- 1Department of Physics, University of Helsinki, Helsinki, Finland
- 2Finnish Geospatial Research Institute FGI, National Land Survey of Finland, Kirkkonummi, Finland
- 3VTT Technical Research Centre of Finland Ltd., Espoo, Finland
- 4Institute of Meteorology and Climate Research, Karlsruhe Institute of Technology, Karlsruhe, Germany
- 5Institute of Physics and Technology, Ural Federal University, Ekaterinburg, Russia
- 6Institute for Atmospheric and Earth System Research, University of Helsinki, Helsinki, Finland
- 7NASA Goddard Space Flight Center, Greenbelt, MD, United States
The Earth’s spherical albedo describes the ratio of light reflected from the Earth to that incident from the Sun, an important variable for the Earth’s radiation balance. The spherical albedo has been previously estimated from satellites in low-Earth orbits, and from light reflected from the Moon. We developed a method to derive the Earth’s spherical shortwave albedo using the images from the Earth Polychromatic Imaging Camera (EPIC) on board National Oceanic and Atmospheric Administration’s (NOAA) Deep Space Climate Observatory (DSCOVR). The satellite is located in the Lagrange 1 point between the Earth and the Sun and observes the complete illuminated part of the Earth at once. The method allows us to provide continuously updated spherical albedo time series data starting from 2015. This time series shows a systematic seasonal variation with the mean annual albedo estimated as 0.295±0.008 and an exceptional albedo maximum in 2020, attributed to unusually abundant cloudiness over the Southern Oceans.
Introduction
Solar radiation is the primary energy source of the Earth and largely determines Earth’s climate. The proportion of the incoming solar radiation reflected back to space by the Earth is described by the spherical (i.e., Bond) albedo. It depends on the reflective properties of the Earth and thus it is affected by the proportion of the highly reflective areas relative to darker areas. For example, the melting of the Antarctic and Greenland ice sheets results in increased absorption and decreased albedo. The Earth’s spherical albedo is an indicator of the radiation budget and thus driving the global weather and climate processes.
In the first half of the 20th century, estimates of the spherical albedo were based on an indirect method of observing the Earth-lit Moon (Stephens et al., 2015), and this method is still used (Goode et al., 2021). The earliest satellite measurement of the spherical albedo was made in 1959 by the Explorer 7 satellite and its value has remained approximately 0.3 ever since (Kandel and Viollier, 2010). Since 1997 the albedo is being overseen by the Clouds and the Earth’s Radiant Energy System (CERES), which includes five satellites and seven CERES radiometers (see Wielicki et al., 1996). As of 2017, only five radiometers are operational. The CERES albedo product is a combination of observations from several instruments seeing different parts of the Earth, and as it takes numerous hours for the CERES to scan the entire Earth while the cloud cover of the Earth evolves in a matter of minutes, the spherical albedo evaluation method by the CERES instruments can have noticeable uncertainties in the measured albedo value (Wielicki et al., 1996; Kandel and Viollier, 2010).
To measure the spherical albedo directly one needs to simultaneously detect radiation reflected by the Earth from all directions, which renders such measurements impractical. To circumvent this, we use the Earth Polychromatic Imaging Camera (EPIC) on board the Deep Space Climate Observatory (DSCOVR) spacecraft combined with angular distribution models (ADM) provided by the CERES based on many years of dedicated measurements. A related approach was presented in Su et al. (2018), Su et al. (2020) for the outgoing flux from Earth, instead of deriving the spherical albedo, using EPIC and NISTAR data.
The DSCOVR is a spacecraft orbiting in the Lagrange point 1 around 1.5 million kilometers from Earth, which allows the EPIC to always view practically the entire sunlit hemisphere of the Earth. The DSCOVR was launched in 2015, and the EPIC has been operational ever since apart from one 6-month maintenance break in 2019 (Marshak et al., 2018). Data from the EPIC instrument, with the CERES ADM models, allows us to propose an algorithm that automatically translates directional reflectance obtained from the EPIC images into estimated value of shortwave spherical albedo. We have launched a web service that collects the computed spherical albedo of the Earth from the whole operational period and updates the data daily with the latest observations1. This daily time series of the Earth’s albedo spans over 7 years in time. This enables us to analyze the pattern of temporal variation in albedo over a year which demonstrates anomalies in albedo behavior. The accurate estimate of the shortwave spherical albedo is important in evaluating the energy balance of the earth’s climate system.
The aim of this article is to derive a novel method for estimating the spherical shortwave albedo of the Earth using EPIC imagery and introduce the results. The objective is to improve the estimation of the global albedo value resulting in enhanced input for the radiation budget models of the Earth. In this article, we introduce first the EPIC imagery and the methods that we use to derive the albedo in Materials and Methods. In Results, we show the results of the albedo estimation over the years 2015–2021. Finally in Discussion we discuss the implications of the results.
Materials and Methods
Spherical Albedo From the EPIC Images
The EPIC images constitute a time series of the sunlit part of the Earth, including the atmosphere, starting from June 2015 and continuing at the present time. There are usually about 22 images per day during Northern Hemisphere summer, and 13 during winter. Each multispectral image has 10 wavelength channels between 317 and 780 nm. The channels have full-width-at-half-maximum (FWHM) values between 1 and 3 nm (Ohtake et al., 2010; Ohtake et al., 2013; Geogdzhayev and Marshak, 2018; Herman et al., 2018).
