. The positive influence was, possibly, because the hired labor increased the already available labor, allowing the household to apply new technology. This study hypothesized a positive relationship between hiring labor and adoption intensity of organic-based technologies.
• FRMEXP measured the farming experience (in years) of the household head. Farming experience is an important attribute to farmers in deciding whether to adopt agricultural technology, especially in evaluating whether the technology is worthwhile to the farmer (Ntshangase et al., 2018). Farming experience is used to measure a farmer's knowledge in farming that she/he has acquired over time. We assumed that experienced farmers are more likely to have a high adoption intensity than farmers who had few years of farming experience.
• TRAINING refers to a learning process that allows acquiring knowledge, skill sharpening, concepts formation, and eventually changing farmer attitudes and behaviors toward applying agricultural technologies. Through training, farmers are enriched with knowledge on how to apply technology to realize optimal benefits (Macharia et al., 2014). We hypothesized that training on organic-based technology would have a positive influence on the adoption intensity.
• HERDSIZE measured the number of mature cattle owned by the household. Cattle is the main source of animal manure in the Central Highlands of Kenya (Macharia et al., 2014; Nganga et al., 2020). We hypothesize that households with a large herd size will have a higher probability of adopting organic-based technologies intensively than households with a small herd size. This is explained by the fact that more cattle mean a large quantity of manure will be available for the farm.
• LANDCULT refers to the size of land under cultivation measured in hectares. Past research has shown that there has been a positive effect of farm size on farmer adoption behavior. For example, Kassie et al. (2011) reported a positive and significant relationship between farm size and the adoption of technologies. The study showed that the farm size increased, the likelihood of adopting agricultural technologies increased.
• TITLEDEED is title deed ownership. Title deed ownership is a proxy for land security. Land security is divided into secure farms with legal documents (title deed) and insecure farms that do not have a title deed as a guarantee of land rights. Mulolwa et al. (2016) assert that land title deed documents are issued as an indicator of full property rights on the land. Secure land-use rights were reported to positively influence the adoption of agricultural technology in Liberia (Fouladbash and Currie, 2015). We hypothesized that title deed ownership would positively affect the adoption intensity of organic-based technologies.
• AGRIGRP measured agricultural group membership. An agricultural group is an organization that is owned and controlled by farmers. It was articulated as zero (0), not a member of an agricultural group, and one (1) member of an agricultural group. The agricultural group is the main channel used to deliver extension services by both governmental and non-governmental organizations. Membership of the agricultural group was reported to positively influence the adoption of technologies because the farmers shared their experiences and challenges, which fostered a positive way forward (Murage et al., 2019).
• CREDITACCESS referred to the farmers' access to agricultural credit. Access to agricultural credit took a value of one (1) if the household obtained agricultural credit and zero (0) otherwise. Credit has been reported to be important in the promotion of agricultural technology (Mugwe et al., 2009). Without access to credit, the majority of the farmers cannot afford to implement agricultural technologies. We hypothesized a positive relationship between adoption intensity and agricultural credit access.
A detailed description, definition, and expected direction of the explanatory variables are presented in Table 1.
Results
Demographic, Socioeconomic, and Farm Characteristics
Among the interviewed households in Murang'a, 55% were male-headed, with the majority (88%) being full-time farmers (Table 2). The respondents that had attained primary school education were 48%, while 9.3% had no formal education. Seventy-six per cent of the respondents were not trained on organic-based technologies, while 52% of the household hired labor. Seventy-two per cent of the households owned a title deed, whereas 25.3% were members of agricultural groups. The respondents that reported lack of credit access were 42% in Murang'a County.
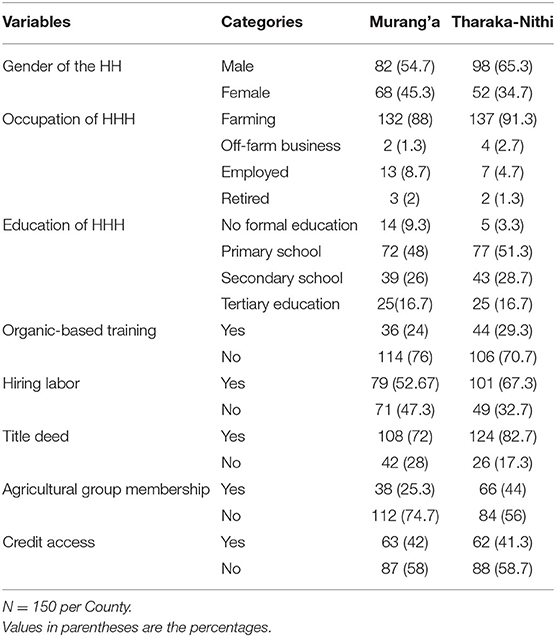
Table 2. Descriptive summary statistics of the sampled smallholder farmers in and Tharaka-Nithi for categorical variables.
In Tharaka-Nithi County, 65.3% of the households were male-headed, with a majority (91.3%) of the respondents being full-time farmers (Table 2). The least (1.3%) of the respondents were retirees; thus, their primary source of livelihood was a pension. Fifty-one per cent of the respondents had attained primary education, while 3.3% had no formal education. The highest proportion of farmers (67.3%) in Tharaka-Nithi hired labor. Results on hired labor indicated that the majority of the households hired labor. The majority of the respondents (82.7%) had a land title deed. Most of the respondents (56%) were not members of an agricultural group, while 41.3% reported that they did not have access to credit.
The average age of the household heads' in was 54 years, with a mean household size of 3.79 (Table 3). The average years of farming experience were 25 years, while the mean cultivated land was 0.49 ha. The tropical livestock unit (TLU) was 35 units. In Tharaka-Nithi, the mean age of the household head was 51 years, and the average household size of 4.23. The mean farming experience was 24.11 years and an average cultivated land of 0.46 ha. The tropical livestock unit (TLU) was 18.12 units.
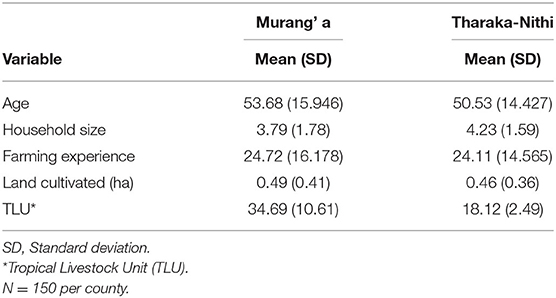
Table 3. Descriptive summary statistics of the sampled smallholder farmers in Murang' a and Tharaka-Nithi for continuous variables.
Adoption of Selected Organic-Based Technologies
Adopters and Non-adopters
In Murang'a, 8.7% of the households were agroforestry adopters, 14.7% were compost adopters, 21.3% residue incorporation, 38.7% cover crop, 42.7% crop rotation, 60.7% mulch, 72% intercrop, 96.7% manure, and 81.3% were manure +fertilizer adopters (Table 4). In Tharaka-Nithi, adopters of agroforestry were 26.7%, 11.3% compost, 11.3% residue incorporation, 86.7% cover crop, 68% crop rotation, 92.7% mulch, 48% intercrop, 90% manure, and 92% were manure +fertilizer adopters (Table 4).
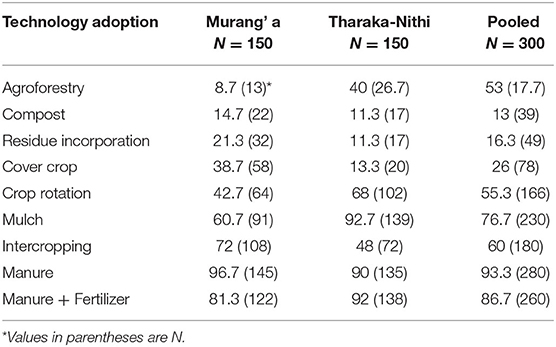
Table 4. Adoption percentages of selected organic-based technologies in Murang'a and Tharaka-Nithi counties.
The adopters of agroforestry were few (17.7%) in the two counties than non-adopters (82.3%) (Table 4). Adopters of compost were 13%, while 87% were non-adopters. Residue incorporation was adopted 16.3 with 83.7% non-adopters. The cover crop was adopted by 26%, with 74% non-adopters. Crop rotation was adopted by 55.3% with 44.7% non-adopters. Mulch adopters were 76.7%, while non-adopters were 23.3%. Intercropping adopters were 60% and 40% non-adopters. Manure adopters were 93.3%, while 6.7% were non-adopters. Lastly, manure +fertilizer adopters were 86.7% and 13.3 non-adopters (Table 4).
Land Under Organic-Based Technologies
Overall, sole manure and manure + fertilizer were used on the largest land sizes in the two counties (Table 5). This was followed by intercropping at 0.17 ha in Murang'a and 0.25 ha under mulch (Table 5). Compost was applied in the least land size in Murang'a and Tharaka-Nithi, 0.03, and 0.02 ha, respectively (Table 5).
