- 1Laboratorio de Genética de la Conservación, Jardín Botánico, Instituto de Biología, Universidad Nacional Autónoma de México, Ciudad de México, Mexico
- 2Laboratorio Nacional de Ciencias de la Sostenibilidad, Instituto de Ecología, Universidad Nacional Autónoma de México, Ciudad de México, Mexico
- 3Red de Estudios Moleculares Avanzados – Campus III, Instituto de Ecología, A.C., Xalapa, Mexico
- 4Laboratorio de Edafología Ambiental, Instituto de Geología, Universidad Nacional Autónoma de México, Ciudad de México, Mexico
- 5Centro de Ciencias de la Complejidad (C3), Universidad Nacional Autónoma de México, Ciudad de México, Mexico
Incorporating the views and perceptions of local farmers and other actors with stakes in agricultural production is critical for better-informed decision making and tackling pressing issues, such as soil degradation. We conducted a study that sought to integrate and analyze perceptions regarding the quality and degradation of agricultural soils across different social sectors in Mexico, including producers of two annual crops (maize and beans) and two perennial crops (coffee and avocado), members of civil society organizations and members of the Federal Government. We analyzed the community perception using Cognitive Maps and network metrics. Our fully documented method to formally gather and analyze local and regional perceptions can be used in future efforts toward the collective design of sustainable food systems. Our results highlighted common and potentially conflicting aspects among the different perceptions and allowed us to identify and discuss drivers and processes of special interest in different regions in Mexico. This study also contributes to a deeper understanding of the current situation of agricultural soils in Mexico and seeks to inform the decision-making process regarding agricultural management in the country.
Introduction
Given the prevalence of unsustainable production systems (Lambin et al., 2001), and in the face of climate change, agricultural systems should increasingly adopt production modes that make an efficient use of natural resources and improve soil quality (Pannell and Schilizzi, 1999; Schmidhuber and Tubiello, 2007). In particular, agricultural soils over the world exhibit severe levels of degradation (Jie et al., 2002). In Mexico, from 45 to 63% of the soils are estimated to exhibit some degree of degradation (SEMARNAT-COLPOS, 2002; CONAFOR-UACH, 2013), jeopardizing food production and several ecosystem services. In order to successfully manage natural resources and increase soil quality, understood as “the capacity of a soil to function within ecosystem boundaries to sustain biological productivity, maintain environmental quality, and promote plant and animal health” (SSSA, 1997), it is critical to integrate the knowledge and perception of diverse social actors, such as local communities, academics and decision makers (Prell et al., 2009; Stone-Jovicich et al., 2011). Indeed, it is widely recognized that natural resource management decisions are better taken after very diverse interactions between different actors and across local to national levels (Buckles, 1999). However, most of the policies and approaches designed for large-scale food production and soil conservation have not considered the needs and points of view of rural communities and the intricate socio-cultural context of pluricultural countries, such as Mexico (Tripp, 2001; Camacho-Villa et al., 2016; CEMDA, 2016). Decision-making processes on agroecosystems1 involve complex interactions among local, regional and global factors (e.g., Barlett, 1980; Dury et al., 2010; Martin-Clouaire, 2017) and decisions cannot be grounded solely on scientific (Soleri et al., 2000) or economic (Ilbery, 1978) perspectives. Local and traditional knowledge and management practices are the base of agroecosystem resilience (Altieri et al., 2012; Gavin et al., 2015), so this knowledge should be included in the design of policies along with scientific knowledge. Thus, the incorporation of the view and perceptions of local food producers and other actors with stakes in agricultural production is critical for better-informed decision making and sustainably securing agriculture food production for future generations.
The degradation of agricultural soils in Mexico has been assessed and approached by focusing mainly on physicochemical features (e.g., Roldán et al., 2003; Govaerts et al., 2006; Fuentes et al., 2009). Undoubtedly important, relying only on these characteristics leads to an incomplete view of soil function, degradation and sustainable productivity. Beyond the physical, geochemical and biological aspects, agricultural management is the result of the interrelated views and actions of producers and landowners (Pulido and Bocco, 2003, 2014; Martin-Clouaire, 2017; González-Morales et al., 2018). Hence, in order to fully characterize soil degradation processes and improve soil quality in the long term it is critical to integrate the perceptions, necessities and socio-cultural context of local producers into agroecosystem understanding (Pulido and Bocco, 2003; Altieri, 2004).
In Mexico, in the last decades, the work of the Ministry of Agriculture (currently known as SADER), as well as some international and national organizations involved in agricultural production, has been mainly focused on increasing crop production through the use of synthetic pesticides and chemical fertilizers, with little or no collaboration or dialogue with local producers. For example, regarding soil conservation, one of the programs fostered by the Mexican government has encouraged the adoption of Conservation Agriculture principles by small producers (MASAGRO, see CEMDA, 2016), without taking into account its pertinence from a biocultural perspective, compatibility with traditional production systems, or the local community's necessities (Winters and Davis, 2009; CEMDA, 2016). This has led to an inefficient use of resources and, in some cases, to the violation of human rights, such as the rights to cultural identity, adequate food and a healthy environment (CEMDA, 2016, 2017; DESCA, 2017). This highlights the crucial role of the active participation of local stakeholders and communities to inform soil-related decisions and design policies with a biocultural approach (i.e., supporting both the cultural and biophysical components of local communities social-ecological systems) (Tengö et al., 2014; Lyver et al., 2019).
Methods for increasing public participation in scientific research and decision making have been widely developed and include approaches as varied as citizen science, participatory action research and participatory modeling (Sandker et al., 2010; Shirk et al., 2012). Such participatory methods contribute to an open dialogue and interaction across several societal actors to achieve a shared view or decision and take responsibility for it, as well as to address problems (Bousset et al., 2005). A frequently used participatory approach for understanding social-ecological systems is the analysis of mental models through Cognitive Mapping (CM) (Gray et al., 2013). Mental models are personal internal representations of the external world. These representations are unique to each individual, dynamic, and context-dependent. Eliciting mental models usually relies on interviewing individuals to develop cognitive map network models (Jones et al., 2011; Halbrendt et al., 2014). Mental models are thus represented as Cognitive Maps (CMs) depicted as the relationships (edges) between concepts (nodes) that describe a system and may represent direct and indirect causality (Özesmi and Özesmi, 2004; Gray et al., 2013). The development of collective mental models is feasible because a certain degree of shared understanding among groups of people with similar experiences is expected (Jones et al., 2011). Collective perceptions within communities can reflect the shared understandings of a social-ecological system which include the physical and biological processes and their interactions; the social actors involved, their expectations and relations; as well as the rules and norms that drive social-ecological change (Halbrendt et al., 2014; Reyers et al., 2018). Community perception is dynamic and may change in time and across sectors, regions or groups (e.g., Bertram and Rehdanz, 2015). Specific cases on which participatory methods and mental models have been employed include water management (Mathevet et al., 2011), learning and education (Elsawah et al., 2013), understanding urban resilience and transformation (Olazabal and Pascual, 2016; Siqueiros-García et al., 2019; Zambrano et al., 2019) and risk management (Devisscher et al., 2016), among others.
Studies with a participatory approach on community perception regarding agricultural soil quality and degradation have been developed mostly in Africa (Barker, 2009; Mekuria et al., 2009; Dawoe et al., 2012; Kuria et al., 2019), Europe (Dury et al., 2010; Lamarque et al., 2013; Karali et al., 2014), and to a lesser extent in Latin America (Barrios and Trejo, 2003; Vignola et al., 2010; Lima et al., 2011). To our knowledge, there are a few studies in Mexico that consider the communities' perception regarding soil quality and degradation (see Tucker and Castellanos, 2010; Barrera-Bassols, 2016; González-Morales et al., 2018). However, none of them examined different perceptions across multiple social sectors, ecoregions and agroecosystems.
In the present study we conducted a study that sought to integrate and analyze different perceptions regarding quality and degradation of agricultural soils across several social sectors including producers of two annual crops (maize and beans) and two perennial crops (coffee and avocado) with high economic and cultural significance, civil society organization members and decision makers or technicians from the Federal Government. We analyzed the community perception using CMs generated by different focus groups, which are planned discussions facilitated by a moderator (Slocum, 2003). In spite of the differences among groups, and the diverse ecological and social contexts of the regions involved, we expected to find some common elements and concerns that could suggest shared perceptions and touchpoints among sectors. We also expected to find exclusive elements and concerns for specific groups, which can reflect potential areas of conflict and should be considered in future decision-making processes. This study contributes to a deeper understanding of the current situation of agricultural soils in Mexico and seeks to inform the decision-making process regarding agricultural management in the country.
Methods
Two types of agricultural systems were included in the study. On the one hand, we included localities with annual crop production that consisted of maize and beans agroecosystems located in the central states of Guanajuato and Puebla. On the other hand, we included localities with perennial crop production that consisted of coffee and avocado agroforestry systems2 located in the eastern state of Veracruz, Mexico. Within these systems, the specific study sites spanned several types of agricultural practices, from backyard orchards and small-scale farming to highly technified and input-dependent production (Figure 1). This selection allowed us to include a wide range of biophysical and social characteristics associated with production systems. For instance, some of the coffee producers that participated in one of the focus groups were following only organic agricultural practices, whereas some others were practicing intensive agriculture. The focus groups were carried out in two localities of Guanajuato (Guarapo and La Quemada), and one locality in Puebla (Zapotitlán Salinas). As for the coffee region, focus groups took place in Veracruz in the localities of San Pablo Coapan, Coatepec and Huatusco. In the latter, a focus group was also conducted with avocado producers. Additionally, two focus groups were carried out in Mexico City at the National Autonomous University of Mexico (UNAM): one with members of three Mexican Civil Society Organizations promoting sustainable agriculture, and another one with members of three federal entities in charge of soil and water conservation in agricultural contexts (see details below).
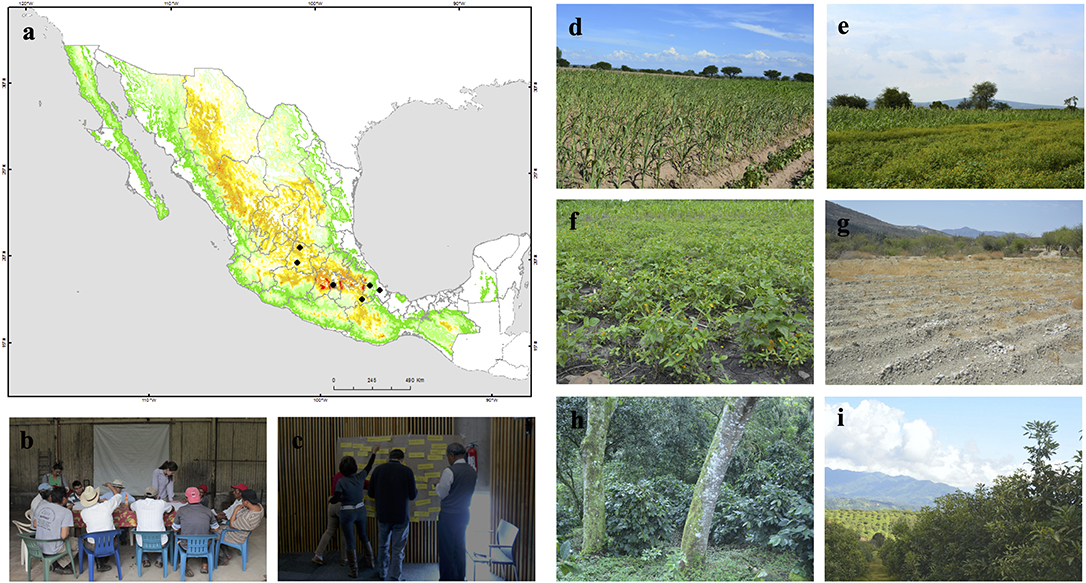
Figure 1. (a) Localities of interest in the states of Guanajuato, Puebla, Veracruz and Mexico City [altitude map by Comisión Nacional para el Conocimiento y Uso de la Biodiversidad (1998)]. Focus groups with coffee producers (two co-authors provided consent to be identifiable in the image) (b) and officials of the federal government (c). Sites where maize and beans are cultivated (d–g) and sites where coffee and avocado are produced (h,i).
Social Sectors Involved in the Study
The four social sectors participating in the project were: (1) Small-scale producers of the annual crops maize and beans (MBP), (2) producers of perennial crops coffee and avocado (CAP), (3) members of several Civil Society Organizations (CSO), and (4) decision makers or technicians from three different offices of the federal government (FG). Most of the participants were males (91%) and showed willingness to participate and be informed about the outcomes of the research.
Small-Scale Producers of Maize and Beans
It is important to note that among food producers there is a large variety of socioeconomic groups, and besides agriculture, other economic activities are developed in the study sites such as cattle raising, trading and tourism. Focus groups were conducted in three localities: Guarapo, La Quemada and Zapotiltán Salinas. The former two in the state of Guanajuato and the latter in the state of Puebla (summary in Table 1; see Supplementary Material 1 to consult characteristics of the localities). We extended an invitation to producers who had previously collaborated with some of the co-authors' projects or institutions. Specifically, maize and bean producers were contacted by one of the co-authors (A.W.).
