- 1Department of Health Policy and Administration, The Pennsylvania State University, University Park, PA, United States
- 2Department of Health Sciences, Lakehead University, Thunder Bay, ON, Canada
- 3Department of Nutritional Sciences, University of Michigan, Ann Arbor, MI, United States
Introduction: Although forests and forest-based ecosystems have been shown to influence health and sustainable diets, there is limited evidence on how deforestation affects the current nutrition transition and the double burden of malnutrition. We examined the relationship between deforestation and the individual- and household-level double burden of malnutrition in 15 countries in Sub-Saharan Africa.
Materials and methods: We combined data from geolocated Demographic and Health Surveys and the Global Forest Change dataset. We defined household-level double burden of malnutrition as the co-occurrence of an overweight woman of childbearing age (WCBA) and a stunted pre-school child (PSC) within the same household. We defined individual-level double burden in two ways: (1) as the co-occurrence of overweight and anemia within an individual WCBA, and (2) as the co-occurrence of overweight and stunting within a PSC. We used logistic regression analysis to examine the association between forest cover loss and these three measures after adjusting for potential confounders. We also assessed the mechanisms linking forest cover loss and nutritional status, such as livestock ownership and access to clean water.
Results: In our sample, the prevalence rates of the three measures of the double burden were: overweight and anemic WCBA: 8.4%, overweight WCBA and stunted PSC: 6.9%, overweight and stunted PSC: 2.7%. After adjusting for the confounders as well as country fixed effects and the month of the survey, forest cover loss was marginally associated with a higher odds of an overweight WCBA and stunted PSC [odds ratio (95% CI): 4.80 (0.82, 28.25)]. We found no association between forest cover loss and odds of an overweight and stunted PSC [odds ratio (95% CI): 2.47 (0.80, 7.60)] or the odds of an anemic and overweight WCBA [odds ratio (95% CI): 0.71 (0.15, 3.32)].
Discussion: Deforestation does not seem to be an important driver of the double burden of malnutrition in SSA. However, deforestation influences several intermediate factors which, in turn, may influence the double burden. The overall weak association between forest cover loss and double burden measures mask significant heterogeneity across regions within SSA. Future research should unpack the mechanisms behind these regional differences.
Introduction
Deforestation is occurring globally at an alarming rate (Hosonuma et al., 2012; Hansen et al., 2013), particularly in the tropics (Lindquist et al., 2012; Hansen et al., 2013), raising concerns about potential impacts on biodiversity, ecosystems services, and human health. Deforestation has been shown to be associated with a number of conditions, such as acute respiratory infection (Pienkowski et al., 2017), malaria (Bauch et al., 2015; Austin et al., 2017; Berazneva and Byker, 2017), and diarrheal disease (Johnson et al., 2013; Berazneva and Byker, 2017).
Simultaneously, many low- and middle-income countries are experiencing a rapid rise in the prevalence of the double burden of malnutrition (Dieffenbach and Stein, 2012; Oddo et al., 2012; Roemling and Qaim, 2013; Wojcicki, 2014; Berazneva and Byker, 2017), wherein obesity and diet-related chronic disease commonly co-occur with conditions of undernutrition (e.g., child growth stunting, micronutrient deficiencies, and associated anemia). An emerging body of literature has examined this double burden as well as its determinants (Lee et al., 2010, 2012; Oddo et al., 2012; Bassete et al., 2014; Aitsi-Selmi, 2015; Kosaka and Umezaki, 2017). The most commonly assessed determinants include socio-economic determinants, such as urban/rural residence, income, and maternal/household-head education level (Kosaka and Umezaki, 2017). Empirical evidence on the role of environmental determinants, such as forest cover loss, is lacking. Given the pace, urgency, and scale of global environmental changes, there is a growing need to address this knowledge gap.
Forests and forest-based ecosystem services have also been shown to be important drivers of healthy and sustainable diets (Dounias and Froment, 2011; Golden et al., 2011; Vinceti et al., 2013; Brown et al., 2014; Ickowitz et al., 2014, 2016; Powell et al., 2015; Vira et al., 2015; Pienkowski et al., 2017; Rowland et al., 2017; Galway et al., 2018; Rasolofoson et al., 2018), suggesting that deforestation has the potential to affect the double burden of malnutrition. To our knowledge, two prior studies (Rasolofoson et al., 2018 and Galway et al., 2018) have provided frameworks for understanding the potential mechanisms linking forests to diets. Broadly speaking, the mechanisms include changes in the availability of forest foods, pollination, the availability of non-forest products, mother's time for food preparation and child care activities, and agricultural techniques (Rasolofoson et al., 2018). In Galway et al. (2018), we provide a finer breakdown of these mechanisms with supporting evidence from the literature.
The mechanisms through which forest cover loss could affect the double burden of malnutrition are likely similar, with changes in diet as an important intermediate factor. Therefore, in Figure 1, we adapt the framework from Galway et al. (2018) with the double burden of malnutrition as the outcome. The shaded areas of the framework are taken from Galway et al. (2018), while the darker boxes and arrows represent additional factors linking forest loss to the nutritional double burden (In Figure 1, the factors we evaluate in the current study are highlighted in bold). Two of the additional factors need elaboration. First, changes in the amount of time spent gathering firewood or changes in agricultural practices can affect women's calorie expenditure, which in turn can affect anthropometric status, in particular weight. Indeed, existing evidence shows that women tend to get less physical activity than men in an urban environment (Shrimpton and Rokx, 2012), which may lead to an increased risk of overweight. Second, individuals' access to clean water and sanitation may change—either because they move in response to deforestation or their existing water source gets contaminated. Such changes in access to water and sanitation can affect children by making them more vulnerable to illnesses, such as diarrheal diseases and infections, which may lead to an increased risk of anemia. If the exposure to pathogens is persistent, children may also be at higher risk of stunting.
