- 1International Livestock Research Institute, Nairobi, Kenya
- 2Animal Production Systems Group, Wageningen University & Research, Wageningen, Netherlands
- 3Commonwealth Scientific and Industrial Research Organisation, St. Lucia, QLD, Australia
- 4World Agroforestry (ICRAF), Nairobi, Kenya
- 5Bioversity International, Turrialba, Costa Rica
- 6Independent Researcher and Consultant, Stockholm, Sweden
- 7World Agroforestry Centre (ICRAF), Kinshasa, Democratic Republic of Congo
- 8School of Natural Sciences, Bangor University, Bangor, United Kingdom
- 9Natural Resource Management Research Area, International Institute of Tropical Agriculture, Nairobi, Kenya
- 10International Institute of Tropical Agriculture, Bukavu, Democratic Republic of Congo
- 11TreeAID, Brunswick Court, Bristol, United Kingdom
- 12Plant Production Systems Group, Wageningen University & Research, Wageningen, Netherlands
- 13Sustainable Intensification Program, International Maize and Wheat Improvement Centre (CIMMYT), Texcoco, Mexico
Our understanding of food security in sub-Saharan Africa (SSA) has been hampered by limitations in the temporal and spatial representativeness of data. Food balance sheets provide scalable estimates of per capita food availability, but fail to represent food access, stability and their causal linkages. In contrast, rural household surveys represent detailed conditions for one or multiple points in time, but are influenced by survey timing and are often limited in geographical coverage. This study draws on a large sample of rural land-holding households in SSA (n = 6,353) to identify household level food access deficiencies and to understand the associations with rural livelihoods and food sourcing behavior throughout the year. Food access deficiencies were identified using food security of access and diet diversity indicators. Dietary diversity and channel of access (farm or purchased) were enumerated for the “flush” and “lean” periods and food security of access was enumerated for the lean period only - making the results of this study independent of survey timing. As many as 39% of households were classified as severely food insecure (in terms of food access) and as many as 49% of households were likely to be deficient in micronutrients in the lean period. Vulnerability to food insecurity and micronutrient deficiencies differed by household composition, agricultural livelihood characteristics and agro-ecological zone. Dairy, fruit and vitamin A-rich produce were predominantly accessed through the farm channel. Households with a livestock component to their farm had a lower prevalence of severe food insecurity and higher diet diversity scores. These findings have implications for the development of nutrition-sensitive and nutrition-specific interventions. Interventions need to be tailored to agro-ecological zone, household composition, scale of operation and production mix. Increasing income will not necessarily result in improved diet diversity or healthy dietary choices. Interventions focused on income generation should monitor and promote crop and livestock production diversity and provide nutrition education.
1. Introduction
Almost one in four people in sub-Saharan Africa (SSA) were estimated to be undernourished in 2017, representing about one-third of the 821 million people suffering from chronic hunger globally (FAO et al., 2018). In addition to a high prevalence of chronic hunger in SSA, many more people suffer from micronutrient deficiencies (Joy et al., 2014; Kumssa et al., 2015; Harika et al., 2017). These deficiencies increasingly co-exist with instances of obesity within the same communities—forming a triple burden on human health and society (May, 2018). Malnutrition is now the greatest risk factor driving a rising global noncommunicable disease burden (GBD Risk Factors Collaborators, 2017), having direct implications for child growth failure, neonatal disorders, immune function, cognitive function, diabetes, heart disease and cancer (Micha et al., 2015; Akombi et al., 2017; de Pee et al., 2017; Pisa et al., 2017; James, 2018; Prentice, 2018). These burdens will only intensify as rural and urban populations grow, diets change and rural employment opportunities stagnate (Popkin, 2014; FAO et al., 2018).
The majority of the food in SSA is produced by smallholder farmers (Herrero et al., 2017) while they are the most vulnerable to food insecurity and poverty (Sibhatu and Qaim, 2017; Fanzo, 2018). Hence, smallholder farmers are a crucial entry point for agricultural orientated interventions to improve food and nutrition security. A number of observational studies have assessed the linkages between agriculture and nutrition in the past 3 years (e.g., Gillespie et al., 2017; Fraval et al., 2018a; Ruel et al., 2018; Beveridge et al., 2019; Ritzema et al., 2019). Despite progress in the analysis of existing observational data and ex-post evaluation of nutrition-sensitive interventions, much remains to be understood with respect to the pathways to improved food and nutrition security of rural households (Carletto et al., 2017; Mary et al., 2018). This is partly due to the diversity of agricultural systems in SSA, where agro-ecological and market conditions drive their occurrence (e.g., Garrity et al., 2012). The amount and timing of rainfall determine which crops can be grown and when they can be harvested, thereby affecting the availability of food items throughout the year. The agro-ecological conditions also drive the occurrence of livestock systems, with (agro-)pastoral systems in dry areas, and mixed crop-livestock systems in higher rainfall zones.
An important question, not yet answered by the existing literature (e.g., Gillespie et al., 2017; Ruel et al., 2018) relates to how market participation mediates food and nutrition security in rural communities—especially access to and consumption of diverse diets. Farmers have two ways to obtain their food: (1) growing food crops and/or rearing livestock to consume the products, or (2) selling these products and use the income to buy food for their own consumption. Whether production-based or income-based channels are more important for food and nutrition security is a question without a straightforward answer. For example, increased incomes and food energy availability are necessary for alleviating undernourishment but may not be sufficient for addressing chronic or hidden hunger (Hoddinott, 2012; McDermott et al., 2015; Schipanski et al., 2016; Gödecke et al., 2018). A better understanding of these relationships is required for improved targeting, designing and implementing nutrition-sensitive interventions within agricultural production systems and value-chains.
Evaluating food and nutrition security (FNS) has traditionally been time-consuming and invasive. More recently, however, proxies have been introduced to enable wide-scale monitoring and evaluation. Food security of access scales and diet diversity scores have been favorably assessed as proxies for nutrient adequacy (Torheim et al., 2004; Steyn et al., 2006; Lachat et al., 2017), diet quality (Savy et al., 2005) and child growth/stunting (see Arimond and Ruel, 2004; Saha et al., 2009 and also Rah et al., 2010). The development of these time-efficient metrics has allowed for food and nutrition security indicators to be incorporated into large-scale, multi-purpose farm household surveys. These metrics have the benefit of being indicative of food access status, while minimizing the risk of respondent fatigue and allowing time for additional, complementary questions. For instance, given the limited understanding of the pathways to food and nutrition security a logical extension of diet diversity indicators is to ask for food sources. So far such questions have only been incorporated in a limited number of small scale studies.
However, complications arise when relating nutrition information and farm information: there is a systematic mismatch of time scales. Food and nutrition security is typically assessed over short time scales (e.g., 24 h or weekly recalls) whereas farm production and consumption of agricultural produce are typically estimated at annual or seasonal time scales (Herrero et al., 2007). Using the annual timescales commonly found in agricultural surveys can create problems because diets of rural households are often highly variable throughout the year (Sibhatu and Qaim, 2017). For instance, diets in the period after crop harvest are substantially different from the most difficult period of the year, generally the period just before harvest. This variability in diet means that survey timing can significantly influence the apparent consumption patterns enumerated in a nutrition survey. As such, relevant insights into typical and extreme dietary patterns within a household gained from typical nutrition surveys may be limited, and comparisons between households and locations can be biased—especially in larger studies that take months to complete.
This present study seeks to combine food security indicators with household-farm characteristics to estimate the prevalence of food access deficiencies and to understand their associations with rural livelihoods across SSA, throughout the year. To do this, we sampled rural households across SSA, allowing us to better represent the diversity of agricultural systems and dietary patterns in SSA. We enumerated where each food category was sourced from (own-farm/purchased), which allowed us to address the question of “how market participation mediates access to and consumption of diverse diets.” These FNS indicators were enumerated for specific periods of the year, with the aim of improving the comparability between households and across regions.
2. Methods
In this study, we utilize household interviews, geospatial data and mixed-effects regression analysis to achieve our research objectives.