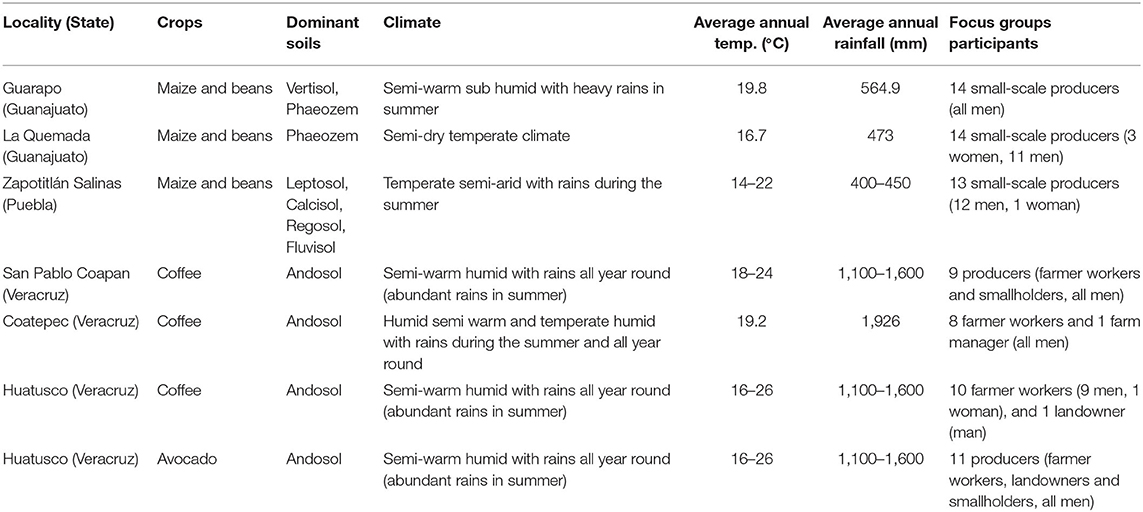
Table 1. Main characteristics of the six localities where focus groups with local food producers were conducted.
Producers of Coffee and Avocado
Four focus groups were conducted with coffee and avocado producers in the state of Veracruz, as summarized in Table 1. Producers were all from municipalities found at the Trans-Mexican Volcanic Belt. Avocado producers were contacted by one of the co-authors, who had previously collaborated with them (F.R.), most of whom are active members of the Veracruz Association of Avocado Growers, whereas coffee producers were contacted through the Cafecol A. C., which is an organization in Veracruz in charge of developing and transmitting traditional, scientific and technological knowledge among producers and where producers, processors, roasters and coffee shops participate.
Civil Society Organizations
We invited organizations working closely with food producers or interested in agroecosystems and soil sustainability topics. The three CSO that participated in the focus group were: (1) Enlace Comunicación y Capacitación (Enlace CC, A.C.) working on the promotion of social programs and productive projects in low income communities (one participant), (2) Grupo de Estudios Ambientales (GEA) working on sustainability projects (one participant), and (3) Centro Mexicano de Derecho Ambiental (CEMDA) working on the legal defense of natural resources and the right for a healthy environment (one participant).
Decision Makers or Technicians From the Federal Government
We extended an invitation to government offices related to policy generation and decision-making regarding soil conservation and agriculture in Mexico. Members of the following ministries agreed to participate in a focus group: (1) Ministry of Natural resources (SEMARNAT) in charge of the protection, conservation and use of the natural resources allowing sustainable development (one participant); (2) National Water Commission (CONAGUA) focused on the administration, regulation and protection of water resources but largely involved in land-use ordering and watershed planning (two participants); and (3) Commission for the Knowledge and Use of Biodiversity (CONABIO), an inter-secretarial commission in charge of promoting the conservation and sustainable use of biological diversity (one participant).
Elicitation of Perceptions and Cognitive Maps Building
Focus groups, a participatory approach involving group dynamics and consensus building, were employed to obtain information about the participants' mental models regarding soil quality (Figure 2). CM were used to represent mental models and group's perceptions (Gray et al., 2014). The design of the activities carried out in each focus group aimed to ensure that the perception of the focus group community was effectively captured and not only the perception of few individuals. The focus groups' activities engaged the participants in a dialogue to discuss agricultural soil quality and degradation at the community level. In addition, a method to systematically analyze the CM components and network structure and to compare multisector perceptions (CM of different social sectors) was introduced (see Figure 2).
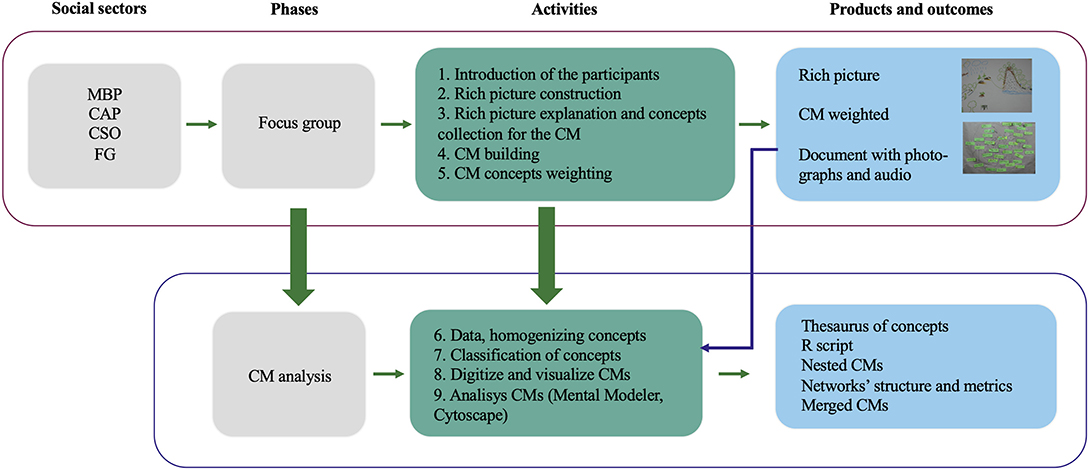
Figure 2. Summary of the workflow and methods involved in the work with focus groups, and in eliciting perceptions and analyzing the CMs.
Focus Groups: Activities, Organization and People (Participants and Facilitators)
In order to obtain a group's CM several activities were carried out with each focus group. The focus groups' dynamics were designed for 5 to 15 participants. The activities were coordinated by three to five facilitators who supervised timing, documented activities, and supported the attendees when required. The overall focus group involves 3 to 4 h of activities. Below we include a detailed description of each one of the activities:
1) Introduction of the participants.–Getting to know the participants and promoting dialogue. This activity promotes the dialogue and helps the attendees to know each other, as well as background and experience. Thus, participants introduce themselves and briefly describe their activities and interests.
2) Rich picture construction.–The activity aims to identify multiple viewpoints and is based on a specific question to be collectively addressed. The central question referred to how the participants perceived the issue of soil degradation in their community or field of expertise, but it was adapted depending on the site. This question is discussed, and participants draw a sketch to illustrate their thoughts and discussion. In the case of large attendance, the group is separated into subgroups of up to five persons. A first community dialogue among the participants around the triggering question is the beginning of the activity and it is followed by the collective sketching of such ideas. The construction of the rich picture should have a duration of 10 to 15 min. During this activity a facilitator is making notes and refocusing the discussion when necessary. The outcome is a rich picture per work group (Figures 3a,b).
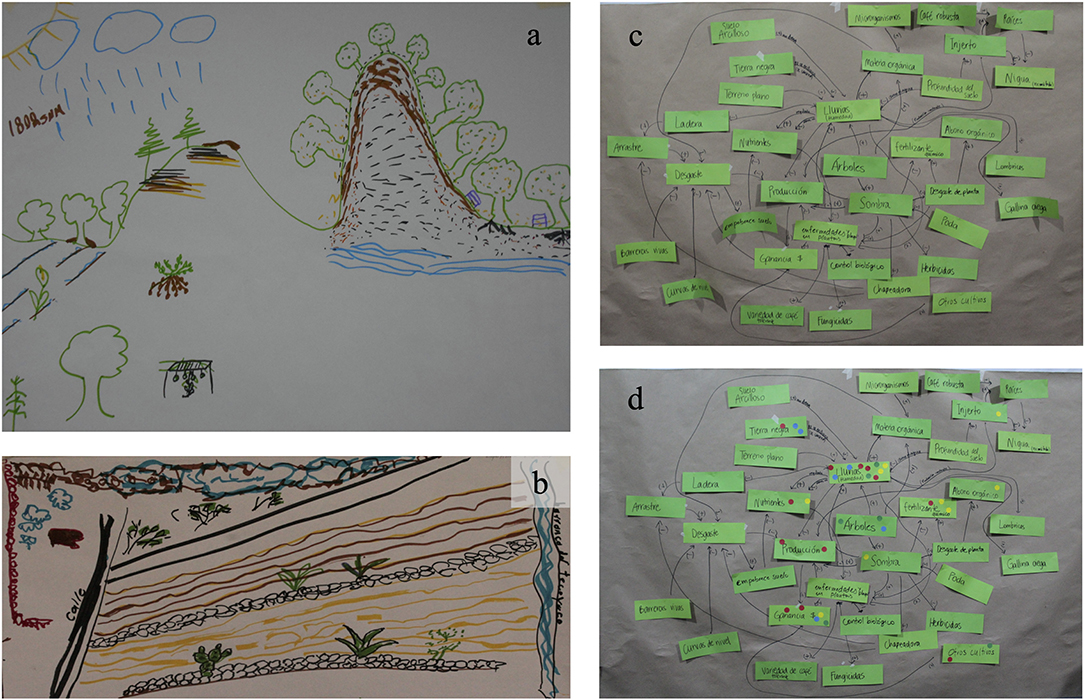
Figure 3. Activities conducted during the focus group. (a) Rich picture drawn at the beginning of a focus group and (b) producers of maize; (c) Group CM built during the focus group with coffee producers, and (d) same group CM depicting weighting scores for the most important concepts according to the participants during the focus group.
3) Rich picture explanation and collecting concepts for the CM.–A member of each group explains the sketch to all of the participants. While the explanation is carried out, two facilitators document the main ideas; one of them writes on paper cards the ideas, phrases or words the exponent mentions, while the other makes a list of words or phrases. This list is visible for everyone and will complement the cards. Through a narrative or qualitative description of the sketches facilitators obtain the words, phrases and concepts that in turn will be the elements of the CM.
4) CM building.–During this activity, a collective CM is constructed by connecting the card words (concepts of the CM). This allows to build a dialogue and consensus on the structure, dynamics and understanding of the central question raised at the beginning of the participatory focus group. To begin with, the participants are asked to choose -among all of the cards- a keyword to start. From this keyword, attendees start to connect components to each other through causal relationships, which takes place by defining links between the cards. A causal relationship refers to one concept that has a direct influence on another, that is, increasing or decreasing it. Thus, variables are connected positively or negatively. At this stage “new” words or variables not mentioned before can be added as long as the participants agree and consider them relevant for the system. A long-enough discussion reflecting the perception of each one of the attendees is crucial to construct a representative system. This activity should have a duration of 60 to 90 min and the outcome is an agreed upon group CM (Figure 3c).
5) CM concepts weighting.–A comprehensive community dialogue is required to accomplish a representative group mental model. Indeed, training of the focus group facilitators was largely focused in the promotion of equal participation of the focus group members, be it by time management or other strategies. This was easier in some cases, for instance, when the participants knew each other beforehand and had trustful relationships (e.g., some of the maize and bean producers were members of the same family). In other cases, equal participation was more difficult to achieve, either because participants did not know beforehand, or they knew beforehand but had asymmetric relationships (e.g., some participants worked in the same coffee plantations but had different employment status). Node weighting was thought as one strategy to collect the relative importance that all participants, including those who could have been less participative, attributed to particular concepts or nodes. Three stickers with a value of one point each are given to the attendees. Each participant has to silently select and to mark the most relevant word (or words) of the system, according to his/her perception. There are several options to weight individually. For instance, to put one sticker per card-word, so that a participant marks three different words with one point each, or the three stickers can be put on the same word, that is, the three points go for one word. The outcome of this activity is a weighted CM (Figure 3d).
Cognitive Maps Analysis
Data, Homogenizing Concepts
For comparing different CMs to identify both similarities and differences, a standardized set of words or concepts was required. CMs of different social sectors, for instance food producers and decision makers, contain different words for the same concept, so that it was necessary to homogenize such words or concepts for the CM to be comparable. To do this a Thesaurus of concepts was generated by the co-authors of this work in collective working sessions. The thesaurus consisted of the original words used by each focus group (e.g., producers, decision makers, etc) and the homogenized/standardized concepts. Homogenization refers to the process of finding a common terminology to unify the understanding of concepts (Olazabal et al., 2018) of the different CMs. The process to build a thesaurus is explained as follows:
i. Databases of the original and homogenized words. A database was built with the original concepts of each one of the CMs to be compared as well as standardized words or concepts. To illustrate, different CMs contained the variable “chemical fertilizer,” or “inorganic fertilizer system” or “synthetic fertilizers”; the suggested standardized concept would be “inorganic fertilizer.”
ii. Depurating concepts. Narrative extracts (audio) from the focus groups are used to confirm and clarify unclear concepts or phrases as well as the type of connection/relation (positive or negative) and doubts on the meaning of words. At this stage we also consolidated a few identical concepts and verified that the terminology was consistent (Jetter, 2006; Alizadeh and Jetter, 2017; Brouwer et al., 2017).
iii. R script. In order to manage the concept database systematically and reproducibly, we generated a set of R scripts that enabled us to organize, consult, compare, visualize or extract information from this database (the script is available upon request).
