- 1Department of Crop Sciences, University of Illinois, Urbana, IL, United States
- 2Departamento de Fitotecnia, Univsersidad Nacional Agraria La Molina, Lima, Peru
- 3Fondo Latinoamericano para Arroz de Riego (FLAR), Palmira, Colombia
- 4Centro Internacional de Agricultura Tropical (CIAT), Palmira, Colombia
- 5Mohammed VI Polytechnic University (UM6P), AgroBioSciences (AgBS), Agricultural Innovations and Technology Transfer Centre (AITTC), Benguerir, Morocco
- 6Department of Plant Sciences, University of California-Davis, Davis, CA, United States
Integrated research efforts are needed to provide insights for simultaneously achieving food production and environmental goals in rice production systems. In this study we evaluated eight Sustainable Rice Platform performance indicators (net profit, labor productivity, grain yield, water use efficiency, nitrogen use efficiency, phosphorus use efficiency, pesticide use efficiency, and greenhouse gas emissions) for 65 rice farmers in Peru. The aim was to (1) identify the major management factors influencing profitability, (2) evaluate potential tradeoffs between economic and environmental sustainability, and (3) understand farmer perceptions and decision-making processes related to profitability. For data analysis, farmers were grouped into profitability categories based on net profit: top (top 20%), middle (middle 60%), and bottom (bottom 20%). Our results indicate that rice yields, grain sale price, and total spending drive profitability in this region of Peru. An important finding is that the top profitability group achieved higher yields while using lower inputs of labor, pesticides, elemental P, and irrigation hours, resulting in improved environmental performance (e.g., higher nutrient and water use efficiency and lower greenhouse gas emissions). Results from focus group discussions highlighted that rice production has changed in recent decades, and there is a need for improved extension support to cope with increasing pests, disease, and water scarcity. Moreover, farmers indicated they are vulnerable to exploitation from vendors along the rice supply chain, with limited access to credit facilities and market channels and little negotiating power over the cost of inputs and rice sale price. Our results suggest that rice productivity and profitability can be improved in this region without negatively influencing environmental footprint per unit production, yet this is unlikely to occur unless key agronomic and socio-economic challenges are addressed, such as improved access to crop management recommendations, alternative financing and credit options, and technologies that decrease the costs of production.
Introduction
Rice (Oryza sativa L.) is a major source of calories for nearly half the world's population and a livelihood source for over one billion people (Van Nguyen and Ferrero, 2006). Rice consumption is drastically increasing in developing countries with expanding populations (Seck et al., 2012). Global projections show that rice production will need to increase by 26% by 2035 to meet the growing demand in regions such as Africa and Latin America (Seck et al., 2012). While production increases are critical for food security, there is also a need for balancing the competing goals of maximizing farmer profits and minimizing negative effects on the environment.
Intensive rice production practices have increased global cereal output, but higher yields do not always mean increased profitability at the farm-level. Starting with The Green Revolution, the use of technologies, such as improved seed, irrigation and the intensive use of fertilizers and pesticides, has increased global rice yields by 130% from 1966 to 2000 (Khush, 2005). During this period, populations of underdeveloped countries grew by 90%, food prices consistently decreased, and an estimated 18 million hectares (ha) of agricultural land was spared from cultivation (Khush, 2005; Stevenson et al., 2013). However, external inputs including fertilizers and agrochemicals represent a significant economic cost for farmers, many of whom do not have access to financial resources or the appropriate knowledge to effectively manage their rice crop and thereby maximize returns to investment (Reardon et al., 2009; Wiggins et al., 2010). For rice production, labor costs can also be exceptionally high for transplanting as a crop establishment method (Toriyama et al., 2005). Lastly, since the 1960's, global grain prices have continued to decline as input costs have increased (FAOSTAT, 2020). While this is beneficial for consumers, producers ultimately generate less revenue per unit. Therefore, if costs are too high and yields or rice prices are too low, there is a risk that rice production will not be profitable. Given the dependence of millions of small-scale farmers on rice production as a livelihood strategy, understanding the major factors influencing profitability in specific biophysical, political, and socioeconomic contexts is necessary to inform the development of effective agricultural research and extension programs.
In the face of growing resource scarcity and a changing climate, crop production targets aimed at attaining global food security must also be achieved sustainably without expanding land under crop production (Godfray and Garnett, 2014). High greenhouse gas emissions (GHG) and water use are two main challenges threatening the sustainability of flooded rice systems. Methane emissions from rice production account for roughly 50% of global cropland emissions (Carlson et al., 2016). In addition, it is estimated that rice production uses ~34–43% of the world's irrigation water (Bouman et al., 2007). High rates of fertilizers and pesticides are also known to pollute air, water, and soil resources which has long-term environmental and social ramifications (De Roos et al., 2003; Pimentel et al., 2004; Fageria and Baligar, 2005; Rosenbaum et al., 2008). Hence, it is necessary to evaluate the environmental performance of rice production using multiple indicators to comprehensively balance between profitability and efficient management practices leading to a reduced environmental footprint. Benchmarking yield and resource-use efficiency gaps among farmers is a well-documented strategy to highlight attainable efficiencies and yield-limiting factors within specific agronomic and socioeconomic contexts (Van Ittersumvan and Rabbinge, 1997; Papademetriou et al., 2000; Lobell et al., 2009; Foley et al., 2011; Stuart et al., 2016; Devkota et al., 2019). In simple terms, yield and resource use gaps are calculated as the difference between the highest attainable values currently achieved in the region and the average value achieved by farmers. While yield gap analysis is a crucial first step in setting agricultural intensification targets, relatively few studies have holistically examined relationships between yield gaps and sustainability indicators to determine tradeoffs between increasing yields and its adverse impacts on environmental footprint.
The Sustainable Rice Platform (SRP) is a multi-stakeholder alliance led by the United Nations (UN) Environment Programme and IRRI (International Rice Research Institute), with the overall goal to create a framework for evaluating and promoting sustainable rice production (Sustainable Rice Platform, 2015). The SRP contains a set of 12 performance indicators designed to evaluate the sustainability of farming systems while also identifying opportunities for improvement. Utilizing performance indicators for benchmarking yield and resource use efficiency gaps allows local evaluation of performance, as well as regional comparisons within a standardized and internationally accepted framework. The majority of research utilizing indicators from the SRP has focused on Southeast Asia, where it has identified areas of improvement for the adoption of sustainable practices (Stuart et al., 2018; Watcharapongchai et al., 2018; Devkota et al., 2019; 2020). To our knowledge, there is no published evidence that the SRP has been utilized to evaluate rice production practices in Latin America.
In Peru, like many countries in Latin America, rice is a staple food and cash crop with demand rapidly increasing due to a growing population with changing dietary preferences. Since 1960, Peru's land area of rice production has expanded by 81% and yields have increased by 49%, but the country is still a net rice importer (FAOSTAT, 2020). Peru has the second-highest average rice yields in Latin America and the fifth-highest in the world (FAOSTAT, 2020). However, gains in productivity have been supported by higher input use which is generally associated with greater environmental costs (Foley et al., 2011). From the year 2007 to 2017, Peru increased total agricultural pesticide use by 69%, and since 2002 has increased total nitrogen fertilizer use by 36% (FAOSTAT, 2020). Thus, there is a need to evaluate how input use affects the sustainability and profitability of farm systems in this region.
Exploring the farmer decision-making process is important to adequately understand farm management decisions within farm systems (Byerlee and Anderson, 1982; Edwards-Jones, 2006; Wilson et al., 2009; Nakazi and Sserunkuuma, 2013). Farmers have a variety of perceptions, biases, and personal beliefs that inform the decisions that they make (Mankad, 2016). Researching extrinsic and intrinsic factors such as profitability, praise, and personal motivation can lead to a better understanding of the adoption or non-adoption of certain agricultural practices (Dono et al., 2013; Meijer et al., 2015). An important outcome of researching farmer perceptions is to determine if farmers perceptions of profitability and successful management are different from those of various stakeholders tasked with implementing solutions.
Balancing the economic and environmental sustainability of rice production systems is a complex challenge. Integrated research efforts accounting for both biophysical and social dimensions are needed to provide insights for achieving food production and environmental sustainability goals in the future. The objectives of this study were to (1) investigate the economic performance of smallholder rice farming systems of Peru to identify the major factors influencing profitability, (2) evaluate environmental sustainability using SRP performance indicators and assess relationships with economic outcomes, and (3) understand perceptions and barriers to increasing productivity and profitability based on discussions of research findings with local farmers.
Methods
Characterization of the Survey Region
This study was conducted within the Ferreñafe irrigation district of the Ferreñafe province (6°38'01.8 “S, 79°47'14.3” W) in the department of Lambayeque, Peru (Figure 1). The department of Lambayeque is one of the largest rice-producing areas in the country and is known for high yields and exceptional grain quality due to favorable climate and availability of irrigation infrastructure. This region is ~20 km inland of the north-west coast of Peru, and is classified as coastal desert with rainfall <100 mm per year (Nguyen et al., 2019). The Ferreñafe irrigation district is the largest district in Lambayeque with ~14,000 ha planted in rice and 4,000 rice farmers. The conventional method of rice production consists of manual transplanting and high rates of fertilizer and pesticide inputs, when compared to small-scale irrigated farming regions in Asia (Devkota et al., 2019). Current production challenges in the region include labor and water shortages, disease pressure, and reduced soil quality due to salinization (Maclean et al., 2013). Farmers in this region typically grow one rice crop per year, without crop rotation, but with small plots of non-rice crops between seasons. The availability of irrigation is dependent on rainfall that occurs in the Andes Mountains, which is harvested from a reservoir situated 45 km away and delivered to the region using canal systems.