2.1. Household Data
This study draws on responses from 7,708 rural land-holding households in SSA. Interviews were conducted through twelve projects operating across eight countries between 2016 and 2018 (Table SI 2 provides a summary of sample size by project and country). Households were sampled with multi-stage clustered sampling strategies, designed to be representative of rural land-holding households within administrative units at different scales (representativeness is addressed further in section 2.2). For the purpose of this study, we filtered the database based on data quality criteria, which resulted in 1,355 observations being removed—largely due to missing dietary diversity information (Table SI 3 summarizes thresholds for excluding observations). The coverage of sampled locations is presented in Figure 1.
The Rural Household Multi-Indicator Survey (RHoMIS) was utilized for data collection in all projects, eliciting information about the household composition, farm characteristics, food insecurity of access and diet diversity. The survey is designed to minimize the time burden on the interviewee, to maximize the reliability of responses, and to improve consistency between different studies (described further in Hammond et al., 2017).
We adapted existing food (in)security indicators for the purpose of this study. First, the household food insecurity of access prevalence (HFIAP) indicator is based on a series of nine questions of increasing severity, from worry about food availability to missing an entire day of food due to access issues (questions provided in Table SI 5; Coates and Bilinsky, 2007). The HFIAP indicator is used to categorize households into those that are food secure, mildly food insecure, moderately food insecure and severely food insecure—in terms of food access. Severe food insecurity (of access) is defined as regularly eating smaller meals than desired, regularly eating fewer meals than desired or worse. Our modified household food insecurity of access prevalence (MHFIAP) indicator recorded conditions in the worst month experienced (“lean” period) by the household during the previous 12 months [a modification of the 24-h recall period recommended in the Food And Nutrition Technical Assistance (FANTA) guidelines; Coates and Bilinsky, 2007]. Second, our modified household diet diversity access (MHDD) indicator was based on the minimum diet diversity for women (MDD-W) indicator, which is a count of 10 food categories (FAO and FHI360, 2016). The modification to the MDD-W indicator allowed households to recall the frequency of access for each food category in the best and worst month (referred to as “flush” and “lean” periods herein) rather than 24-h recall across multiple visits in a year. As an extension of diet diversity indicators, we also asked households to recall the channel of access for each food category—own-farm, purchased or free/traded. The MHDD indicator was used to assess risk of micronutrient deficiency (with a threshold of 5 food categories as recommended in FAO and FHI360, 2016) and as a count of categories. The modifications to the recall period were made so that indicator results would be independent of survey timing and provide greater temporal coverage, rather than providing a snapshot of 1 or 2 days. These two modified indicators are referred to collectively as indicators of “food access deficiencies” herein.
Our analysis also incorporated demographic, agro-ecological, farm, and economic metrics. Adult equivalents were calculated as the ratio of energy requirements for an age and gender class relative to the average energy requirement of adult males and females between 25 and 50 (2,500 kcal; following Claro Rafael et al., 2010 and using energy requirements from FAO, 2001). Agro-ecological zone (AEZ) was extracted from the Harvest Choice (2015) AEZ-16 spatial layer based on global-positioning system (GPS) points. Market participation was calculated as the proportion of crop and livestock calories sold. Tropical Livestock Units (TLUs) were calculated using conversion factors provided by Njuki et al. (2011). The Progress out of Poverty Index (PPI) was calculated using 10 questions on household characteristics (e.g., housing structure, asset ownership, school attendance) that are correlated with country specific poverty levels (Hammond et al., 2017). Income was calculated based on responses to questions about farm sales and off-farm income. To compare income across countries, we express these values in dollars, converted by purchasing power parity (PPP). Purchasing power parity was determined using the World Bank database (World Bank, 2018) and exchange rates were taken from the time of survey execution.
2.2. Associations Between Food Security Indicators and Livelihood Characteristics
The prevalence of food insecurity indicators were weighted based on population estimates of the administrative unit that the households were sampled to represent. Population estimates of the year 2015 were extracted from the “gridded population of the world” dataset (version 4; CIESIN, 2017)—masking out densely populated areas (>1,000 people per km2). Observations were then weighted based on the average population density in the administrative unit (persons per km2), relative to the average population density of other administrative units—such that each household within an administrative boundary was weighted equally and relative to the population density of other administrative units. The weights of studies that only sampled livestock keeping households were adjusted, assuming that they represent 50% of the rural population. The sum of weights equated to the sample size.
We modeled associations between food security of access and diet diversity as dependent variables, and household composition, farm production mix, market participation and income as independent variables. Agroecological zone (AEZ) was also included as a fixed effect in the regression models to control for differences between semi-arid locations (Length of growing period; LGP 75–180 days) and humid/sub-humid locations (LGP > 181 days). We incorporated varying effects on the intercept from villages nested within projects in our models. We used logistic regressions with mixed-effects to model whether households were severely food insecure (of access) or not and negative binomial regressions with mixed-effects to model overdispersed count variables (MHDD).
To further explore modeled associations, we developed a farm typology based on the composition of farm production—which influences both cash availability and diversity of own-farm food availability (Jones, 2016; Headey et al., 2018). Farms were classified as “specialized cropping” if they reported two or fewer crop species as being important for their livelihood or sourced two or fewer plant-based food categories (e.g., “legumes” or “leafy vegetables”) from their farm daily; farms with three or more crop species/plant-based categories were classified as having “diverse cultivation.” Livestock holdings (animals under the care of the household) were represented as Tropical Livestock Units (TLU), and households with over 1.5 TLUs (the equivalent of one head of cattle—1 TLU, one sow—0.3 TLU and five chickens—0.04 TLU) were categorized as having a livestock product (meat, milk or eggs) component to their farm, in combination with their cropping activities. This threshold was set marginally higher than one TLU to reduce instances of false positive classifications of households keeping an ox or donkey (0.8 TLU) for draft power purposes. We identified four distinct farm types: “Specialized cropping,” “Diverse cropping,” “Specialized cropping and livestock,” and “Diverse cropping and livestock” (spatial distribution in Table SI 4). We use this farm typology to characterize channels of food access and to explore the prevalence of food insecurity.
Differences between farm types were modeled using mixed-effects linear and logistic regressions, with varying effects on the intercept from villages nested within projects. Dependent variables of these models included farm-household characteristics, consumption behavior and food security indicators. The independent variable in these models was either farm type or an aggregation of farm types (e.g., livestock keeping). All regression models were estimated using a hybrid Monte-Carlo Markov Chain (MCMC) method, implemented in R using the BRMS package (v 1.0.1; Buerkner, 2016). Weakly informative priors (Student's t-distribution with df = 5) were used, allowing extreme values but maintaining an expectation of minimal association (i.e., central tendency near zero). The posterior distributions were analyzed and if 95% of the density was above or below zero then it was considered to be statistically significant. Models were based on a core set of household and farm variables, with interactions tested additively. The core set of variables incorporated key household and farm variables as well as variables associated both with the dependent and independent variable (such as AEZ). All regressions were weighted by population density—minimizing bias in generalizations to the sampled locations.
3. Results
3.1. Prevalence of Severe Food Insecurity of Access and Dietary Diversity
Severe food insecurity (of access) was widespread across the surveyed households, with as many as 39% of households classified as severely food insecure in the lean period—according to the MHFIAP indicator (Table 1). In the lean period, 53% of households had less than five diet diversity categories, in contrast to 21% of households in the flush period.
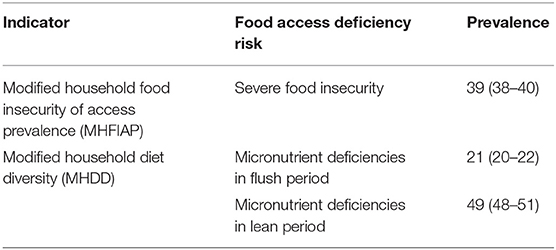
Table 1. Prevalence of severe food insecurity (MHFIAP) and below household diet diversity (MHDD) threshold, weighted by population (n = 6,353; % of weighted sample; 95% CI in parentheses).
There are several factors associated with the MHFIAP and MHDD indicators (Table 2). Regression results show that the number of household inhabitants was positively associated with diet diversity in the flush and lean periods. Female headed households were negatively associated with food security of access in the lean period. Having children under the age of 10 in the household was also negatively associated with food security of access and diet diversity in the lean period. These results suggest that there are household compositions with greater vulnerability to food access conditions that could lead to chronic and hidden hunger.