The minimum possible percentage of land under a given technology was 0%, while the maximum possible land allocated to any given technology was 100%. Where the technology was not applied at all, a 0% allocation was reported, while 100% was reported where technology was fully applied. In Murang'a and Tharaka-Nithi, the maximum percentage of land under manure compared to total cultivated land was 100% (Table 6). Manure followed by manure+fertilizer had the highest per cent allocation in Murang'a, 31.26, and 23.39%, respectively. In Tharaka-Nithi, manure+fertilizer and manure had the highest percentage of land allocated, at 24.84 and 24.34%, respectively. In Murang's agroforestry, a and cover crop in Tharaka-Nithi had the smallest allocation of 1.64 and 1.61%, respectively.
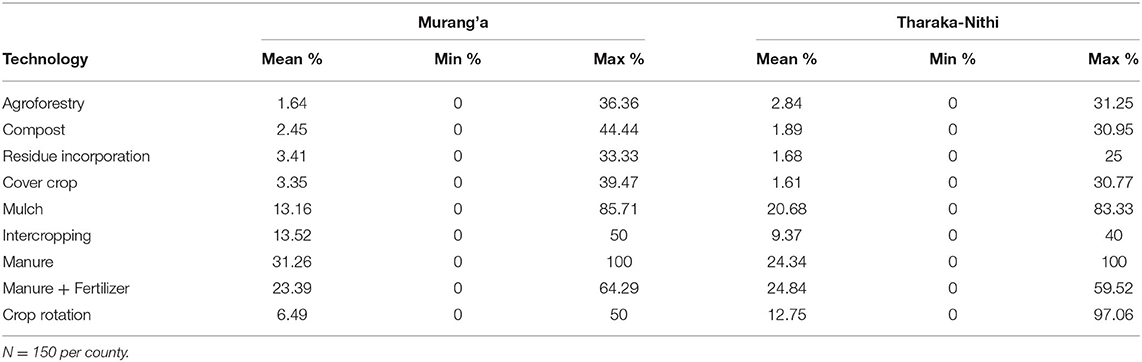
Table 6. Minimum, maximum, and the average per cent of land under organic-based technologies in relationship to total cultivated land in Murang' a and Tharaka-Nithi.
Socioeconomic Factors Influencing Adoption Intensity of Mulch, Intercropping, Manure, Manure Plus Fertilizer and Crop Rotation
In Murang'a, gender of the household head had a significant positive influence on the adoption intensity of manure + fertilizer (β = 0.132, p = 0.05) (Table 7). Age of the household head had a negative influence on the adoption intensity of intercropping (β = −0.004, p = 0.038) and manure + fertilizer (β = −0.004, p = 0.074). The level of education attained by the household head positively influenced the adoption intensity of manure + fertilizer (β = 0.049, p = 0.041). The household size had a positive influence on the adoption intensity of mulch (β = 0.024, p = 0.029), intercropping (β = 0.024, p = 0.027), manure (β = 0.026, p = 0.095) and manure + fertilizer (β = 0.035, p = 0.029). Access to external labor influenced positively the adoption intensity of mulch (β = 0.080, p = 0.043), intercropping (β = 0.225, p = 0.039), manure (β = 0.110, p = 0.029), manure + fertilizer (β = 0.084, p = 0.026) in Murang'a County (Table 7).
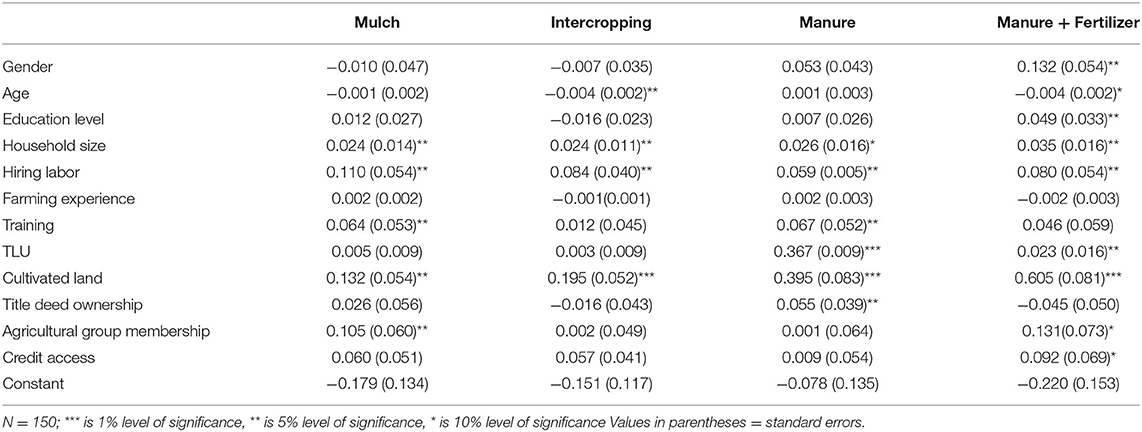
Table 7. Parameter estimates of the Tobit model for the determinants of adoption intensity of the selected organic-based technologies in Murang' a County.
Training of the household head had a positive influence on the adoption intensity of mulch (β= 0.064, p = 0.042) and manure (β = 0.067, p = 0.050) in Murang'a (Table 7). Tropical Livestock Unit (TLU) had a positive influence on the adoption intensity of manure (β = 0.367, p = 0.0001) and manure + fertilizer (β = 0.023, p = 0.050) (Table 7). Total land under cultivation had a positive influence on the adoption intensity of mulching (β = 0.132, p = 0.017), intercropping (β = 0.195, p = 0.0001), manure (β = 0.395, p = 0.0001), and manure + fertilizer (β = 0.605, p = 0.0001). Title deed ownership had a significant and positive influence on the adoption intensity of manure (β = 0.055, p = 0.046). Household membership to an agricultural group positively affected the adoption intensity of mulch (β = 0.105, p = 0.083) and manure + fertilizer (β = 0.131, p = 0.074) (Table 7). Household access to credit affected positively and significantly the adoption intensity of manure + fertilizer (β = 0.092, p = 0.098).
In Tharaka-Nithi County, the gender of the household head influenced significantly and positively the adoption intensity of intercropping (β = 0.102, p = 0.044) (Table 8). The household head's education level significantly influenced the adoption intensity of manure + fertilizer (β = 0.058, p = 0.019). The household's size was significant and it positively influenced the adoption intensity of mulch (β = 0.132, p = 0.000) and manure (β = 0.134, p = 0.048). Household access to external labor was significant and positively influenced the adoption of mulch (β = 0.066, p = 0.036), and manure (β = 0.057, p = 0.066). Years of household head experience had a positive and significant influence on the adoption intensity of manure + fertilizer (β = 0.006, p = 0.006) (Table 8).
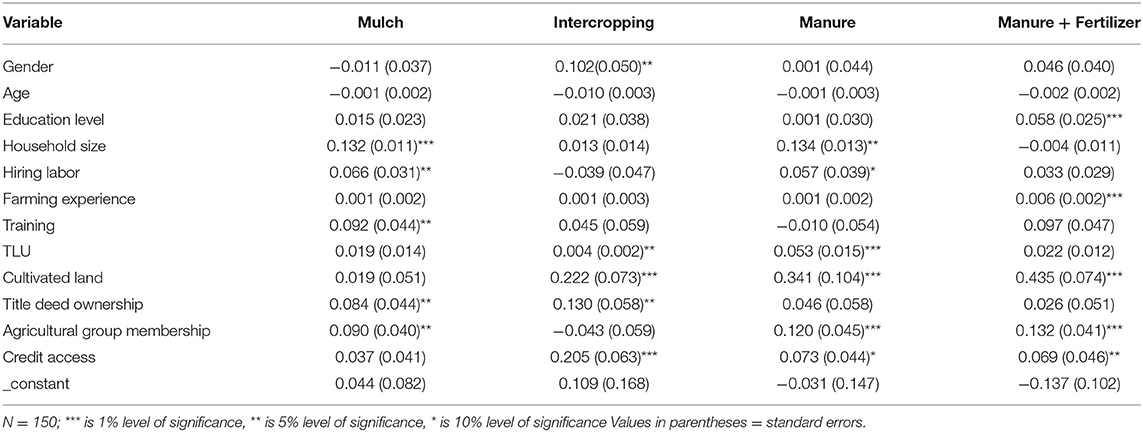
Table 8. Parameter estimates of the Tobit model for the determinants of adoption intensity of the selected organic-based technologies in Tharaka-Nithi County.
Training of the household head on organic-based technology, positively and significantly affected the adoption intensity of mulch (β = 0.092, p = 0.037), and manure + fertilizer (β = 0.097, p = 0.042) (Table 8). The TLU owned by the household was significant and positively influenced the adoption intensity of intercropping (β = 0.004, p = 0.034), manure (β = 0.053, p = 0.0001), and manure + fertilizer (β = 0.022, p = 0.077). Land under cultivation was positively significant in influencing the adoption intensity of intercropping (β = 0.222, p = 0.003), manure (β = 0.341, p = 0.001), manure+ fertilizer (β = 0.435, p = 0.000) in Tharaka-Nithi.