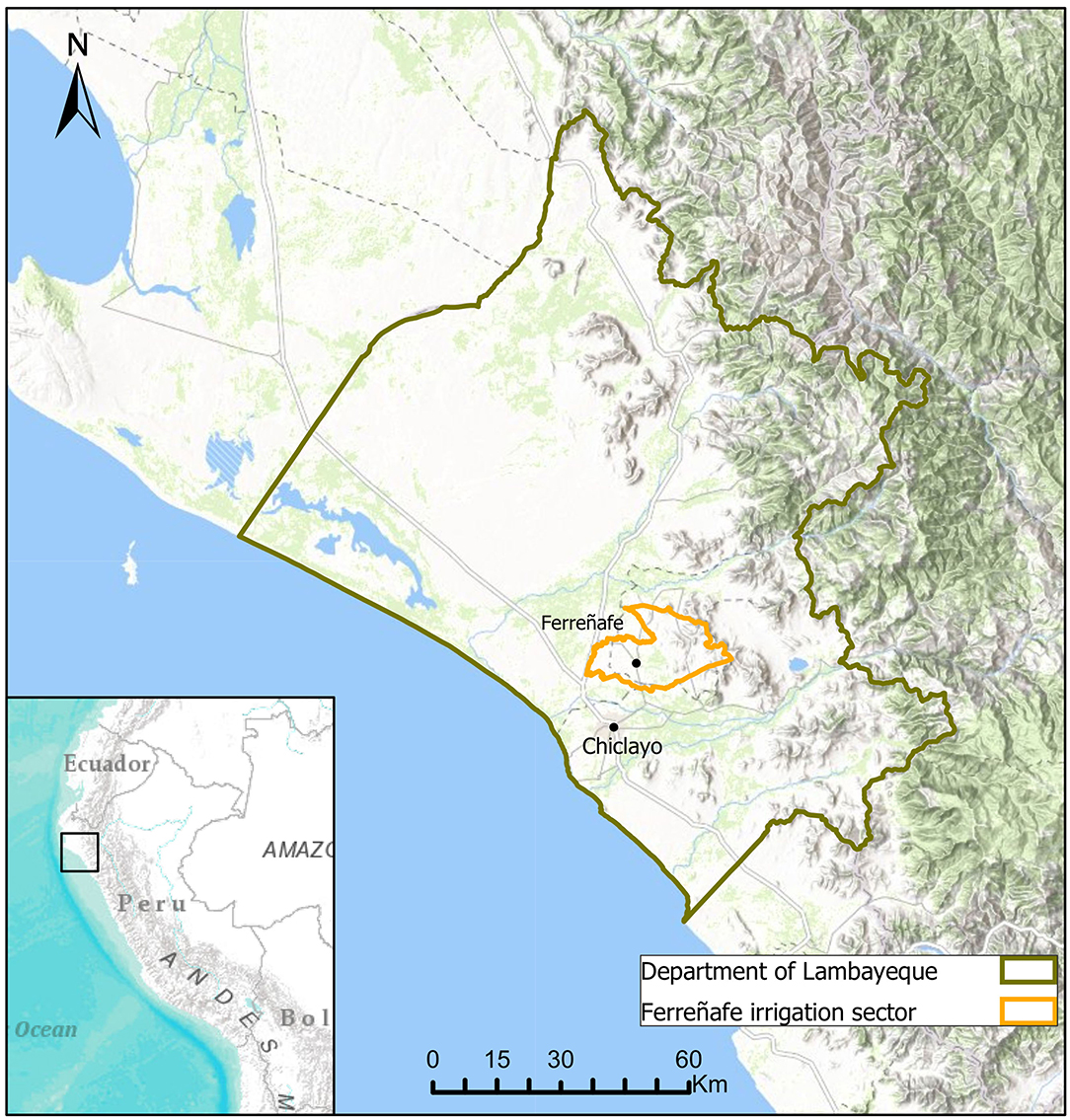
Figure 1. Map showing the Ferreñafe irrigation sector in the department of Lambayeque, Peru. Surveys were completed in the Ferreñafe irrigation sector. Sources: Esri, DeLorme, HERE, TomTom, Intermap, increment P Corp., GEBCO, USGS, FAO, NPS, NRCAN, GeoBase, IGN, Kadaster NL, Ordnance Survey, Esri Japan, METI, Esri China (Hong Kong), swisstopo, MapmyIndia, and the GIS User Community.
Farmer Survey
A paper-based survey was created in collaboration with CIAT (Centro Internacional de Agricultura Tropical) and the Universidad Nacional Agraria La Molina to collect detailed data on farm management practices and production costs. In turn, this information was used to calculate eight SRP performance indicators (Sustainable Rice Platform, 2015). The survey consisted of 115 questions in 14 sections: demographics, previous crop and residue management, land preparation, seed, fertilizer, irrigation fees, application of water, perceptions of water use, pesticide, herbicide, weeding, harvest, yield, and sale price (Supplementary Figure 1). The survey was piloted with six farmers in the district to verify that the questions were appropriate and applicable to the local context. Farmer surveys were conducted during July and August 2019. Respondents were limited to those that cultivated rice in the 2018-2019 growing season and confirmed that they were the sole decision-makers on management and input purchases for the farm. Farmers were identified within the Ferreñafe irrigation district through snowball sampling. This convenience technique relies on initial subjects referring additional subjects and is useful for identifying hard to reach populations where a sampling database does not exist (Biernacki and Waldorf, 1981). The total sample size for the survey was 65 farmers, representing 1.6% of the farmers in the region. Interviews lasted ~45 min, where farmers were asked to answer in-depth questions about the previous growing season.
Sustainable Rice Platform Performance Indicators
The SRP is an international framework for measuring the economic and environmental sustainability of rice systems across 12 performance indicators. Information from surveys allowed eight indicators to be calculated in this study: net profit, labor productivity, grain yield, water use efficiency, nitrogen use efficiency (NUE), phosphorus use efficiency (PUE), pesticide use efficiency, and GHG emission. The indicators for food safety, worker health and safety, child labor, and women empowerment were omitted to shorten the survey to an appropriate length for conducting the interview. Several modifications to indicator calculations were made based on local management practices and available data obtained from the farmers. Net profit ($ ha−1) was calculated by subtracting total variable costs from gross returns (grain revenue). The total variable cost is the sum of production costs for seedbed and field production (labor, fertilizer, harvest, transportation, land preparation, irrigation and fees, pesticides, herbicides, and seed), and the cost of milling and rent (if applicable) per hectare. An opportunity cost for land ownership could be included in total variable costs, but this is only valid in situations where land-owning farmers could easily find a renter. Based on our conversations with farmers, rice production is becoming less attractive as a livelihood strategy in this region and the potential for renting farmland is limited. Therefore, the opportunity cost for land ownership was excluded because conditions did not meet the assumption of perfect competition. Gross return was calculated from paddy yield and the market price the farmer received or expected to receive. Labor productivity (kg grain $ labor−1 ha−1) was calculated by dividing grain yield by the total cost of labor for production. Total labor hours and costs were calculated from farmer responses regarding the amount of time for a field activity and the rate of pay, including own and family labor. Farmers reported grain yield (kg grain ha−1) standardized to 14% moisture content. Water use efficiency (kg grain water-hour −1 ha−1) was calculated by dividing grain yield by the number of water-hours the farmer purchased. Farmers purchase water-hours from the irrigation commission, as a means to account and limit water usage in the district. The irrigation commission estimates that one water-hour is the equivalent to 575 m3 of water, but field variation made the calculation of volume usage imprecise. Thus, water-hour was used as the input value in this study. Indicators for NUE and PUE are expressed as kg grain kg−1 elemental N or P applied, and were calculated from farmer reported fertilizer use. Pesticide use efficiency was calculated using the IRRI field calculator, which computes a score based on the number and timing of herbicide, insecticide, fungicide, rodenticide, and molluscicide applications. Rodenticide and molluscicide were not included, as use of these pesticides is not common. Pesticide use efficiency range from zero to 75 points, with a higher score indicating more sustainable practices. Field GHG emissions were expressed as kg grain kg−1 CO2 equivalent (CO2e). Estimations of methane emissions were made using Tier 1 emission factors given in the IPCC's greenhouse gas inventory methodology (IPCC, 2006) which accounts for flooding duration, irrigation regime, drying events, the timing of organic inputs, and residue burning. Drying events were reported from farmers as periods of aeration of 3 days or more (IPCC, 2006). Methane was adjusted to CO2e by multiplying by a factor of 28.
Focus Group Discussions
In November 2019, two focus groups were conducted within the Ferreñafe irrigation district to present preliminary results from the study and better understand farmers' perceptions of profitable farming systems, barriers to production, and decision-making process regarding crop management inputs. Focus groups are a qualitative research method that allows insight into the complex nature of personal beliefs within the context of the participants' lives (Krueger and Casey, 2014). Focus groups allow farmers to openly engage with ideas and each other in a semi-controlled environment, supporting in-depth discussions on a variety of topics (Onwuegbuzie et al., 2009). Each focus group was semi-structured and lasted ~1.5 h. Farmers in the region were invited from an announcement in the regional irrigation district. The focus group started with a short questionnaire about perceptions of spending, and then a guided group discussion was facilitated by the author and a local translator and agronomy student. Forty-five farmers attended the focus groups, with 22 farmers individually responding to the questionnaire. The moderators presented handouts of figures summarizing the results of the survey and had participants provide feedback and assess the validity of the results by discussing their perceptions of profitable vs. non-profitable systems. Focus groups were recorded and an assistant took notes to emphasize significant themes. This stimulus-based methodology allowed participants to interact with survey results and one another, thereby enabling an in-depth understanding of their perceptions and the barriers preventing the adoption of more profitable or sustainable management practices (Onwuegbuzie et al., 2009).