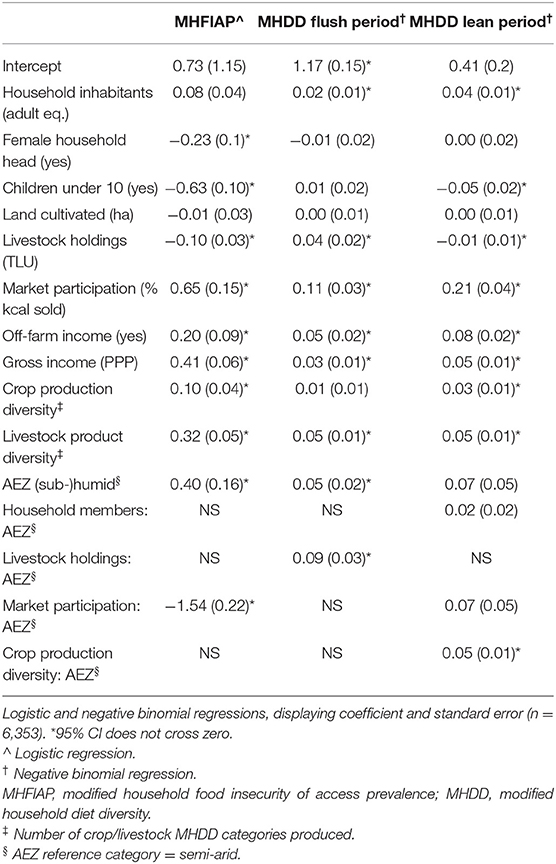
Table 2. Associations between food security indicators (dependent variables in columns) and livelihood characteristics and agro-ecological zone (AEZ).
Livestock holdings were negatively associated with food security of access and diet diversity in the lean period. However, the association with diet diversity in the lean period was the weakest of all significant coefficients (Table 2). Livestock holdings were positively associated with diet diversity in the flush period (Figure 2).
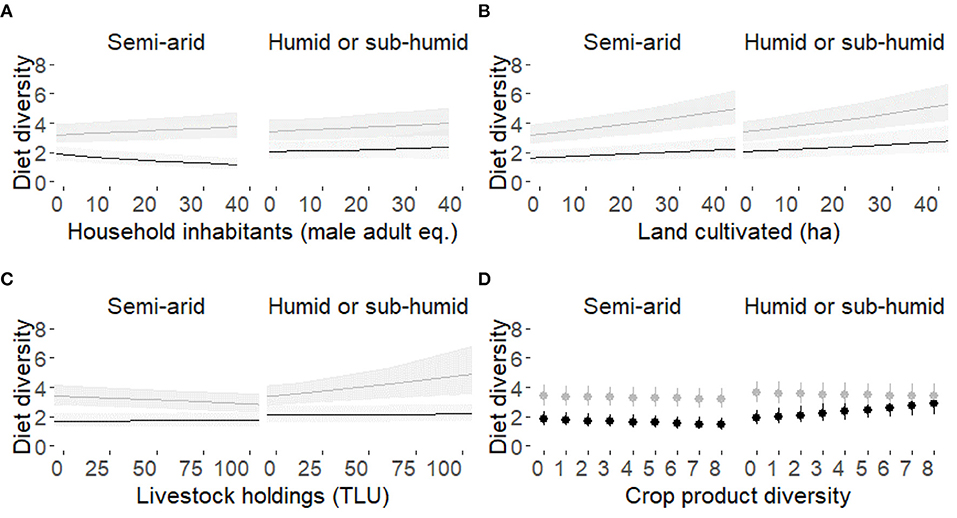
Figure 2. Predicted associations between MHDD (count of food categories) and (A) household inhabitants (adult eq), (B) land cultivated, (C) livestock holdings (Tropical Livestock Units), (D) crop product diversity in the flush (gray line/point) and lean (black line/point) periods by agro-ecological zone.
Market participation was positively associated with food security of access in the lean period and diet diversity in both the flush and lean periods. Off-farm income was positively associated with food security of access in the lean period and diet diversity in both periods. Gross income was positively associated with food security of access in the lean period and diet diversity in the flush and lean periods.
Crop production diversity was positively associated with food security of access in semi-arid zones and diet diversity in the flush and lean periods. In humid/sub-humid zones, crop production diversity was negatively associated with food security of access. The diversity of livestock products produced by a household was positively associated with food security of access and diet diversity in the lean period.
These results suggest that associations between diets and farm-household characteristics differ between AEZs. These differences between AEZs were most substantial for market participation and crop production diversity (Figure 2; additional marginal effects presented in Figure SI 1).
3.2. Channel of Food Access by Farm Type
Households with a livestock component tended to have more people in the household, larger land area cultivated, better “progress out of poverty” scores and—naturally—more livestock (mixed-effects linear regressions; 95% CI >0; Table SI 6). “Diverse cropping” and “Diverse cropping and livestock” households tended to have greater market participation than their specialized counterparts (Table SI 8). Farm types with a livestock component had significantly higher MHDD scores in the flush and lean periods compared to other farm types (Table 3, Table SI 6). As expected, all farm types had higher median MHDD scores in the flush period, compared to the lean period.
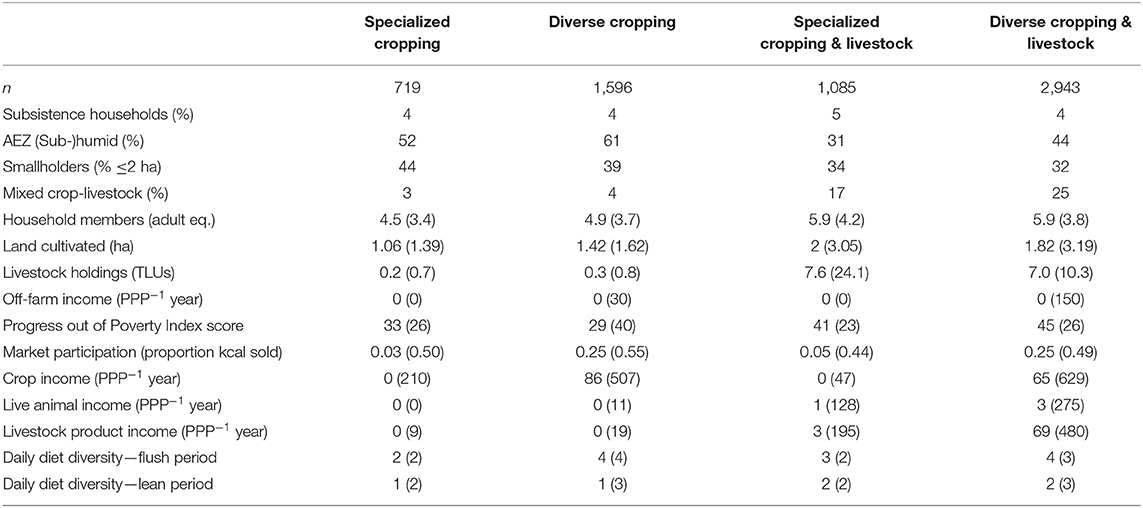
Table 3. Summary of resources, income, and diet diversity of sampled households by farm type (number, proportion and median-IQR).
The channel of accessing MHDD food categories differed by farm type. In Figure 3, the diversity of food acquired through two channels of access—own-farm sourced and purchased—and total diet diversity for the four farm types are presented for the lean period (equivalent results for the flush period are presented in Figure SI 2).