Classification of Concepts
Based on the thesaurus, a classification of the concepts corresponding to environmental, socio-cultural, government, financial and technological aspects and practices (focused on water, soil, crop and agricultural system) was carried out. Overall, the categories include the three main axes of sustainability (environment, society, and economy), although the society and economy axes were specified as: financial, technological, policy, and socio-cultural aspects. In addition, since most of the cognitive maps included a large number of concrete practices that we deemed convenient not to collapse into a single category, we organized practices as related to water, soil, crop and whole agroecosystems. This allowed us to analyze the commonalities and differences among the categories represented in the CMs, not only in terms of broad sustainability-related categories, but also in terms of concrete practices that varied in scale, scope and approach. Concepts in the thesaurus were arranged in these categories by five of the authors, who reviewed each of the terms in a series of group working sessions where they collectively coded them. This classification was later used to analyze in depth and characterize the concepts, as well as to provide additional insight into the overall structure of the CMs and how these categories relate to each other.
Digitizing and Visualizing Cognitive Maps
The CMs generated during the focus groups were visualized and analyzed using the specialized network Mental Modeler (Gray et al., 2013) and the open source software platform Cytoscape v3.7.1 (Shannon et al., 2003). Since the CMs can be understood as networks of concepts, we analyzed and compared them on the basis of their network features. The networks' structure was characterized by calculating the total number of concepts (nodes), type of node (driver, receiver, ordinary), total number of connections, connections per node determined as the number of connections divided by the number of nodes, density calculated as number of connections divided by the maximum number of possible connections (Hage and Harary, 1983; Özesmi and Özesmi, 2004), and complexity calculated as the ratio of receiver to driver nodes (Özesmi and Özesmi, 2004; Gray et al., 2014). Three measures of centrality were employed. Degree centrality, hereafter called connectivity, is used for finding highly connected nodes, those that are directly linked to many of the nodes (concepts in CMs) (Sharma and Surolia, 2013). This centrality measure is the simplest measure of node connectivity. On the other hand, betweenness centrality shows which nodes act as “bridges” between nodes in a network, is used for finding the nodes that indirectly link many nodes of the network and largely influence the flow of information in CMs (Freeman, 1979; Raghavan Unnithan et al., 2014). Several studies on the evaluation of complex systems, sustainability science and social-ecological systems have employed these two measures (see Lü et al., 2016; Horcea-Milcu et al., 2020). The third measure was the weighting of the concepts determined by participants during workshops. The latter, as mentioned before (see section Small-Scale Producers of Maize and Beans of the methods) is a measure that reflects the view of the participants and gives voice to those that find it difficult to express verbally. Although connectivity, betweenness centrality and node weighting by the participants are all centrality measures, they are estimated and interpreted in slightly different ways, which is why they were used as complementary measures. Each one of the networks associated with a CM was arranged hierarchically (Hierarchical Layout algorithm in Cytoscape) to help visualize the flow of information from the base to the top nodes.
Merging and Comparing Cognitive Maps
For analytical purposes, the three CMs created by the maize-beans producers (Guarapo + La Quemada + Zapotitlán) were merged into a representative collective CM (MBP). Similarly, the four CMs developed by the coffee-avocado producers (Naolinco + Coatepec + HuatuscoC + HuatuscoA) were combined into a single collective CM (CAP). CMs were merged by using the Advanced Network Merge interface function available in Cytoscape. Within this function, we chose the mathematical union of the networks, based on the column that contained the names of the nodes. As described before, we considered these two types of producers as belonging to two distinct sectors because they exhibit important differences in the type of agriculture they practice (plot scale, cash crop vs. self-consumption agriculture, etc.). Since we only performed one focus group for CSO and one for FG, there was no need to merge CMs in these two cases. It is important to bear in mind the limitations associated with the fact that the CMs of some social sectors (e.g., maize-beans producers) were created from multiple sub-CMs generated in multiple separated focus groups. In those cases, the participants across focus groups had no social interactions. Therefore, the final CMs of all social sectors are not representing the same scale of social-influence and group dynamics.
From the four networks representing MBP, CAP, CSO and FG CMs, an exploration to identify the most important nodes was conducted through the CytoHubba application (Chin et al., 2014) implemented in Cytoscape with a centrality criterion. The top 10 (and top 11 for CSO) ranked nodes were obtained. Then, for each of the four social sectors a network with the top ten ranked connectivity and betweenness nodes was obtained (Figures 5–8) and visualized with the corresponding weighting of nodes. Finally, by using the thesaurus of the concepts, visualizing them with Venn diagrams and applying a merging networks tool (intersection and difference) in Cytoscape, a comparison of the CMs was conducted, identifying unique and shared CM concepts.
Cognitive Map Socialization
We developed a poster with a stylized version of the CM for each site and sector, visited each one of the localities where the focus group took place to present the results (final CMs) and discussed them with the participants. These posters contained the original terms used by the participants of the focus groups, not those used in the thesaurus. Posters were designed in order to be used on a table or a wall in an individual or collective manner (examples of these posters can now be found as Supplementary Material 2). Printed or digital versions were handed to the participants of the focus groups and, in some cases, also to local authorities or local institutions. However, in these follow-up visits we could not gather the focus groups again and collected mostly individual and incomplete impressions of the CMs. Therefore, the final CMs were not strictly validated at the end of the project by the focus groups.
Results
Cognitive Maps, Their Representation and Interpretation
For each focus group a CM was built. These were visualized as networks connecting nodes (concepts) through either positive (gray) or negative (red) edges (Figure 4). Each node represented a concept that could be an abstract idea such as “distrust in government programs,” a tangible object like “organic matter” (Vasslides and Jensen, 2016), or any ideas that individuals or a group considered part of the system (Levy et al., 2018). The size of the nodes represents the connectivity, calculated as the total number of connections with other nodes. The color of the nodes (from warm to cold colors) corresponds to the betweenness centrality or the number of minimal paths passing through each node. Large, warm-colored nodes are postulated as key concepts in the CM (see complete CMs of the four sectors in Supplementary Material 3). Black dots indicate the node weighting made by the focus group participants.
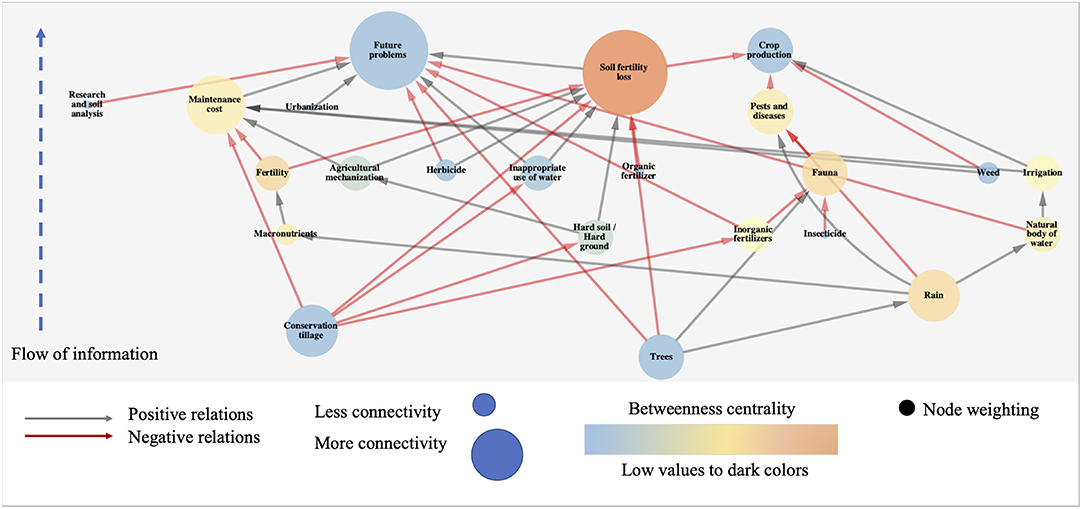
Figure 4. Example of a hierarchized Cognitive Map (CM). The blue arrow to the left shows the proposed flow of information. Positive and negative relations are in gray and red, respectively. The size of the nodes represents the connectivity and the colors of the nodes (from warm to cold colors) corresponds to the betweenness centrality. Black dots indicate the node weighting.
The blue arrow to the left shows the proposed flow of information. At the bottom of the network, the driver nodes are localized (nodes that influence the system but are not affected by others in the spatio-temporal scale that the group considered in the discussion). Toward the middle section are located the ordinary nodes (nodes that are affected by others but also influence other nodes). At the top of the network are the receiver nodes (nodes that are only affected by others) (Figure 4). We calculated connectivity and betweenness centrality of each node, as these metrics are often used to indicate the relative importance of a node in structure and integration of the whole system (Newman, 2010). An example of a CM generated from a single focus group is presented in Figure 4.
Cognitive Maps per Sector
The merged network for the MBP (Supplementary Material 3) showed that among the driver nodes were Trees, Government support and Soil depth. The nodes with the highest connectivity were Rain, Crop production, and Own economic resources, whereas that the two former nodes along with Organic fertilizers were the nodes with the highest betweenness. The greatest percentage of node weighting was for Rain (28.8%), followed by Water and soil retention techniques and Organic fertilizers (19.2% each) and these concepts corresponded to the categories of Environmental aspects, Practices focused on agroecosystem and soil (Figure 5).
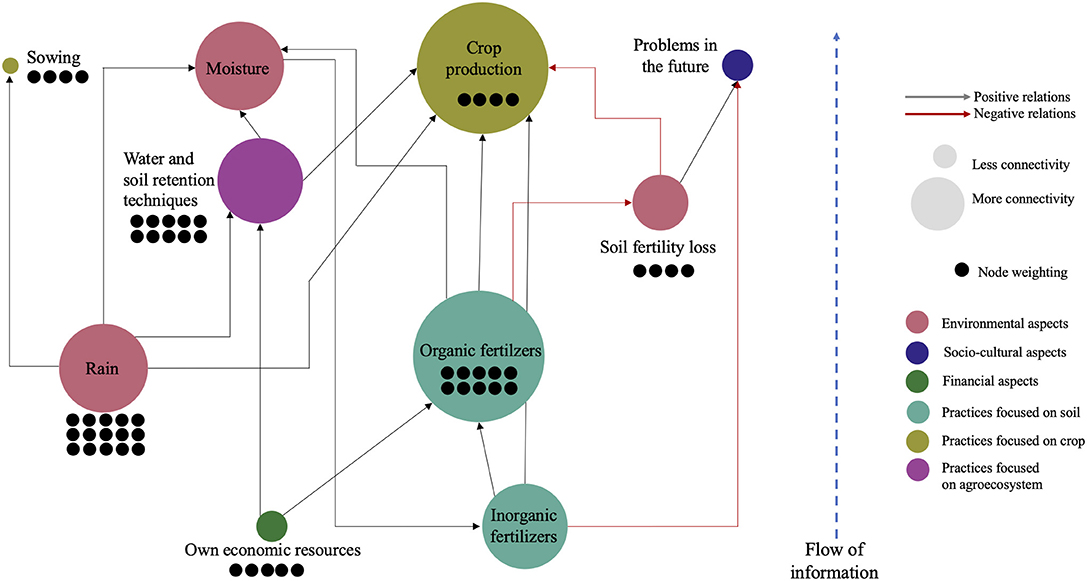
Figure 5. Top ten nodes for the MBP CM based on centrality measures. The size of the nodes corresponds to connectivity (the bigger the node, the more connections it has). Black dots represent the weighting scores.
As for the merged network of the CAP (Supplementary Material 3), among the driver nodes were Extensive production model, Traditional plantation, Sustainable plantation, and Gravel. The nodes with the highest centrality (connectivity and betweenness) were Permeable soil, Tree shade and Moisture which corresponded to Environmental aspects category (Figure 6) and were also among the concepts with the highest weighting percentages (Moisture 30.3% and Permeable soil with 24.2%).