Household title deed ownership positively and significantly influenced the adoption intensity of mulch (β = 0.084, p = 0.050) and intercropping (β = 0.138, p = 0.026) (Table 8). Household head agricultural group membership affected positively and significantly the adoption intensity of mulch (β = 0.090, p = 0.027), manure (β = 0.120, p = 0.009), and manure + fertilizer (β = 0.321, p = 0.001) (Table 8). Household access to credit was an important factor that positively and significantly affected the adoption intensity of intercropping (β = 0.205, p = 0.001), manure (β = 0.073, p = 0.094) and manure + fertilizer (β = 0.069, p = 0.028) (Table 8).
Discussion
Demographic, Socioeconomic, and Farm Characteristics
The majority of the households in the two counties were male-headed. This finding corroborates with a study conducted in the Central Highlands of Kenya by Mugwe et al. (2009). However, in the Central Highlands of Kenya, men are dominant household heads who make nearly all farm-related decisions (Mugwe et al., 2009; Macharia et al., 2014). Therefore, the possibility of having many males than female-headed households in this region is high. Many household heads in Murang'a and Tharaka-Nithi were full-time farmers with few household heads being retirees. The findings agree with Kisaka et al. (2016), who reported that farming is the main livelihood for most households in the Central Highlands of Kenya. Household head practicing full-time farming indicates how interested the household head may be to try new technology and ultimately adopt the technology (Murage et al., 2019).
Education plays a vital role in farmer's capacity to understand and obtain information from different sources (Adolwa et al., 2012). Many of the respondents in both counties had attained primary education, with fewer having no formal education. It is more likely that farmers with a low level or no education may find comprehending information daunting (Adolwa et al., 2012). We attributed the high level of primary education attainment to the high number of primary schools distributed strategically and in proximity to the respondents' household location (Ministry of Education Science and Technology, 2014).
Training is a capacity-building approach that aims to empower farmers with specific knowledge (Barrios et al., 2006). Fewer respondents in Murang'a and Tharaka-Nithi were trained on organic-based technologies. We ascribed the low number of trained farmers to the cost of training. The cost to farmers could be either in the form of opportunity cost or financial costs, which may be a significant hurdle that hinders them from attending the training (Fischer and Qaim, 2012). Given that the smallholding farming system in Murang'a and Tharaka-Nithi was largely unmechanised, manual labor hiring is predominant. The reliance on manual labor leads to a higher number of households hiring labor to meet the labor demand (Wanjiku et al., 2007). Additionally, the capital required to buy or rent machines to use on the farm is high and often unavailable to smallholder farmers (Johansen et al., 2012).
Title deed ownership is a crucial indicator of land tenure rights because it signifies land ownership security (Nhemachena and Hassan, 2007). Many households in the two counties had title deeds. Ownership of a title deed also provokes long term investment in the land. It is an essential pointer of whether or not households can adopt new technologies (Nhemachena and Hassan, 2007). The results showed that few households belonged to an agricultural group. We attributed the findings on agricultural group membership to the fact that the farmer's decision to be a member of a farming group depends on the expected fixed cost and benefits of being a member (Fischer and Qaim, 2012). Therefore, the farmers may have considered the fixed cost of joining the agricultural group as higher than the benefits received from being a member (Fischer and Qaim, 2012). Access to credit is a significant variable in determining a household's probability of adopting technology because many technologies have a higher capital requirement (Mal et al., 2013). Respondents reported that they had low credit access in both counties. We attributed the low access to credit to the high interested charged on commercial loans (Irungu, 2013). Additionally, lack of collateral, low profitability, and macroeconomic uncertainty occasions banks to perceive the agricultural sector as a high-risk consumer (Irungu, 2013). Lack of access to credit means market failure that prompts for intervention from the government and other stakeholders in agriculture (Beyene et al., 2019).
The households' mean age in the study area implied that the household heads were still in their labor active years. This finding corroborates with a study by Takusewanya et al. (2018), which reported that household heads in their study area had similar ages and were still active. This finding contrast with a study by Bala et al. (2011) that reported younger household heads than the average age reported in this study. Thus, the CHK farmers are still in their active labor years can participate in farming (Ramaekers et al., 2013). The average household size in Murang'a and Tharaka-Nithi showed that in the Central Highlands of Kenya, the households have family labor at their disposal and the ability to work off-farm (Kidane et al., 2005).
Additionally, this finding corroborates with Mignouna et al. (2011), who reported a similar household size in their study area who were vital sources of labor. However, this finding differs from a study conducted by Murage et al. (2019) that found smaller household sizes in the Central Highlands of Kenya. The results of a high number of farming experiences in both counties can be attributed to the farming culture of the communities in the Central Highlands of Kenya (Macharia et al., 2014). In these communities, farming is the primary source of livelihood, and therefore, the average household heads amass many years of farming experience (Muatha et al., 2017; Murage et al., 2019).
Results showed that the total land cultivated was small in both counties. This finding may be co-attributed to land fragmentation that has been taking place in the smallholder setting in most parts of SSA (Mulinge et al., 2016). The increased fragmentation of arable land to provide settlement space and to meet the ever-increasing food demand is a serious impendent in the agricultural production process (Curran-Cournane et al., 2016; Bacior and Prus, 2018). The tropical livestock unit in both counties indicated that the number of livestock in the two counties was high. Murang'a had an intensive livestock system characterized by the housing of animals, a high level of animal husbandry, and an optimum feed plan. However, Tharaka-Nithi had an extensive livestock system marked by crosses of indigenous breeds on a pasture-based production system to limited housing of livestock. This explains the variation in the number of livestock reported in Murang'a and Tharaka-Nithi counties.
Adoption of Selected Organic-Based Technologies
Adopters and Non-adopters
Manure and combination of manure +fertilizer were the most adopted technologies in Murang'a and Tharaka-Nithi combined. The high rate of adoption was attributed to the number of livestock owned by the households in the study area, as indicated by the TLU in Table 3 above. A study by Macharia et al. (2014) in the Central Highlands of Kenya revealed that the majority of the households keep cattle. Cattle ownership among households enhances the adoption of manure and manure + fertilizer (Mugwe et al., 2009). Compost was the least adopted technology of the selected organic-based technologies in Tharaka-Nithi. The low adoption may be explained by the high labor and capital requirement and the intensive process in preparing the compost (Vigneswaran et al., 2016). Crop rotation and intercropping were averagely adopted in Murang'a and Tharaka-Nithi combined. The average uptake of crop rotation and intercrop technologies can be attributed to legumes' accessibility in the study area (Murage et al., 2019).
Land Under Organic-Based Technologies
Results show that large land sizes were under manure and manure + fertilizer both in Murang'a and Tharaka-Nithi. This meant that the technologies might have been readily available for farmers to use them without many challenges. Previous studies in the Central Highlands of Kenya report that manure is readily available because many households own cattle (Kimani et al., 2004; Mugwe et al., 2009; Macharia et al., 2014). Small land sizes were dedicated to compost in Murang'a and Tharaka-Nithi. This finding was ascribed to the low adoption of the technology, as indicated in Table 4. The process of making compost is rigorous, and most households are not ready to undertake it. The land dedicated to agroforestry in Murang'a may indicate that households in Murang'a do not perceive it as an accessible technology in soil conversation. This was explained by the long term investment period required before farmers experience the benefits from agroforestry.
The minimum possible land allocation for selected organic-based technology was zero (Table 6). This meant that some households did not adopt any technology completely. On the other hand, the maximum possible percentage of land allocation for selected organic-based technology was a 100%. It implied that some households had applied some organic-based technologies fully on their cultivated land. Manure followed by manure + fertilizer had a high percentage of land allocated in Murang'a and Tharaka-Nithi. This meant that farmers adopted manure and manure + fertilizer on large pieces of land compared to other technologies. The plausible explanation of this pattern is that farmers are rational decision-makers, and they adopt technologies that are easy to apply and whose resources are not strenuous to obtain (De-Miguel et al., 2019).
The percentage of land dedicated to agroforestry in contrast to the total cultivated land was small in Murang'a. Agroforestry is a long term investment that may take time for the farmers to benefit from it (Simelton et al., 2017). The low adoption in agroforestry may be attributed to the small pieces of land available to the smallholder farmers. A study by Nyaga et al. (2015) in Rift Valley Kenya associated low adoption of agroforestry to small land sizes. In Tharaka-Nithi, the cover crop had the least percentage allocation compared to other organic-based technologies. The low allocation of land under cover crops may be because cover cropping is still not very popular among farmers (Alonso-Ayuso et al., 2018).
Socioeconomic Factors Influencing Adoption Intensity of Selected Organic-Based Technologies
The gender of the household head positively influenced the adoption of organic-based technologies (Tables 7, 8). This finding meant that male-headed households were more likely to adopt a higher number of organic-based technologies than female-headed households. We ascribed this to a male-dominated hierarchical cultural set-up whereby males tend to benefit more than females (Bradshaw, 2013). This trend is seen in small -scale farming systems in SSA, where male-headed households are more likely to have more resources to facilitate the adoption of organic-based technologies than female-headed households. The unequal access to resources can hinder women from adopting organic-based technologies. This corroborates with the findings of Agegnehu and Amede (2017).