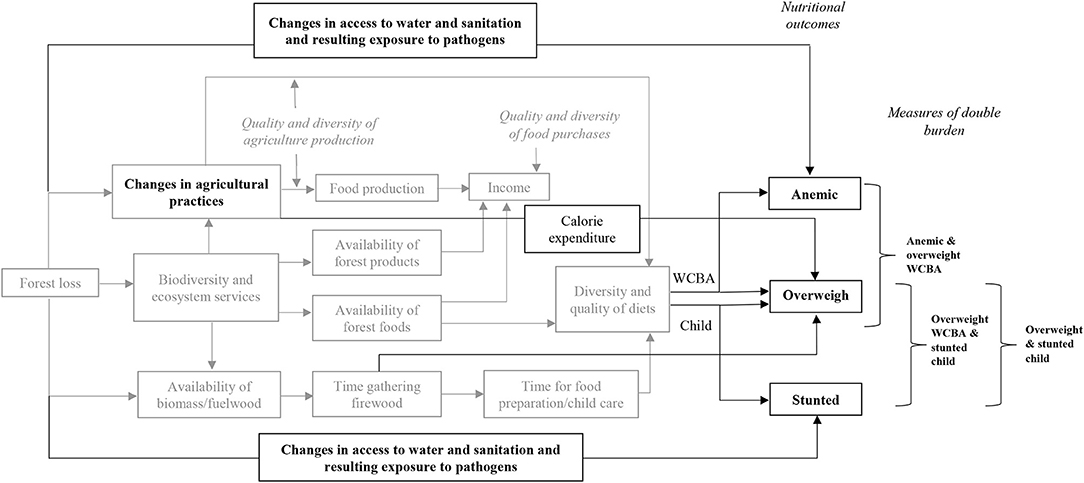
Figure 1. Conceptual framework illustrating hypothesized links between forest loss and double burden of malnutrition*. The shaded part of the framework is adapted from Galway et al. (2018) with the authors' permission. The outcomes we evaluate in the current study are highlighted in bold.
Deforestation may also affect different population segments differently, even within the same household. For example, mothers might be impacted differently than young children. If the primary reason for deforestation is to make space for cultivation of crops, mothers, now having to work in the field, may end up having less time for breastfeeding and other caregiving activities, potentially worsening children's nutritional status. Their own nutritional status may improve or worsen, depending on how their work burden changes. By altering diets, deforestation may also affect the multiple measures of malnutrition differently even for the same child. For example, if more iron-rich crops are grown, the likelihood of being anemic may fall, but if mother's caregiving is adversely affected—for example, through reduced frequency and duration of breastfeeding—a child may be at increased risk of stunting or wasting.
The overall effect of deforestation on the nutritional double burden, both at the household level and at the individual level remains an unanswered and important empirical question. Against this backdrop, the goal of this study was to examine the association between deforestation and the household- and individual-level double burden of malnutrition. We conducted this study in the context of Sub-Saharan Africa (SSA), where the rate of deforestation is twice the world average (UNEP, 2008; FAO, 2010; Gibson et al., 2011; D'Annunzio et al., 2015; Austin et al., 2017). The nutritional double burden is also prevalent throughout SSA and is certain to increase (Zeba et al., 2012; Wojcicki, 2014; Jones et al., 2016).
Materials and Methods
Data
We used geolocated data collected by the USAID-funded Demographic and Health Survey (DHS) program. The DHS program collects nationally representative health, fertility and nutrition data from more than 90 countries using a two-stage cluster sampling procedure (Measure DHS/ICF International, 2012). We compiled individual and children's recode DHS data files from all countries in SSA for which the standard DHS survey was conducted between 2012 and 2016 and for which geolocated data were available. In total, datasets from 15 countries met these criteria and were included in our analyses (Table 1). We included all non-pregnant women of childbearing age (WCBA) from 15 to 49 years of age who were interviewed in the DHS as well as pre-school children (PSC) aged 12–59 months. In our analysis of PSC, we included all children from a mother except in few instances where two children from the same mother were coded as having the same birth index.
We obtained data on forest cover loss (a proxy of deforestation) from the publicly available Global Forest Change dataset developed (Hansen et al., 2013). The Global Forest Change dataset, developed by Hansen and colleagues, measures forest cover loss worldwide (excluding Antarctica and the Arctic) at a spatial resolution of ~30 m (Hansen et al., 2013). Hansen et al. (2013) define forest loss as stand-replacement disturbance or the complete removal of tree cover canopy at the pixel scale while trees are defined as vegetation taller than 5 m in height. Following our previous study (Galway et al., 2018), we used version 1.0 of the dataset which measured forest cover loss between 2000 and 2012 using a time-series analysis of more than 600,000 multispectral satellite images from Landsat 7. We used version 1.0 rather than the updated version of the dataset to ensure that our measurement of forest lost preceded the DHS survey data collection. We also used data on percent tree cover for the year 2000 from the Global Forest Change dataset (Hansen et al., 2013). Information on road location was obtained from the Global Roads Open Access Data Set (Center for International Earth Science Information Network, 2013). Finally, we used the Global Aridity Index from the Consultative Group on International Agricultural Research (CGIAR, 2009) to measure climate across DHS clusters. We combined data from the DHS and the Global Forest Change dataset at the cluster-level using a geographic information system and ArcGIS software (ESRI, 2011).
Outcome Variables
We defined overweight or obesity in WCBA using standard BMI (in kg/m2) cutoffs (≥25). Anemia in WCBA was defined as a hemoglobin concentration <120 g/L, the recommended cutoff for non-pregnant women aged ≥15 years. We considered children with a HAZ <2 SD below the mean according to the WHO Child Growth Standards to be stunted and those with WHZ >2 SD above the mean to be overweight.
We used three measures of double burden: two at the individual level and one at the household level. We defined individual-level double burden in two ways: (1) as the co-occurrence of overweight and anemia within an individual WCBA, and (2) as the co-occurrence of overweight and stunting within a PSC. We defined household-level double burden of malnutrition as the co-occurrence of an overweight WCBA and a stunted PSC within the same household.
In an effort to understand potential mechanisms linking deforestation to the nutritional double burden, we also examined the relationship between forest loss and five intermediate outcomes: (i) ownership of livestock, (ii) ownership of agricultural land, (iii) access to improved water, (iv) access to improved sanitation, and (v) occurrence of diarrhea among the PSCs.
As discussed in the results section below, for the overall sample, we found only a weak association between forest cover loss and the co-occurrence of overweight and stunting within a PSC and no association between forest cover loss and the two other measures of double burden. This contradicted with the negative associations we found between forest cover loss and many of the intermediate outcomes. Therefore, we also examined the association between forest cover loss and individual components of nutritional status; in these analyses, the outcome variables were the components used to construct the double-burden measures, specifically occurrences of: (i) overweight WCBA, (ii) anemic WCBA, (iii) overweight PSC, and (iv) stunted PSC.