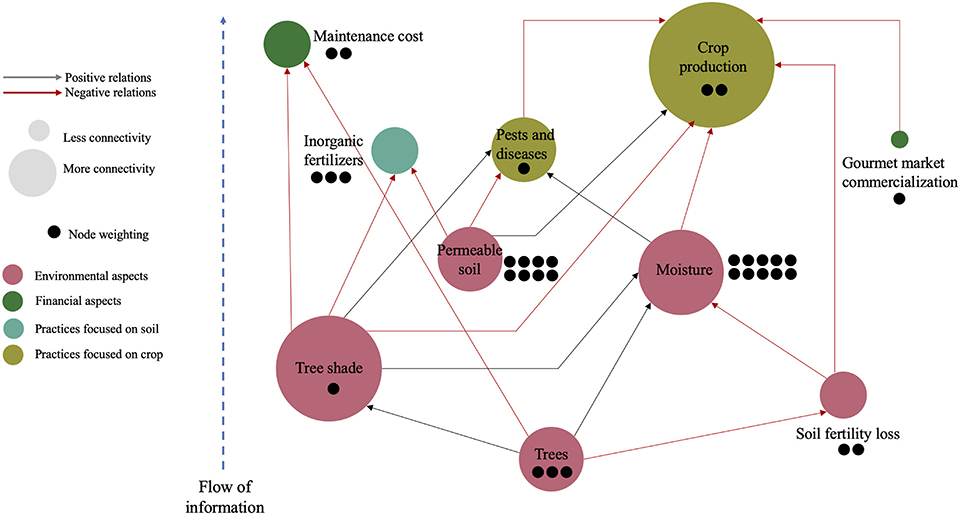
Figure 6. Top ten nodes for the CAP CM based on centrality measures. Size of the nodes correspond to connectivity. Black dots represent the weighting scores.
For the CSO CM (Supplementary Material 3) the driver nodes included Public policies, Extensive agriculture, Extensive production model, Climate change, and Green fertilizer among others. The nodes with the highest connectivity were Cultural identification, organization, and collective knowledge, Extensive agriculture and Public policies which fell into the Socio-cultural aspects, Public policy and programs and Practices focused on agroecosystem categories (Figure 7). As for the betweenness, again, the nodes Cultural identification, organization, and collective knowledge, Extensive agriculture and Crop production had the highest values. The former concept along with Public policies showed the highest weighting percentage with 33.3% each.
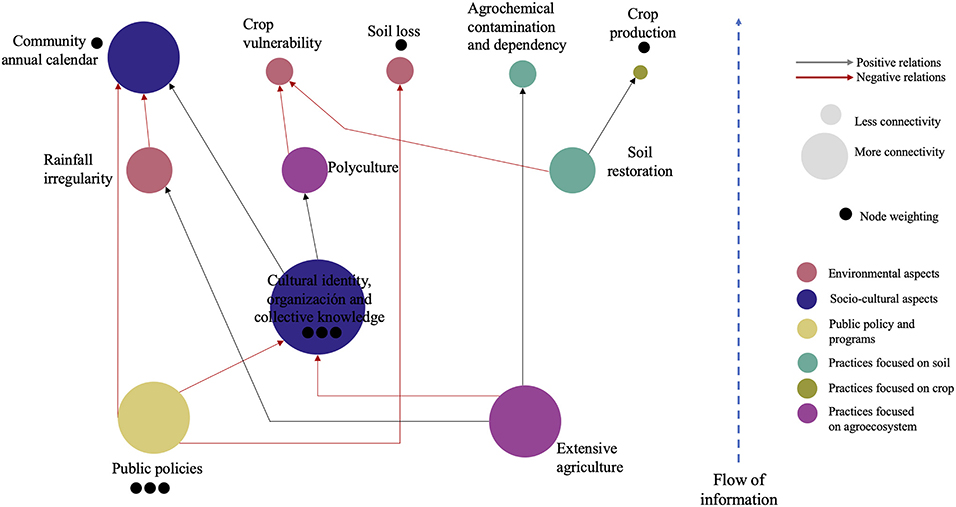
Figure 7. Top eleven nodes for the CSO CM based on centrality measures. Size of the nodes correspond to connectivity. Black dots represent the weighting scores.
The FG network (Supplementary Material 3) had among the driver nodes Abuse of agrochemicals, Lack of continuity of programs, Deforestation, Irrigation systems technification, Land tenure, and Taxes. The driver nodes fell into almost all of the proposed categories of concepts. The node Land use change had the highest connectivity followed by Public policies and Soil compaction. Again the nodes Land use change and Soil compaction along with Livestock had the highest betweenness. Land use change was also the one with the highest weighting percentage (60%) given by participants (Figure 8).
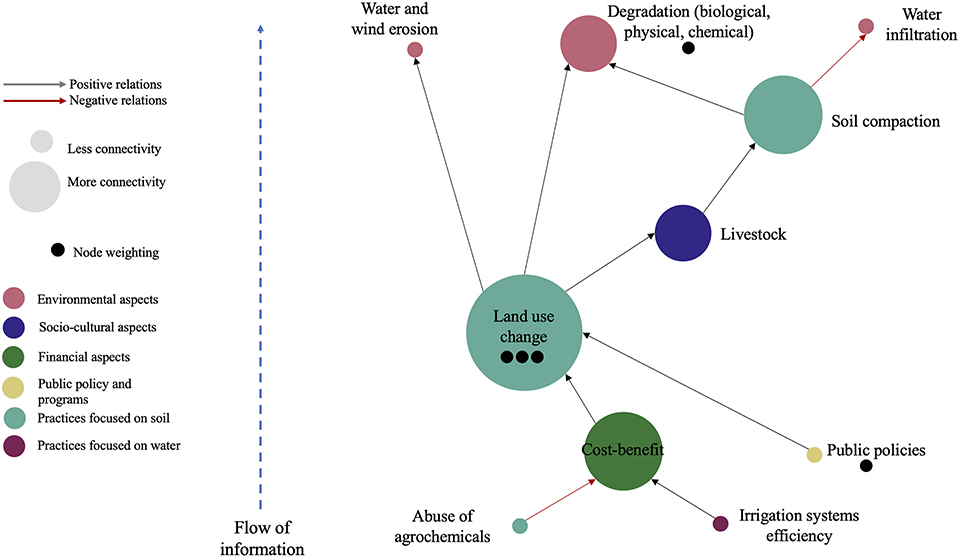
Figure 8. Top ten nodes for the FG CM based on centrality measures. Size of the nodes correspond to connectivity. Black dots represent the weighting scores.
In general, the concepts corresponding to the Environmental and Socio-cultural aspects as well as Practices focused on soil and crop categories prevailed in the CMs (see Figure 9). The highest proportion of categories across the four social sector networks based on connectivity (Figure 9A) was Environmental aspects (having MBP the highest proportion followed by CAP, CSO and finally FG with the lowest proportion). As for betweenness centrality (Figure 9C) the highest proportion was for the Environmental aspects (for CAP), Socio-cultural aspects (for MBP and CSO) and Practices focused on soil (for FG). In addition, the Cognitive color spectrum charts (see Cholewicki et al., 2019; Hodges et al., 2019) were developed to show the sequence of dominance based on the connectivity and betweenness (Figures 9B,D, respectively). The category of Environmental aspects was dominant across the four networks based on connectivity (Figure 9B), and the less dominant categories were Technological aspects (for MBP and FG) and Practices focused on soil (for CAP and CSO). In terms of betweenness (Figure 9D), the dominating categories were Socio-cultural aspects (for MBP and CSO), Environmental aspects (for CAP) and Practices focused on soil (for FG), whereas the less dominating categories were Technological aspects (for MBP), Practices focused on water (CAP and CSO) and Practices focused on agroecosystem (for FG).
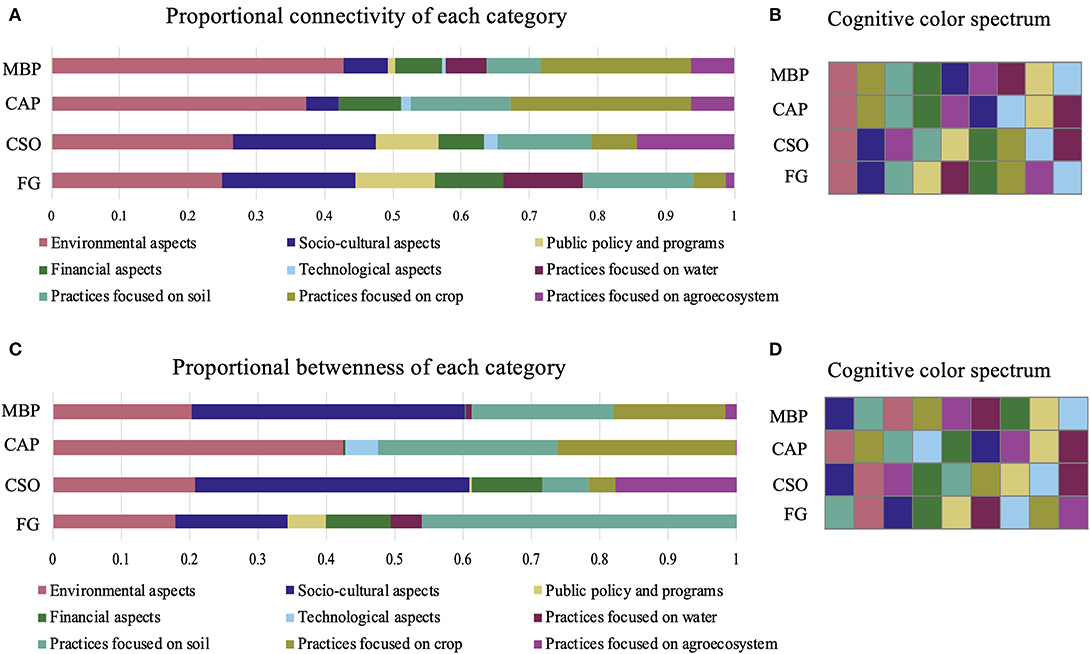
Figure 9. Proportional centrality for each category for the sum of connectivity (A) and the sum of betweenness (C) per network as well as the Cognitive color spectrum charts of the four networks based on connectivity (B) and betweenness (D).
Cognitive Map Intersectoral Comparisons, Commonalities and Specificities
For each one of the complete CMs the metrics summarizing the network structure were obtained (Table 2). The networks with the highest and lowest number of nodes (81 and 51) and the total number of connections (188 and 74) were CAP and FG, respectively. As for connections per node, MBP and FG presented the highest (2.33) and the lowest (1.45) values, respectively. Regarding complexity, the CSO and MBP networks presented the highest (1.64) and the lowest (0.82) values, respectively. It is important to note that higher values of complexity indicate complex systems thinking (Özesmi and Özesmi, 2004; Gray et al., 2014). Similar values of density were observed across the four networks (see Methods).
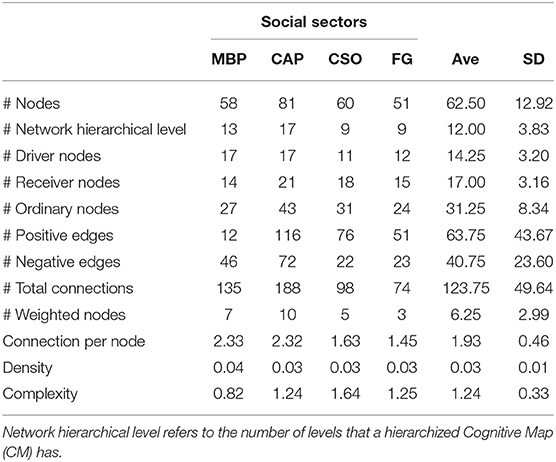
Table 2. Summary of metrics and structure statistics for each one of the four sector's networks. Average (Ave) and standard deviation (SD) for the metrics.
The comparison of the concepts of the four social sector's CM (Figures 10, 11) revealed that the following five concepts were shared among all the participants: Deforestation, Water and wind erosion, Inorganic fertilizers, Agricultural mechanization, and Cultural identity, organization and collective knowledge (Figure 10). These concepts fell into the categories of Environmental aspects, Socio-cultural aspects, Practices focused on soils and Practices focused on crops. It is also worth noting that each one of the sectors had exclusive concepts, that is, concepts not shared with any other social sector (Figure 11). For instance, CSO is the only sector that mentioned a Technological aspects category, specifically the Transgenic concept or within the category of Environmental aspects the concept of Climate change. Likewise, concepts such as Corruption, Distrust of government programs and Landowner decisions were mentioned only by the FG sector (See all of the exclusive words in Supplementary Material 4).