Data Analysis
To evaluate the potential for optimizing input use and management factors, we used the concept of attainable profitability to compare average- or below-average farmers to those that maximized profitability in the region. Farmers were grouped into three profitability categories based on net profit: top (top 20%), middle (middle 60%), and bottom (bottom 20%). This method was adjusted for a small sample size but based on similar studies exploring yield and resource use efficiency gaps using the top decile (10%) (Samarasinghe, 2003; Lobell et al., 2009; Stuart et al., 2016). Likewise, to analyze possible yield gaps and resource use efficiencies for different SRP performance indicators, relative gaps were calculated among farmers as the percent difference between the mean of the top 20% (target) and the population mean (baseline). Radar charts show the relative difference of SRP indicators between profitability groups, with data normalized to one from the largest value of each variable. A linear mixed effect model was built using lmer() from R package lme4 and analysis of variance (ANOVA) was used to test for significant factors influencing yield. The assumptions of the model were examined for residual normality. Analysis of variance (ANOVA) was performed to test differences between profitability groups for demographics, input use, and performance indicators. Assumptions of normality and homogeneity of variance were evaluated using the Shapiro-Wilk and Levene tests. All statistical analyses were completed with R version 3.4 (RStudio Team, 2020).
Results
Production Practices and Input Use
The majority of farms practiced transplanting for crop establishment (97%, n = 65) with a small number of farmers who practiced manual direct seeding with an average of 100 kg of seed per hectare. Mean farm size was 7.8 ha with mean planting and harvest dates of January 19 and June 2 (Table 1). Farms were characterized by high mean production costs (1,793 $ ha−1), more than 2-fold than production costs observed in flooded regions in Vietnam, Myanmar, Thailand, and Sri Lanka (Devkota et al., 2019). High input use was observed in these same rice producing regions (mean fertilizer rates of 290 kg N ha−1, 31 kg P ha−1, and 35 kg K ha−1). On average, farms also had multiple applications of pesticides (six). Rainfall in this region was minimal during the growing season, ~74 mm (Nguyen et al., 2019), thus farmers relied on multiple irrigations (nine) throughout the growing season. A mean of 17.8 water-hours was purchased, with an estimated volume usage of 10,235 m3 per hectare. At least one soil drying event occurred in 42% of farmers' fields, with some experiencing up to four drying events due to shortages of water and the complexities of irrigation scheduling.
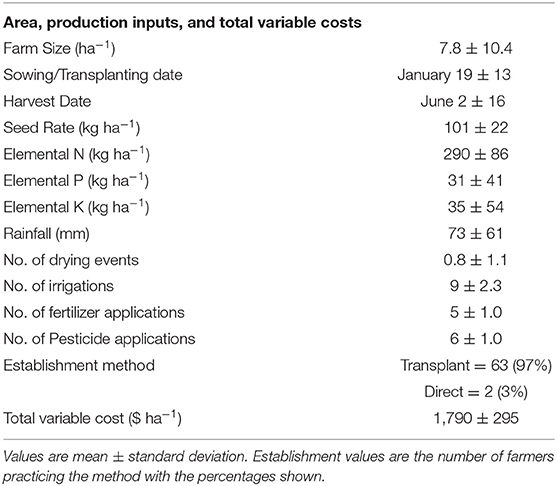
Table 1. Characterization of farm size and production inputs in Ferreñafe, Peru from 65 farmer surveys conducted in 2019.
Yield, Total Variable Cost, Sale Price, and Net Profit
Yield was identified as a major factor driving profitability (R2 = 0.65). The top profitability group had the highest yield (9,482 kg ha−1) with the average yield 26% higher than the middle (6,981 kg ha−1) and 47% higher than the bottom (5,031 kg ha−1) profitability group (Figure 2). Predictor variables explained 30% of yield variation across farms (Supplementary Table 1). In the study regions, planting date was the only variable significantly associated with yield. The top group had the lowest production costs (1,721 $ ha−1) with production costs 15% less than the bottom (2,019 $ ha−1) profitability group. The top group spent 20% less on labor (538 $ ha−1), 24% less on irrigation and fees (146 $ ha−1), and 46% less on pesticides than the bottom profitability group (Figure 3). When accounting for total variable costs, the top group (1,790 $ ha−1) spent 21% less than the bottom (2,266 $ ha−1), due to fewer farmers renting land in the top profitably group. The top group had the highest sale price (0.352 $ kg−1), with a sale price 16% higher than the bottom (0.296 $ kg−1) group. The top group had the highest net profit (1,416 $ ha−1), which was 78% higher than the middle (305 $ ha−1) and 154% higher than the bottom (-768 $ ha−1) profitability group.
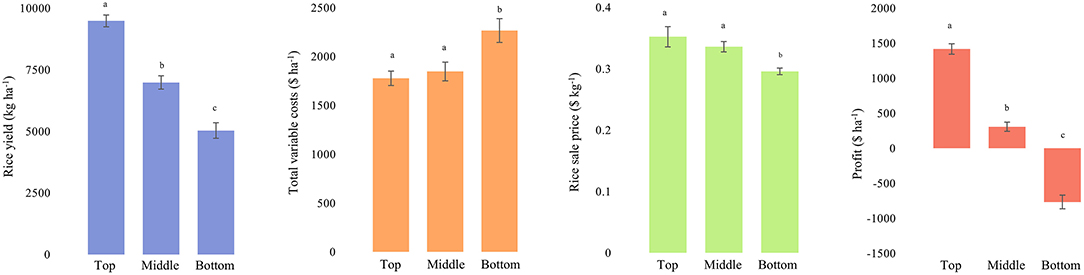
Figure 2. Yield, total variable costs, grain sale price, and net profit per hectare delineated by profit group (top, middle, bottom) for 65 farmers in Ferreñafe, Peru in 2019. Farmers are grouped into profitability categories based on net profit: top (top 20%, n = 13), middle (middle 60%, n = 39), and bottom (bottom 20%, n = 13).
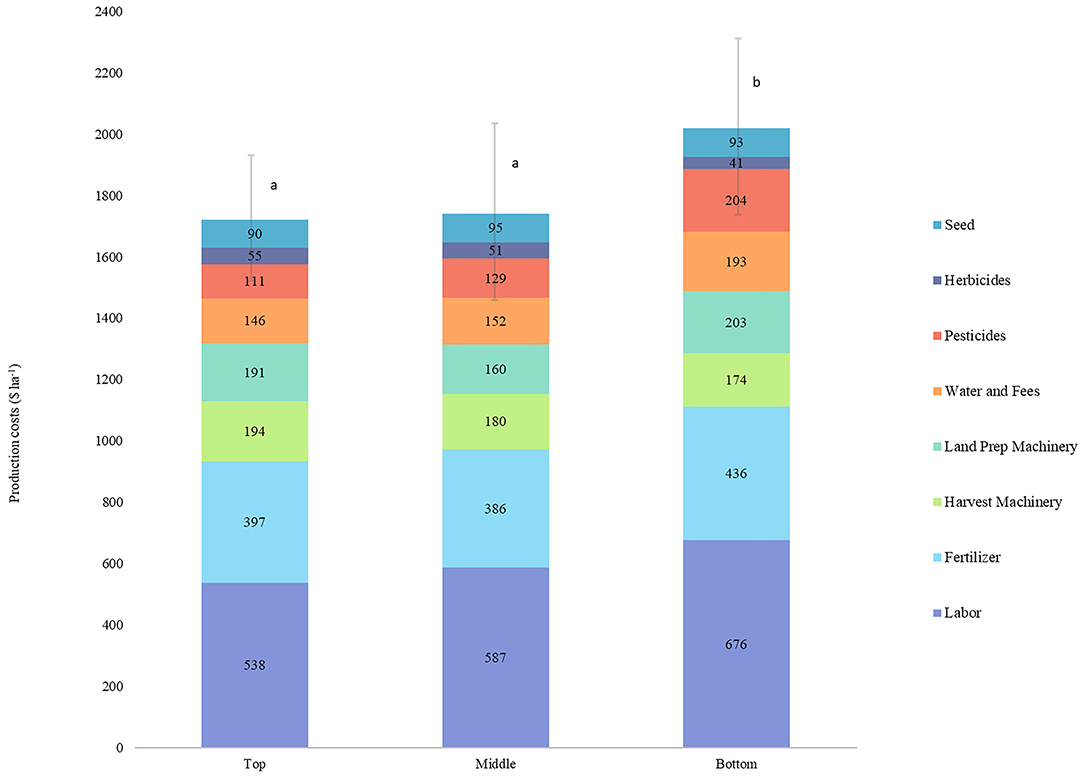
Figure 3. Average production costs delineated by profit groups (top, middle, bottom) for 65 farmers in Ferreñafe, Peru. Farmers are grouped into profitability categories based on net profit: top (top 20%; n = 13), middle (middle 60%, n = 39), and bottom (bottom 20%, n = 13).