Independent Variable
The primary independent variable we examined was deforestation measured as forest cover loss between 2000 and 2012. Though the construction of this variable has been previously described (Galway et al., 2018), briefly, the Global Forest Change dataset defines forest cover loss as stand-replacement disturbance or a change from a forest to non-forest state during the 2000–2012 period in a 30 m by 30 m grid cell. In the dataset, each 30 m by 30 m pixel is coded as “1” for forest cover loss or “0” for no loss of forest cover. The Global Forest Change data are downloadable as tiff panels; we downloaded those panels covering the spatial extent of our 15 study countries in SSA (Hansen et al., 2013). The georeferenced DHS cluster locations are randomly displaced in order to protect the confidentiality of the survey respondents (Warren et al., 2016). The large majority (99%) of the locations are displaced by 0–5 km, with a remaining 1% of rural clusters displaced to a maximum of 10 km (Measure DHS/ICF International, 2012). Using ArcGIS software (ESRI, 2011), we aggregated the original 30 m pixels to 5 km pixels to account for the displacement. We then spatially joined the aggregated pixels to the georeferenced DHS data to extract the percentage of forest cover loss in the 5 km area surrounding each DHS cluster.
Covariates
We included several covariates in analyses to adjust for potential confounding of the relationship between forest loss and the double burden of malnutrition. We selected these covariates based on previous evidence of the determinants of nutritional status (Guldan et al., 1993; Variyam et al., 1999; Vereecken et al., 2004; Black et al., 2013). The child-level covariates included sex, episode of diarrhea during the 2 weeks preceding the survey, and age in months. Other covariates included the highest attained education level of the child's mother, household access to an improved source of water and sanitation (WHO/UNICEF Joint Monitoring Programme for Water Supply and Sanitation, 2012), and a measure of household wealth available from the DHS. DHS creates the wealth index from several items related to household assets (e.g., radio, refrigerator), housing characteristics (e.g., type of flooring), and utilities and infrastructure (e.g., number of persons sleeping per room) (Rutstein and Johnson, 2004). Households are divided into quintiles based on the index. In a subset of the analyses, we also include household's ownership of agricultural land and ownership of livestock as covariates. We show results from both sets of regressions (i.e., those with and without controlling for agricultural land and livestock ownership) in an attempt to shed light on changes in the double burden measures originating from changes in deforestation independent of agricultural land use (to the extent that changes in land use are reflected in the ownership of agricultural land and livestock).
At the cluster level, we adjusted for whether the cluster was urban or rural based on DHS definitions and distance of the DHS cluster to the nearest road. We calculated the Euclidian distance of the DHS cluster centroid to the nearest road using road location data from the Global Roads Open Access Data Set (Center for International Earth Science Information Network, 2013). Climatic differences may in part explain geographic variation in mother and child nutrition outcomes (Dulal et al., 2017). To adjust for such confounding, we used the Global Aridity Index (CGIAR, 2009), aggregated to the 5 km level and spatially linked to each cluster. We also adjusted for baseline forest cover using percent tree cover in year 2000 (Hansen et al., 2013). As with the forest cover loss data, we aggregated the original 30 m pixels to 5 km pixels and spatially joined the data to each DHS cluster. In an attempt to account for nutritional differences that may have been driven by factors specific to a country (e.g., country's gross domestic product and government's policies on nutrition and health) or DHS cluster, and by seasonal variation in food availability (Abizari et al., 2017), we adjusted for country fixed effects, cluster-level random effects, and the month of the DHS survey in our analyses.
Statistical Analysis
We calculated means and proportions for the outcome and independent variables, as well as for key household- and child-level characteristics. Given the binary nature of the outcome variables, we used logistic regression analysis to examine the association between forest cover loss and the measures of the double burden of malnutrition, after adjusting for potential individual-, household- and community-level confounders. Specifically, we estimated the following equation:
where Yijk is the relevant double burden of malnutrition measure for either child, mother, or child-mother pair i in household j living in DHS cluster k. Forest cover loss, the independent variable, varied by the DHS cluster. We reported odds ratios. In the equation, the odds ratio β1 reflects the association between forest cover loss and the dependent variable. X represents child-, household- and cluster-level characteristics mentioned above that potentially influence the double burden of nutrition. η represents the country of the child, θ represents the cluster random effect, while ω represents the month of the survey.
In all models, we clustered the standard errors at the level of the DHS enumeration cluster to account for arbitrary correlation between observations within a cluster. Recall that the variation in forest cover loss is at the DHS cluster level. Clustering standard errors at the level of DHS sampling units also accounts for intra-household correlations among those households with multiple children or mothers in the sample.
The statistical significance of associations is reported at the P < 0.1, P < 0.05, and P < 0.01 levels. Given current debates on the arbitrary nature of these cutoffs (Wasserstein et al., 2019), we report 95% confidence intervals for all major findings. The analyses were carried out using the Stata statistical software package version 15 (StataCorp, 2017) and the ArcGIS software (ESRI, 2011). We checked the robustness of the main results by estimating the relationship between key variables in a step-wise manner, controlling for different set of covariates in each step.
Results
Descriptive Data
In our analytical sample of 25,285 WCBA for whom both measures of the nutritional double burden (overweight and anemia) were available, 73,941 PSC, and 69,082 mother-child pairs, the prevalence rates of the three measures of the double burden were: overweight and anemic WCBA: 8.4%; overweight and stunted PSC: 2.7%; overweight WCBA and stunted PSC: 6.9% (Table 2). The average forest cover loss between 2000 and 2012, the independent variable, was ~2%.
The average age of the child was 35 months and there were equal proportions of boys and girls in the sample. The vast majority of mothers in the sample (75%) had primary level education or lower. Among the mothers, the average age was 30 years, nearly 43% were anemic and 22% were overweight.
The average number of members in a household was 7.4. Approximately 61% households had access to an improved source of drinking water and 43% had access to improved sanitation. Two-thirds of the children lived in areas classified as rural. The average forest cover in 2000, the aridity index and the distance to the nearest road were 20%, 8, and 16 km, respectively.