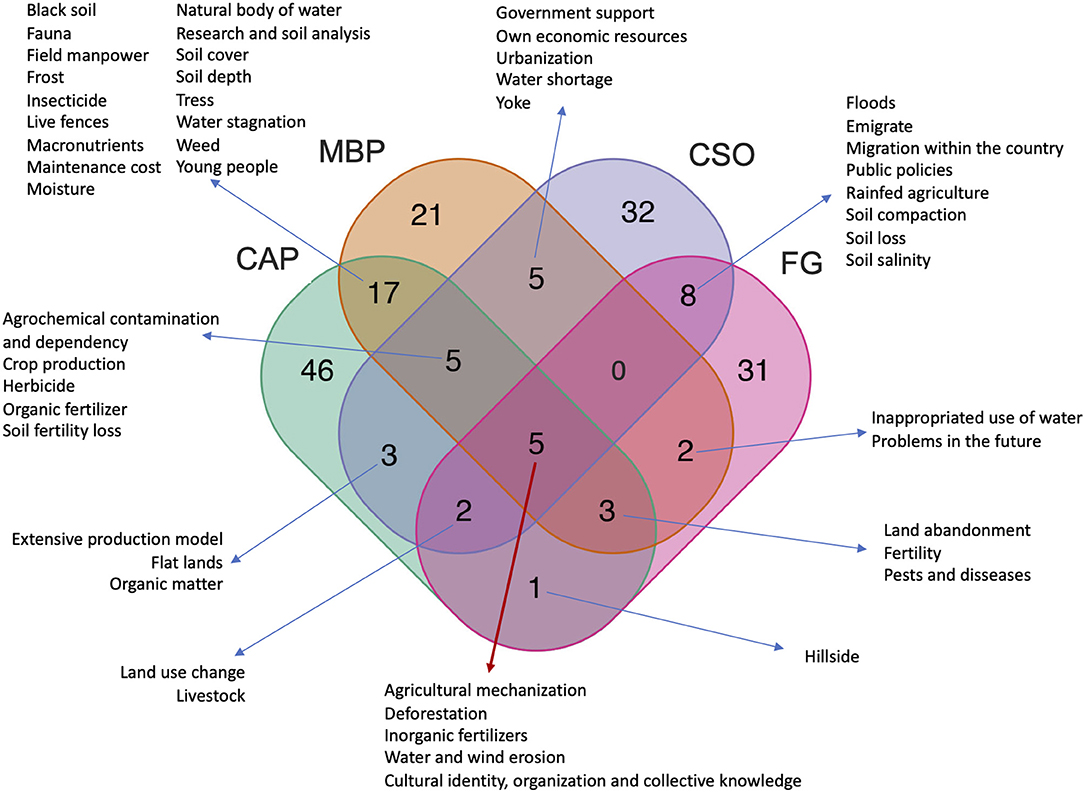
Figure 10. Venn diagram showing the shared concepts between sectors and the five concepts shared among all of them (red arrow).
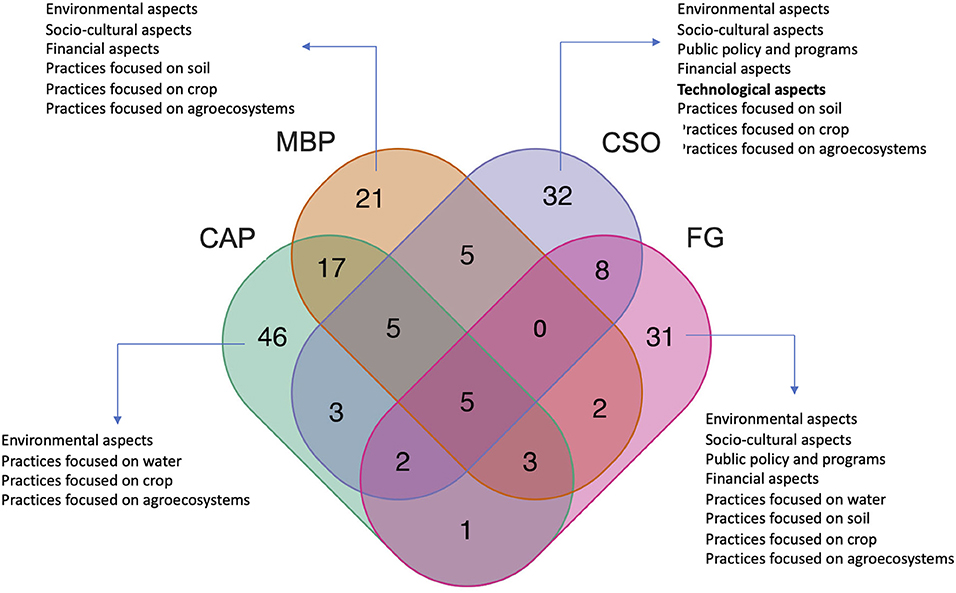
Figure 11. Venn diagram showing the number of exclusive concepts and the categories in which these fell for each one of the sectors. In bold a category mentioned only by one of the four sectors (see details in Supplementary Material 4).
As for the weighting of nodes, the scores (given by participants during the focus groups) generally matched the top ten betweenness nodes in almost all of the networks (Figure 12). This suggests that the top ten selection upon network properties recovers the set of nodes that were considered the most important by participants and that, therefore, network properties might be good proxies for the individual perception of importance. Among the concepts with the highest scores were Rain, Water and soil retention techniques and Organic fertilizer and these were present in the MBP sector. The weighted and shared nodes between several social sectors were Crop production (contained in three out of the four practitioner groups, MBP, CAP, and CSO), Soil fertility loss (shared between the sectors MBP and CAP), and Public policies (shared between CSO and FG sectors) (see Figure 12).
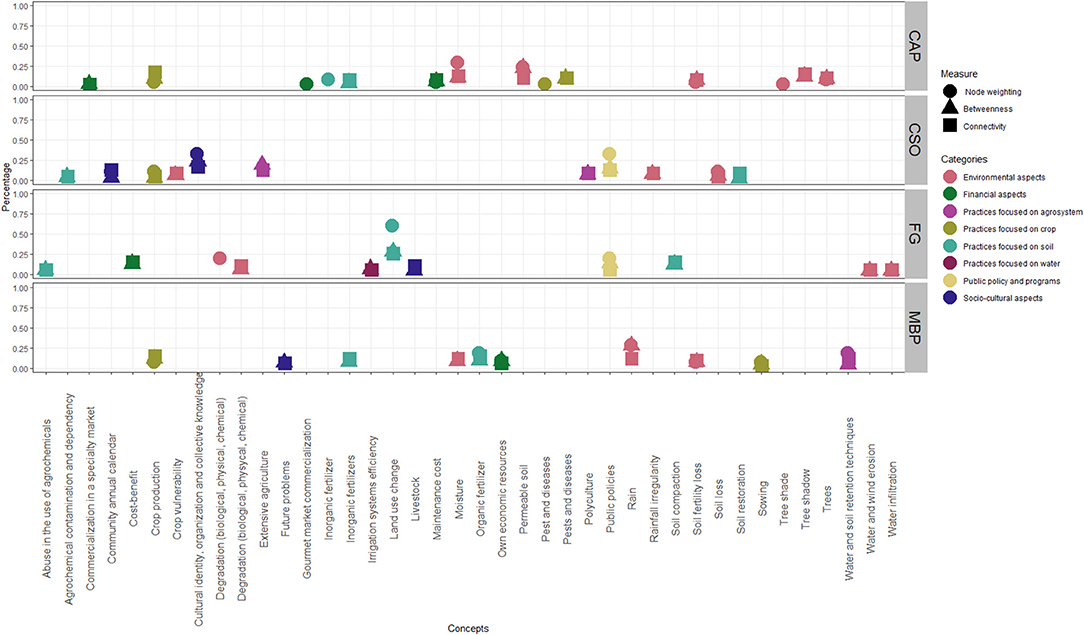
Figure 12. Distribution of nodes centrality (connectivity and betweenness) and nodes weighting for each of the CMs.
Finally, the CMs generated and digitally visualized after the focus groups were shared with the actors who participated in the focus groups, as well as with other people in the localities interested in the research project. A second round of meetings was carried out at the same localities in the states of Guanajuato, Puebla and Veracruz to present and hand over material containing the main results of the analyses and the interpretation of the CMs (Section Cognitive map socialization and Supplementary Material 2). Such dissemination material was distributed and discussed with local producers during visits, whereas that for the members of the CSO and FG reports summarizing the outcomes were delivered by mail. Overall, the participants agreed with the final representation of the CMs and the posters were sometimes used by the participants to imagine scenarios in which one or some of the nodes were removed. In one of the sites (Puebla), the map was used to collectively explore scenarios in which one or more nodes were modified. This exercise allowed some of the local producers to discuss the specifics and value of traditional techniques for water accumulation in steep plots, especially under scenarios of extended dry seasons.
Discussion
Perceptions, necessities and the socio-cultural context of several social actors are required to attain a comprehensive characterization of soil degradation processes, as well as to develop integral and efficient soil conservation strategies and to increase soil quality. In this study, we integrated and analyzed the perceptions of several social sectors involved in the management of agricultural soils in Mexico. Participatory methods have been successfully used in other soil studies conducted in Mexico with the aim of exploring the perception of various social sectors on soil processes and integrating different knowledge systems (e.g., Grossman, 2003; Reséndiz-Paz et al., 2013; Barrera-Bassols, 2016). However, most of these studies assess the understanding of soil processes through interviewing food producers from a single community with the main objective of comparing local and scientific soil knowledge. Here, we used a series of activities with focus groups for different social sectors, which were aimed to elicit the collective perceptions regarding soil quality and degradation and to render CMs for each sector.
Through the comparison of the CMs, we aimed to identify concepts that could help establish dialogues among sectors, as well as areas of potential conflict that require attention in future decision-making processes. Some of these concepts are further detailed in the following paragraphs. Due to the commitment and active participation of the four social sectors in the focus groups (see Tucker and Castellanos, 2010; González-Morales et al., 2018), interesting outcomes were obtained nourishing further our analysis and discussion. Like other studies, we found that by applying a model collection technique with concepts chosen freely (Gray et al., 2014; Henly-Shepard et al., 2015), the participants were able to debate and articulate their perception of the system with no apparent constraints (Jetter and Kok, 2014; Siqueiros-García et al., 2019). A hierarchical arrangement of CMs and the network analyses contributed to: (1) visualize the order of influence from the components over the whole system and to identify driver, ordinary and receiver nodes, (2) to hint on the potential long- or short-term effects of actions taken to improve soil quality, and (3) to identify key components, concerns and conflicts across social sectors that could be potential action areas and focus for upcoming decision making processes (Figures 5–8).
It is worth noting that the network analyses allowed to identify nodes or concepts with high connectivity and betweenness values within CMs and that such nodes coincided with those weighted individually by the focus group participants, which supports the use of network analyses to uncover key concepts in CMs (Figures 5–8, 12). For example, for MBP the concept Organic fertilizer was highly weighted (19.2%) and presented a relatively high value of betweenness. The concepts Crop production, Soil fertility loss and Public policies appeared among the top ten connectivity and betweenness nodes in several of the social sector networks and these were highly weighted as well. Thus, concept score weighting represents a reliable method to identify the most central components of a system. It also reflects that during the group work and discussion the participants are aware or become aware of the crucial role of the interactions in the overall system. Moreover, the commonalities found among sectoral CMs suggest that these representations are coherently capturing the perceptions around soil degradation and encourage us to further our interpretations and use of the obtained CMs.
As expected, all of the CMs shared some concepts belonging to the categories of Environmental aspects and Practices focused on soil (see Figures 5–9), although there was no consistent pattern regarding their location (bottom, middle or at the top) in the CMs. For the CMs of the four sectors (Figures 5–8) the highest values of centrality (connectivity and betweenness) were observed for concepts belonging to the Environmental and Socio-cultural aspects and Practices categories, particularly those focused on crop and soil (which were also the most dominant categories, see Figure 9), highlighting a shared understanding regarding the relevance of soil management on soil quality. Indeed, some soil quality indicators like organic matter content and aggregation, change with management over the years (Karlen et al., 2008; Weil and Brady, 2017). Moreover, these concepts were consistently connected to concepts belonging to the Environmental aspects category, which include soil characteristics that are unaffected by management. Three out of the four CMs contained the word Crop production (Practices focused on crops) including MBP, CAP, and CSO, and in all of these cases, this concept was a highly connected receiver node suggesting that for food producers, crop productivity is an important index for soil quality. These results reveal the farmers' practical approach to soil quality assessment, as found by Barrera-Bassols (2016) in an indigenous community of Central Mexico. In accordance with other studies conducted in Latin America, other indicators of soil quality among food producers were related to the presence of certain soil biota (like earthworms) and soil color (Barrios and Trejo, 2003; Barrios et al., 2006).
It is known that soil quality is related to soil functionality, such as productivity, biodiversity, filtering and buffering contaminants, nutrient cycling, water and solute flow, and physical stability (Karlen et al., 2008). However, food producers, members of CSOs and the government also relate soil quality to various socio-cultural, financial and technological aspects that are usually overlooked when dealing with soil degradation and developing soil conservation strategies. More importantly, some of these concepts are driver nodes. When modified, they can have large consequences on the whole agroecosystem. For instance, in the CM of FG Public policies was a strong driver node, reflecting their historical and current influence in many agricultural systems in Mexico. Likewise, the concept of Cultural identity, organization and collective knowledge was present in all CMs and influenced other concepts related to soil and agroecosystem management. Driver nodes by definition have considerable influence upon the other components and the connections going toward the top. While this definition of driver nodes may be artificially lineal in systems that often exhibit feedback, it is important to consider the long-lasting effect of changes on these nodes. For instance, based on the complete version of the CMs, specifically for the CM of CSO (Figure 7) if action is taken to improve Public policies (driver, at the bottom of the CM) the outcomes will be reflected in the medium and long term on aspects such as Crop vulnerability and Soil loss (receiver nodes, at the top of the CM). On the other hand, the components on the highest levels of the network tend to refer to problems and immediate necessities, that is, those urgent to satisfy and possibly attracting most of the resources. Among these are, for example, pest control, crop productivity, water and wind erosion, degradation and water infiltration. While reflecting important matters and often corresponding to central and highly connected nodes, decisions made to solve urgent issues may contribute relatively little to the entire system sustainability in the long term. In this sense, public policies aimed at improving agricultural soils quality and agroecosystems sustainability should focus on strengthening cultural identity, organization, and collective knowledge, as well as increasing farmers economic resources and maximizing their cost-benefit ratio (Figures 5, 7, 8). In contrast, public policies are too often focused on increasing crop yields (e.g., CEMDA, 2016), disregarding the drivers that could have a positive longer lasting effect on agroecosystems sustainability. Mexico is a country in which many people live and have the right to self-determination, and public policies have been largely criticized for being imposed on these people (CEMDA, 2017). In this sense, we suggest that the Public policy nodes could also be interpreted as the collective decision over the territories made by local or communitarian institutions whose actions may have integrative and long-term effects.