SRP Performance Indicators and Resource Use Efficiency Gaps
The largest resource use efficiency gap was for net profit (78%, Figure 4), with a baseline value of 312 $ ha−1 and a target of 1,415 $ ha−1 (Table 2). Profit values ranged from $-1,409 to $2,102 per hectare, with 38% of farmers being unprofitable in this growing season. Net profit was strongly correlated with yield (r = 0.65, p < 0.001). The majority of farmers used fertilizers containing P (75%), and a large P-use efficiency gap (51%) was observed with a baseline value of 273 kg grain kg P−1 ha−1 and a target value of 553 kg grain kg P−1 ha−1. Labor productivity had a 42% gap with a baseline of 12.9 and a target of 22.3 kg grain labor hour−1. There was a 42% gap in water use efficiency with a baseline of 449 and a target of 772 kg grain water-hour−1. Yield-scaled GHG emissions had a 42% gap with a baseline of 1.836 and a target of 3.191 kg grain kgCO2e−1. All farmers surveyed used N fertilizer, and there was a resource gap of 40% with a baseline NUE of 26.3 and a target of 36.3 kg grain kg−1 N. Pesticide use efficiency had a 31% gap with a baseline of 44.5 and a target of 64.5 points. The smallest resource gap observed was grain yield (22%) with a baseline value of 7,091 and a target yield of 9,070 kg ha−1.
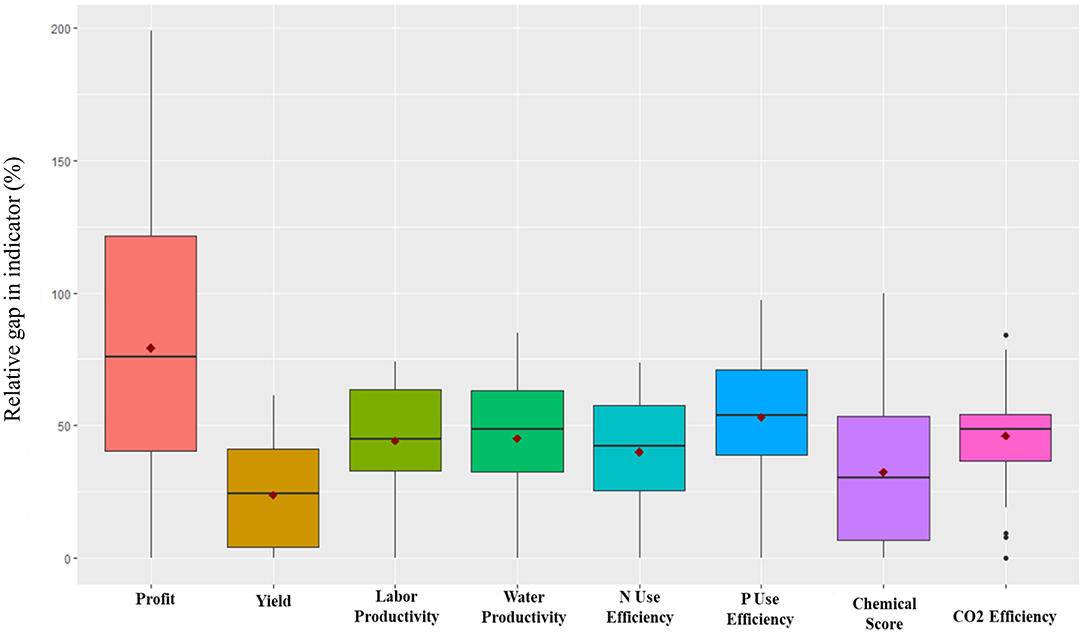
Figure 4. Percent gap for SRP indicators calculated among farmers as the percent difference between the mean of the top 20% (target) and the population mean (baseline) for 65 farmers in Ferreñafe, Peru.
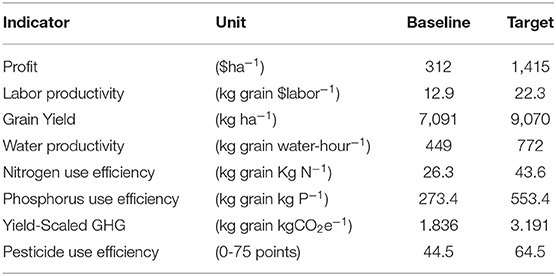
Table 2. Baseline (population mean) and target (mean of top 20% performers: each category) values of performance indicators for 65 farms in Ferreñafe, Peru.
The top profit group generally used lower levels of inputs, except for seed and nitrogen, but achieved higher yields (Figure 5). For example, the top profit group had 22% lower total variable costs (1,777 $ ha−1), 20% lower labor costs (212 $ ha−1), 65% lower elemental P (20 kg ha−1), and 24% fewer chemicals (7.8 chemicals ha−1) than the bottom profitability group. Seed rate was consistent across the profit groups, but the top group used ~10% more N fertilizer (323 kg ha−1) than the bottom group. Tradeoffs between economics and performance indicators were not observed, as the top profit group had 154% higher profits (1,416 $ ha−1), 47% higher yield (9,483 kg ha−1), and 61% higher labor productivity (18 kg grain $ labor−1). The top group also had 44% higher NUE (32 kg grain kg N−1), 59% higher PUE (436 kg grain kg P−1), 56% higher water productivity (615 kg grain water-hour labor−1), and 39% higher yield-scale GHG (2.148 Kg grain kg CO2 equiv−1) than the bottom profitability group.
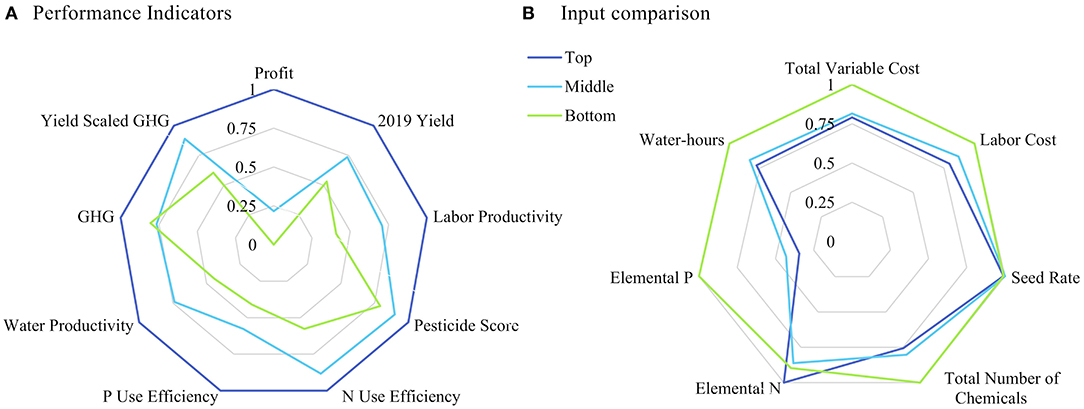
Figure 5. Comparison of SRP indicators (A) and production inputs (B) and among three categories of farmers delineated by profit percentiles in Ferreñafe, Peru for the 2019 season. Farmers are grouped into profitability categories based on net profit: top (top 20%, n = 13), middle (middle 60%, n = 39), and bottom (bottom 20%, n = 13). Data is normalized to 1 based on the largest value for each variable. Profit = ($ ha−1), Yield = (kg ha−1), Labor productivity = (kg grain $labor −1), Pesticide use efficiency = (0-75 points), N use efficiency = (kg grain kg N−1), P use efficiency = (kg grain kg P−1), Water productivity = (kg grain water-hour−1), Yield-Scaled GHG = (kg grain kg CO2 equiv−1), Total variable costs = ($ ha−1), Labor cost = ($ ha−1), Seed rate = (kg ha−1), Total pesticides = (total chemicals), Elemental N = (kg ha−1), Elemental P = (kg ha−1), Irrigation = (water-hours purchased).
Farmers' Perceptions of Production and Profitability
Farmers indicated that being profitable was becoming more difficult, due to increasing economic challenges and production barriers, which limited yields. Frequent water shortages were described as one of the most challenging aspects to rice production in the region. From the farmers surveyed, 78% said that there were occasions during the previous growing season when water was not available when needed. In addition, 58% of farmers said their crop experienced water stress during the vegetative period, while 17% of farmers said their crop experienced water stress during the reproductive phase. Farmers purchase water from the irrigation commission on a per water-hour basis, and the total hours are limited to each farmer based on the number of hectares, non-rice crops grown, and the level of the reservoir in the current season. In recent years, farmers reported that water shortages have become more common due to reduced rainfall and the complexity of irrigation scheduling, causing extended periods of drought conditions in their fields.
The cost of production was also described as a major barrier to profitability. Concerning access to capital, most farmers lack financial resources and access to cheap credit facilities, so they rely on the rice milling industry for high interest loans to finance their production, which ultimately reduces their grain marketing options. Historically, farmers explained that rice farming was profitable, but with increasing production costs and constraints to crop productivity, farmers are unsure about the future of rice farming. From the focus groups, 82% of farmers responded that low production costs were important or very important for increasing profitability (Figure 6). When the same group was questioned about their estimates on the amounts of money a farmer should spend to maximize yield and profit, 23 and 36% of participants responded that farmers should spend more than 1,800 and 2,100 $ ha−1 respectively, both of which are greater than the mean production cost of the top profitability group (1,721 $ ha−1), and the population mean (1,793 $ ha−1). Additionally, when asked if farmers knew the exact amounts they were spending throughout the growing season, 15 farmers responded yes, while seven responded no. In the discussion portion of the focus groups, farmers explained the tension that exists between the pressure to purchase inputs from salespersons, the lack of knowledge in how to use inputs appropriately, and the lack of agricultural extension to provide recommendations. Farmers highlighted the fact that production costs are very high, mainly because the only people they talk to about improving their management are sales people. This creates a scenario where there is an incentive for salespersons to continue a business as usual approach, which does not support low input use, as this would reduce their sales. Farmers feel at odds between improving their management by purchasing additional inputs, and reducing their cost of production. Furthermore, farmers financed by the milling industry claimed that purchases are often made in the field on credit from traveling salespersons, which made it difficult to keep track of expenditures throughout the growing season.