Main Results
In Table 3, we report odds ratios from estimating equation (1), separately for the three dependent variables. In each case, we first show results from estimating the equation controlling for all covariates except household's ownership of agricultural land and livestock. We then show odds ratios from estimating the equation with all covariates, including ownership of agricultural land and livestock. As mentioned earlier, we present results in this manner in an attempt to shed light on changes in the double burden measures originating from changes in deforestation independent of agricultural land use.
In regressions that do not control for the ownership of agricultural land and livestock, forest cover loss was marginally associated with overweight and stunted PSC [odds ratio (95% CI): 4.74 (0.80, 27.88)], but not with overweight and anemic WCBA [odds ratio (95% CI): 0.71 (0.15, 3.35)] or with overweight WCBA and stunted child [odds ratio (95% CI): 2.53 (0.82, 7.81)]. The odds ratios remain largely unchanged even when we control for the ownership of agricultural land and livestock.
Among the covariates, in both set of models, primary education among WCBA was associated with higher odds of concurrent anemia and overweight relative to no education. However, there was marginal or no difference between women with no education and those with higher education. Higher education among women were associated with lower odds of overweight mother and stunted child, while secondary education was associated with overweight and stunted child. Household wealth was strongly positively associated with two of the three measures, but not with overweight and stunted child. Other factors strongly associated with concurrent anemia and overweight among WCBA included women's age, urban location, access to improved water, access to improved sanitation, and aridity index. Factors strongly associated with overweight mother and stunted child included women's age, urban location, household size, access to improved sanitation, child's age and gender, forest cover in 2000, and distance to the nearest road. Finally, factors strongly associated with overweight and stunted child included mother's age (negative association), access to improved sanitation, child's age, and forest cover in 2000 (negative and marginally significant association). In sum, different sets of covariates influenced the double burden measures depending on the measure we examined, and the only covariate that influenced all three measures in the same direction was improved sanitation. Ownership of agricultural land and livestock were associated with lower odds of concurrent anemia and overweight among WCBA, while only the ownership of livestock was associated with lower odds of overweight mother and stunted child. We found no association between the ownership of agricultural land or livestock and overweight and stunted PSC.
Although the association between forest loss and overweight and stunted PSC were only marginally significant (i.e., significant only at the 10% significance level), the estimated odds ratio was robust to controlling for different set of covariates, as shown in Appendix Table A1.
Potential Mechanisms, and Results by Region
Discussion in this subsection proceeds in the following manner. We first discuss the relationships between forest cover loss and intermediate outcomes (e.g., ownership of livestock), and between forest cover loss and the individual components of our double burden measures (e.g., anemic WCBA) for the overall sample. We then discuss the relationships by region.
For the overall sample, forest cover loss was strongly associated with lower odds of owning livestock, but not associated with ownership of agricultural land (Table 4A). It was strongly associated with lower odds of having access to improved source of water and marginally associated with improved sanitation. It was strongly associated with higher odds of the occurrence of diarrhea among the PSC.
In terms of the individual components of the double burden measures, forest cover loss was associated with higher odds of anemic WCBA and not associated with any of the remaining three components (Table 5A).
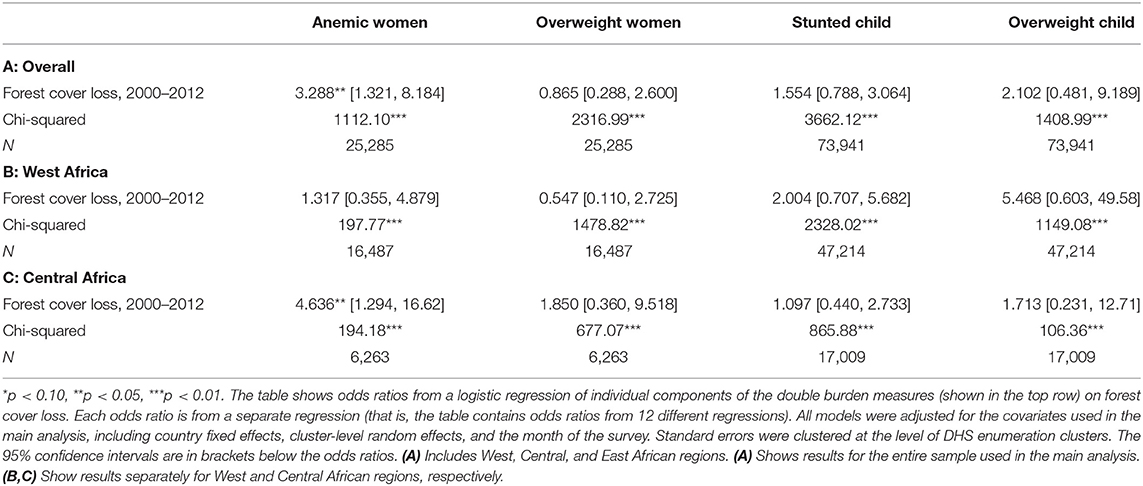
Table 5. Odds ratio from logistic regressions of individual components of double burden measures on forest cover loss.
Table 5A also provides some insight into our main finding reported in Table 3 and suggests that the strong association between forest cover loss and only one of the three double-burden measures is due to approach in which we construct the double-burden measures. In Table 5, among the three measures of double burden, the associations with forest cover and individual components are in the same direction only for the components related to PSC (stunted and overweight child). For the remaining two measures the associations are in the opposite direction. For example, take anemic WCBA and overweight WCBA. Forest cover loss is positively associated with the odds of anemic WCBA but negatively associated (although statistically not significant) with overweight women. Likewise, forest cover loss is positively associated with stunted PSC but negatively associated with overweight WCBA.
The wide confidence intervals reported in this table preclude an analysis at a lower geographic level (e.g., country), which would be more meaningful for designing policies. The small sample size is a concern particularly for eastern Africa (which includes only two countries: Kenya and Rwanda). Therefore, in assessing the relationship between forest cover and intermediate outcomes as well as the individual components of the double burden at the regional level, we focus on western and central Africa.
The overall results mask significant heterogeneity across geographic regions within SSA. As shown in Table 6, the significant association between forest cover loss and overweight and stunted PSC are driven by the association in West Africa, while no such relationship exists in Central and East Africa.