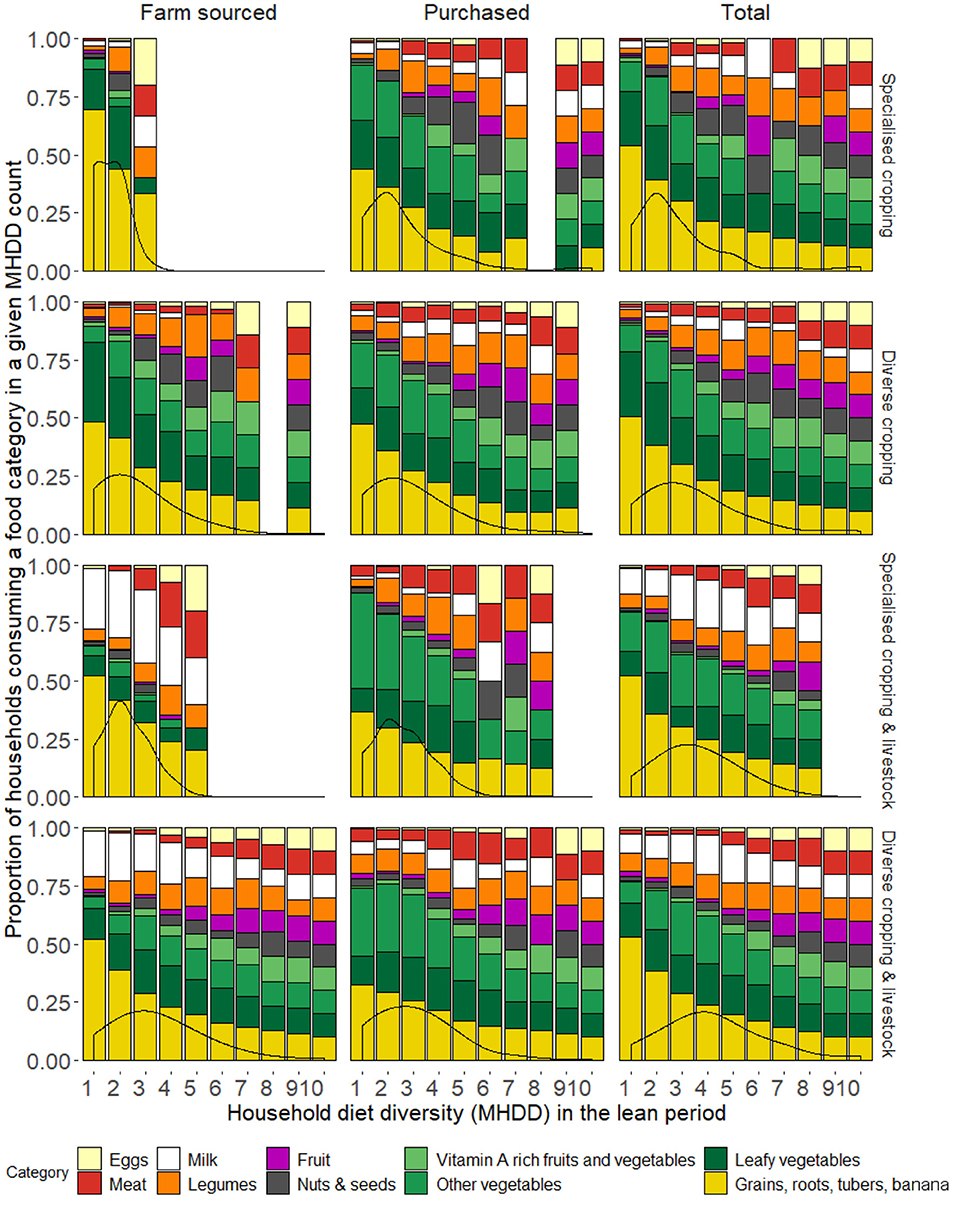
Figure 3. Diet diversity: density and proportion of sampled households (n = 6,353) consuming specific food categories by farm type, channel of access and total diet diversity in the lean period. The distribution (probability density function) of diet diversity for each period is represented as a black line on the lower half of each figure facet. Food categories are represented by different colors, showing the proportion of households consuming each category at specific diet diversity levels.
In Figure 3, the probability density function of the count of household diet diversity (MHDD) categories is presented as a black line on the lower half of each facet. The line indicates the probability that a household of a specific farm type consumes a given number of food categories (1-10). Each facet also presents the proportion of households that consume certain categories for a given count of MHDD. For example, in the top right-hand facet showing “Specialized cropping” and “total,” the second pillar shows households with only two MHDD food categories, where the most common daily sourced food category was “grains, roots, tubers, and plantains,” followed by “leafy vegetables,” “other vegetables,” and then “legumes.” In contrast, since the far-right pillar shows households which consume all 10 food categories, the proportions of food categories consumed are equal. Figure 3 allows comparison between farm types for a specific channel and within farm types, between channels. Inference from this figure is focused on the central mass of the probability density function—generally between 1 and 5 MHDD categories.
At the aggregate level, farm types with a substantial livestock component consume more livestock products—milk, meat and eggs—in both flush and lean periods (Table SI 7). In the lean period, 50% of households with a livestock component to their farm consumed dairy products compared to <17% of crop-oriented households. In Figure 3, it is evident that dairy products are predominantly accessed through the farm channel and are sometimes the only daily sourced food category. Meat consumption—a source of vitamin B12 among several other micronutrients—in the lean period was marginally higher in households with a livestock component (38% compared to 28% of crop-based households). Meat was most often purchased, particularly in the flush period—irrespective of farm type. Daily egg consumption—also a source of vitamin B12 and riboflavin—was less common, but consistently higher in households with a livestock component (results not tabulated elsewhere).
A greater proportion of households with a diverse cropping component to their farm tended to source plant-based food categories in the flush period—except “nuts and seeds” and “grains, roots, tubers, and banana” (Table SI 8). In the lean period, when compared to other farm types, a smaller proportion of households in the “Specialized cropping” farm type sourced “legumes,” “fruit,” “vitamin A rich fruits and vegetables,” and “other vegetables” (Table SI 8).
At the minimum diet diversity threshold (five food categories), there were commonalities and divergences in the food categories that were lacking. Households at risk of micronutrient deficiencies tended not to consume eggs, fruits and vitamin A rich produce in the lean period— this was common across farm types. Farm types without a livestock component to their farm tended to lack dairy products in the lean period when below the threshold, while their livestock keeping counterparts did access dairy products below the threshold (Table SI 9). These findings suggest that the specific micronutrients lacking when below the minimum diet diversity threshold differ between households and are determined by farm type.
The prevalence of severe food insecurity (of access) was not independent of farm type in both humid/sub-humid and semi-arid agro-ecological zones. Specialized cropping households tended to have a higher prevalence of severe food insecurity—when compared with all other farm types (Table 4). In humid/sub-humid AEZs, “Diverse cropping & livestock” households had a lower prevalence of severe food insecurity and a lower prevalence of substandard (below five food categories) diet diversity (in the flush and lean periods) when compared to other farm types (Table SI 10; Figure 4). In semi-arid zones, households with a livestock component to their farm had a significantly lower prevalence of severe food insecurity and a lower prevalence of substandard diet diversity in the lean period (Table SI 11). In semi-arid zones, households with a diverse cropping component to their farm had a lower prevalence of substandard diet diversity in the flush period (Table SI 11).
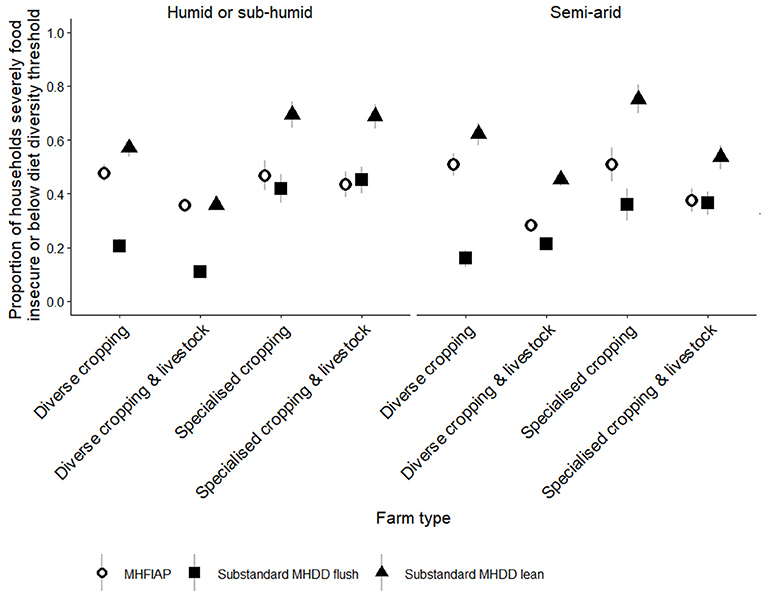
Figure 4. Proportion of households (n = 6,353 weighted by population) with insecure access to food (MHFIAP) or substandard household diet diversity (MHDD) by farm type and agro-ecological zone. 95% confidence intervals are represented by gray vertical lines.