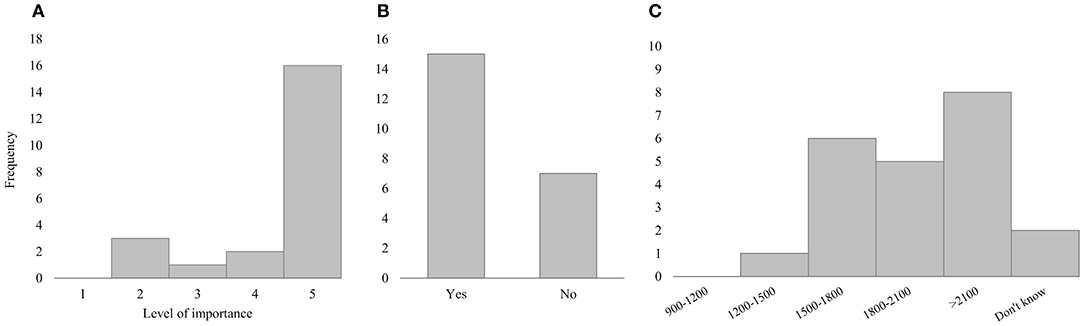
Figure 6. Farmer's responses to questions about perceptions of production costs and profitability from 22 farmers in Ferreñafe, Peru. (A) Farmer's responses to the importance of low production costs for profitability: 1 = not important, 2 = low importance, 3 = neutral, 4 = important, 5 = very important. (B) Farmer's responses (yes or no) to whether they know how much they spend per hectare during the growing season. (C) Farmers response to how much they should spend to maximize profit and yield.
Discussion
Profitability and Decision-making
Our results suggest that rice farmers in this region of Peru can achieve the highest profitability through efforts aimed at increasing yields, marketing grain at the most competitive price, and decreasing the cost of production. The importance of these factors is consistent with the concepts of agricultural development for small-scale farmers, which focuses on improving productivity while reducing input costs (Norton, 2004). It is noteworthy that agronomic management practices, and specifically the level of inputs, were not directly responsible for increasing yields and profitability across farms (discussed below). The strength of our study is that factors influencing profitability were analyzed from survey data, and focus groups revealed additional important insights to the perceptions and decision-making process of farmers. Namely, the underlying explanation of why some farmers were more profitable than others appeared to be primarily related to the decision-making process regarding financing, spending and management decisions. Farmers explained that access to credit for capital expenses and agriculture education was needed meaning that overcoming barriers to higher profitability is as much of a socioeconomic challenge as an agronomic challenge. Below we discuss the major opportunities for improving profitability in this region and the need for research and extension programs that would provide farmers access to knowledge on improved management and technological options. We also discuss the need to not only address yield-limiting factors, but navigate the complex socioeconomic challenges related to credit facilities and market channels. The small sample size in this study is the primary limitation to the generalization of these results, and we suggest that more research and extension efforts are needed working across a large number of farms to identify crop management practices that can reliably increase yield in this region without negatively influencing the SRP performance indicators.
In this study, we identified a significant gap in profitability, where the top farmers (top 20%) were 154% more profitable than the bottom group. This gap was associated with considerable variations in yield and management practices, as well as related production costs. The rice yields were identified as a major factor driving profitability, with the top profitability group having yields 46% higher than the bottom group. This gap in productivity is not uncommon in small-scale rice systems (Affholder et al., 2013; Stuart et al., 2016). In focus groups, farmers explained that water scarcity, pests, and diseases often cause low yields and there is a lack of research extension efforts to help them address these issues. When discussing the rationale behind management decisions leading to high yields, farmers felt strongly that the emphasis during recent decades in Peru has been on increased inputs for higher production. Farmers' management decisions are influenced by subjective beliefs pertaining to risk aversion (Babcock, 1992; Sheriff, 2005; Menapace et al., 2013), hence it is likely that increasing economic uncertainty has contributed to the high seed, nutrient, and agrochemical inputs in this study (Table 1). Combined with a suitable biophysical environment, high input levels may help explain why Peru has the second-highest average yields in Latin America (FAOSTAT, 2020). Nevertheless, farmers discussed that production challenges have changed in recent decades and maintaining high yields is becoming more difficult.
Although the identification of variables leading to yield increases can help establish guidelines for closing yield gaps (Lobell, 2013; Zhang et al., 2016), we found that only planting date was significantly associated with yield. This finding is likely due to the relatively small sample size combined with a large variation in management and crop production outcomes. In addition, farmers reported that yields were lower than average due to disease pressure that was not captured in the survey data. Unfortunately, in this region, planting date is primarily out of farmers' hands, as farmers plant as soon as the water is available in their irrigation branch. Efforts to make water available earlier, to those who receive it the latest, could improve yields. Despite the lack of significant management factors influencing yield, yields were still a driver of profitability.
Further research and extension geared toward closing yield gaps is critical for improving farmer livelihood outcomes (Tittonell and Giller, 2013; Dzanku et al., 2015) without negatively influencing the SRP performance indicators below. In the pursuit of maintaining high yields, farmers explained a tension that exists in the decision-making process of whether to manage more intensively, often in a prophylactic manner, or to risk yield loss by reducing production costs. Interestingly, we found that the top profitability group spent significantly less on production than the bottom profitability group (Figure 2). This result suggests that the top profitability group was optimizing their spending, mainly related to labor, fertilizer, water, and pesticides. Conversely, farmers spending large amounts on production do so at economic risk. For labor, the top profitability group spent 20% less on total labor costs (538 vs. 676 $ ha−1) than the bottom group, with a 20% reduction in transplanting costs (269 vs. 333 $ ha−1), mainly from hiring fewer laborers. In the case of fertilizer, the top profitability group generally spent less (397 vs. 436 $ for NPK inputs), but applied higher rates of N fertilizer in their fields (323 vs. 290 kg N ha−1). These farmers may be optimizing N inputs due to more extensive knowledge of fertilizer management and crop requirements, or possibly better access to higher N fertilizer sources such as urea (46-0-0) compared to the more expensive compound fertilizers (20-20-20) with lower N content. For pesticides, the top profitability group spent 43% less than the bottom group (111 vs. 204 $ ha−1), which could be due to increased knowledge of when pesticides are required or from reduced disease and pest pressure. For water usage and fees, the top profitability group spent 25% less than the bottom group (146 vs. 193 $ ha−1), mainly from using fewer water-hours (16 vs. 21 hours ha−1), although irrigation fees varied based on the cost of cleaning and maintaining the section of the canal where the farm is located. Furthermore, farmers with limited access to purchase water-hours in person, opted to pay an additional price to send someone to purchase water-hours for them.
When these findings were discussed in focus groups, farmers suggested that the top profitability group was more knowledgeable about inputs and their appropriate usage, which allowed them to achieve high yields with lower production costs. This observation is consistent with studies indicating the influence of information and knowledge to the decision-making process, which can benefit famer profitability and sustainable management practices (Feder et al., 1985; Phillips, 1994; Anderson, 2008; Nakano et al., 2018). Also, while yields have increased due to the use of technologies, fewer efforts have been made to teach farmers about technologies that can decrease costs such as direct manual or mechanized seeding. For example, in this region, the overwhelming majority of farmers transplant rice, which represents nearly 50% of labor costs and 16% of total production costs. Farmers that practiced direct seeding, rather than transplanting, saved nearly 300 dollars per hectare. Farmers discussed their reluctance to try direct seeding due to worries about water scheduling and increased weed pressure. Recent research has highlighted the benefits of direct seeding in Peru (Pando et al., 2017), but there is a need to increase extension opportunities to inform farmers about specific production or post-production options that could generate higher profits.
Another driving factor for profit was farmers selling their grain for higher prices. This was accomplished by either selling their grain first after the start of harvest, price shopping among different mills, or milling their rice and then selling to retailers. However, these options are not feasible for most farmers who do not have sufficient capital and rely on external loans to cover production costs each season. The majority of farmers (74%) sold their paddy at the farm gate immediately after harvest. This practice is common in rice-growing regions and is consistent with research highlighting that small-scale rice farmers with limited access to market channels are more likely to sell at the farm gate for lower prices (Cazzuffi and McKay, 2012; Muhunyu, 2012; Soe et al., 2015). While not as profitable, this arrangement allows farmers to be paid quickly, and it reduces the costs associated with drying, storing, or milling. Of the 65 farmers surveyed, 43 were financed by the milling operations, which typically has the stipulation of selling the paddy at the farm gate. Furthermore, if farmers enter into debt with a given milling operation, a cycle of reduced market choices ensues until the farmer pays off debt or decapitalization occurs. Ultimately, this relationship reinforces a lack of bargaining power, which makes farmers vulnerable to exploitation and further reduces the farmer's sense of agency. In our focus groups, farmers expressed a desire to be organized so they could protect themselves from low prices and aggressive financial intervention from the milling operations. Farmers in this region have concerns about the future of rice production due to the influence of the milling operations over input use and sale prices. Many studies suggesting that small-scale farmers lacking power, negotiating capacity, and political representation would benefit from a farmer organization (Stockbridge et al., 2003; Alene et al., 2008; Aku et al., 2018), although the success of farmer organizations is mixed (Key and Runsten, 1999; Masakure and Henson, 2005; Thorp et al., 2005). In addition to the sale price, farmers desired additional options for credit, including access to regulated long-term credit, and research efforts concentrated toward helping farmers make educated management decisions will likely boost their confidence.