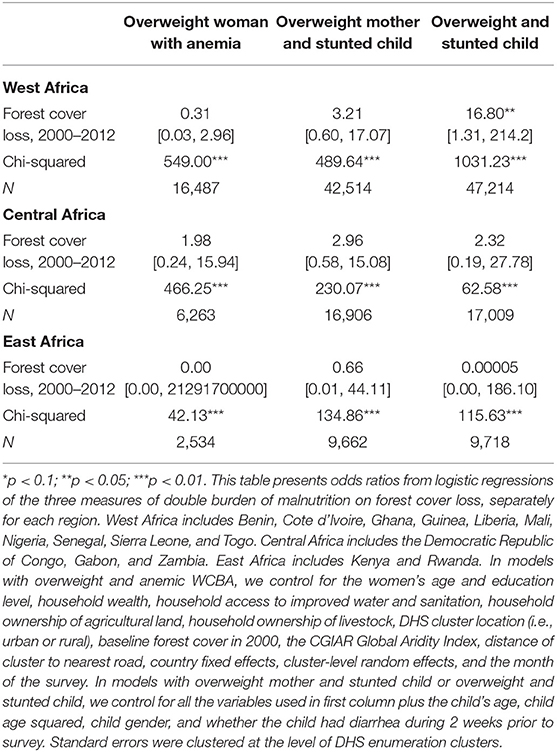
Table 6. Odds ratio from a logistic regression of measures of double burden on forest cover loss, by region.
There are also significant regional differences in the association of forest cover with the intermediate factors as well as the individual measures of nutritional status. With respect to the intermediate factors, forest cover loss is associated with lower odds of owning livestock and lower odds of owning agricultural land in West Africa (Table 4B). It is associated with higher odds of the incidence of diarrhea among the PSCs in the region. Unlike in the overall sample, there is no association between forest cover loss and access to improved water or sanitation. In contrast, in the central region, forest cover loss is associated with higher odds of owning agricultural land and lower odds of the incidence of diarrhea (Table 4C). The association between forest cover loss and access to improved water is in the same direction as that of the overall sample. The key message from Table 4 is that the mechanisms through which forest cover loss influences nutritional double burden differ between the regions. With respect to the individual components of the double burden measures, however, the relationships at the regional level are in agreement with those for the entire sample (Tables 5B,C).
Discussion
The double burden of malnutrition is linked to the ongoing epidemiologic transition, whereby non-communicable conditions, such as cardiovascular diseases and diabetes, are replacing communicable conditions, such as malaria, as the primary causes of morbidity and mortality in low- and middle-income countries (Shrimpton and Rokx, 2012). An emerging body of literature has examined the socio-economic determinants (e.g., education, urbanicity, and income) of the double burden within the same household (Kosaka and Umezaki, 2017). Existing literature has also shown that environmental changes such as deforestation are linked to changes in diet, a key input to an individual's nutritional status (Rasmussen et al., 2017; Reed et al., 2017), as well as measures of malnutrition, such as underweightness (Pienkowski et al., 2018). However, remarkably little has been written on the possible linkages between deforestation and the nutritional double burden. Generally speaking, the ecological determinants of the double burden are poorly understood. This is an important omission because the double burden of malnutrition may be an important mechanism linking environmental changes to diets and non-communicable conditions (Frumkin and Haines, 2019). More importantly, deforestation may have a temporal dimension, through which different segments of the population might be affected differently and the same individual might be affected differently based on the outcome measured. In the current study, however, we found only a marginal association between the measure of the double burden pertaining to the child (overweight and stunted PSC) and forest cover loss and no association between forest cover loss and the remaining two measures. These findings suggest that the effect of deforestation observed in other health outcomes may either not extend or extend only marginally to the nutritional double burden, although the lack of association seems in part due to the way the double burden measures are constructed.
We must interpret our findings with a number of caveats. First, as we have pointed out previously (Galway et al., 2018), the Global Forest Change dataset defines trees as vegetation taller than five meters, and therefore underestimates true forest loss. Second, the forest loss data used does not take into account reforestation that may have taken place during the period. If the current double burden of malnutrition reflects the net effect of deforestation and reforestation, our estimates are underestimates of the true association.
Third, although we controlled for a range of potential confounders in our analysis, we cannot interpret the observed associations as causal. Our data are cross-sectional and as such we are unable to employ panel data methods available for establishing causal relationships. There is limited possibility of reverse causality (i.e., double burden of malnutrition triggering deforestation), in part because, by our study design, the timing of forest cover loss (2000–2012) precedes that of the DHS surveys (after 2013). Nonetheless, there could be omitted variables—such as food availability and access to market—for which we could not control given the data. Finally, we are unable to comment fully on how land use patterns, income, and lifestyle (e.g., amount of movement, time spent on fetching water and firewood or collecting fodder for livestock) may have changed as a result of deforestation and how those changes may influence the nutritional double burden.
Notwithstanding these limitations, the association between forest cover loss and the measure having child-level indicators—although it is only marginal—is worrying from a policy perspective, given the long-lasting nature of early-life nutritional deficiencies. A large body of research has shown that poor nutritional status in childhood has lasting effects into adulthood. For example, early-life nutrition is an important determinant of one's long-term productivity, earnings, and health (Alderman et al., 2006; Dewey and Begum, 2011; Currie and Vogl, 2013). Our findings contribute to the ongoing dialogue about the need to prevent deforestation and conserve biodiversity at a range of spatial scales for multiple goals, including limiting the potential adverse impacts on child malnutrition and children's long-term wellbeing.
More generally, we have previously shown that deforestation is associated with the diversity and quality of children's diet, and proposed various mechanisms for the association (Galway et al., 2018). The analysis presented here—using the same set of countries and periods as the previous study—showed that the association of forest cover loss may extend, albeit marginally, to children's anthropometric measures of the double burden of malnutrition, specifically the likelihood of simultaneously being overweight and stunted. One possible mechanism for this association is that reduced consumption of legumes and nuts, flesh foods, and other fruits and vegetables resulting from deforestation—as we established in the previous study—deleteriously affects child linear growth. Simultaneously, deforestation may raise the risk of overweight if calorie intake is increased by consuming energy-dense processed foods. When we examined the association between components of double burden measures—stunting and overweight—we find that both of these measures are positively associated with forest cover loss although the associations are not statistically significant.