4. Discussion
4.1. Food Insecurity Prevalence
The aim of this study was to combine food security indicators with household-farm characteristics to estimate prevalence of food access deficiencies and to understand their associations with rural livelihoods and food sourcing behavior throughout the year. An estimated 39% of sampled households were categorized as being severely food insecure in terms of access; this is higher than reported by the FAO using the Food Insecurity Experience Scale (FIES; Table SI 5 provides a comparison of the questions asked)—with an estimated 34% of the SSA population being severely food insecure in 2017 (FAO et al., 2018). An estimated 49% of households had substandard diet diversity in the lean period—indicating risk of micronutrient deficiencies. There is no direct comparison to our estimate of micronutrient deficiency risk based on the minimum diet diversity threshold. Micronutrient deficiencies, however, have been estimated to be between 1 and 54% for SSA in Joy et al. (2014) and between 26 and 93% of sampled women in Burkina Faso, Mali, and Uganda (Martin-Prével et al., 2015; summarized in Table SI 6).
4.2. Contextual Factors for Targeting Nutrition-Sensitive Interventions
There are several factors associated with food access deficiencies. From the perspective of designing nutrition-sensitive agricultural interventions, we discuss (a) contextual factors that can improve the targeting of interventions and (b) factors that can form the basis of an intervention or be incorporated as a complementary intervention.
The contextual factors identified in this study are either not possible to change through agricultural interventions (AEZ and household composition), or relate to complex farmer/household decisions that are beyond the scope of current public and civil society interventions in SSA (farm scale and farm type). These contextual factors can be used as inputs into the intervention targeting decision-making process, where the aim is to maximize the potential for practice adoption and impact on well-being. On the basis of our results, we expect households with “female heads” and children under 10 years of age to have a higher likelihood of severe food insecurity in the lean period—supporting the need for programmatic strategies on gender inclusion (Mason et al., 2015; Tavenner et al., 2019), as well as focusing on the first 1,000 days of life (de Pee et al., 2017). Similarly, households with small land cultivation areas can be targeted to counteract the tendency of limited access to micronutrient sources (land size has been linked to both chronic and hidden hunger; Gödecke et al., 2018).
Understanding the existing production systems (farm types) of rural households within and between locations can inform more advanced intervention targeting decisions (Bosire et al., 2019; Steinke et al., 2019). For example, an intervening agent (e.g., government, NGO, etc.) may initially target their interventions based on AEZ, farm scale and sub-sector (e.g., dairy), and then in the advanced stages, design and target interventions based on the prevailing farm types. This approach can improve the suitability of interventions to existing conditions (i.e., by enhancement, diversification, or substitution; Fiorella et al., 2016) and therefore maximizes adoption potential. This contextual factor also provides opportunities to improve the nutrition sensitivity of interventions. Our results show that prevalence rates differ by farm type and AEZ (Figure 4). Intervention packages can be developed to improve dietary outcomes, targeting specific farm types in specific AEZs (Hoddinott, 2012; Hetherington et al., 2017; Mulmi et al., 2017). This is also reinforced by Carletto et al. (2015), who review a series of studies showing that a household's agricultural production can directly influence the dietary patterns of household members, where the extent of impact depends on a variety of factors including location, commodities produced, and whether a household keeps livestock for direct consumption.
4.3. Designing Nutrition-Sensitive Interventions
There are three factors identified in this study that could be incorporated in nutrition-sensitive agricultural orientated interventions. Increasing farm income (in-part through increasing market participation; Ogutu et al., 2019), improving off-farm employment opportunities (including along the agricultural value-chain; Davis et al., 2017) and diversifying production (crops, home garden and livestock products). Each of these factors had positive effects on food security of access and dietary diversity. These results are in line with the overview presented by Ruel et al. (2018, p. 147), stating that “production diversity and livestock ownership are consistently associated with household and dietary diversity and, when measured, with increased intake of essential micronutrients.” There are, however, instances of negative associations with these variables, such as livestock holdings and diet diversity in the lean period. Also, even extreme increases in income or production diversity (i.e., from the lowest end of the scale to the highest), are only predicted to increase diet diversity in the lean period by 1 or 2 food categories (Figure 2). This limitation needs to be taken into account when designing and monitoring nutrition-sensitive interventions. Success may not be measured in leaps in diet diversity, but rather by adding specific food categories (in sufficient frequency and quantity) that provide micronutrient sources that would otherwise be lacking.
4.4. How Do Farm Types Differ in Food Sourcing Behavior?
Our results clearly demonstrate that sourcing of food categories was strongly related to farm type (Figure 3). We found that in addition to purchased food, the farm-based route to dietary diversity was extremely important: consumption of specific food groups is strongly linked to what farm households produce on-farm. This is a key finding because a substantial proportion of households that lacked an aspect of production diversity—and thereby miss certain food categories in the farm-based route to dietary diversity—did not choose to supplement their lack of production diversity with an equivalent diversity of purchased food categories. Households with a livestock component to their farm consumed more livestock products—in-part due to their own-farm availability (also identified by Hetherington et al., 2017). Dairy, for example, was predominantly sourced from the farm in the lean period, where 50% of households with a livestock component to their farm consumed dairy products compared to <17% of crop-oriented households. Similarly, households with a diverse cropping component to their farm sourced a greater diversity of fruit and vegetable food categories (legumes, fruit, vitamin A rich produce, and other vegetables)—mainly from the farm.
The finding that only a subset of households supplement gaps in production diversity with an equivalent diversity of purchased food categories indicates that extra income does not necessarily translate into food and nutrition security—particularly for hidden hunger. Rather, dietary choices are—in part—driven by the retail environment, resulting in increased consumption of processed and sweetened foods (categorized as “grains, roots, tubers, and banana”). This in turn increases the risk of obesity and a higher burden of disease (Popkin, 2014; GBD Risk Factors Collaborators, 2017; Demmler et al., 2018). Therefore, interventions aiming at improving dietary diversity through increasing incomes need to accompany their interventions with nutritional education to stimulate more diverse and nutritious purchases (Dhanarajan, 2017), or by stimulating production to improve local availability (globally, we lack sufficient fruit and vegetables for 22% of the human population; Siegel et al., 2014). Otherwise, our results suggest that income-based interventions are unlikely to be nutrition-sensitive.
4.5. Policy Implications
Recent work has suggested that global chronic hunger can be brought to an end by 2030 with an additional annual investment of $11 bn (Fan et al., 2018). The potential benefits of such an investment would be immense–evidenced by the high prevalence of severe food insecurity presented in Table 1. This potential can only be realized with highly targeted and well-designed interventions. Our results suggest that targeting can be based upon contextual factors, such as agro-ecological potential, market conditions, land size, farm type and household composition. In this way, the most vulnerable segments of society can be prioritized, and differing intervention needs can be identified. Our results also identify three priorities for designing nutrition-sensitive agricultural interventions, namely: increasing farm income, improving off-farm income opportunities and diversifying production. Our results indicate that households with less diverse food production may not compensate for this by purchasing diverse food baskets, thereby achieving poorer nutritional outcomes than the more diversified producers. Therefore, income-oriented interventions might need to be coupled with nutrition education to be effective. Nutrition education delivered alongside agricultural extension in Malawi and Zambia, for example, has been found to significantly improve household food consumption practices (Heumesser and Kray, 2019).
The two goals of increasing farm income as well as improving access to diverse food categories can be at odds, where the former is driven by specialization and the latter by diversification. So how can policy support both specialization and diversification with limited budgets? Heumesser and Kray (2019) identify three pathways from specialization/diversification to improved livelihoods, resilience and nutrition: the ecosystem pathway, the income pathway, and the food environment pathway. With this lens and with our analytical approach, the implications of promoting specialization or diversification can be evaluated for a given context. For example, investments in agricultural research and development can focus on non-staple crops for locations with limited chronic hunger, limited access to diverse foods and increasing soil acidification from high input, specialized production systems. On the other hand, it might be effective to concentrate on specific market-oriented products where both output and food markets are easily accessible and production systems are already diverse. Similarly, agricultural advisory services may target farm households more specifically, considering contextual factors, such as land size and farm type—recommending greater specialization or diversification to different households within the one community. By doing so, policy could more effectively and sustainably improve rural livelihoods, resilience and human nutrition.