Resource Use Efficiencies and Prospects for Sustainable Intensification
To achieve the dual goals of enhancing food security and environmental performance, yield increases cannot come at the cost of lower resource use efficiencies. In this study, we observed a wide range of input levels and resource use efficiencies for eight SRP performance indicators across the three profitability groups (Table 3, Figure 4), indicating significant room for improvement among farmers. Notably, the top profitability group used lower rates of inputs, with the exception of N fertilizer and seed, suggesting that intensive use of inputs does not translate to increased yield but rather economic and environmental risk. As discussed below, this finding goes against the common assumption that high yield farming, which is associated with profitability, is often viewed synonymously with environmental degradation (Foley et al., 2011).
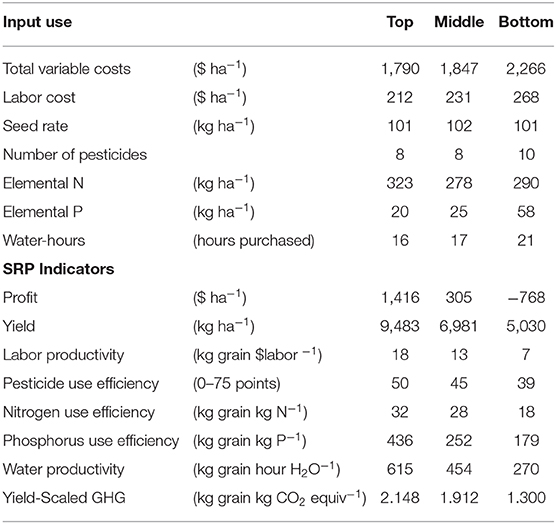
Table 3. Production inputs and SRP indicators among three categories of farmers delineated by profit percentile in Ferreñafe, Peru for the 2019 season.
In the case of P fertilizer and chemical applications, lack of recommendations and farmer knowledge appeared to be the basis for the highest rates of input use. Farmers that chose to use compound fertilizers, in a bag-for-bag manner, applied excessively high P rates at a higher cost and environmental risk. Extension efforts aimed at helping farmers understand nutrient requirements and the elemental composition of fertilizer will help reduce over or under application of fertilizers and potentially increase profitability. Similar programs have been effective in optimizing fertilizer efficiency for increasing environmental sustainability and profitability in rice production elsewhere (Pingali et al., 1998; Huang et al., 2008; Emmanuel et al., 2016). For pesticide use efficiency, the top profitability group used 25% fewer chemicals than the bottom group. Many famers applied prophylactic pesticides or took recommendations from sales representatives that consisted of tank mixes of several chemicals applied multiple times over the season. Ultimately, many farmers felt conflicted between their knowledge gaps in the use of pesticides, the rising costs of such products, and the desire to protect yields. Extension efforts geared toward integrated pest management will help farmers make informed choices, and potentially reduce the environmental footprint of these systems.
For both labor and water use, we found that the top profitability group used less than the middle and bottom profit groups. This practice translates to lower production costs and reduced input use and environmental footprint. We hypothesize that this is due to the level of involvement the farmer has in day-to-day management activities. Production activities such as transplanting and irrigation require in-depth understanding and involvement of the activity, field, and community. An earlier study (Abdulai and Huffman, 1998) found that small-scale rice farmers engaged in non-farm activities tended to exhibit higher levels of inefficiency due to a reduction of time allocated to farm work. Similarly, our focus group discussions highlighted that many farmers are managing their fields while also focusing on other means of employment. If rice production continues to be viewed as a poor or risky way to make a living, then production efficiencies may decrease as farmers continually shift their resources to find more profitable means of work. A reduction in the farmer population is a trend that is not only happening in rice systems but agriculture worldwide (McNally, 2002; Phimister and Roberts, 2006; Rigg, 2006; Nasir and Kotu, 2014).
Our research shows that the top profitability group achieved the highest scores for all SRP performance indicators. In the case of labor productivity, pesticide use efficiency, PUE, and water productivity, a combination of high yields and low input use was a driving factor for these high efficiency rates. For NUE, the top profitability group applied higher N rates, but still were 40% more efficient than the bottom group due to achieving higher yields. Likewise, for GHG emissions, the top profitability group also had higher methane emissions per unit area, but 40% lower emissions than the bottom group when expressed per unit production. Consistent with our findings, previous studies have shown that measuring the efficiencies of farm systems by per unit area of farmland underestimates the fact that lower-yield systems require more land per unit yield (Balmford et al., 2018). A recent study assessing SRP indicators in six countries (Devkota et al., 2019), observed that high yielding farmers in Indonesia used higher rates of inputs, and were more efficient in their use, with higher indicator scores. Similarly, in Guangdong, China, Devkota et al. (2019) observed a similar synergy between high yields and high SRP indicators.
All farms in the target group (i.e., top 20%) for GHG efficiency experienced at least one period of drying in their field, which can reduce estimated methane emissions by 48% (IPCC, 2006). These drying periods constitute a significant cause of stress for farmers, as they are not planned but occur due to limited control over irrigation scheduling and community water use. Still, the farmers with the highest GHG efficiency were able to maintain yields while experiencing drying events, which is consistent with studies showing that alternate wetting and drying (AWD) can significantly reduce methane emissions without yield loss (Linquist et al., 2015; Xu et al., 2015; Liang et al., 2016; Setyanto et al., 2018). Recent research (Pando et al., 2017) has demonstrated AWD and direct seeding as successful options in northern Peru, but changes in water distribution and suitable cultivars that tolerate drying periods are needed. Extension efforts demonstrating effective AWD and efficient weed management could change the perception that drying events are harmful for the crop. If farmers view this as a viable technology rather than an insurmountable problem, farmers could likely regain a sense of confidence, which is a critical aspect of the farmer decision-making process.
Our results are the first to benchmark resource efficiency gaps for rice production in Latin America using SRP indicators, which is an essential step for identifying options for sustainable intensification. While previous work in other countries used similar sample sizes to assess SRP indicators (Devkota et al., 2019), we recognize there are limitations to interviewing 65 farmers and we encourage more extensive assessments in Peru and other countries. Overall, synergies were found between crop production and sustainability goals because the top profitability group used fewer inputs, achieved the highest yields, and scored the highest across all SRP performance indicators. While this provides necessary evidence that sustainable intensification can be achieved at the farm-level, there is still considerable room for improvement for the majority of farmers across SRP indicators (resource use efficiency gaps ranged 22-77%). Indeed, the largest gap was for profitability, underscoring the need for efforts aimed at helping farmers manage inputs wisely while simultaneously increasing yields. Our results suggest that farmers are capable of achieving high levels of profitability and productivity at low environmental costs per unit production.
Conclusions
Globally, agriculture faces the challenge of balancing the need to feed a growing population, improving the livelihoods of farmers, and protecting the environment for long-term sustainability. In this study, we provide a holistic assessment of these challenges for rice production in Peru by analyzing potential synergies and tradeoffs across multiple performance indicators, while also exploring the farmer decision-making process. Our results indicate that the factors driving profitability are yield, market price, and production costs. However, socio-ecological factors such as farmer access to knowledge and financing also impact how rice production systems are managed and specifically the level of inputs and costs of production, influencing both economic and environmental footprint. These findings are essential to inform future research and extension in this region, which should concentrate efforts toward identifying improved agronomic practices to increase yield under on-farm conditions, while also recognizing the role of economic and socio-ecological factors that constrain farm management practices. This study indicated the absence of tradeoffs between profit, yield, and environmental performance per unit of production, which suggests a synergy can exist between simultaneous gains in resource efficiency and profitability. Management practices such as AWD and direct seeding have the potential to change farmers' perceptions of water use and reduce production costs, to increase farm profitability while reducing GHG emissions. Therefore, our results are critical for exploring pathways for achieving sustainable development goals, and to help policy-makers prioritize investments in agriculture and food security.
Data Availability Statement
The raw data supporting the conclusions of this article will be made available by the authors, without undue reservation.
Ethics Statement
The studies involving human participants were reviewed and approved by The Urbana-Champaign Office for the Protection of Research Subjects (OPRS) and Institutional Review Board (IRB). Written informed consent for participation was not required for this study in accordance with the national legislation and the institutional requirements.
Author Contributions
MW and CP conceived the research idea. MW carried out the experiment, data analysis with supervision from CP, EH, EG, and NC, and led the writing of the manuscript, with all authors providing edits and input to the analysis and final version. All authors participated in the design of study objectives, the survey tool, helped interpret results, and develop conclusions.
Funding
This research was funded by the Crop Sciences Department of the University of Illinois Urbana-Champaign. Additional funding was awarded through The Global Food Security Graduate Fellowship from the ACES Office of International Programs and International Food Security at Illinois.
Conflict of Interest
The authors declare that the research was conducted in the absence of any commercial or financial relationships that could be construed as a potential conflict of interest.
Acknowledgments
The authors are thankful to the Ferreñafe irrigation district in Peru and the many farmers who shared data and helpful insights. Robert Andrade (CIAT), Sergio Urioste (CIAT), Diego Marin (CIAT), and Moises Bustamante provided valuable input on the creation and implementation of the survey tool for this research. Thank you to Sandra Loaiza and Rossana Porras Jorge for input on the creation of the map. The authors would also like to thank Andrew Margenot and Hope Michelson at the University of Illinois for critical feedback on this work.