Given the limitations of our data, we are unable to examine why deforestation is not associated with the prevalence of anemic and overweight WCBA or overweight WCBA and stunted PSC and is only marginally associated with the prevalence of overweight and stunted PSC. Additional data—including on, for example, the drivers of deforestation and usages of the deforested areas—will be needed to examine such questions. Likewise, because the prevalence of overweight also depends on lifestyle and habits (see Figure 4 in WHO, 2017), information on how deforestation influences these factors will be central to understanding the temporal dimension, if one exists. One can hypothesize, for example, that when forests are cleared for construction of building, it increases the amount of time women spend on finding fodder for cattle or firewood, thus expending more calories and reducing the chances of gaining excess weight (thus reducing the chances of being overweight and anemic). For the children, on the other hand, cleared land and development of a local marketplace may mean easier access to processed foods that contribute to unhealthy weight gains (thus increasing the chances of being overweight). Similarly, one can hypothesize that, other environmental factors that deforestation affects, such as access to clean drinking water (Mapulanga and Naito, 2019), may affect the nutritional status of young children, but not adults. These examples are only illustrative of the complex ways that deforestation may influence individual behavior and contribute to the double burden of malnutrition.
Additional research will be required to confirm the findings in our study, establish mechanisms, and to uncover any potential temporal dimensions (specifically, to examine different effects of forest cover loss among different population segments). For effective policy design, the mechanisms will need to be examined at the regional and country levels, as the mechanism may vary at those levels—as our analysis at the level of the regions within SSA suggests. For example, it is not clear why forest cover loss is negatively associated with the ownership of agricultural land in the West Africa region but positively associated in the Central Africa region. Some of the differences in potential mechanisms may be due to social and economic factors, such as education and wealth as the regions vary widely in these dimensions, but this needs to be investigated further.
For effective policy design, it is also important to understand the relative importance of different mechanisms shown in Figure 1. Among the various mechanisms, as mentioned before, several studies have hypothesized and assessed the association between forest cover loss and diets. This focus on diets is not surprising given SSA's reliance on forests for food. For example, 60 percent of the households in 11 African countries have been shown to collect wild food from forests (Hickey et al., 2016). Likewise, using data from 37 communities in 24 countries, including five in Africa, Rowland et al. (2017) find that more than half of the households in their sample collected forest food for consumption. However, the research linking forest cover loss to diets is far from conclusive, and the reliance on forests for food varies across regions, cultures, and population sub-groups. The strength of other mechanisms, therefore, likely varies across these dimensions as well.
Conclusion
Deforestation does not seem to be an important driver of the double burden of malnutrition in SSA. We found no association between deforestation and measures of the double burden pertaining to the same WCBA or mother-child pair within the household. The association we found between deforestation and the measure based on the same child is worrying from a policy perspective, even though additional research will be required to confirm it. If this association is robust, the effect of deforestation may have a temporal aspect, which also warrants further research. More generally, there is a need to better understand the potential mechanisms linking forest cover loss to health and nutrition, their relative contributions, and differences across geographic regions and countries.
Data Availability Statement
Data used in this study were obtained from Demographic and Health Surveys (DHS) and the Global Forest Change dataset. The DHS data are available after a simple registration process (https://dhsprogram.com/data/new-user-registration.cfm). The Global Forest Change data are available from http://earthenginepartners.appspot.com/science-2013-global-forest.
Ethics Statement
Ethical approval was not required for this study as it used publicly-available de-identified data.
Author Contributions
YA, LG, and AJ conceptualized the study. YA and LG prepared the dataset. YA and SN conducted the statistical analysis. All authors contributed in the preparation of the final manuscript and approved it for publication.
Conflict of Interest
The authors declare that the research was conducted in the absence of any commercial or financial relationships that could be construed as a potential conflict of interest.
References
Abizari, A.-R., Azupogo, F., Nagasu, M., Creemers, N., and Brouwer, I. D. (2017). Seasonality affects dietary diversity of school-age children in northern Ghana. PLoS ONE 12:e0183206. doi: 10.1371/journal.pone.0183206
Aitsi-Selmi, A. (2015). Households with a stunted child and obese mother: trends and child feeding practices in a middle-income country, 1992–2008. Matern. Child Health J. 19, 1284–1291. doi: 10.1007/s10995-014-1634-5
Alderman, B. H., Hoddinott, J., Kinsey, B., and Bank, W. (2006). Long term consequences of early childhood malnutrition. Oxf. Econ. Pap. 58, 450–474. doi: 10.1093/oep/gpl008
Austin, K. G., González-Roglich, M., Schaffer-Smith, D., Schwantes, A. M., and Swenson, J. J. (2017). Trends in size of tropical deforestation events signal increasing dominance of industrial-scale drivers. Environ. Res. Lett. 12:054009. doi: 10.1088/1748-9326/aa6a88
Bassete, M. N., Romaguera, D., Giménez, M. A., Lobo, M. O., and Samman, N. C. (2014). Prevalence and determinants of the dual burden of malnutrition at the household level in Puna and Quebrada of Humahuaca, Jujuy, Argentina | Prevalencia y determinantes de la doble carga de malnutrición en hogares en la puna y quebrada de humahuaca, Jujuy. Nutr. Hosp. 29, 322–330. doi: 10.3305/nh.2014.29.2.7075