4.6. Methodological Considerations
In this study we identified important associations between agricultural activities and pathways toward food security of access and diet diversity. These results, however, are limited by the means of data collection, the approximations made, the limited scope of analysis and the indicators used.
We used information collected in one-off (i.e., cross-sectional) household surveys, which are prone to non-credible values and reduced measurement precision (Fraval et al., 2018b). In total 1,355 observations (20%) were removed from the original dataset—largely due to missing MHDD data, as well as non-credible household or farm characteristics, and enumerator evaluated quality (Table SI 3). The sampled households were more likely to be in lower socio-economic regions of rural SSA and so can not be taken to represent rural SSA as a whole. Population weightings were based on modeled data, derived from national statistics with variable quality.
The standard recall procedures of our food security indicators were adapted to enumerate both the lean and flush periods—allowing us to capture the substantial variation of farm household diets throughout the year. This adaptation on the recall procedure, however, means that respondents may need to remember a set of circumstances from up to 11 months prior in order to answer a question—potentially having a negative impact on measurement precision (Beegle et al., 2012).
Limiting our scope to the household level automatically limited the depth of the analysis. This has consequences for the interpretation of results. For example, it is not always clear whether we can translate household level findings to individual members of the family, for example: young children (Caraher, 2016).
In this study, we assessed a limited set of indicators and associations. Although agriculture is a crucial determinant of food and nutrition security in landholding households, it is important to understand interactions with factors like education, gender, food preparation, and sanitation to understand the full nutritional, and health consequences of our findings (in contrast, Gödecke et al., 2018 and Ramankutty et al., 2018 do show associations with education and sanitation and Dzanku, 2019 shows associations with gender). A substantial number of studies have reported the indicativeness of food security of access and a diverse diet on intermediary nutrition outcomes, such and child and maternal intake of “target” foods and micronutrients. Evidence of impact on nutrition outcomes—particularly child anthropometry and micronutrient status—was much more limited (Gillespie et al., 2017), stressing the limitations of using indicators like dietary diversity when inferring nutritional consequences (e.g., Hetherington et al., 2017 showed weak and inconsistent associations between animal-source food consumption and anthropometric measurements).
5. Conclusions
In this study we have quantitatively linked food security indicators to household and farming system characteristics across a wide range of production systems in sub-Saharan Africa. A high proportion of rural land-holding households were classified as being severely food insecure or having substandard diet diversity. The factors associated with these food access deficiencies can be used to improve the targeting of interventions or can be used to inform the design of interventions. In our assessment of food sourcing behavior, we found that farm-based food availability is an important route for specific nutrient dense food categories. This food sourcing behavior indicates that higher incomes do not necessarily result in improved food and nutrition security outcomes. Rather, for income enhancing interventions to be “nutrition sensitive,” greater attention on nutrition education and maintaining production diversity will be required.
Data Availability Statement
Data is available on the Harvard dataverse: https://dataverse.harvard.edu/dataset.xhtml?persistentId=doi:10.7910/DVN/9M6EHS.
Ethics Statement
The studies involving human participants were reviewed and approved by the Internal Review Ethics Committee (IREC) of the International Livestock Research Institute. The implementing partner research organizations Bioversity International, World Agroforestry Centre (ICRAF), and Tree AID did not have internal ethical committees but were approved internally by senior management at each organization after careful evaluation of the content, methodology, and oral informed consent statement built-in to the survey. Survey participants were not particularly vulnerable, data was processed in anonymized form, and survey participants had the possibility to skip questions. Explicit oral informed consent was obtained from all survey participants prior to survey enumeration and documented as the opening question in the RHoMIS survey upon informing survey participants of the study's purpose. If consent was denied, survey enumeration was terminated. Permission for obtaining oral rather than written consent from survey respondents was granted by the Internal Review Ethics Committee (IREC) of the International Livestock Research Institute, implementing research organizations and local agricultural officers, given literacy limitations among the target population.Additional research permissions were granted in Tanzania through the Tanzania Commission for Science and Technology (COSTECH) and from the District Agricultural, Irrigation and Cooperative Officers (DAICOs) in all administrative districts included in the survey. In Ethiopia, following the ABS proclamation 482–2006 of the Ethiopian government, farmers were interviewed after getting the necessary permission from local agricultural office administrators. In Kenya, research permissions were granted through the County Minister for Agriculture in each site and the local administration (chief, assistant chiefs, and village elders), who approved the survey dates, areas the survey would be implemented, and the methods used. Written informed consent for participation was not required for this study in accordance with the national legislation and the institutional requirements.
Author Contributions
SF and MW led the conceptual development. SF led the statistical analysis and writing process. JH and MW developed the survey tool. JB and MN provided expert input on the human nutrition elements of the study. MW, JH, SO, IB, ML, NT, JE, MH, JW, CL, TR, TP, BV, PD-N, DB, AA, and PC provided extensive comments on the manuscript. NT led the I4IGalvmed Tanzania data collection. TR led the FORETS DRC data collection. SC and PN led the SCAN Zambia data collection. JE and JS led the SAIRLA data collection. DB, PC, and TS coordinated the TreeAID data collection. JW led the CCAFS Rakai data collection. NT and EK led the CCAFS Kenya data collection. CS led the CCAFS Malawi data collection. BV, PD-N, and CO led the CLiP project. AA and VY led the SIIL Mali and Burkina Faso data collection. CB and ER led the AVCD Kenya data collection.
Conflict of Interest
The authors declare that the research was conducted in the absence of any commercial or financial relationships that could be construed as a potential conflict of interest.
Acknowledgments
This research was made possible by the livestock CGIAR research project (CRP), its donors and the organizations listed in Table SI 1. We would like to extend our gratitude to the rural households that participated in this research and the enumerators who guided the conversations. We also thank the two reviewers and the editor, Ademola Braimoh, for their time and comments.
Supplementary Material
The Supplementary Material for this article can be found online at: https://www.frontiersin.org/articles/10.3389/fsufs.2019.00104/full#supplementary-material
References
Akombi, B. J., Agho, K. E., Hall, J. J., Wali, N., Renzaho, A. M. N., and Merom, D. (2017). Stunting, wasting and underweight in sub-Saharan Africa: a systematic review. Int. J. Environ. Res. Public Health 14, 1–18. doi: 10.3390/ijerph14080863
Arimond, M., and Ruel, M. T. (2004). Community and international nutrition dietary diversity is associated with child nutritional status: evidence from 11 demographic and health surveys. J. Nutr. 134, 2579–2585. doi: 10.1093/jn/134.10.2579
Beegle, K., Carletto, C., and Himelein, K. (2012). Reliability of recall in agricultural data. J. Dev. Econ. 98, 34–41. doi: 10.1016/j.jdeveco.2011.09.005
Beveridge, L., Whitfield, S., Fraval, S., van Wijk, M., van Etten, J., Mercado, L., et al. (2019). Experiences and drivers of food insecurity in Guatemala's dry corridor: insights from the integration of ethnographic and household survey data. Front. Sustain. Food Syst. 3:65. doi: 10.3389/fsufs.2019.00065
Bosire, C. K., Rao, J., Muchenje, V., Wijk, M. V., Ogutu, J. O., Mekonnen, M. M., et al. (2019). Agriculture, ecosystems and environment adaptation opportunities for smallholder dairy farmers facing resource scarcity: integrated livestock, water and land management. Agric. Ecosyst. Environ. 284:106592. doi: 10.1016/j.agee.2019.106592
Carletto, C., Corral, P., and Guelfi, A. (2017). Agricultural commercialization and nutrition revisited: empirical evidence from three African countries. Food Policy 67, 106–118. doi: 10.1016/j.foodpol.2016.09.020
Carletto, G., Ruel, M., Winters, P., and Zezza, A. (2015). Farm-level pathways to improved nutritional status: introduction to the special issue. J. Dev. Stud. 51, 945–957. doi: 10.1080/00220388.2015.1018908
Claro Rafael, M., Levy, R. B., Bandoni, D. H., and Mondini, L. (2010). Per capita versus adult-equivalent estimates of calorie availability in household budget surveys. Cad Saude Publica 26, 2188–2195. doi: 10.1590/S0102-311X2010001100020
Coates, J., and Bilinsky, P. (2007). Household Food Insecurity Access Scale (HFIAS) for Measurement of Food Access: Indicator Guide. Technical report, Washington, DC: FHI 360/FANTA.