The concepts Deforestation, Water and wind erosion, Inorganic fertilizers, Agricultural mechanization, and Cultural identity, organization and collective knowledge were shared among the four social sectors (Figure 10) and were always mentioned as problems or sources of conflict. For instance, one of the most mentioned sources of problems during the focus groups was the excessive use of inorganic fertilizers, which is clearly reflected in the CMs of MBP and CAP (Figures 5, 6). Interestingly, despite all social sectors consider the use of inorganic fertilizers problematic, their use in Mexico and in most Latin American countries has been steadily increasing over time (Betancourt, 2020). Even though these five concepts were not the ones with the highest values of centrality (connectivity and betweenness) (see Figures 5–8), their common emergence in CMs highlights their importance in the design of government programs and public policies. Specifically, the shared mentions to Cultural identity, organization and collective knowledge draws attention to the historical disregard of these aspects in the design of government policies and calls for truly participatory processes for the design and continuous assessment of public policies in agricultural matter (see Winters and Davis, 2009; CEMDA, 2016). In Mexico, poorly designed policies or programs to support agricultural productivity strongly affects agricultural management choices (e.g., Zarazúa-Escobar et al., 2011; Appendini, 2014; CEMDA, 2016). For instance, some programs actively promote the use of chemical fertilizers to increase crop production (Winters and Davis, 2009). Furthermore, the lack of systemic actions supporting the adoption and application of locally-produced organic fertilizers is notorious (CEMDA, 2016). This supports the idea that traditional practices including the application of organic fertilizers as well as their preparation through local techniques are not being taken into account, promoted nor financially supported by government policies. In this regard, only in the CM of MBP appeared the concept Organic fertilizers, that besides being one with the highest connectivity and weighting score was connected to Crop production and Own economic resources. As mentioned during the focus groups, local producers must invest their own economic resources, that along with many other complex circumstances, force them to take government financial support with whatever conditions attached, adopting for instance the use of chemical inputs and foremost depending thoroughly on them and giving up traditional practices including crop rotation and green manure (Rasmussen and Collins, 1991; Appendini, 2014).
Some exclusive concepts per sector were directly or indirectly related to time scales, the effects of soil conservation and degradation or were related to site-specific characteristics. For instance, Conservation tillage was an exclusive concept for the MBP, while Conservation of soil permeability was only mentioned by CAP. Both are site-specific concepts. Conservation tillage is a soil conservation strategy that has been promoted in areas that produce annual crops and would hardly be relevant in avocado and coffee production. On the other hand, conservation of soil permeability was an important concept in localities with high average annual rainfall and does not represent a problem in other sites (Table 1). The concept Abuse on the use of agrochemicals was a private concept for FG (Supplementary Material 4), underscoring the notion of conflict around the use of inorganic fertilizers, herbicides, and pesticides. These concepts are reflecting actions that can be executed day by day or somehow imply short-term modifications. On the other hand, the exclusive concepts of CSO included Soil restoration that represents a more abstract but long-termed concern for securing agriculture food production for future generations.
By going deeper in the analysis of unique concepts, we found that CSO social sector was the only one that mentioned the concepts: Transgenic crops, Impacts on human health and Climate change, revealing concerns that involve long-term processes and problems that are not so directly related to soil quality or degradation but are important for agroecosystems sustainability. We could also observe a difference in the spatial scales considered by the different sectors. For instance, the FG CM and rich pictures refer to a basin or regional scale (Figure 8; Supplementary Material 4), while the MBP CM refer to a plot or local scale (Figure 5; Supplementary Material 4). This coincides with other soil studies conducted in the Global South where local practitioners have greater knowledge of local soil processes but lack a broader understanding of soil processes at larger spatial scales. Conversely, technical or scientific knowledge systems fail to grasp site-specific details but exhibit a general understanding of soil processes at regional and global scales (Barrios et al., 2006; Barrera-Bassols, 2016). These complementary sources of information should be considered in the design and implementation of public policies and programs that are pertinent for each context. It is worth noting that shared concepts, categories and scales considered altogether point to OSC as ideal bridges in the dialogue among sectors, which is why they should probably be continuously involved in the design of programs and policy regarding soil quality and degradation.
It is noteworthy that the sites where maize and beans are cultivated exhibit a high incidence of land abandonment. Indeed, most of the young population from Guarapo migrate to the United States looking for job opportunities. Although seemingly disconnected, migration turned out to be an important concept for soil quality among maize and beans producers. Since many public policies and programs supporting agricultural productivity (through technological improvements and marketing) promote capitalization with equipment, that is, purchase of machinery and agricultural implements (Zarazúa-Escobar et al., 2011; Orozco-Hernández et al., 2017), migration abroad is also encouraged or stimulated as it represents an opportunity to obtain enough capital and to adopt technologies for crop management, that, in turn, are expected to ensure crop production, among other aspects (Zarazúa-Escobar et al., 2011; Figures 5, 6; Supplementary Material 4). In contrast, coffee and avocado producers did not mention migration, land abandonment and other socio-cultural concepts. However, their CM revealed a great influence of the coffee and avocado regional, national and international markets. Differences among producers like the ones emerging from their CMs have not been adequately considered in the development of public programs and policies and may thus lead to the partial or total failure of such policies (Camacho-Villa et al., 2016; CEMDA, 2016).
As for the limitations of our study, the uneven number of participants in the focus groups might lead to biases in the outcomes. However, in the case of the CSO (three participants) and FG (four participants), the small number of participants was partially overcome by a thorough selection of participants, as they were working directly with local food producers or were members of the ministry in charge of the design of public policy and programs focused on agricultural soils and water resources in Mexico. Moreover, the participatory elicitation process aimed to capture all relevant concepts for a group of practitioners no matter the number of participants (Singer et al., 2017; Siqueiros-García et al., 2019). On the other hand, the edaphic, environmental and socio-cultural conditions involved in soil management in Mexico are extremely heterogeneous and are surely not fully represented by the set of sites and participants in the study. Indeed, while we considered diverse crops of cultural and economic importance (annual and perennial), localities and ecoregions, some other important crops and types of production were not considered, and further studies could focus on them. Nevertheless, this study contributes to a deeper understanding of the current situation of agricultural soils in Mexico as it spans several agroecosystems, regions and social sectors.
Among the main contributions of our study for better understanding the current situation of agricultural soils in Mexico are, first, the need of identifying the drivers that improve or diminish soil quality beyond the effects of biophysical processes. These drivers include, but are not limited to, public policies, cultural identity, organization and collective knowledge, and economic resources. In other words, the current situation of agricultural soils in Mexico is largely driven by soil management practices that are conducted by food producers but are promoted by public policies and constrained by economics. Both traditional and scientific knowledge about strategies that could increase soil quality exist in different sectors, but many are labor intensive and food producers are rarely encouraged to put them into practice. Secondly, our results show that the use of agrochemicals is regarded as a highly problematic practice. Although in many cases their use increases crop yields, all sectors agreed on the fact that it also decreases soil quality. Mexican agricultural soils are undoubtedly affected by the use of synthetic fertilizers and other agrochemicals across different agroecosystems and regions. Moreover, one of the conflicting points found when comparing CMs was precisely related to the use of agrochemicals. While for FG the abuse of agrochemicals is a driver concept that generates other problems (like a poor cost-benefit ratio), for CSO the use of agrochemicals is a receiver node that results from the tendency of intensifying agriculture and generates dependency and pollution. Finally, Mexican agroecosystems are highly heterogeneous and improving soil quality will largely depend on site-specific strategies. In fact, another difference among CMs was the scale in which different sectors assess soil quality. As noted, food producers focused on local scales whereas FG and CSO focused on landscape or regional scales. This is not necessarily a conflicting point but rather shows the complementarity of different knowledge systems. Integrating different knowledge systems is crucial for the sustainable management of agroecosystems and could have positive synergistic outcomes for soil quality (Barrios et al., 2006; Reséndiz-Paz et al., 2013; Barrera-Bassols, 2016).
The methods presented here can contribute to designing sustainable food systems because they allow to identify current problems in agroecosystems, as well as their drivers and consequences. CMs are flexible enough to be unique for each focus group but their structure and metrics (connectivity and betweenness) make comparisons across focus groups possible. Thus, these methods are a powerful tool for exploring conflicts and agreements among several social sectors. Moreover, identifying drivers and receivers provide the opportunity of suggesting changes to the system for avoiding some paths and strengthening others. Although we focused our study on agroecosystems soil quality, these methods are applicable for assessing any social-ecological system (Gray et al., 2015).
Research on the perceptions from different sectors involved in soil management, degradation and conservation can provide insights in the actions required to successfully conserve agricultural soils in the long term. In this respect, this study: (i) allows to identify local cultural and practical aspects, some of them revealed as key elements of the system, that have been left out from major programs and that could be incorporated in the design of sustainable productive strategies, (ii) highlights the importance of tackling specific issues that appear to be a general concern, such as the control of synthetic fertilizers, (iii) suggests factors that are recognized in each community as important and that can be the focus of long-term actions (driver nodes) or of monitoring strategies (receptor nodes), and (iv) highlights the current role of CSOs as knowledge breakers and key actors in the establishment of potentially meaningful dialogue among sectors. Overall, the incorporation of these diverse perceptions may help overcome the systemic pattern of infringement of human rights documented for rural Mexico (CEMDA, 2016; DESCA, 2017). Along with scientific knowledge, multisectoral perceptions harbor invaluable information regarding the shared concerns, particularities and potential sources of conflict that can inform the design, monitoring and evaluation of public policies and programs.
Data Availability Statement
The datasets generated for this study are available on request to the corresponding author.
Ethics Statement
The studies involving human participants were reviewed and approved by Ethics Committee of the Ecology Institute, UNAM. Written informed consent for participation was not required for this study in accordance with the national legislation and the institutional requirements.
Author Contributions
MB, AW, AE, JR, and FR participated in the design of the project and coordinated the study. DA-L, AU, MB, AW, VP, and VV-B participated in the design of the focus group and the analyses of cognitive maps. DA-L, MB, AU, and AW wrote the manuscript. VP-R and FR included relevant information within their areas of expertise and reviewed the manuscript. All authors contributed to the article and approved the submitted version.
Funding
This work was financially supported by the Consejo Nacional de Ciencia y Tecnología (CONACYT-PN247672) and Programa de Apoyo a Proyectos de Investigación e Innovación Tecnológica (PAPIIT-IV200117, PAPIIT-IN207819, and PAPIIT-IN214719).
Conflict of Interest
The authors declare that the research was conducted in the absence of any commercial or financial relationships that could be construed as a potential conflict of interest.
The reviewer JS-G declared a shared affiliation, though no other collaboration, with several of the authors, DA-L, AU, VP, VV-B, JR, AE, VP-R, MB, and AW, to the handling Editor.
Acknowledgments
We would like to thank each one of the attendees of the participatory focus groups for their time and interest in sharing their experiences and knowledge including producers from Guarapo, La Quemada, Zapotitlán Salinas, San Pablo Coapan, Coatepec and Huatusco, as well as the members of the CSOs: Enlace Comunicación y Capacitación (Enlace CC, A.C.), GEA (Grupo de Estudios Ambientales, A.C.), CEMDA (Centro Mexicano de Derecho Ambiental A.C.), and representatives of the offices: SEMARNAT, CONAGUA, CONABIO. We are grateful to all the collaborators including students, technicians and researchers of the Consejo Nacional de Ciencia y Tecnología project CONACYT-PN247672. We appreciate the technical advice and ideas made by Lakshmi E. Charli Joseph and Bertha Hernández to improve the focus group activities. The authors acknowledge the technical assistance of Morena Avitia, Rodrigo González Chauvet, Adny Celis, Valeria List as well as the students Diana Ysimoto, Jennifer Mejía, Karla Peña, Fabiola Estrada. We would like to thank Salvador Montes, Gerardo Hernández and Karina Boege for the valuable support in linking with the producers. We also thank CONACYT for financial support (PN247672) and for the scholarship to VV-B (477713) to conduct Graduate Studies in Biological Sciences, UNAM. AU is also supported by CONACYT (scholarship No. 709969).