Supplementary Material
The Supplementary Material for this article can be found online at: https://www.frontiersin.org/articles/10.3389/fsufs.2020.564418/full#supplementary-material
References
Abdulai, A., and Huffman, W. (1998). An Examination of Profit Inefficiency of Rice Farmers in Northern Ghana. Staff General Research Papers. Econ. Staff Pap. Ser. 298, Iowa State University, Department of Economics. Available online at: https://lib.dr.iastate.edu/econ_las_staffpapers/298
Affholder, F., Poeydebat, C., Corbeels, M., Scopel, E., and Tittonell, P. (2013). The yield gap of major food crops in family agriculture in the tropics: assessment and analysis through field surveys and modelling. Field Crops Res. 143, 106–118. doi: 10.1016/j.fcr.2012.10.021
Aku, A., Mshenga, P., Afari-Sefa, V., and Ochieng, J. (2018). Effect of market access provided by farmer organizations on smallholder vegetable farmer's income in tanzania. Cogent Food Agric. 4:1560596. doi: 10.1080/23311932.2018.1560596
Alene, A. D., Manyong, V. M., Omanya, G., Mignouna, H. D., Bokanga, M., and Odhiambo, G. (2008). Smallholder market participation under transactions costs: maize supply and fertilizer demand in Kenya. Food Policy 33, 318–328. doi: 10.1016/j.foodpol.2007.12.001
Anderson, J. R. (2008). Agricultural Advisory Services. Washington, DC: World Bank. Available online at: https://openknowledge.worldbank.org/handle/10986/9041
Babcock, B. (1992). The effects of uncertainty on optimal nitrogen applications. Rev. Agric. Econ. 14, 271–280. doi: 10.2307/1349506
Balmford, A., Amano, T., Bartlett, H., Chadwick, D., Collins, A. L., Edwards, D., et al. (2018). The environmental costs and benefits of high-yield farming. Nat. Sustain. 1, 477–485 doi: 10.1038/s41893-018-0138-5
Biernacki, P., and Waldorf, D. (1981). Snowball sampling: problems and techniques of chain referral sampling. Sociol. Methods Res. 10, 141–163. doi: 10.1177/004912418101000205
Bouman, B., Lampayan, R. M., and Tuong, T. M. (2007). Water Management in Irrigated Rice. Coping with Water Scarcity. (Manila, Philippines: International Rice Research Institute).
Byerlee, D. R., and Anderson, J. R. (1982). Risk, utility and the value of information in farmer decision making. Rev. Market. Agric. Econ. 50:12524.
Carlson, K. M., Gerber, J. S., Mueller, N. D., Herrero, M., MacDonald, G. K., Brauman, K. A., et al. (2016). Greenhouse gas emissions intensity of global croplands. Nat. Clim. Change 7, 63–68. doi: 10.1038/nclimate3158
Cazzuffi, C., and McKay, A. (2012). Rice Market Participation and Channels of Sale in Rural Vietnam (No. 1007-2016-79533). AgEcon Search. doi: 10.22004/ag.econ.126566
De Roos, A. J., Zahm, S., Cantor, K., Weisenburger, D., Holmes, F., Burmeister, L., et al. (2003). Integrative assessment of multiple pesticides as risk factors for non-hodgkin's lymphoma among men. Occup. Environ. Med. 60:e11. doi: 10.1136/oem.60.9.e11
Devkota, K. P., Pasuquin, E., Elmido-Mabilangan, A., Dikitanan, R., Singleton, G. R., Stuart, A. M., et al. (2019). Economic and environmental indicators of sustainable rice cultivation: a comparison across intensive irrigated rice cropping systems in Six Asian countries. Ecol. Indic. 105, 199–214. doi: 10.1016/j.ecolind.2019.05.029
Dono, G., Cortignani, R., Doro, L., Giraldo, L., Ledda, L., Pasqui, M., et al. (2013). Adapting to uncertainty associated with short-term climate variability changes in irrigated mediterranean farming systems. Agric. Syst. 117, 1–12. doi: 10.1016/j.agsy.2013.01.005
Dzanku, F. M., Jirström, M., and Marstorp, H. (2015). Yield gap-based poverty gaps in rural Sub-Saharan Africa. World Dev. 67, 336–362. doi: 10.1016/j.worlddev.2014.10.030
Edwards-Jones, G. (2006). Modelling farmer decision-making: concepts, progress and challenges. Anim. Sci. 82, 783–790. doi: 10.1017/ASC2006112
Emmanuel, D., Owusu-Sekyere, E., Owusu, V., and Jordaan, H. (2016). Impact of agricultural extension service on adoption of chemical fertilizer: implications for rice productivity and development in Ghana. NJAS-Wagen. J. Life Sci. 79, 41–49. doi: 10.1016/j.njas.2016.10.002
Fageria, N. K., and Baligar, V. C. (2005). Enhancing nitrogen use efficiency in crop plants. Adv. Agron. 88, 97–185. doi: 10.1016/S0065-2113(05)88004-6
FAOSTAT (2020). Retrieved from: http://www.fao.org/faostat/en/#data (accessed January 12, 2020).
Feder, G., Just, R. E., and Zilberman, D. (1985). Adoption of agricultural innovations in developing countries: a survey. Econ. Dev. Cult. Change 33, 255–298. doi: 10.1086/451461
Foley, J. A., Ramankutty, N., Brauman, K. A., Cassidy, E. S., Gerber, J. S., Johnston, M., et al. (2011). Solutions for a cultivated planet. Nature 478, 337–342. doi: 10.1038/nature10452
Godfray, H. C. J., and Garnett, T. (2014). Food security and sustainable intensification. Philos. Trans. R Soc. B Biol. Sci. 369:20120273. doi: 10.1098/rstb.2012.0273
Huang, J., Hu, R., Cao, J., and Rozelle, S. (2008). Training programs and in-the-field guidance to reduce China's overuse of fertilizer without hurting profitability. J. Soil Water Conserv. 63, 165A−167A. doi: 10.2489/jswc.63.5.165A
IPCC (2006). “Guidelines for National greenhouse gas inventories. Volume 4: agriculture, forestry and other land use, Ch 5: Cropland,” in National Greenhouse Gas Inventories Programme, eds H. S. Eggleston, L. Buendia, K. Miwa, T. Ngara, and K. Tanabe (Hayama: Institute for Global Environmental Strategies), 66.
Key, N., and Runsten, D. (1999). Contract farming, smallholders, and rural development in Latin America: the organization of agroprocessing firms and the scale of outgrower production. World Dev. 27, 381–401. doi: 10.1016/S0305-750X(98)00144-2
Khush, G. S. (2005). What it will take to feed 5.0 Billion rice consumers in 2030. Plant Mol. Biol. 59, 1–6. doi: 10.1007/s11103-005-2159-5
Krueger, R. A., and Casey, M. A. (2014). Focus Groups: A Practical Guide for Applied Research. SAGE Publications.
Liang, K., Zhong, X., Huang, N., Lampayan, R. M., Pan, J., Tian, K., et al. (2016). Grain yield, water productivity and ch4 emission of irrigated rice in response to water management in South China. Agric. Water Manag. 163, 319–331. doi: 10.1016/j.agwat.2015.10.015
Linquist, B. A., Anders, M. M., Adviento-Borbe, M. A. A., Chaney, R. L., Nalley, L. L., da Rosa, E. F. F., et al. (2015). Reducing greenhouse gas emissions, water use, and grain arsenic levels in rice systems. Glob. Change Biol. 21, 407–417. doi: 10.1111/gcb.12701
Lobell, D. B. (2013). The use of satellite data for crop yield gap analysis. Field Crops Res. 143, 56–64. doi: 10.1016/j.fcr.2012.08.008
Lobell, D. B., Cassman, K. G., and Field, C. B. (2009). Crop yield gaps: their importance, magnitudes, and causes. Ann. Rev. Environ. Resour. 34, 179–204. doi: 10.1146/annurev.environ.041008.093740
Maclean, J., Hardy, B., and Hettel, G. (2013). Rice Almanac, 4th Edition: Source Book for One of the Most Important Economic Activities on Earth. IRRI
Mankad, A. (2016). psychological influences on biosecurity control and farmer decision-making. a review. Agron. Sustain. Dev. 36:40. doi: 10.1007/s13593-016-0375-9
Masakure, O., and Henson, S. (2005). Why do small-scale producers choose to produce under contract? lessons from nontraditional vegetable exports from Zimbabwe. World Dev. 33, 1721–1733. doi: 10.1016/j.worlddev.2005.04.016
McNally, S. (2002). Are ‘Other Gainful Activities’ on farms good for the environment? J. Environ. Manag. 66, 57–65. doi: 10.1006/jema.2002.0576
Meijer, S. S., Catacutan, D., Ajayi, O. C., Sileshi, G. W., and Nieuwenhuis, M. (2015). The role of knowledge, attitudes and perceptions in the uptake of agricultural and agroforestry innovations among smallholder farmers in Sub-Saharan Africa. Int. J. Agric. Sustain. 13, 40–54. doi: 10.1080/14735903.2014.912493
Menapace, L., Colson, G., and Raffaelli, R. (2013). Risk aversion, subjective beliefs, and farmer risk management strategies. Am. J. Agric. Econ. 95, 384–389. doi: 10.1093/ajae/aas107
Muhunyu, J. G. (2012). Is doubling rice production in Kenya by 2018 achievable? J. Dev. Sustain. Agric. 7, 46–54. doi: 10.11178/jdsa.7.46
Nakano, Y., Tsusaka, T. W., Aida, T., and Pede, V. O. (2018). Is farmer-to-farmer extension effective? the impact of training on technology adoption and rice farming productivity in Tanzania. World Dev. 105, 336–351. doi: 10.1016/j.worlddev.2017.12.013
Nakazi, F., and Sserunkuuma, D. (2013). Factors affecting the decision and extent of rice-milling before sale among ugandan farmers. Asian J. Agric. Rural Dev. 3, 1–8. doi: 10.22004/ag.econ.198235
Nasir, M., and Kotu, B. H. (2014). The effect of off farm employment on agricultural production and productivity: evidence from gurage zone of southern Ethiopia. J. Econ. Sustain. Dev. 5, 85–98. Available online at: https://cgspace.cgiar.org/handle/10568/76047
Nguyen, P., Shearer, E. J., Tran, H., Ombadi, M., Hayatbini, N., Palacios, T., et al. (2019). The CHRS data portal, an easily accessible public repository for persiann global satellite precipitation data. Sci. Data 6:180296. doi: 10.1038/sdata.2018.296
Norton, R. D. (2004). Agricultural development policy: Concepts and experiences. Agricultural Development Policy: Concepts and Experiences. Available online at: https://www.cabdirect.org/cabdirect/abstract/20056701982
Onwuegbuzie, A. J., Dickinson, W. B., Leech, N. L., and Zoran, A. G. (2009). A qualitative framework for collecting and analyzing data in focus group research. Int. J. Qual. Methods 8, 1–21. doi: 10.1177/160940690900800301
Pando, L. R. G., Villacorta, H. S., Peralta, G. S., and Aguilar, E. C. H. (2017). Siembra directa: una alternativa para mejorar la sustentabilidad del cultivo de arroz (Oryza sativa L.) en el Perú. Producción Agropecuaria y Desarrollo Sostenible 6, 13–26. doi: 10.5377/payds.v6i0.5716
Papademetriou, M. K., Dent, F. J., and Herath, E. M. (2000). Bridging the Rice Yield Gap in the Asia-Pacific Region: This Publication Brings Together Edited Manuscripts of Papers Presented at the Expert Consultation on “Bridging the Rice Yield Gap in Asia and the Pacific.” Bangkok: FAO. Retrieved from: http://www.fao.org/publications/card/es/c/6f856aa2-aa27-58e5-93d6-fa4ff0a076f8/ (accessed October 8, 2020).