The household head's age had a negative effect on the adoption of organic-based technologies, especially of compost and crop rotation. This meant that households headed by older people are less likely to have a high adoption intensity of the selected organic-based technologies compared to the younger household head. As household heads advance in age, their risk aversion abilities increase, unlike younger farmers with low-risk aversion (Odendo et al., 2010). Therefore, elderly farmers are less likely to adopt new technologies (Odendo et al., 2010). Additionally, the age of the household head indicates their capacity to work (Anjichi et al., 2007), implying that as the age of the farmer advances, they are less likely to participate in strenuous farming activities such as composting, and this reduces the possibility of adopting labor-intensive technologies (Odendo et al., 2010).
In contrast, other studies, for example, Abdulai and Huffman (2004), reported that elderly household heads adopted new agricultural technologies compared to younger household heads. This was attributed to the heavy capital requirement; older households may have accumulated more capital over time (Abdulai and Huffman, 2004). Further, the credit institution may prefer the elderly than the young households head.
Many years of farming experience exposes farmers to more knowledge, and they are more likely to adopt (Edmeades et al., 2008). Years of farming experience had a significant positive influence on the adoption intensity of organic-based technologies. As the years of farming experience increases, the household head was more likely to have a higher adoption intensity. It is expected that a long farming experience would increase the likelihood of a farmer to predict the success of technology correctly. This finding is because farmers face complex constraints, and as they delve into solving the low soil fertility problem, they gain more experience. Farming experience enhances the farmer to decide which technologies meet his/her complex constraints; thus, she/he adopts those technologies (Shiferaw et al., 2009). A study by Edmeades et al. (2008) reported that farmers with many years of experience were more likely to adopt improved agricultural technologies in Uganda.
Household size had a significant positive effect on the adoption intensity of selected organic-based technologies, suggesting that larger households are more likely to have a higher adoption intensity than smaller households. Household size is used as a proxy for labor availability in the household (Staal et al., 2002). The use of organic-based technologies has high labor demand; thus, larger households can supply family labor, and as a result, they can adopt organic-based technologies (Kalinda et al., 2000). Thuo et al. (2011) found out that the household's size increases the probability of adoption of technologies. Access to external labor had a significant positive influence on the adoption intensity of selected organic-based technologies. This suggested that households that hired labor were more likely to have a greater adoption rate than households who did not hire labor. We attributed this to the fact that external labor employment promotes efficient utilization of resources in the farm (Mburu et al., 2007). Farmers may employ labor to supplement the already available family labor. Additionally, use external labor signals farmers' willingness to invest in long -term strategies that replenish the soil (Udayakumara et al., 2010). Therefore, farmers will adopt and invest in greater extends, the technologies they perceive to be worthwhile with short-term income (Corbera and Brown, 2010).
Education is broadly defined as the process of learning and is often used as a proxy of human capital (Asfaw and Admassie, 2004). For example, Udayakumara et al. (2010) suggested that education creates awareness of soil conversation technologies. Additionally, farmers with high education levels are more likely to apply new technologies in an efficient way (Asfaw and Admassie, 2004). We found a significant positive relationship between the household head's education level and the intensity of adoption of organic-based technologies (Tables 7, 8). Household heads who had attained a higher level of education were more likely to have a higher adopt intensity than the household heads who had a lower level of education. This underpins that education indicates the capacity to make adoption decisions. The household training coefficient was positive and significant in influencing the extent of the adoption of selected organic-based technologies. This result suggested that household heads trained on organic-based technologies were more likely to adopt organic-based technologies to a greater extent than the non-trained household heads. Training is a critical component in instilling skills of low soil fertility correction (Asfaw and Admassie, 2004). A study conducted in the Central Highlands of Kenya by Macharia et al. (2014) reported farmers who had higher levels of knowledge were more likely to adopt organic ways of replenishing soils than household heads who had a moderate or low level of knowledge.
Agricultural group membership positively influenced the adoption intensity of selected organic-based technologies (Tables 7, 8). This meant that household heads that were members of an agricultural group were more likely to have a higher adoption intensity. Participation in a group is a proxy of knowledge acquisition (Macharia et al., 2014). Further, farmers in an agricultural group have higher bargaining power in purchasing inputs such as mineral fertilizer that are unaffordable to individual farmers (Macharia et al., 2014). The positive effect of group membership on the extent of adoption may be further explained by the incentives provided to farmers when buying inputs such as inorganic fertilizers. Hence, they are more likely to adopt a combination of manure and fertilizer compared to the household heads that are not in agricultural groups. This is no similar incentive to farmers who are not members of an agricultural group (Njuki et al., 2008).
As anticipated, the coefficient of land under cultivation had a significant positive influence on the adoption intensity of selected organic-based technologies (Tables 7, 8). This finding implied that as the land under cultivation increases, households are more likely to put larger pieces of land under the selected organic-based technologies than households with smaller pieces of land under cultivation. The land is a proxy of many factors in agriculture, such as production factor, capacity to carry risk, size of wealth, and collateral in access to credit (Enki et al., 2001). The income obtained from the framing activity helps hire labor to apply organic-based technologies (Enki et al., 2001). This finding agrees with what Danso-Abbeam et al. (2017) reported. According to Nigussie et al. (2017), land under cultivation is a measure of available economic resources and the willingness to adopt new technology.
Land title deed ownership had a positive and significant influence on the adoption intensity, meaning that households with title deed were more likely to have a higher adoption intensity than households who did not possess a title deed (Tables 7, 8). Title deed is an imperative proxy of security and land rights identified as a key component that encourages long term investments on the soil (Adimassu et al., 2013). Therefore, farmers who have a secure tenure system will have an incentive to invest in long term soil replenishment strategies such as organic-based technologies (Adimassu et al., 2016).
Access to credit had a positive influence on the adoption intensity of selected organic-based technologies (Tables 7, 8). This finding is ascribed to the fact that access to credit enhances the households' purchasing power (Waithaka et al., 2007). Households with a higher purchasing power can purchase inputs such as tree seedlings, inorganic fertilizer, and manure. Also, high purchasing power empowers households to use animal feed for livestock and instead utilize Maize stalk for soil correction. TLU had a positive and significant effect on the extent of adopting the selected organic-based technologies (Tables 7, 8). This suggested that households that owned a higher TLU were more likely to adopt selected organic-based technologies to a greater extent compared to households that had a lower TLU. This finding is explained by the fact that cattle are an important source of animal manure. Therefore, it is expected that as the number of cattle per household increases, then the adoption intensity of cattle manure and other organic-based technologies are likely to increase. However, the challenge attributed to TLU is the insufficiency of manure in the Central Highlands of Kenya that was reported by Mugwe et al. (2009).
Conclusion
The study determined the specific organic-based technologies used by farmers and the socioeconomic factors that influenced the adoption intensity of mulching, intercropping, manure and manure plus fertilizer in the central highlands of Kenya. We found that nine organic-based techniques were used in the central highlands of Kenya. Out of the nine technologies, manure and manure combined with fertilizer were adopted by the majority of households in Murang'a and Tharaka-Nithi, respectively. On the other hand, agroforestry, compost, and residue incorporation in Murang'a and compost, residue incorporation and cover crop in Tharaka-Nithi were poorly adopted.
We also noted that manure and manure + fertilizer had the highest adoption intensity in Murang'a and Tharaka-Nithi, respectively, while agroforestry in Murang'a and cover crop in Tharaka-Nithi had the lowest adoption intensity as indicated by the land allocated to the technologies. Results also revealed that socioeconomic determinants of intensity of adoption were gender, age of the household head, level of education, household size, access to external labor, training, TLU, agriculture group membership, access to credit, land cultivated, title deed ownership and farming experience. Gender influenced positively the adoption intensity of manure + fertilizer, which implied women headed households were disadvantaged. Age of the household head had a negative effect on the adoption intensity of organic-based technologies, especially compost and crop rotation meaning that older household heads will have low adoption intensity. The education level had a significant positive relationship with the intensity of adoption. Household size had a significant positive effect on the adoption intensity of selected organic-based technologies, suggesting that larger households are more likely to have a higher adoption intensity than smaller households. Access to external labor had a significant positive influence on the adoption intensity of selected organic-based technologies. Household training was positive and significant in influencing the extent of adoption of selected organic-based technologies. Tropical Livestock Unit had a positive and significant effect on the extent of adoption. Agricultural group membership positively influenced the adoption intensity. Access to credit had a positive influence on adoption. Land under cultivation had a significant positive influence on the adoption intensity. Land title deed ownership had a positive and significant influence on the adoption intensity. Years of farming experience had a significant positive influence on the adoption intensity of organic-based technologies. These findings implies that empowering women households, training of households on the technologies, encouraging households to join agricultural groups, improved accessibility to agricultural credit, focusing on land use rights, and targeting young farmers would play a major role in promoting high adoption intensity.