Supplementary Material
The Supplementary Material for this article can be found online at: https://www.frontiersin.org/articles/10.3389/fsufs.2020.522661/full#supplementary-material
Footnotes
1. ^Agroecosystems are ecological, productive and social units of food production composed by living and nonliving elements, as well as their interactions and the processes they enable (Rosset and Altieri, 2018).
2. ^Agroforestry systems are productive units in which woody and herbaceous perennials are combined, temporally or spatially, with animals and crops (Rosset and Altieri, 2018).
References
Alizadeh, Y., and Jetter, A. (2017). “Content analysis using fuzzy cognitive map (FCM): a guide to capturing causal relationships from secondary sources of data,” in 2017 Portland International Conference on Management of Engineering and Technology (PICMET) (Portland, OR: IEEE), 1–11. doi: 10.23919/PICMET.2017.8125305
Altieri, M. A. (2004). Linking ecologists and traditional farmers in the search for sustainable agriculture. Front. Ecol. Environ. 2, 35–42. doi: 10.1890/1540-9295(2004)002[0035:LEATFI]2.0.CO;2
Altieri, M. A., Funes-Monzote, F. R., and Petersen, P. (2012). Agroecologically efficient agricultural systems for smallholder farmers: contributions to food sovereignty. Agr. Sustain. Dev. 32, 1–13. doi: 10.1007/s13593-011-0065-6
Appendini, K. A. de (2014). De la Milpa a Los Tortibonos: la Restructuración de la Política Alimentaria en México. Alicante: Biblioteca Virtual Miguel de Cervantes.
Barker, D. (2009). Appropriate methodology: an example using a traditional african board game to measure farmers' attitudes and environmental images1. IDS Bull. 10, 37–40. doi: 10.1111/j.1759-5436.1979.mp10002006.x
Barlett, P. F. (1980). Agricultural Decision Making: Anthropological Contributions to Rural Development. New York, NY: Academic Press. 395.
Barrera-Bassols, N. (2016). “Linking ethnopedology and geopedology: a synergistic approach to soil mapping. Case study in an indigenous community of Central Mexico,” in Geopedology: An Integration of Geomorphology and Pedology for Soil and Landscape Studies, eds. J. A. Zinck, G. Metternicht, G. Bocco, and H. F. Del Valle (Cham: Springer International Publishing), 167–181. doi: 10.1007/978-3-319-19159-1_9
Barrios, E., Delve, R. J., Bekunda, M., Mowo, J., Agunda, J., Ramisch, J., et al. (2006). Indicators of soil quality: a South–South development of a methodological guide for linking local and technical knowledge. Geoderma 135, 248–259. doi: 10.1016/j.geoderma.2005.12.007
Barrios, E., and Trejo, M. T. (2003). Implications of local soil knowledge for integrated soil management in Latin America. Geoderma 111, 217–231. doi: 10.1016/S0016-7061(02)00265-3
Bertram, C., and Rehdanz, K. (2015). Preferences for cultural urban ecosystem services: comparing attitudes, perception, and use. Ecosystem Services 12, 187–199. doi: 10.1016/j.ecoser.2014.12.011
Betancourt, M. (2020). The effect of Cuban agroecology in mitigating the metabolic rift: A quantitative approach to Latin American food production. Glob. Environ. Change 63:102075. doi: 10.1016/j.gloenvcha.2020.102075
Bousset, J.-P., Macombe, C., and Taverne, M. (2005). Participatory methods, guidelines and good practice guidance to be applied throughout the project to enhance problem definition, co-learning, synthesis and dissemination. Report No.10 SEAMLESS - Cemagref
Brouwer, H., Brouwers, J., Sonkin, F., Pisters, S., Premchander, S., de Hauwere, K., et al. (2017). The MSP Tool Guide: Sixty Tools to Facilitate Multi-Stakeholder Partnerships. Companion to The MSP Guide. Wageningen: Wageningen University and Research, CDI. doi: 10.3362/9781780446691.000
Buckles, D. (1999). Cultivating Peace: Conflict and Collaboration in Natural Resource Management. Ottawa: International Development Research.
Camacho-Villa, T. C., Almekinders, C., Hellin, J., Eulalia Martinez-Cruz, T., Rendon-Medel, R., Guevara-Hernández, F., et al. (2016). The evolution of the masagro hubs: responsiveness and serendipity as drivers of agricultural innovation in a dynamic and heterogeneous context. J. Agricult. Educ. Exten. 22, 455–470. doi: 10.1080/1389224X.2016.1227091
CEMDA (2016). Informe sobre la pertinencia biocultural de la legislación mexicana y su política pública para el campo. El caso del programa de “Modernización Sustentable de la Agricultura Tradicional” (MasAgro). Centro Mexicano de Derecho Ambiental, A.C., México, Ciudad de México.
CEMDA (2017). Human Rights and Biocultural Heritage The Milpa System as the Foundation for a Culturally and Environmentally Sustainable Public Policy. Mexico: Centro Mexicano de Derecho Ambiental, A.C.
Chin, C.-H., Chen, S.-H., Wu, H.-H., Ho, C.-W., Ko, M.-T., and Lin, C.-Y. (2014). cytoHubba: identifying hub objects and sub-networks from complex interactome. BMC Syst. Biol. 8 (Suppl. 4):S11. doi: 10.1186/1752-0509-8-S4-S11
Cholewicki, J., Popovich, J. M., Aminpour, P., Gray, S. A., Lee, A. S., and Hodges, P. W. (2019). Development of a collaborative model of low back pain: report from the 2017 NASS consensus meeting. Spine J. 19, 1029–1040. doi: 10.1016/j.spinee.2018.11.014
Comisión Nacional para el Conocimiento y Uso de la Biodiversidad (1998). “Curvas de nivel para la República Mexicana,” in Extraído del Modelo Digital del Terreno (México: Instituto Nacional de Estadística, Geografía e Informática (INEG)).
CONAFOR-UACH (2013). Línea Base Nacional de Degradación de Tierras y Desertificación. Informe final. México: CONAFOR-UACH.
Dawoe, E. K., Quashie-Sam, J., Isaac, M. E., and Oppong, S. K. (2012). Exploring farmers' local knowledge and perceptions of soil fertility and management in the Ashanti Region of Ghana. Geoderma 179–180, 96–103. doi: 10.1016/j.geoderma.2012.02.015
DESCA (2017). Informe Conjunto de Sociedad Civil Sobre los Derechos Económicos, Sociales, Culturales y Ambientales en México 2017. Informe alternativo a los Informes V y VI Periódicos combinados del Estado mexicano ante el Comité DESC de la ONU. Sandoval Terán, A., Eri
Devisscher, T., Boyd, E., and Malhi, Y. (2016). Anticipating future risk in social-ecological systems using fuzzy cognitive mapping: the case of wildfire in the Chiquitania, Bolivia. Ecol. Soc. 21:art18. doi: 10.5751/ES-08599-210418
Dury, J., Garcia, F., Reynaud, A., and Therond, O. (2010). “Modelling the complexity of the cropping plan,” in International Congress on Environmental Modelling and Software (Ottawa, ON: International Environmental Modelling and Software Society - iEMSs), 257.
Elsawah, S., Mclucas, A., and Mazanov, J. (2013). Using a cognitive mapping approach to frame the perceptions of water users about managing water resources: a case study in the australian capital territory. Water Resour. Manag. 27, 3441–3456. doi: 10.1007/s11269-013-0357-5
Freeman, L. C. (1979). Centrality in social networks conceptual clarification. Soc. Netw. 1, 215–239. doi: 10.1016/0378-8733(78)90021-7
Fuentes, M., Govaerts, B., De León, F., Hidalgo, C., Dendooven, L., Sayre, K. D., et al. (2009). Fourteen years of applying zero and conventional tillage, crop rotation and residue management systems and its effect on physical and chemical soil quality. Eur. J. Agronomy 30, 228–237. doi: 10.1016/j.eja.2008.10.005
Gavin, M. C., McCarter, J., Mead, A., Berkes, F., Stepp, J. R., Peterson, D., et al. (2015). Defining biocultural approaches to conservation. Trends Ecol. Evol. 30, 140–145. doi: 10.1016/j.tree.2014.12.005
González-Morales, S. B., Mayer, A., and Ramírez-Marcial, N. (2018). Assessment of soil erosion vulnerability in the heavily populated and ecologically fragile communities in Motozintla de Mendoza, Chiapas, Mexico. Solid Earth 9, 745–757. doi: 10.5194/se-9-745-2018
Govaerts, B., Sayre, K. D., and Deckers, J. (2006). A minimum data set for soil quality assessment of wheat and maize cropping in the highlands of Mexico. Soil Tillage Res. 87, 163–174. doi: 10.1016/j.still.2005.03.005
Gray, S., Gray, S., Cox, L. J., and Henly-Shepard, S. (2013). “Mental modeler: a fuzzy-logic cognitive mapping modeling tool for adaptive environmental management,” in 2013 46th Hawaii International Conference on System Sciences (Wailea, HI: IEEE), 965–973. doi: 10.1109/HICSS.2013.399
Gray, S., Zanre, E., and Gray, S. R. J. (2014). “Fuzzy cognitive maps as representations of mental models and group beliefs,” in Fuzzy Cognitive Maps for Applied Sciences and Engineering, ed. E. I. Papageorgiou (Berlin: Springer), 29–48. doi: 10.1007/978-3-642-39739-4_2
Gray, S. A., Gray, S., de Kok, J. L., Helfgott, A. E. R., O'Dwyer, B., Jordan, R., et al. (2015). Using fuzzy cognitive mapping as a participatory approach to analyze change, preferred states, and perceived resilience of social-ecological systems. Ecol. Soc. 20:art11. doi: 10.5751/ES-07396-200211
Grossman, J. M. (2003). Exploring farmer knowledge of soil processes in organic coffee systems of Chiapas, Mexico. Geoderma 111, 267–287. doi: 10.1016/S0016-7061(02)00268-9
Hage, P., and Harary, F. (1983). Structural Models in Anthropology. Cambrigde: Cambridge University Press. doi: 10.1017/CBO9780511659843
Halbrendt, J., Gray, S. A., Crow, S., Radovich, T., Kimura, A. H., and Tamang, B. B. (2014). Differences in farmer and expert beliefs and the perceived impacts of conservation agriculture. Glob. Environ. Change 28, 50–62. doi: 10.1016/j.gloenvcha.2014.05.001
Henly-Shepard, S., Gray, S. A., and Cox, L. J. (2015). The use of participatory modeling to promote social learning and facilitate community disaster planning. Environ. Sci. Policy 45, 109–122. doi: 10.1016/j.envsci.2014.10.004
Hodges, P. W., Cholewicki, J., Popovich, J. M., Lee, A. S., Aminpour, P., Gray, S. A., et al. (2019). Building a collaborative model of sacro-iliac joint dysfunction and pelvic girdle pain to understand the diverse perspectives of experts. PM R 11(Suppl 1):S11–S23. doi: 10.1002/pmrj.12199
Horcea-Milcu, I., Martín-López, B., Lam, D., and Lang, D. (2020). Research pathways to foster transformation: linking sustainability science and social-ecological systems research. Ecol. Soc. 25:13. doi: 10.5751/ES-11332-250113
Ilbery, B. W. (1978). Agricultural decision-making. Progr. Geogr. 2, 448–466. doi: 10.1177/030913257800200303
Jetter, A. J. (2006). “Fuzzy cognitive maps for engineering and technology management: what works in practice?” in Portland International Conference on Management of Engineering and Technology (Istanbul: Engineering and Technology Management Faculty Publications), 498–512. doi: 10.1109/PICMET.2006.296648
Jetter, A. J., and Kok, K. (2014). Fuzzy cognitive maps for futures studies-a methodological assessment of concepts and methods. Futures 61, 45–57. doi: 10.1016/j.futures.2014.05.002
Jie, C., Jing-zhang, C., Man-zhi, T., and Zi-tong, G. (2002). Soil degradation: a global problem endangering sustainable development. J. Geogr. Sci. 12, 243–252. doi: 10.1007/BF02837480
Jones, N. A., Ross, H., Lynam, T., Perez, P., and Leitch, A. (2011). Mental models: an interdisciplinary synthesis of theory and methods. Ecol. Soc. 16:46. doi: 10.5751/ES-03802-160146
Karali, E., Brunner, B., Doherty, R., Hersperger, A., and Rounsevell, M. (2014). Identifying the factors that influence farmer participation in environmental management practices in Switzerland. Hum. Ecol. 42, 951–963. doi: 10.1007/s10745-014-9701-5
Karlen, D. L., Susan, S., Andrews, B. J., Wienhold, and Ted, M., Zobeck. (2008). Soil Quality Assessment: Past, Present and Future. Publications from USDA-ARS / UNL Faculty. 1203
Kuria, A. W., Barrios, E., Pagella, T., Muthuri, C. W., Mukuralinda, A., and Sinclair, F. L. (2019). Farmers' knowledge of soil quality indicators along a land degradation gradient in Rwanda. Geoderma Region. 16:e00199. doi: 10.1016/j.geodrs.2018.e00199
Lamarque, P., Artaux, A., Barnaud, C., Dobremez, L., Nettier, B., and Lavorel, S. (2013). Taking into account farmers' decision making to map fine-scale land management adaptation to climate and socio-economic scenarios. Landsc. Urban Plan. 119, 147–157. doi: 10.1016/j.landurbplan.2013.07.012
Lambin, E. F., Turner, B. L., Geist, H. J., Agbola, S. B., Angelsen, A., Bruce, J. W., et al. (2001). The causes of land-use and land-cover change: moving beyond the myths. Glob. Environ. Change 11, 261–269. doi: 10.1016/S0959-3780(01)00007-3
Levy, M. A., Lubell, M. N., and McRoberts, N. (2018). The structure of mental models of sustainable agriculture. Nat. Sustain. 1, 413–420. doi: 10.1038/s41893-018-0116-y
Lima, A. C. R., Hoogmoed, W. B., Brussaard, L., and Sacco dos Anjos, F. (2011). Farmers' assessment of soil quality in rice production systems. NJAS Wageningen J Life Sci. 58, 31–38. doi: 10.1016/j.njas.2010.08.002
Lü, L., Chen, D., Ren, X.-L., Zhang, Q.-M., Zhang, Y.-C., and Zhou, T. (2016). Vital nodes identification in complex networks. Phys. Rep. 650, 1–63. doi: 10.1016/j.physrep.2016.06.007
Lyver, P. O., Timoti, P., Davis, T., and Tylianakis, J. M. (2019). Biocultural hysteresis inhibits adaptation to environmental change. Trends Ecol. Evol. 34, 771–780. doi: 10.1016/j.tree.2019.04.002
Martin-Clouaire, R. (2017). Modelling operational decision-making in agriculture. Agricult. Sci. 8, 527–544. doi: 10.4236/as.2017.87040
Mathevet, R., Etienne, M., Lynam, T., and Calvet, C. (2011). Water management in the camargue biosphere reserve: insights from comparative mental models analysis. Ecol. Soc. 16:43. doi: 10.5751/ES-04007-160143
Mekuria, W., Veldkamp, E., Haile, M., Gebrehiwot, K., Muys, B., and Nyssen, J. (2009). Effectiveness of exclosures to control soil erosion and local communities perception on soil erosion. Afr. J. Agricult. Res. 4, 365–377. Retrieved from: http://www.academicjournals.org/AJAR (accessed November 17, 2020).