Davis, B., Di Giuseppe, S., and Zezza, A. (2017). Are African households (not) leaving agriculture? Patterns of households' income sources in rural Sub-Saharan Africa. Food Policy 67, 153–174. doi: 10.1016/j.foodpol.2016.09.018
de Pee, S., Taren, D., and Bloem, M. W. editors (2017). Nutrition and Health in Developing World. 3rd Edn. New York, NY: Springer Nature.
Demmler, K. M., Ecker, O., and Qaim, M. (2018). Supermarket shopping and nutritional outcomes: a panel data analysis for Urban Kenya. World Dev. 102, 292–303. doi: 10.1016/j.worlddev.2017.07.018
Dzanku, F. M. (2019). Food security in rural sub-Saharan Africa: exploring the nexus between gender, geography and off-farm employment. World Dev. 113, 26–43. doi: 10.1016/j.worlddev.2018.08.017
Fan, S., Headey, D., Laborde, D., Croz, D. M.-D., Rue, C., Sulser, T. B., et al. (2018). Quantifying the cost and benefits of ending hunger and undernutrition: examining the differences among alternative approaches. Technical Report February, Washington, DC: IFPRI.
Fanzo, J. (2018). The role of farming and rural development as central to our diets. Physiol. Behav. 193, 291–297. doi: 10.1016/j.physbeh.2018.05.014
FAO (2001). Human Energy Requirements: Report of a Joint FAO/WHO/UNU Expert Consultation. Technical report, FAO, Rome.
FAO IFAD, UNICEF, WFP, and WHO. (2018). The State of Food Security and Nutrition in the World 2018. Building Climate Resilience for Food Security and Nutrition. Technical report, FAO, Rome.
Fiorella, K. J., Chen, R. L., Milner, E. M., and Fernald, L. C. H. (2016). Agricultural interventions for improved nutrition: a review of livelihood and environmental dimensions. Glob. Food Security 8, 39–47. doi: 10.1016/j.gfs.2016.03.003
Fraval, S., Hammond, J., Lannerstad, M., Oosting, S. J., Sayula, G., Teufel, N., et al. (2018a). Livelihoods and food security in an urban linked, high potential region of Tanzania: changes over a three year period. Agric. Syst. 160, 87–95. doi: 10.1016/j.agsy.2017.10.013
Fraval, S., Hammond, J., Wichern, J., Oosting, S. J., de Boer, I. J. M., Teufel, N., et al. (2018b). Making the most of imperfect data: a critical evaluation of standard information collected in farm household surveys. Exp. Agric. 55, 230–250. doi: 10.1017/S0014479718000388
Garrity, D., Dixon, J., and Boffa, J. (2012). Understanding African Farming Systems: Science and Policy Implications. Technical report. Canberra, ACT: ACIAR.
GBD 2016 Risk Factors Collaborators (2017). Global, regional, and national comparative risk assessment of 84 behavioural, environmental and occupational, and metabolic risks or clusters of risks, 1990–2016: a systematic analysis for the Global Burden of Disease Study 2016. Lancet 390, 1345–1422. doi: 10.1016/S0140-6736(17)32366-8
Gillespie, S., Bold, M. V. D., and Team, S. (2017). Stories of change in nutrition: an overview. Glob. Food Security 13, 1–11. doi: 10.1016/j.gfs.2017.02.004
Gödecke, T., Stein, A. J., and Qaim, M. (2018). The global burden of chronic and hidden hunger: trends and determinants. Glob. Food Security 17, 21–29. doi: 10.1016/j.gfs.2018.03.004
Hammond, J., Fraval, S., van Etten, J., Suchini, J. G., Mercado, L., Pagella, T., et al. (2017). The Rural Household Multi-Indicator Survey (RHoMIS) for rapid characterisation of households to inform climate smart agriculture interventions: description and applications in East Africa and Central America. Agric. Syst. 151, 225–233. doi: 10.1016/j.agsy.2016.05.003
Harika, R., Faber, M., Samuel, F., Mulugeta, A., Kimiywe, J., and Eilander, A. (2017). Are low intakes and deficiencies in iron, vitamin A, zinc, and iodine of public health concern in Ethiopian, Kenyan, Nigerian, and South African children and adolescents? Food Nutr. Bull. 38, 405–427. doi: 10.1177/0379572117715818
Harvest Choice (2015). AEZ (16-Class, 2009). Technical report, Washington, DC; St. Paul, MN: International Food Policy Research Institute; University of Minnesota.
Headey, D., Hirvonen, K., and Hoddinott, J. (2018). Animal sourced foods and child stunting. Am. J. Agric. Econ. 100, 1302–1319. doi: 10.1093/ajae/aay053
Herrero, M., González-Estrada, E., Thornton, P. K., Quirós, C., Waithaka, M. M., Ruiz, R., et al. (2007). IMPACT: generic household-level databases and diagnostics tools for integrated crop-livestock systems analysis. Agric. Syst. 92, 240–265. doi: 10.1016/j.agsy.2006.03.008
Herrero, M., Thornton, P. K., Power, B., Bogard, J. R., Remans, R., Fritz, S., et al. (2017). Farming and the geography of nutrient production for human use: a transdisciplinary analysis. Lancet Planet. Health 1, e33–e42. doi: 10.1016/S2542-5196(17)30007-4
Hetherington, J. B., Wiethoelter, A. K., Negin, J., and Mor, S. M. (2017). Livestock ownership, animal source foods and child nutritional outcomes in seven rural village clusters in Sub-Saharan Africa. Agric. Food Security 6:9. doi: 10.1186/s40066-016-0079-z
Heumesser, C., and Kray, H. A. (2019). Productive Diversification in African Agriculture and Its Effects on Resilience and Nutrition. Washington, DC: World Bank.
Hoddinott, J. (2012). “Agriculture, health, and nutrition: toward conceptualizing the linkages,” in Reshaping Agriculture for Nutrition and Health, Chapter 2, eds S. Fan and R. Pandya-Lorch (Washington, DC: International Food Policy Research Institute), 13–20.
James, W. P. T. (2018). From treating childhood malnutrition to public health nutrition. Ann. Nutr. Metab. 72, 202–209. doi: 10.1159/000487273
Jones, A. D. (2016). On-farm crop species richness is associated with household diet diversity and quality in subsistence- and market-oriented farming households in Malawi. J. Nutr. 147, 86–96. doi: 10.3945/jn.116.235879
Joy, E. J. M., Ander, E. L., Young, S. D., Black, C. R., Watts, M. J., Chilimba, A. D. C., et al. (2014). Dietary mineral supplies in Africa. Physiol. Plant. 151, 208–229. doi: 10.1111/ppl.12144
Kumssa, D. B., Joy, E. J. M., Ander, E. L., Watts, M. J., Young, S. D., Walker, S., et al. (2015). Dietary calcium and zinc deficiency risks are decreasing but remain prevalent. Sci. Rep. 5:10974. doi: 10.1038/srep10974
Lachat, C., Raneri, J. E., Smith, K. W., Kolsteren, P., Van Damme, P., Verzelen, K., et al. (2017). Dietary species richness as a measure of food biodiversity and nutritional quality of diets. Proc. Natl. Acad. Sci. U.S.A. 115, 127–132. doi: 10.1073/pnas.1709194115
Martin-Prével, Y., Allemand, P., Wiesmann, D., Arimond, M., Ballard, T., Deitchler, M., et al. (2015). On Choosing a Standard Operational Indicator of Women's Dietary Diversity. Rome: FAO.