Based on the smallholder farmers' adoption behavior, this study can be used to disaggregate the farming households better in order to tailor specific organic-based soil fertility technologies solutions that meet their unique needs. One group would be those households that face specific constraints, as reflected in their low adoption rates, and thus require more targeted / intensive efforts to overcome these barriers. The other group would be those households that require less focus because, when confronted with the technologies, they are more likely to adopt them easily. Hence, the smallholder farmers' adoption behavior, can enable policymakers to form a base for designing appropriate policies that encourage the adoption of organic-based soil fertility technology by smallholder farmers.
Data Availability Statement
The original contributions presented in the study are included in the article/supplementary materials, further inquiries can be directed to the corresponding author/s.
Author Contributions
GM: formal analysis, data curation, wrote-original draft preparation, conceptualization. MK, EB, and JM: wrote-reviewing and editing. AM: supervision, funding acquisition. GN: funding acquisition, wrote-reviewing and editing. FN: wrote-reviewing and editing, supervision, funding acquisition, data curation, conceptualization. All authors contributed to the article and approved the submitted version.
Conflict of Interest
The authors declare that the research was conducted in the absence of any commercial or financial relationships that could be construed as a potential conflict of interest.
Acknowledgments
The authors acknowledge the Swiss Agency for Development and Cooperation (SDC) and Swiss National Science Foundation (SNSF) in the Swiss Program for Research on Global Issues for Development (r4d program) and Research Institute of Organic Agriculture (FiBL), Switzerland for the support provided through the Organic Resource Management for Soil Fertility project.
References
Abdulai, A., and Huffman, W. E. (2004). The diffusion of new agricultural technologies: the case of crossbred-cow technology in Tanzania. Am. J. Agric. Econ. 27, 247–270. doi: 10.1111/j.1467-8276.2005.00753.x
Adimassu, Z., Kessler, A., Yirga, C., and Stroosnijder, L. (2013). Farmers' perceptions of land degradation and their investments in land management: a case study in the central rift valley of Ethiopia. Environ. Manage. 51, 989–998. doi: 10.1007/s00267-013-0030-z
Adimassu, Z., Langan, S., and Johnston, R. (2016). Understanding determinants of farmers' investments in sustainable land management practices in Ethiopia: review and synthesis. Environ. Dev. Sustain. 18, 1005–1023. doi: 10.1007/s10668-015-9683-5
Adnan, N., Nordin, S. M., and bin Abu Bakar, Z. (2017). Understanding and facilitating sustainable agricultural practice: a comprehensive analysis of adoption behaviour among Malaysian paddy farmers. Land Use Policy 68, 372–382. doi: 10.1016/j.landusepol.2017.07.046
Adolwa, I. S., Okoth, P. F., Mulwa, R. M., Esilaba, A. O., Mairura, F. S., and Nambiro, E. (2012). Analysis of communication and dissemination channels influencing the adoption of integrated soil fertility management in western Kenya. J. Agric. Educ. Extension 18, 71–86. doi: 10.1080/1389224X.2012.638782
Agegnehu, G., and Amede, T. (2017). Integrated soil fertility and plant nutrient management in tropical agro-ecosystems: a review. Pedosphere 27, 662–680. doi: 10.1016/S1002-0160(17)60382-5
Ajayi, O. C., Akinnifesi, F. K., Sileshi, G., and Chakeredza, S. (2007). Adoption of renewable soil fertility replenishment technologies in the southern African region: lessons learnt and the way forward. Nat. Res. Forum 31, 306–317. doi: 10.1111/j.1477-8947.2007.00163.x
Akinola, A., and Owombo, P. (2012). Economic analysis of adoption of mulching technology in yam production in Osun State, Nigeria. Int. J. Agric. For. 2, 1–6. doi: 10.5923/j.ijaf.20120201.01
Alene, A. D., Poonyth, D., and Hassan, R. M. (2000). Determinants of adoption and intensity of use of improved maize varieties in the central highlands of Ethiopia: a tobit analysis. Agrekon 39, 633–643. doi: 10.1080/03031853.2000.9523679
Ali, S., McCann, L., and Allspach, J. (2012). Manure transfers in the midwest and factors affecting adoption of manure testing. J. Agric. Appl. Econ. 44, 533–548. doi: 10.1017/S1074070800024093
Alonso-Ayuso, M., Quemada, M., Vanclooster, M., Ruiz-Ramos, M., Rodriguez, A., and Gabriel, J. L. (2018). Assessing cover crop management under actual and climate change conditions. Sci. Total Environ. 621, 1330–1341. doi: 10.1016/j.scitotenv.2017.10.095
Amsalu, A., and De Graaff, J. (2007). Determinants of adoption and continued use of stone terraces for soil and water conservation in an Ethiopian highland watershed. Ecol. Econ. 61, 294–302. doi: 10.1016/j.ecolecon.2006.01.014
Anjichi, V. E., Mauyo, L. W., and Kipsat, M. J. (2007). “The effect of socioeconomic factors on a farmer's decision to adopt farm soil conservation measures. An application of multivariate logistic analysis in Butere/Mumias District, Kenya,” in: Advances in Integrated Soil Fertility Management in sub-Saharan Africa: Challenges and Opportunities, eds A. Bationo, B. Waswa, J. Kihara, J. Kimetu (Dordrecht: Springer), 915–920. doi: 10.1007/978-1-4020-5760-1_87
Asfaw, A., and Admassie, A. (2004). The role of education on the adoption of chemical fertiliser under different socioeconomic environments in Ethiopia. Agric. Econ. 30, 215–228. doi: 10.1111/j.1574-0862.2004.tb00190.x
Bacior, S., and Prus, B. (2018). Infrastructure development and its influence on agricultural land and regional sustainable development. Ecol. Inform. 44, 82–93. doi: 10.1016/j.ecoinf.2018.02.001
Bala, A., Osunde, A. O., and Odofin, A. J. (2011). “Organic matter utilisation and the determinants of organic manure use by farmers in the Guinea savanna zone of Nigeria,” in Innovations as Key to the Green Revolution in Africa, eds A. Bationo, B. Waswa, J. Okeyo, F. Maina, and J. Kihara (Springer), 965–974. doi: 10.1007/978-90-481-2543-2_97
Bargaz, A., Lyamlouli, K., Chtouki, M., Zeroual, Y., and Dhiba, D. (2018). Soil microbial resources for improving fertilizers efficiency in an integrated plant nutrient management system. Front. Microbiol. 9:1606. doi: 10.3389/fmicb.2018.01606
Barrios, E., Delve, R. J., Bekunda, M., Mowo, J., Agunda, J., Ramisch, J., et al. (2006). Indicators of soil quality: a South–South development of a methodological guide for linking local and technical knowledge. Geoderma 135, 248–259. doi: 10.1016/j.geoderma.2005.12.007
Beyene, A. D., Mekonnen, A., Randall, B., and Deribe, R. (2019). Household level determinants of agroforestry practices Adoption in rural Ethiopia. For. Trees Livelihoods 28, 194–213. doi: 10.1080/14728028.2019.1620137
Bezuayehu, T., Gezahegn, A., Yigezu, A., Jabbar, M. A., and Paulos, D. (2002). Nature and causes of land degradation in the Oromiya region: a review (Socioeconomics and Policy Research Working Paper 36). Nairobi: ILRI (International Livestock Research Institute). 82p.