Phillips, J. M. (1994). Farmer education and farmer efficiency: a meta-analysis. Econ. Dev. Cult. Change 43, 149–165. doi: 10.1086/452139
Phimister, E., and Roberts, D. (2006). The effect of off-farm work on the intensity of agricultural production. Environ. Resour. Econ. 34, 493–515. doi: 10.1007/s10640-006-0012-1
Pimentel, D., Berger, B., Filiberto, D., Newton, M., Wolfe, B., Karabinakis, E., et al. (2004). Water resources: agricultural and environmental issues. BioScience 54, 909–918. doi: 10.1641/0006-3568(2004)0540909:WRAAEI2.0.CO
Pingali, P. L., Hossain, M., Pandey, S., and Price, L. L. (1998). Economics of nutrient management in asian rice systems: towards increasing knowledge intensity. Field Crops Res. 56, 157–176. doi: 10.1016/S0378-4290(97)00126-3
Reardon, T., Barrett, C. B., Berdegué, J. A., and Swinnen, J. F. M. (2009). Agrifood industry transformation and small farmers in developing countries. World Dev. 37, 1717–1727. doi: 10.1016/j.worlddev.2008.08.023
Rigg, J. (2006). Land, farming, livelihoods, and poverty: rethinking the links in the Rural South. World Dev. 34, 180–202. doi: 10.1016/j.worlddev.2005.07.015
Rosenbaum, R. K., Bachmann, T. M., Gold, L. S., Huijbregts, M. A. J., Jolliet, O., Juraske, R., et al. (2008). USEtox—the UNEP-SETAC toxicity model: recommended characterisation factors for human toxicity and freshwater ecotoxicity in life cycle impact assessment. Int. J. Life Cycle Assess. 13, 532–546. doi: 10.1007/s11367-008-0038-4
Samarasinghe, G. B. (2003). Growth and yields of Sri Lanka's major crops interpreted from public domain satellites. Agric. Water Manag. 58, 145–157. doi: 10.1016/S0378-3774(02)00130-0
Seck, P. A., Diagne, A., Mohanty, S., and Wopereis, M. C. S. (2012). Crops that feed the world 7: rice. Food Secur. 4, 7–24. doi: 10.1007/s12571-012-0168-1
Setyanto, P., Pramono, A., Adriany, T. A., Susilawati, H. L., Tokida, T., Padre, A. T., et al. (2018). Alternate wetting and drying reduces methane emission from a rice paddy in central java, indonesia without yield loss. Soil Sci. Plant Nutr. 64, 23–30. doi: 10.1080/00380768.2017.1409600
Sheriff, G. (2005). Efficient waste? why farmers over-apply nutrients and the implications for policy design. Appl. Econ. Perspect. Policy 27, 542–557. doi: 10.1111/j.1467-9353.2005.00263.x
Soe, W. P. P., Moritaka, M., and Fukuda, S. (2015). An analysis of the factors influencing marketing channel choice by paddy rice farmers in Myanmar. J. Faculty Agric. 60, 535–542.
Stevenson, J. R., Villoria, N., Byerlee, D., Kelley, T., and Maredia, M. (2013). Green revolution research saved an estimated 18 to 27 million hectares from being brought into agricultural production. Proc. Natl. Acad. Sci. U. S. A. 110, 8363–8368. doi: 10.1073/pnas.1208065110
Stockbridge, M., Dorward, A., and Kydd, J. (2003). Farmer Organizations for Market Access: A Briefing Paper.
Stuart, A. M., Devkota, K. P., Sato, T., Pame, A. R. P., Balingbing, C., Phung, N. T. M., et al. (2018). On-farm assessment of different rice crop management practices in the Mekong Delta, Vietnam, using sustainability performance indicators. Field Crops Res. 229, 103–114. doi: 10.1016/j.fcr.2018.10.001
Stuart, A. M., Pame, A. R. P., Silva, J. V., Dikitanan, R. C., Rutsaert, P., Malabayabas, A. J. B., et al. (2016). Yield gaps in rice-based farming systems: insights from local studies and prospects for future analysis. Field Crops Res. 194, 43–56. doi: 10.1016/j.fcr.2016.04.039
Sustainable Rice Platform (2015). Available online at: http://www.sustainablerice.org/assets/docs/SRP%20Standard%20for%20Sustainable%20Rice%20Cultivation%20v%201.0.pdf. (accessed March 26, 2020).
Thorp, R., Stewart, F., and Heyer, A. (2005). When and how far is group formation a route out of chronic poverty? World Dev. 33, 907–920. doi: 10.1016/j.worlddev.2004.09.016
Tittonell, P., and Giller, K. E. (2013). When yield gaps are poverty traps: the paradigm of ecological intensification in african smallholder agriculture. Field Crops Res. 143, 76–90. doi: 10.1016/j.fcr.2012.10.007
Toriyama, K., Heong, K. L., and Hardy, B., (eds.). (2005). “Rice is life: scientific perspectives for the 21st century,” in Proceedings of the World Rice Research Conference held in Tokyo and Tsukuba, Japan (Los Baños: International Rice Research Institute; Tsukuba: Japan International Research Center for Agricultural Sciences), 590.
Van Ittersumvan, M. K., and Rabbinge, R. (1997). Concepts in production ecology for analysis and quantification of agricultural input-output combinations. Field Crops Res. 52, 197–208. doi: 10.1016/S0378-4290(97)00037-3
Van Nguyen, N., and Ferrero, A. (2006). Meeting the challenges of global rice production. Paddy Water Environ. 4, 1–9. doi: 10.1007/s10333-005-0031-5
Watcharapongchai, A., Yooprasert, B., and Keowan, B. (2018). Erd area based management extension for sustainable rice production: lessons learned from sustainable rice farmers groups in Det Udom District, Ubon Ratchathani Province. Int. J. Environ. Rural Dev. 9, 1–6.
Wiggins, S., Kirsten, J., and Llambí, L. (2010). The future of small farms. World Dev. 38, 1341–1348. doi: 10.1016/j.worlddev.2009.06.013
Wilson, R. S., Hooker, N., Tucker, M., LeJeune, J., and Doohan, D. (2009). Targeting the farmer decision making process: a pathway to increased adoption of integrated weed management. Crop Protect. 28, 756–764. doi: 10.1016/j.cropro.2009.05.013
Xu, Y., Ge, J., Tian, S., Li, S., Nguy-Robertson, A. L., Zhan, M., et al. (2015). Effects of water-saving irrigation practices and drought resistant rice variety on greenhouse gas emissions from a no-till paddy in the Central Lowlands of China. Sci. Total Environ. 505, 1043–1052. doi: 10.1016/j.scitotenv.2014.10.073
Keywords: rice, environmental performance, Peru, small-scale, economics, sustainability, SRP, perceptions
Citation: White M, Heros E, Graterol E, Chirinda N and Pittelkow CM (2020) Balancing Economic and Environmental Performance for Small-Scale Rice Farmers in Peru. Front. Sustain. Food Syst. 4:564418. doi: 10.3389/fsufs.2020.564418
Received: 21 May 2020; Accepted: 22 September 2020;
Published: 30 October 2020.
Edited by:
Rebecca Chaplin-Kramer, Stanford University, United StatesReviewed by:
Julie Gwendolin Zaehringer, University of Bern, SwitzerlandKikuko Shoyama, National Research Institute for Earth Science and Disaster Resilience (NIED), Japan
Copyright © 2020 White, Heros, Graterol, Chirinda and Pittelkow. This is an open-access article distributed under the terms of the Creative Commons Attribution License (CC BY). The use, distribution or reproduction in other forums is permitted, provided the original author(s) and the copyright owner(s) are credited and that the original publication in this journal is cited, in accordance with accepted academic practice. No use, distribution or reproduction is permitted which does not comply with these terms.
*Correspondence: Marshal White, bWFyc2h3aGl0ZTg1QGdtYWlsLmNvbQ==