Bauch, S. C., Birkenbach, A. M., Pattanayak, S. K., and Sills, E. O. (2015). Public health impacts of ecosystem change in the Brazilian Amazon. Proc. Natl. Acad. Sci.U.S.A. 112, 7414–7419. doi: 10.1073/pnas.1406495111
Berazneva, J., and Byker, T. S. (2017). Does forest loss increase human disease? Evidence from Nigeria. Am. Econ. Rev. 107, 516–521. doi: 10.1257/aer.p20171132
Black, R. E., Victora, C. G., Walker, S. P., Bhutta, Z. A., Christian, P., De Onis, M., et al. (2013). Maternal and child undernutrition and overweight in low-income and middle-income countries. Lancet 382, 427–451. doi: 10.1016/S0140-6736(13)60937-X
Brown, M. E., Grace, K., Shively, G., Johnson, K. B., and Carroll, M. (2014). Using satellite remote sensing and household survey data to assess human health and nutrition response to environmental change. Popul. Environ. 36, 48–72. doi: 10.1007/s11111-013-0201-0
CGIAR (2009). Global Aridity and PET Database. Available online at: https://www.cgiar-csi.org
Currie, J., and Vogl, T. (2013). Early-life health and adult circumstance in developing countries. Ann. Rev. Econ. Ann. Rev. 5, 1–36. doi: 10.3386/w18371
D'Annunzio, R., Sandker, M., Finegold, Y., and Min, Z. (2015). Projecting global forest area towards 2030. For. Ecol. Manage. 352, 124–133. doi: 10.1016/j.foreco.2015.03.014
Dewey, K. G., and Begum, K. (2011). Long-term consequences of stunting in early life. Matern. Child Nutr. 7, 5–18. doi: 10.1111/j.1740-8709.2011.00349.x
Dieffenbach, S., and Stein, A. D. (2012). Stunted child/overweight mother pairs represent a statistical artifact, not a distinct entity. J. Nutr. 142, 771–773. doi: 10.3945/jn.111.153387
Dounias, E., and Froment, A. (2011). From foraging to farming among present-day forest hunter-gatherers: consequences on diet and health. Int. For. Rev. 13, 294–304. doi: 10.1505/146554811798293818
Dulal, B., Mundy, G., Sawal, R., Rana, P. P., and Cunningham, K. (2017). Homestead food production and maternal and child dietary diversity in Nepal: variations in association by season and agroecological zone. Food Nutr. Bull. 38, 338–353. doi: 10.1177/0379572117703264
Frumkin, H., and Haines, A. (2019). Global environmental change and noncommunicable disease risks. Annu. Rev. Public Health 40, 261–282. doi: 10.1146/annurev-publhealth-040218-043706
Galway, L. P., Acharya, Y., and Jones, A. D. (2018). Deforestation and child diet diversity: a geospatial analysis of 15 Sub-Saharan African countries. Heal. Place 51, 78–88. doi: 10.1016/j.healthplace.2018.03.002
Gibson, L., Lee, T. M., Koh, L. P., Brook, B. W., Gardner, T. A., Barlow, J., et al. (2011). Primary forests are irreplaceable for sustaining tropical biodiversity. Nature 478, 378–381. doi: 10.1038/nature10425
Golden, C. D., Fernald, L. C. H., Brashares, J. S., Rasolofoniaina, B. J. R., and Kremen, C. (2011). Benefits of wildlife consumption to child nutrition in a biodiversity hotspot. Proc. Natl. Acad. Sci. U.S.A. 108, 19653–19656. doi: 10.1073/pnas.1112586108
Guldan, G. S., Zeitlin, M. F., Beiser, A. S., Super, C. M., Gershoff, S. N., and Datta, S. (1993). Maternal education and child feeding practices in rural Bangladesh. Soc. Sci. Med. 36, 925–935. doi: 10.1016/0277-9536(93)90084-H
Hansen, M. C., Potapov, P. V., Moore, R., Hancher, M., Turubanova, S. A., Tyukavina, A., et al. (2013). High-resolution global maps of 21st-century forest cover change. Science 342, 850–853. doi: 10.1126/science.1244693
Hickey, G. M., Pouliot, M., Smith-Hall, C., Wunder, S., and Nielsen, M. R. (2016). Quantifying the economic contribution of wild food harvests to rural livelihoods: a global-comparative analysis. Food Policy 62, 122–132. doi: 10.1016/j.foodpol.2016.06.001
Hosonuma, N., Herold, M., Sy, V., De Fries, R. S., De Brockhaus, M., Verchot, L., et al. (2012). An assessment of deforestation and forest degradation drivers in developing countries. Environ. Res. Lett. 7:044009. doi: 10.1088/1748-9326/7/4/044009
Ickowitz, A., Powell, B., Salim, M. A., and Sunderland, T. C. H. (2014). Dietary quality and tree cover in Africa. Glob. Environ. Chang. 24, 287–294. doi: 10.1016/j.gloenvcha.2013.12.001
Ickowitz, A., Rowland, D., Powell, B., Salim, M. A., and Sunderland, T. (2016). Forests, trees, and micronutrient-rich food consumption in Indonesia. PLoS ONE 11:e0154139. doi: 10.1371/journal.pone.0154139
Johnson, K. B., Jacob, A., and Brown, M. E. (2013). Forest cover associated with improved child health and nutrition: evidence from the Malawi demographic and health survey and satellite data. Glob. Heal. Sci. Pract. 1, 237–248. doi: 10.9745/GHSP-D-13-00055
Jones, A. D., Acharya, Y., and Galway, L. P. (2016). Urbanicity gradients are associated with the household- and individual-level double burden of malnutrition in Sub-Saharan Africa. J. Nutr. 146, 1257–1267. doi: 10.3945/jn.115.226654
Kosaka, S., and Umezaki, M. (2017). A systematic review of the prevalence and predictors of the double burden of malnutrition within households. Br. J. Nutr. 117, 1118–1127. doi: 10.1017/S0007114517000812
Lee, J., Houser, R. F., Must, A., De Fulladolsa, P. P., and Bermudez, O. I. (2010). Disentangling nutritional factors and household characteristics related to child stunting and maternal overweight in Guatemala. Econ. Hum. Biol. 8, 188–196. doi: 10.1016/j.ehb.2010.05.014
Lee, J., Houser, R. F., Must, A., De Fulladolsa, P. P., and Bermudez, O. I. (2012). Socioeconomic disparities and the familial coexistence of child stunting and maternal overweight in guatemala. Econ. Hum. Biol. 10, 232–241. doi: 10.1016/j.ehb.2011.08.002
Lindquist, J. J., D'Annunzio, R., Gerrand, A., MacDicken, K., Achard, F., Beuchle, R., et al. (2012). Global Forest Land-Use Change 1990–2005. FAO Foestry Paper 169, ed E. J. Lindquist. Rome: Food and Agriculture Organization of the United Nations.
Mapulanga, A. M., and Naito, H. (2019). Effect of deforestation on access to clean drinking water. Proc. Natl. Acad. Sci. U.S.A. 116, 8249–8254. doi: 10.1073/pnas.1814970116
Measure DHS/ICF International (2012). Demographic and Health Surveys Methodology: Sampling and Household Listing Manual. Calverton: ICF International.