Bradshaw, S. (2013). Women's decision-making in rural and urban households in Nicaragua: the influence of income and ideology. Environ. Urban. 25, 81–94. doi: 10.1177/0956247813477361
Celette, F., Gaudin, R., and Gary, C. (2008). Spatial and temporal changes to the water regime of a Mediterranean vineyard due to the adoption of cover cropping. Eur. J. Agron. 29, 153–162. doi: 10.1016/j.eja.2008.04.007
Chianu, J. N., Tsujii, H., and Kormawa, P. (2004). Agriculture in the Savannas of Northern Nigeria: pressures, transformations, damage and coping strategies. Outlook Agric. 33, 247–253. doi: 10.5367/0000000042664828
Choudhury, H. K., and Goswami, K. (2013). Determinants of expansion of area under jatropha plantation in North East India: a tobit analysis. For. Policy Econ. 30, 46–52. doi: 10.1016/j.forpol.2013.02.006
Corbera, E., and Brown, K. (2010). Offsetting benefits? Analysing access to forest carbon. Environ. Plan. A 42, 1739–1761. doi: 10.1068/a42437
Cordingley, J. E., Snyder, K. A., Rosendahl, J., Kizito, F., and Bossio, D. (2015). Thinking outside the plot: addressing low adoption of sustainable land management in sub-Saharan Africa. Curr. Opin. Environ. Sustain. 15, 35–40. doi: 10.1016/j.cosust.2015.07.010
Curran-Cournane, F., Cain, T., Greenhalgh, S., and Samarsinghe, O. (2016). Attitudes of a farming community towards urban growth and rural fragmentation—an Auckland case study. Land Use Policy 58, 241–250. doi: 10.1016/j.landusepol.2016.07.031
Danso-Abbeam, G., Bosiako, J. A., Ehiakpor, D. S., and Mabe, F. N. (2017). Adoption of improved maize variety among farm households in the northern region of Ghana. Cogent Econ. Finan. 5:1416896. doi: 10.1080/23322039.2017.1416896
De Graaff, J., Amsalu, A., Bodnar, F., Kessler, A., Posthumus, H., and Tenge, A. (2008). Factors influencing adoption and continued use of long-term soil and water conservation measures in five developing countries. Appl. Geogr. 28, 271–280. doi: 10.1016/j.apgeog.2008.05.001
De-Miguel, M. D., Caballero, P., and Fernández-Zamudio, M. A. (2019). Varietal change dominates adoption of technology in Spanish citrus production. Agronomy 9:631. doi: 10.3390/agronomy9100631
Diwani, T. N., Asch, F., Becker, M., and Mussgnug, F. (2013). Characterising farming systems around kakamega forest, Western Kenya, for targeting soil fertility–enhancing technologies. J. Plant Nutr. Soil Sci. 176, 585–594. doi: 10.1002/jpln.201200036
Doss, C. R., and Morris, M. L. (2000). How does gender affect the adoption of agricultural innovations? The case of improved Maize technology in Ghana. Agric. Econ. 25, 27–39. doi: 10.1111/j.1574-0862.2001.tb00233.x
Edmeades, S., Phaneuf, D. J., Smale, M., and Renkow, M. (2008). Modelling the crop variety demand of semi-subsistence households: bananas in Uganda. J. Agric. Econ. 59, 329–349. doi: 10.1111/j.1477-9552.2007.00153.x
Enki, M., Belay, K., and Dadi, L. (2001). Determinants of adoption of physical soil conservation measures in central highlands of Ethiopia the case of three districts of North Shewa. Agrekon 40, 293–315. doi: 10.1080/03031853.2001.9524955
Erb, K. H., Haberl, H., Jepsen, M. R., Kuemmerle, T., Lindner, M., Müller, D., et al. (2013). A conceptual framework for analysing and measuring land-use intensity. Curr. Opin. Environ. Sustain. 5, 464–470. doi: 10.1016/j.cosust.2013.07.010
Ervin, C. A., and Ervin, D. E. (1982). Factors affecting the use of soil conservation practices: hypotheses, evidence, and policy implications. Land Econ. 58, 277–292. doi: 10.2307/3145937
Finger, R., and El Benni, N. (2013). Farmers' adoption of extensive wheat production–determinants and implications. Land Use Policy 30, 206–213. doi: 10.1016/j.landusepol.2012.03.014
Fischer, E., and Qaim, M. (2012). Linking smallholders to markets: determinants and impacts of farmer collective action in Kenya. World Dev. 40, 1255–1268. doi: 10.1016/j.worlddev.2011.11.018
Fouladbash, L., and Currie, W. S. (2015). Agroforestry in Liberia: household practices, perceptions and livelihood benefits. Agrofor. Syst. 89, 247–266. doi: 10.1007/s10457-014-9763-9
Gunawan, M. (1998). Adoption and bias of new agricultural innovation in Jawa Barat, Indonesia (PhD Thesis). Minnesota: University of Minnesota.
Irungu, J. W. (2013). Relationship between Agricultural Credit Financing and Financial Performance: A Case of Small Scale Farmers in Kiria Division in Muranga County (M.Sc. thesis), Nairobi Kenya: University of Nairobi.
Jaetzold, R., Schmidt, H., Hornetz, B., and Shisanya, C. (2007). Farm Management Handbook of Kenya. Natural Conditions and Farm Management Information. Part B. Central Kenya. Subpart B2. South Rift, 2. Nairobi: Ministry of Agriculture/GTZ.
Jingchao, Z., Kotani, K., and Saijo, T. (2019). Low-quality or high-quality coal? Household energy choice in rural Beijing. Energy Econ. 78, 81–90. doi: 10.1016/j.eneco.2018.11.005
Johansen, C., Haque, M. E., Bell, R. W., Thierfelder, C., and Esdaile, R. J. (2012). Conservation agriculture for smallholder rainfed farming: opportunities and constraints of new mechanised seeding systems. Field Crops Res. 132, 18–32. doi: 10.1016/j.fcr.2011.11.026
Kalinda, T., Filson, G., and Shute, J. (2000). Resources, household decision making and organisation of labour in food production among small-scale farmers in southern Zambia. Dev. South. Afr. 17, 165–174. doi: 10.1080/713661404
Kangai, R. (2004). Assessment of factors affecting adoption of soil fertility improvement technologies in eastern Kenya: the case of kirege location, Chuka (M.Sc. thesis). Nairobi Kenya: Kenyatta University.
Kassie, M., Jaleta, M., Shiferaw, B., Mmbando, F., and Mekuria, M. (2013). Adoption of interrelated sustainable agricultural practices in smallholder systems: evidence from rural Tanzania. Technol. Forecast. Soc. Change 80, 525–540. doi: 10.1016/j.techfore.2012.08.007
Kassie, M., Shiferaw, B., and Muricho, G. (2011). Agricultural technology, crop income, and poverty alleviation in Uganda. World Dev. 39, 1784–1795. doi: 10.1016/j.worlddev.2011.04.023
Kenya National Bureau of Statistics (KNBS) (2019). Kenya Population and Housing Census Volume I : Population By County and Sub-County. Nairobi: KNBS.
Kiboi, M. N., Ngetich, K. F., Fliessbach, A., Muriuki, A., and Mugendi, D. N. (2019). Soil fertility inputs and tillage influence on Maize crop performance and soil water content in the central highlands of Kenya. Agric. Water Manage. 217, 316–331. doi: 10.1016/j.agwat.2019.03.014
Kiboi, M. N., Ngetich, K. F., Mugendi, D. N., Muriuki, A., Adamtey, N., and Fliessbach, A. (2018). Microbial biomass and acid phosphomonoesterase activity in soils of the Central Highlands of Kenya. Geoderma Reg. 15:e00193. doi: 10.1016/j.geodrs.2018.e00193
Kidane, H., Alemu, Z. G., and Kundhlande, G. (2005). Causes of household food insecurity in Koredegaga peasant association, Oromiya zone, Ethiopia. Agrekon 44, 543–560. doi: 10.1080/03031853.2005.9523727
Kimani, S. K., Macharia, J. M., Gachengo, C., Palm, C. A., and Delve, R. J. (2004). Maize production in the central Kenya Highlands using cattle manures combined with modest amounts of mineral fertilizer. Uganda J. Agric. Sci. 9, 480–490. Available online at: https://www.ajol.info/index.php/ujas/article/view/134989
Kisaka, M. O., Mucheru-Muna, M., Ngetich, F. K., Mugwe, J. N., Mugendi, D. N., Mairura, F., et al. (2016). Using APSIM-model as a decision-support-tool for long-term integrated-nitrogen-management and Maize productivity under semi-arid conditions in Kenya. Exp. Agric. 52, 279–299. doi: 10.1017/S0014479715000095
Kpadonou, R. A. B., Owiyo, T., Barbier, B., Denton, F., Rutabingwa, F., and Kiema, A. (2017). Advancing climate-smart-agriculture in developing drylands: joint analysis of the adoption of multiple on-farm soil and water conservation technologies in West African Sahel. Land Use Policy 61, 196–207. doi: 10.1016/j.landusepol.2016.10.050
Krah, K., Michelson, H., Perge, E., and Jindal, R. (2019). Constraints to adopting soil fertility management practices in Malawi: a choice experiment approach. World Dev. 124, 1–1. doi: 10.1016/j.worlddev.2019.104651
Lehtinen, T., Schlatter, N., Baumgarten, A., Bechini, L., Krüger, J., Grignani, C., et al. (2014). Effect of crop residue incorporation on soil organic carbon and greenhouse gas emissions in European agricultural soils. Soil Use Manage. 30, 524–538. doi: 10.1111/sum.12151
Liverpool-Tasie, L. S. O., Omonona, B. T., Sanou, A., and Ogunleye, W. O. (2017). Is increasing inorganic fertiliser use for Maize production in SSA a profitable proposition? Evidence from Nigeria. Food Policy 67, 41–51. doi: 10.1016/j.foodpol.2016.09.011
Lundy, M. E., Pittelkow, C. M., Linquist, B. A., Liang, X., van Groenigen, K. J., Lee, J., et al. (2015). Nitrogen fertilisation reduces yield declines following no-till adoption. Field Crops Res. 183, 204–210. doi: 10.1016/j.fcr.2015.07.023
Lynne, G. D., Shonkwiler, J. S., and Rola, L. R. (1988). Attitudes and farmer conservation behavior. Am. J. Agric. Econ. 70, 12–19. doi: 10.2307/1241971
Macharia, J., Mugwe, J., Mucheru-Muna, M., and Mugendi, D. (2014). Socioeconomic factors influencing levels of knowledge in soil fertility management in the central highlands of Kenya. J. Agric. Sci. Technol. B, 4, 701–711. doi: 10.17265/2161-6264/2014.09.003
Mal, P., Anik, A. R., Bauer, S., and Schmitz, P. M. (2013). Bt cotton adoption: a double-hurdle approach for north Indian farmers. AgBioForum 15, 294–302.