Olazabal, M., Chiabai, A., Foudi, S., and Neumann, M. B. (2018). Emergence of new knowledge for climate change adaptation. Environ. Sci. Policy 83, 46–53. doi: 10.1016/j.envsci.2018.01.017
Olazabal, M., and Pascual, U. (2016). Use of fuzzy cognitive maps to study urban resilience and transformation. Environ. Innov. Soc. Trans. 18, 18–40. doi: 10.1016/j.eist.2015.06.006
Orozco-Hernández, M. E., García-Fajardo, B., Álvarez-Arteaga, G., and Mireles-Lezama, P. (2017). Tendencias del sector agrícola, estado de méxico. Quivera 19, 99–121. Retrieved from: http://hdl.handle.net/20.500.11799/68523 (accessed November 17, 2020).
Özesmi, U., and Özesmi, S. L. (2004). Ecological models based on people's knowledge: a multi-step fuzzy cognitive mapping approach. Ecol. Modell. 176, 43–64. doi: 10.1016/j.ecolmodel.2003.10.027
Pannell, D. J., and Schilizzi, S. (1999). Sustainable agriculture: a matter of ecology, equity, economic efficiency or expedience? J. Sustain. Agricult. 13, 57–66. doi: 10.1300/J064v13n04_06
Prell, C., Hubacek, K., and Reed, M. (2009). Stakeholder analysis and social network analysis in natural resource management. Soc. Nat. Resourc. 22, 501–518. doi: 10.1080/08941920802199202
Pulido, J., and Bocco, G. (2003). The traditional farming system of a Mexican indigenous community: the case of Nuevo San Juan Parangaricutiro, Michoacán, Mexico. Geoderma 111, 249–265. doi: 10.1016/S0016-7061(02)00267-7
Pulido, J., and Bocco, G. (2014). Local perception of land degradation in developing countries: a simplified analytical framework of driving forces, processes, indicators and coping strategies. Living Rev. Landsc. Res. 8:4. doi: 10.12942/lrlr-2014-4
Raghavan Unnithan, S. K., Kannan, B., and Jathavedan, M. (2014). Betweenness centrality in some classes of graphs. Int. J. Combin. 2014, 1–12. doi: 10.1155/2014/241723
Rasmussen, P. E., and Collins, H. P. (1991). Long-term impacts of tillage, fertilizer, and crop residue on soil organic matter in temperate semiarid regions. Adv. Agronomy 45, 93–134. doi: 10.1016/S0065-2113(08)60039-5
Reséndiz-Paz, M., de la, L., Gutiérrez-Castorena, M. de, C., Gutiérrez-Castorena, E. V., Ortiz-Solorio, C. A., Cajuste-Bontempts, L., et al. (2013). Local soil knowledge and management of anthrosols: a case study in Teoloyucan, Mexico. Geoderma 193–194, 41–51. doi: 10.1016/j.geoderma.2012.09.004
Reyers, B., Folke, C., Moore, M.-L., Biggs, R., and Galaz, V. (2018). Social-ecological systems insights for navigating the dynamics of the anthropocene. Ann. Rev. Environ. Resour. 43, 267–289. doi: 10.1146/annurev-environ-110615-085349
Roldán, A., Caravaca, F., Hernández, M. T., García, C., Sánchez-Brito, C., Velásquez, M., et al. (2003). No-tillage, crop residue additions, and legume cover cropping effects on soil quality characteristics under maize in Patzcuaro watershed (Mexico). Soil Tillage Res. 72, 65–73. doi: 10.1016/S0167-1987(03)00051-5
Rosset, P., and Altieri, M. (2018). Agroecología. Ciencia y politica. Sociedad Científica Latinoamericana de Agroecología SOCLA.
Sandker, M., Campbell, B. M., Ruiz-Pérez, M., Sayer, J. A., Cowling, R., Kassa, H., et al. (2010). The role of participatory modeling in landscape approaches to reconcile conservation and development. Ecol. Soc. 15:13. doi: 10.5751/ES-03400-150213
Schmidhuber, J., and Tubiello, F. N. (2007). Global food security under climate change. Proc. Natl. Acad. Sci. U.S.A. 104, 19703–8. doi: 10.1073/pnas.0701976104
SEMARNAT-COLPOS (2002). Evaluación de la Degradación del Suelo Causada Por el Hombre en la República Mexicana, Escala 1:250,000: Memoria Nacional. México: SEMARNAT-COLPOS.
Shannon, P., Andrew, M., Owen, O., Nitin, S., B., Jonathan, T., et al. (2003). Cytoscape: a software environment for integrated models of biomolecular interaction networks. Genome Res. 13, 2498–2504. doi: 10.1101/gr.1239303
Sharma, D., and Surolia, A. (2013). “Degree Centrality BT,” in Encyclopedia of Systems Biology, eds. W. Dubitzky, O. Wolkenhauer, K.-H. Cho, and H. Yokota (New York, NY: Springer New York), 558. doi: 10.1007/978-1-4419-9863-7_935
Shirk, J. L., Ballard, H. L., Wilderman, C. C., Phillips, T., Wiggins, A., Jordan, R., et al. (2012). Public participation in scientific research: a framework for deliberate design. Ecol. Soc. 17:29. doi: 10.5751/ES-04705-170229
Singer, A., Gray, S., Sadler, A., Schmitt Olabisi, L., Metta, K., Wallace, R., et al. (2017). Translating community narratives into semi-quantitative models to understand the dynamics of socio-environmental crises. Environ. Modell. Softw. 97, 46–55. doi: 10.1016/j.envsoft.2017.07.010
Siqueiros-García, J. M., Lerner, A. M., Eakin, H. C., and Hernández Aguilar, B. (2019). A standardization process for mental model analysis in socio-ecological systems. Environ. Modell. Softw. 112, 108–111. doi: 10.1016/j.envsoft.2018.11.016
Slocum, N. (2003). Participatory Methods Toolkit. A Practitioner's Manual. King Baudouin Foundation and the Flemish Institute for Science and Technology Assessment (viWTA). Retrieved from: www.kbs-frb.be; www.viWTA.be; https://cris.unu.edu/participatory-methods-toolkit-practitioners-manual (accessed November 17, 2020).
Soleri, D., Smith, S. E., and Cleveland, D. A. (2000). Evaluating the potential for farmer and plant breeder collaboration: a case study of farmer maize selection in Oaxaca, Mexico. Euphytica 116, 41–57. doi: 10.1023/A:1004093916939
SSSA. (1997). Glossary of Soil Science Terms 1996. Madison, WI: Soil Science Society of America Inc., 139 p
Stone-Jovicich, S. S., Lynam, T., Leitch, A., and Jones, N. A. (2011). Using consensus analysis to assess mental models about water use and management in the crocodile river catchment, South Africa. Ecol. Soc. 16:45. doi: 10.5751/ES-03755-160145
Tengö, M., Brondizio, E. S., Elmqvist, T., Malmer, P., and Spierenburg, M. (2014). Connecting diverse knowledge systems for enhanced ecosystem governance: the multiple evidence base approach. Ambio 43, 579–591. doi: 10.1007/s13280-014-0501-3
Tripp, R. (2001). Agricultural technology policies for rural development. Dev. Policy Rev. 19, 479–489. doi: 10.1111/1467-7679.00146
Tucker, C. M., and Castellanos, E. J. (2010). Perceptions of risk and adaptation: Coffee producers, market shocks, and extreme weather in Central America and Mexico. Glob. Environ. Change 20, 23–32. doi: 10.1016/j.gloenvcha.2009.07.006
Vasslides, J. M., and Jensen, O. P. (2016). Fuzzy cognitive mapping in support of integrated ecosystem assessments: developing a shared conceptual model among stakeholders. J. Environ. Manag. 166, 348–356. doi: 10.1016/j.jenvman.2015.10.038
Vignola, R., Koellner, T., Scholz, R. W., and Mcdaniels, T. L. (2010). Decision-making by farmers regarding ecosystem services: factors affecting soil conservation efforts in Costa Rica. Land Use Policy 27, 1132–1142. doi: 10.1016/j.landusepol.2010.03.003
Winters, P., and Davis, B. (2009). Designing a programme to support smallholder agriculture in Mexico: Lessons from PROCAMPO and oportunidades. Dev. Policy Rev. 27, 617–642. doi: 10.1111/j.1467-7679.2009.00462.x
Zambrano, L., Cano-Santana, Z., Wegier, A., Arroyo-Lambaer, D., Zúñiga-Vega, J. J., Suárez, A., et al. (2019). Evaluating socio-ecological interactions for the management of protected urban green spaces. Front. Environ. Sci. 7:144. doi: 10.3389/fenvs.2019.00144
Zarazúa-Escobar, J. A., Almaguer-Vargas, G., and Ocampo-Ledesma, J. G. (2011). El programa de apoyos directos al campo (PROCAMPO) y su impacto sobre la gestión del conocimiento productivo y comercial de la agricultura del Estado de México. Agricult. Soc. Desarrollo 8, 89–105. Retrieved from: http://www.scielo.org.mx/scielo.php (accessed November 17, 2020).
Keywords: soil, sustainability, collective perception, social-ecological systems, participatory methods, focus group
Citation: Arroyo-Lambaer D, Uscanga A, Piña Tejeda VM, Vázquez-Barrios V, Reverchon F, Rosell JA, Escalante AE, Peña-Ramírez VM, Benítez M and Wegier A (2021) Cognitive Maps Across Multiple Social Sectors: Shared and Unique Perceptions on the Quality of Agricultural Soils in Mexico. Front. Sustain. Food Syst. 4:522661. doi: 10.3389/fsufs.2020.522661
Received: 23 December 2019; Accepted: 09 December 2020;
Published: 13 January 2021.
Edited by:
Steven Gray, Michigan State University, United StatesReviewed by:
Payam Aminpour, Michigan State University, United StatesJesús M. Siqueiros-García, National Autonomous University of Mexico, Mexico
Copyright © 2021 Arroyo-Lambaer, Uscanga, Piña Tejeda, Vázquez-Barrios, Reverchon, Rosell, Escalante, Peña-Ramírez, Benítez and Wegier. This is an open-access article distributed under the terms of the Creative Commons Attribution License (CC BY). The use, distribution or reproduction in other forums is permitted, provided the original author(s) and the copyright owner(s) are credited and that the original publication in this journal is cited, in accordance with accepted academic practice. No use, distribution or reproduction is permitted which does not comply with these terms.
*Correspondence: Ana Wegier, awegier@ib.unam.mx
†Present Address: Denise Arroyo-Lambaer, Laboratorio de Interacciones y Procesos Ecológicos, Facultad de Ciencias, Universidad Nacional Autónoma de México, Ciudad de México, Mexico
Adriana Uscanga, Department of Geography, University of Oregon, Eugene, OR, United States