Oddo, V. M., Rah, J. H., Semba, R. D., Sun, K., Akhter, N., Sari, M., et al. (2012). Predictors of maternal and child double burden of malnutrition in rural Indonesia and Bangladesh. Am. J. Clin. Nutr. 95, 951–958. doi: 10.3945/ajcn.111.026070
Pienkowski, T., Dickens, B. L., Sun, H., and Carrasco, L. R. (2017). Empirical evidence of the public health benefits of tropical forest conservation in Cambodia: a generalised linear mixed-effects model analysis. Lancet Planet. Heal. 1, e180–e187. doi: 10.1016/S2542-5196(17)30081-5
Pienkowski, T., Dickens, B. L., Sun, H., and Carrasco, L. R. (2018). Linking forests, deforestation, and nutritional outcomes: an observational study in nine African countries. Lancet Planet. Heal. 2:S4. doi: 10.1016/S2542-5196(18)30089-5
Powell, B., Thilsted, S. H., Ickowitz, A., Termote, C., Sunderland, T., and Herforth, A. (2015). Improving diets with wild and cultivated biodiversity from across the landscape. Food Sec. 7, 535–554. doi: 10.1007/s12571-015-0466-5
Rasmussen, L. V., Watkins, C., and Agrawal, A. (2017). Forest contributions to livelihoods in changing agriculture-forest landscapes. For. Policy Econ. 84, 1–8. doi: 10.1016/j.forpol.2017.04.010
Rasolofoson, R. A., Nielsen, M. R., and Jones, J. P. G. (2018). The potential of the global person generated index for evaluating the perceived impacts of conservation interventions on subjective well-being. World Dev. 105, 107–118. doi: 10.1016/j.worlddev.2017.12.032
Reed, J., van Vianen, J., Foli, S., Clendenning, J., Yang, K., MacDonald, M., et al. (2017). Trees for life: the ecosystem service contribution of trees to food production and livelihoods in the tropics. For. Policy Econ. 84, 62–71. doi: 10.1016/j.forpol.2017.01.012
Roemling, C., and Qaim, M. (2013). Dual burden households and intra-household nutritional inequality in Indonesia. Econ. Hum. Biol. 11, 563–573. doi: 10.1016/j.ehb.2013.07.001
Rowland, D., Ickowitz, A., Powell, B., Nasi, R., and Sunderland, T. (2017). Forest foods and healthy diets: quantifying the contributions. Environ. Conserv. 44, 102–114. doi: 10.1017/S0376892916000151
Rutstein, S. O., and Johnson, K. (2004). The DHS Wealth Index. DHS comparative reports no. 6. Calverton, MD: ORC Macro.
Shrimpton, R., and Rokx, C. (2012). The Double Burden of Malnutrition: A Review of Global Evidence. Washington, DC: World Bank.
UNEP (2008).Africa: Atlas of our Changing Environment. Nairobi: Division of Early Warning and Assessment (DEWA) United Nations Environment Programme (UNEP).
Variyam, J. N., Blaylock, J., Ralston, K., and Smallwood, D. (1999). Mother' s nutrition knowledge and children' s dietary intakes. Am. Agric. Econ. Assoc. 81, 373–384. doi: 10.2307/1244588
Vereecken, C. A., Keukelier, E., and Maes, L. (2004). Influence of mother's educational level on food parenting practices and food habits of young children. Appetite 43, 93–103. doi: 10.1016/j.appet.2004.04.002
Vinceti, B., Termote, C., Ickowitz, A., Powell, B., Kehlenbeck, K., and Hunter, D. (2013). The contribution of forests and trees to sustainable diets. Sustainability 5, 4797–4824. doi: 10.3390/su5114797
Vira, B., Wildburger, C., and Mansourian, S. (2015). Forests, Trees and Landscapes for Food Security and Nutrition: A Global Assessment Report. Vienna: International Union of Forest Research Organizations (IUFRO).
Warren, J. L., Perez-Heydrich, C., Burgert, C. R., and Emch, M. E. (2016). Influence of demographic and health survey point displacements on distance-based analyses. Spat. Demogr. 4, 155–173. doi: 10.1007/s40980-015-0014-0
Wasserstein, R. L., Schirm, A. L., and Lazar, N. A. (2019) Moving to a World Beyond “p < 0.05”. Am. Stat. 73(Suppl.), 1–19. doi: 10.1080/00031305.2019.1583913
WHO/UNICEF Joint Monitoring Programme for Water Supply Sanitation (2012). Millennium Development Goals Indicators. Available online at: https://millenniumindicators.un.org/unsd/mdg/Metadata.aspx?IndicatorId=30 (accessed February 10, 2019).
Wojcicki, J. (2014). The double burden household in sub-Saharan Africa: maternal overweight and obesity and childhood undernutrition from the year 2000: results from World. BMC Public Health 14:1124. doi: 10.1186/1471-2458-14-1124
Zeba, A. N., Delisle, H. F., Renier, G., Savadogo, B., and Baya, B. (2012). The double burden of malnutrition and cardiometabolic risk widens the gender and socio-economic health gap: a study among adults in Burkina Faso (West Africa). Public Health Nutr. 15, 2210–2219. doi: 10.1017/S1368980012000729
Appendix
Keywords: deforestation, double burden, Sub-Saharan Africa, malnutrition, food systems
Citation: Acharya Y, Naz S, Galway LP and Jones AD (2020) Deforestation and Household- and Individual-Level Double Burden of Malnutrition in Sub-saharan Africa. Front. Sustain. Food Syst. 4:33. doi: 10.3389/fsufs.2020.00033
Received: 21 April 2019; Accepted: 06 March 2020;
Published: 03 April 2020.
Edited by:
Amy Ickowitz, Center for International Forestry Research (CIFOR), IndonesiaReviewed by:
Bhavani Shankar, SOAS University of London, United KingdomWanjiku N. Gichohi-Wainaina, International Crops Research Institute for the Semi-arid Tropics (ICRISAT), India
Copyright © 2020 Acharya, Naz, Galway and Jones. This is an open-access article distributed under the terms of the Creative Commons Attribution License (CC BY). The use, distribution or reproduction in other forums is permitted, provided the original author(s) and the copyright owner(s) are credited and that the original publication in this journal is cited, in accordance with accepted academic practice. No use, distribution or reproduction is permitted which does not comply with these terms.
*Correspondence: Yubraj Acharya, eXVhMzZAcHN1LmVkdQ==