Mary, S., Saravia-Matus, S., and Gomez y Paloma, S. (2018). Does nutrition-sensitive aid reduce the prevalence of undernourishment? Food Policy 74, 100–116. doi: 10.1016/j.foodpol.2017.11.008
Mason, R., Ndlovu, P., Parkins, J. R., and Luckert, M. K. (2015). Determinants of food security in Tanzania: gendered dimensions of household headship and control of resources. Agric. Hum. Values 32, 539–549. doi: 10.1007/s10460-014-9568-5
May, J. (2018). Keystones affecting sub-Saharan Africa's prospects for achieving food security through balanced diets. Food Res. Int. 104, 4–13. doi: 10.1016/j.foodres.2017.06.062
McDermott, J., Johnson, N., Kadiyala, S., Kennedy, G., and Wyatt, A. J. (2015). Agricultural research for nutrition outcomes–rethinking the agenda. Food Security 7, 593–607. doi: 10.1007/s12571-015-0462-9
Micha, R., Khatibzadeh, S., Shi, P., Andrews, K. G., Engell, R. E., and Mozaffarian, D. (2015). Global, regional and national consumption of major food groups in 1990 and 2010: a systematic analysis including 266 country-specific nutrition surveys worldwide. BMJ Open 5:e008705. doi: 10.1136/bmjopen-2015-008705
Mulmi, P., Masters, W. A., Ghosh, S., Namirembe, G., Rajbhandary, R., Manohar, S., et al. (2017). Household food production is positively associated with dietary diversity and intake of nutrient-dense foods for older preschool children in poorer families: results from a nationally-representative survey in Nepal. PLoS ONE 12:e0186765. doi: 10.1371/journal.pone.0186765
Njuki, J., Poole, J., Johnson, N., Baltenweck, I., Pali, P., Lokman, Z., et al. (2011). Gender, Livestock and Livelihood Indicators. Technical Report October. Nairobi: International Livestock Research Institute.
Ogutu, S. O., Gödecke, T., and Qaim, M. (2019). Agricultural commercialisation and nutrition in smallholder farm households. J. Agric. Econ. doi: 10.1111/1477-9552.12359
Pisa, P. T., Landais, E., Margetts, B., Vorster, H. H., Friedenreich, M., Huybrechts, I., et al. (2017). Inventory on the dietary assessment tools available and needed in africa: a prerequisite for setting up a common methodological research infrastructure for nutritional surveillance, research, and prevention of diet-related non- communicable diseases. Crit. Rev. Food Sci. Nutr. 58, 37–61. doi: 10.1080/10408398.2014.981630
Popkin, B. M. (2014). Nutrition, agriculture and the global food system in low and middle income countries. Food Policy 47, 91–96. doi: 10.1016/j.foodpol.2014.05.001
Prentice, A. M. (2018). The double burden of malnutrition in countries passing through the economic transition. Hot Top. Nutr. 72, 47–54. doi: 10.1159/000487383
Rah, J. H., Akhter, N., Semba, R. D., de Pee, S., Bloem, M. W., Campbell, A. A., et al. (2010). Low dietary diversity is a predictor of child stunting in rural Bangladesh. Eur. J. Clin. Nutr. 64, 1393–1398. doi: 10.1038/ejcn.2010.171
Ramankutty, N., Mehrabi, Z., Waha, K., Jarvis, L., Kremen, C., Herrero, M., et al. (2018). Trends in global agricultural land use: implications for environmental health and food security. Annu. Rev. Plant Biol. 69, 789–815. doi: 10.1146/annurev-arplant-042817-040256
Ritzema, R. S., Douxchamps, S., Fraval, S., Bolliger, A., Hok, L., and Phengsavanh, P. (2019). Household-level drivers of dietary diversity in transitioning agricultural systems: evidence from the Greater Mekong Subregion. Agric. Syst. 176:102657. doi: 10.1016/j.agsy.2019.102657
Ruel, M. T., Quisumbing, A. R., and Balagamwala, M. (2018). Nutrition-sensitive agriculture: what have we learned so far? Glob. Food Security 17, 128–153. doi: 10.1016/j.gfs.2018.01.002
Saha, K. K., Frongillo, E. A., Alam, D. S., Arifeen, S. E., Persson, L. A., and Rasmussen, K. M. (2009). Household food security is associated with growth of infants and young children in rural Bangladesh. Public Health Nutr. 12, 1556–1562. doi: 10.1017/S1368980009004765
Savy, M., Martin-Prével, Y., Sawadogo, P., Kameli, Y., and Delpeuch, F. (2005). Use of variety/diversity scores for diet quality measurement: relation with nutritional status of women in a rural area in Burkina Faso. Eur. J. Clin. Nutr. 59, 703–716. doi: 10.1038/sj.ejcn.1602135
Schipanski, M. E., MacDonald, G. K., Rosenzweig, S., Chappell, M. J., Bennett, E. M., Kerr, R. B., et al. (2016). Realizing resilient food systems. Bioscience 66, 600–610. doi: 10.1093/biosci/biw052
Sibhatu, K. T., and Qaim, M. (2017). Rural food security, subsistence agriculture, and seasonality. PLoS ONE 12:e0186406. doi: 10.1371/journal.pone.0186406
Siegel, K. R., Ali, M. K., Srinivasiah, A., Nugent, R. A., and Narayan, K. M. (2014). Do we produce enough fruits and vegetables to meet global health need? PLoS ONE 9:e0104059. doi: 10.1371/journal.pone.0104059
Steinke, J., Mgimiloko, M. G., Graef, F., Hammond, J., van Wijk, M. T., and van Etten, J. (2019). Prioritizing options for multi-objective agricultural development through the positive deviance approach. PLoS ONE 14:e0212926. doi: 10.1371/journal.pone.0212926
Steyn, N. P., Nel, J. H., Nantel, G., Kennedy, G., and Labadarios, D. (2006). Food variety and dietary diversity scores in children: are they good indicators of dietary adequacy? Public Health Nutr. 9, 644–650. doi: 10.1079/PHN2005912
Tavenner, K., Wijk, M. V., Fraval, S., Hammond, J., Baltenweck, I., Teufel, N., et al. (2019). Intensifying inequality? Gendered trends in commercializing and diversifying smallholder farming systems in East Africa. Front. Sustain. Food Syst. 3:10. doi: 10.3389/fsufs.2019.00010
Torheim, L. E., Ouattara, F., Diarra, M. M., Thiam, F. D., Barikmo, I., Hatløy, A., et al. (2004). Nutrient adequacy and dietary diversity in rural Mali: association and determinants. Eur. J. Clin. Nutr. 58, 594–604. doi: 10.1038/sj.ejcn.1601853
Keywords: nutrition-sensitive, crops, livestock, diet diversity, farm systems, rural development, Bayesian
Citation: Fraval S, Hammond J, Bogard JR, Ng'endo M, van Etten J, Herrero M, Oosting SJ, de Boer IJM, Lannerstad M, Teufel N, Lamanna C, Rosenstock TS, Pagella T, Vanlauwe B, Dontsop-Nguezet PM, Baines D, Carpena P, Njingulula P, Okafor C, Wichern J, Ayantunde A, Bosire C, Chesterman S, Kihoro E, Rao EJO, Skirrow T, Steinke J, Stirling CM, Yameogo V and van Wijk MT (2019) Food Access Deficiencies in Sub-saharan Africa: Prevalence and Implications for Agricultural Interventions. Front. Sustain. Food Syst. 3:104. doi: 10.3389/fsufs.2019.00104
Received: 23 June 2019; Accepted: 25 October 2019;
Published: 19 November 2019.
Edited by:
Ademola Braimoh, World Bank Group, United StatesReviewed by:
Louise Elizabeth Buck, Cornell University, United StatesGabriel da Silva Medina, University of Brasilia, Brazil
Copyright © 2019 Fraval, Hammond, Bogard, Ng'endo, van Etten, Herrero, Oosting, de Boer, Lannerstad, Teufel, Lamanna, Rosenstock, Pagella, Vanlauwe, Dontsop-Nguezet, Baines, Carpena, Njingulula, Okafor, Wichern, Ayantunde, Bosire, Chesterman, Kihoro, Rao, Skirrow, Steinke, Stirling, Yameogo and van Wijk. This is an open-access article distributed under the terms of the Creative Commons Attribution License (CC BY). The use, distribution or reproduction in other forums is permitted, provided the original author(s) and the copyright owner(s) are credited and that the original publication in this journal is cited, in accordance with accepted academic practice. No use, distribution or reproduction is permitted which does not comply with these terms.
*Correspondence: Simon Fraval, c2ltb24uZnJhdmFsQG91dGxvb2suY29t