Marenya, P. P., and Barrett, C. B. (2007). Household-level determinants of adoption of improved natural resources management practices among smallholder farmers in western Kenya. Food Policy 32, 515–536. doi: 10.1016/j.foodpol.2006.10.002
Martey, E., Wiredu, A. N., Etwire, P. M., Fosu, M., Buah, S. S. J., Bidzakin, J., et al. (2014). Fertiliser adoption and use intensity among smallholder farmers in Northern Ghana: a case study of the AGRA soil health project. Sustain. Agric. Res. 3, 24–36. doi: 10.5539/sar.v3n1p24
Mazvimavi, K., and Twomlow, S. (2009). Socioeconomic and institutional factors influencing adoption of conservation farming by vulnerable households in Zimbabwe. Agric. Syst. 101, 20–29. doi: 10.1016/j.agsy.2009.02.002
Mburu, L., Wakhungu, J. W., and Gitu, K. W. (2007). Determinants of smallholder dairy farmers' adoption of various milk marketing channels in Kenya highlands. Livestock Res. Rural Dev. 19, 9–18.
McDonald, J. F., and Moffitt, R. A. (1980). The uses of tobit analysis. Rev. Econ. Stat. 62, 318–321. doi: 10.2307/1924766
Meijer, S. S., Catacutan, D., Ajayi, O. C., Sileshi, G. W., and Nieuwenhuis, M. (2015). The role of knowledge, attitudes and perceptions in the uptake of agricultural and agroforestry innovations among smallholder farmers in sub-Saharan Africa. Int. J. Agric. Sustain. 13, 40–54. doi: 10.1080/14735903.2014.912493
Mercer, D. E., and Pattanayak, S. K. (2003). “Agroforestry adoption by smallholders,” in Forests in a Market Economy, eds E. O. Sills and K. L. Abt (Dordrecht: Springer), 283–299 doi: 10.1007/978-94-017-0219-5_16
Mignouna, D. B., Manyong, V. M., Rusike, J., Mutabazi, K. D. S., and Senkondo, E. M. (2011). Determinants of adopting imazapyr-resistant Maize technologies and its impact on household income in Western Kenya. AgBioForum 14, 158–163.
Ministry of Education Science and Technology (2014). Basic Education Stastical Booklet. Nairobi: Government of Kenya press.
Muatha, I. T., Otieno, D. J., and Nyikal, R. A. (2017). Determinants of smallholder farmers awareness of agricultural extension devolution in Kenya. Afr. J. Agric. Res. 12, 3549–3555. doi: 10.5897/AJAR2017.12603
Mucheru-Muna, M., Mugendi, D., Pypers, P., Mugwe, J., Kung'u, J., Vanlauwe, B., et al. (2014). Enhancing Maize productivity and profitability using organic inputs and mineral fertiliser in central Kenya small-hold farms. Exp. Agric. 50, 250–269. doi: 10.1017/S0014479713000525
Mucheru-Muna, M., Pypers, P., Mugendi, D., Kung' u, J., Mugwe, J., Merckx, R., et al. (2010). A staggered Maize–legume intercrop arrangement robustly increases crop yields and economic returns in the highlands of Central Kenya. Field Crops Res. 115, 132–139. doi: 10.1016/j.fcr.2009.10.013
Mugendi, D. N., Nair, P. K. R., Mugwe, J. N., O'neill, M. K., and Woomer, P. (1999). Alley cropping of Maize with calliandra and leucaena in the subhumid highlands of Kenya: part 2. Soil-fertility changes and maize yield. Agrofor. Syst. 46, 39–50. doi: 10.1023/A:1006288301044
Mugwe, J., Mugendi, D., Mucheru-Muna, M., Merckx, R., Chianu, J., and Vanlauwe, B. (2009). Determinants of the decision to adopt integrated soil fertility management practices by smallholder farmers in the central highlands of Kenya. Exp. Agric. 45, 61–75. doi: 10.1017/S0014479708007072
Mulinge, W., Gicheru, P., Murithi, F., Maingi, P., Kihiu, E., Kirui, O. K., et al. (2016). “Economics of land degradation and improvement in Kenya,” in Economics of Land Degradation and Improvement – A Global Assessment for Sustainable Development, eds E. Nkonya, A. Mirzabaev, and J. von Braun (Cham: Springer), 471–498. doi: 10.1007/978-3-319-19168-3_16
Mulolwa, A., Mfune, O., Shakachite, O., Mubanga, D., Umar, B., Nsombo, P., et al. (2016). Land Governance Assessment: Zambia Country Report. Washington, DC: World Bank. doi: 10.1596/28503
Murage, F. M., Mugwe, J. N., Ngetich, K. F., Mucheru-Muna, M. M., and Mugendi, D. N. (2019). Adoption of soybean by smallholder farmers in the Central Highlands of Kenya. Afr. J. Agric. Econ. Rural Dev. 7, 001–012.
Mutegi, E. M., Kung' u, J. B., Muna, M., Pieter, P., and Mugendi, D. N. (2012). Complementary effects of organic and mineral fertilisers on Maize production in the smallholder farms of Meru South District, Kenya. Agric. Sci. 03, 221–229. doi: 10.4236/as.2012.32026
Muzira, R., Vanlauwe, B., Rwakaikara, S. M., Basamba, T., Chianu, J., and Farrow, A. (2011). “Variation in socioeconomic characteristics and natural resource management in communities with different potato market linkages in the highlands of Southwestern Uganda,” in Innovations as Key to the Green Revolution in Africa, eds A. Bationo, B. Waswa, J. Okeyo, F. Maina, J. Kihara (Dordrecht: Springer), 999–1008. doi: 10.1007/978-90-481-2543-2_101
Ndiritu, S. W., Kassie, M., and Shiferaw, B. (2014). Are there systematic gender differences in the adoption of sustainable agricultural intensification practices? Evidence from Kenya. Food Policy 49, 117–127. doi: 10.1016/j.foodpol.2014.06.010
Neupane, R. P., Sharma, K. R., and Thapa, G. P. (2002). Adoption of agroforestry in the hills of Nepal: a logistic regression analysis. Agric. Syst. 72, 177–196. doi: 10.1016/S0308-521X(01)00066-X
Nganga, W. B., Ng'etich, K. O., Macharia, M. J., Kiboi, N. M., Adamtey, N., and Ngetich, K. F. (2020). Multi-influencing-factors' evaluation for organic-based soil fertility technologies out-scaling in Upper Tana Catchment in Kenya. Sci. Afr. 7:e00231. doi: 10.1016/j.sciaf.2019.e00231
Ngetich, F. K., Shisanya, C. A., Mugwe, J. N., Mucheru-Muna, M. W., and Mugendi, D. N. (2012). The potential of organic and inorganic nutrient sources in Sub-Saharan African crop farming systems, soil fertility improvement and integrated nutrient management - a global perspective. IntechOpen 135–156. doi: 10.5772/28728
Ngetich, K. F., Diels, J., Shisanya, C. A., Mugwe, J. N., Mucheru-Muna, M., and Mugendi, D. N. (2014). Effects of selected soil and water conservation techniques on runoff, sediment yield and Maize productivity under sub-humid and semi-arid conditions in Kenya. Catena 121, 288–296. doi: 10.1016/j.catena.2014.05.026
Nhemachena, C., and Hassan, R. M. (2007). Micro-Level Analysis of Farmers Adaptation to Climate Change in Southern Africa. IFPRI Discussion Paper 714. Washington, DC: IFPRI.
Nigussie, Z., Tsunekawa, A., Haregeweyn, N., Adgo, E., Nohmi, M., Tsubo, M., et al. (2017). Factors influencing small-scale farmers' adoption of sustainable land management technologies in north-western Ethiopia. Land Use Policy 67, 57–64. doi: 10.1016/j.landusepol.2017.05.024
Njuki, J. M., Mapila, M. T., Zingore, S., and Delve, R. (2008). The dynamics of social capital in influencing use of soil management options in the chinyanja triangle of southern Africa. Ecol. Soc. 13, 9–25. doi: 10.5751/ES-02539-130209
Ntshangase, N. L., Muroyiwa, B., and Sibanda, M. (2018). Farmers' perceptions and factors influencing the adoption of no-till conservation agriculture by small-scale farmers in Zashuke, KwaZulu-Natal province. Sustainability 10:555. doi: 10.3390/su10020555
Nyaga, J., Barrios, E., Muthuri, C. W., Öborn, I., Matiru, V., and Sinclair, F. L. (2015). Evaluating factors influencing heterogeneity in agroforestry adoption and practices within smallholder farms in Rift Valley, Kenya. Agric. Ecosyst. Environ. 212, 106–118. doi: 10.1016/j.agee.2015.06.013
Odendo, M., Obare, G., and Salasya, B. (2010). “Determinants of the speed of adoption of soil fertility-enhancing technologies in Western Kenya,” in Joint 3rd African Association of Agricultural Economists (AAAE) and 48th Agricultural Economists Association of South Africa (AEASA) Conference (Cape Town).
Odendo, M., Obare, G., and Salasya, B. (2011). “Farmers' perception of soil fertility depletion and its influence on uptake of integrated soil nutrient management techniques: evidence from Western Kenya,” in Innovations as Key to the Green Revolution in Africa, eds A. Bationo, B. Waswa, J. Okeyo, F. Maina, J. Kihara (Dordrecht: Springer), 1055–1059. doi: 10.1007/978-90-481-2543-2_107
O'Donoghue, C., and Heanue, K. (2018). The impact of formal agricultural education on farm level innovation and management practices. J. Technol. Trans. 43, 844–863. doi: 10.1007/s10961-016-9529-9
Ogunlana, E. A. (2004). The technology adoption behavior of women farmers: the case of alley farming in Nigeria. Renewable Agric. Food Syst. 19, 57–65. doi: 10.1079/RAFS200366
Okuro, J. O., Muriithi, F. M., Mwangi, W., Verjuikl, H., Gethi, M., and Groote, H. (2002). Adoption of Maize Seed and Fertiliser Technologies in Embu District, Kenya. Mexico: CIMMYT and Kenya Agricultural Research Institute (KARI).
Olwande, J., Sikei, G., and Mathenge, M. (2009). Agricultural Technology Adoption: A Panel Analysis of Smallholder Farmers' Fertiliser Use in Kenya. Agriculture for Development, Center of Evaluation for Global Action, UC Berkeley.
Omenda, J. A., Ngetich, K. F., Kiboi, M. N., Mucheru-Muna, M. W., and Mugendi, D. N. (2019). Soil organic carbon and acid phosphatase enzyme activity response to phosphate rock and organic inputs in acidic soils of central highlands of Kenya in maize. Int. J. Plant Soil Sci. 30, 1–13. doi: 10.9734/ijpss/2019/v30i230169
Paul, J., Sierra, J., Causeret, F., Guindé, L., and Blazy, J. M. (2017). Factors affecting the adoption of compost use by farmers in small tropical Caribbean islands. J. Clean. Prod. 142, 1387–1396. doi: 10.1016/j.jclepro.2016.11.168
Peterman, A., Behrman, J. A., and Quisumbing, A. R. (2014). “A review of empirical evidence on gender differences in nonland agricultural inputs, technology, and services in developing countries,” in Gender in Agriculture, eds A. Quisumbing, R. Meinzen-Dick, T. Raney, A. Croppenstedt, J. Behrman, and A. Peterman (Dordrecht: Springer), 145-186. doi: 10.1007/978-94-017-8616-4_7
Place, F., Barrett, C. B., Freeman, H. A., Ramisch, J. J., and Vanlauwe, B. (2003). Prospects for integrated soil fertility management using organic and inorganic inputs: evidence from smallholder African agricultural systems. Food Policy 28, 365–378. doi: 10.1016/j.foodpol.2003.08.009
Ramaekers, L., Micheni, A., Mbogo, P., Vanderleyden, J., and Maertens, M. (2013). Adoption of climbing beans in the central highlands of Kenya: an empirical analysis of farmers' adoption decisions. Afr. J. Agric. Res. 8, 1–19.
Rauniyar, G. P., and Goode, F. M. (1992). Technology adoption on small farms. World Dev. 20, 275–282. doi: 10.1016/0305-750X(92)90105-5
Salasya, B., Mwangi, W. M., Mwabu, D., and Diallo, A. (2007). Factors influencing adoption of stress-tolerant maize hybrid (WH 502) in western Kenya. Afr. J. Agric. Res. 2, 544–551.
Sauer, C. M., Mason, N. M., Maredia, M. K., and Mofya-Mukuka, R. (2018). Does adopting legume-based cropping practices improve the food security of small-scale farm households? Panel survey evidence from Zambia. Food Secur. 10, 1463–1478. doi: 10.1007/s12571-018-0859-3
Shiferaw, B. A., Okello, J., and Reddy, R. V. (2009). Adoption and adaptation of natural resource management innovations in smallholder agriculture: reflections on key lessons and best practices. Environ. Dev. Sustain. 11, 601–619. doi: 10.1007/s10668-007-9132-1
Simelton, E. S., Catacutan, D. C., Dao, T. C., Dam, B. V., and Le, T. D. (2017). Factors constraining and enabling agroforestry adoption in Viet Nam: a multi-level policy analysis. Agrofor. Syst. 91, 51–67. doi: 10.1007/s10457-016-9906-2
Spiegel, H., Zavattaro, L., Guzmán Díaz, M. G., D'Hose, T., Pecio, A., Lehtinen, T., et al. (2015). Impacts of Soil Management Practices on Crop Productivity, on Indicators for Climate Change Mitigation, and on the Chemical, Physical and Biological Quality of Soil. Wageningen, The Netherlands. FP7 CATCH-C project.
Staal, S. J., Baltenweck, I., Waithaka, M. M., DeWolff, T., and Njoroge, L. (2002). Location and uptake: integrated household and GIS analysis of technology adoption and land use, with application to smallholder dairy farms in Kenya. Agric. Econ. 27, 295–315. doi: 10.1111/j.1574-0862.2002.tb00122.x
Takusewanya, R., Namayanja, A., Ugen, M. A., Nkalubo, S., Odong, T. L., and Bwogi, G. V. (2018). Climbing beans in Uganda: a perspective of smallholder farmers on their determinants, associated challenges and implications for research. Afr. J. Agric. Res. 13, 1374–1388. doi: 10.5897/AJAR2017.12131
Teklewold, H., Kassie, M., and Shiferaw, B. (2013). Adoption of multiple sustainable agricultural practices in rural Ethiopia. J. Agric. Econ. 64, 597–623. doi: 10.1111/1477-9552.12011
Thuo, M., Bravo-Ureta, B. E., Hathie, I., and Obeng-Asiedu, P. (2011). Adoption of chemical fertiliser by smallholder farmers in the peanut basin of Senegal. Afr. J. Agric. Resour. Econ. 6, 1–17. doi: 10.22004/ag.econ.156960
Twarog, S. (2006). Organic agriculture: a trade and sustainable development opportunity for developing countries. Trade Environ. Rev. 141, 223. doi: 10.18356/6ad7c431-en
Udayakumara, E. P. N., Shrestha, R. P., Samarakoon, L., and Schmidt-Vogt, D. (2010). People's perception and socioeconomic determinants of soil erosion: a case study of Samanalawewa watershed, Sri Lanka. Int. J. Sediment Res. 25, 323–339. doi: 10.1016/S1001-6279(11)60001-2
Vigneswaran, S., Kandasamy, J., and Johir, M. A. H. (2016). Sustainable operation of composting in solid waste management. Procedia Environ. Sci. 35, 408–415. doi: 10.1016/j.proenv.2016.07.022
Waithaka, M. M., Thornton, P. K., Shepherd, K. D., and Ndiwa, N. N. (2007). Factors affecting the use of fertilisers and manure by smallholders: the case of Vihiga, western Kenya. Nutr. Cycling Agroecosyst. 78, 211–224. doi: 10.1007/s10705-006-9087-x
Wanjiku, J., Manyengo, J. U., Oluoch-Kosura, W., and Karugia, J. T. (2007). Gender “Differentiation in the analysis of alternative farm mechanisation choices on small farms in Kenya,” in Food Insecurity, Vulnerability and Human Rights Failure. Studies in Development Economics and Policy, eds B. Guha-Khasnobis, S. S. Acharya, and B. Davis (London: Palgrave), 194–218 doi: 10.1057/9780230589506_8
Keywords: socioeconomic determinants, organic inputs, smallholder farmers, Sub-Saharan Africa, manure, tropical livestock unit
Citation: Mwaura GG, Kiboi MN, Bett EK, Mugwe JN, Muriuki A, Nicolay G and Ngetich FK (2021) Adoption Intensity of Selected Organic-Based Soil Fertility Management Technologies in the Central Highlands of Kenya. Front. Sustain. Food Syst. 4:570190. doi: 10.3389/fsufs.2020.570190
Received: 06 June 2020; Accepted: 11 December 2020;
Published: 13 January 2021.
Edited by:
Patrick Meyfroidt, Catholic University of Louvain, BelgiumReviewed by:
Fabio Bartolini, University of Pisa, ItalyTherese Mwatitha Gondwe, Independent Researcher, Lilongwe, Malawi
Copyright © 2021 Mwaura, Kiboi, Bett, Mugwe, Muriuki, Nicolay and Ngetich. This is an open-access article distributed under the terms of the Creative Commons Attribution License (CC BY). The use, distribution or reproduction in other forums is permitted, provided the original author(s) and the copyright owner(s) are credited and that the original publication in this journal is cited, in accordance with accepted academic practice. No use, distribution or reproduction is permitted which does not comply with these terms.
*Correspondence: George G. Mwaura, Z2cubXdhdXJhMUBnbWFpbC5jb20=