Each image pixel represents the radiance reflected by the corresponding area of Earth. This radiance is a function of the reflective properties of the area, and the solar and satellite angles. The DSCOVR satellite is located in the first Lagrange point between the Earth and the Sun, therefore the radiance is observed close to the backscattering geometry with satellite and solar angles being almost equal. To convert measured narrowband radiances at backscattering into integrated broadband albedo values at top-of-atmosphere (TOA; see Narrowband-to-Broadband and Radiance-to-Radiosity Transformations for more details), we apply the ADMs provided by the CERES project (Su et al., 2015a; Su et al., 2015b). The ADMs are provided for several surface types and cloud fractions, and we combine these into three types for our analysis: clear land, clear ocean, and cloud-covered areas. The reason for this is that the angular resolution of the tabulated ADMs available at the CERES project web pages2 is only 10°, and we want to interpolate with a finer resolution in the backscattering direction and combining similar surface types gives us more robust estimation (see Scattering Geometry and Interpolation of Angular Distribution Models). Additionally, estimating temporary cloud fractions from EPIC images is challenging, a more robust method can be developed for simple clear versus cloud-covered area estimation. Land or ocean surface classification is available from the International Geosphere–Biosphere Programme, and temporal cloud coverage we estimate from the EPIC images using a logistic regression model with input from EPIC channels at 325, 551, and 780 nm (see Cloud Classification in EPIC Images).
The TOA albedo values for each pixel over the Earth’s sunlit disk are averaged for albedo of the Earth at each narrowband wavelength channel. Finally, the narrowband albedos are summed with weights from incident solar spectra at each channel, obtained from NOAA’s climate data record of solar spectral irradiance (Coddington et al., 2015; see Narrowband-to-Broadband and Radiance-to-Radiosity Transformations). The solar spectrum is taken as constant in this study with only the distance between the Earth and the Sun influencing the total flux level.
The daily averaged time series has been filtered to remove outliers. For some EPIC images, not all the wavelength channels are present. If there is not a proper coverage of usable images over the day, some parts of Earth are not present, and this would introduce a bias to the mean albedo of that day. Therefore, these days are left out from our time series data. There is a period between mid-2019 and early 2020 when the EPIC camera was not operational.
We derive the mean yearly shortwave spherical albedo of the Earth by grouping the daily time series values by the day in the year, averaging per day, and finally averaging over days in a year.
Narrowband-to-Broadband and Radiance-to-Radiosity Transformations
The EPIC images contain 10 narrowband channels, namely at 317, 325, 340, 388, 443, 551, 680, 688, 764, and 780 nm. The FWHM values for the channels are between 1 and 3 nm. The channels are measuring the directional radiance. On the other hand, we are interested in the broadband value of the TOA integrated radiosity, i.e., reflected flux integrated over all directions, and the broadband albedo, i.e., the ratio of the reflected flux to the incident flux.
The ADMs provide the conversion from directional broadband radiance to TOA integrated radiosity. They are applied for example in Su et al. (2018), Su et al. (2020) for broadband radiance that is first derived with a narrowband-to-broadband conversion model from the EPIC images. In this work we first apply the ADMs and second the narrowband-to-broadband conversion, but we would like to point out that both of these conversations can be described together with a single linear transform and that the order of narrowband-to-broadband and radiance-to-radiosity is interchangeable.
After the radiance-to-radiosity conversion with the ADMs we combine the narrowband channels into a broadband estimate. We use 8 of the 10 EPIC channels for this conversion. Channels at 688 and 764 nm are measuring the O2 absorption and are left out of the conversion, since they do not describe the reflectance outside these narrow channels. The solar spectral irradiance (Coddington et al., 2015) is convoluted with the response curves of the eight EPIC channels to give weights for the narrowband contributions that are summed together for the broadband estimate.
As the EPIC channels range from 317 to 780 nm, strictly speaking this is the coverage of our broadband estimate. The portion of the solar input in this range is 52.7%. In ultraviolet (UV) below 317 nm there is only 0.5% of the total energy, so we can safely extend the validity of our broadband range into UV. The CERES ADMs, on the other hand, are derived for shortwave broadband range of 300–5000 nm. The use of ADMs to the broadband with wavelength range of 317–780 nm only can be a source of bias in our estimate. However, Su et al. (2018) reports successful usage of ADMs with only three EPIC channels (443, 551, and 680 nm) for narrowband-to-broadband transformation.
Cloud Classification in EPIC Images
The algorithm for cloud classification in the EPIC images was trained with a manually prepared data set of spectral radiances together with subjective cloudy/clear label decision. For image data, we collected images from the first week of every month in 2018. We converted the original HDF5 library data for one multi-channel image into PNG image stack of all the wavelength channels. We opened the image stacks in ImageJ3, and manually selected areas of either clear or cloud-covered land and ocean surfaces. The spectral radiance values of pixels within these areas were recorded together with the label (1) clear land/(2) clear ocean/(3) cloud-covered area. Altogether, we produced 338 areas of clear land, 331 areas of clear ocean, and 481 areas of cloud-covered areas. Each area contains several tens of pixels, the mean values over the areas were employed in the analysis. The ice sheet covered areas, such as Antarctica, are indistinguishable from cloud-covered areas.
A logistic regression model was separately fitted into data with clear land and cloud-covered areas, and data with clear ocean and cloud-covered areas. A logistic regression model has a linear function modeling the log-odds of the probability of an event. In our case, the event is that the surface is covered with clouds. The model can be written as
where
We executed forward-selection stepwise regression to optimize the model with the best value of the Bayesian Information Criterion (BIC) statistics of the model. We ended up with models with a constant coefficient

TABLE 1. The fitted coefficient values for the logistic regression models for cloud-covered vs. clear surface.
Scattering Geometry and Interpolation of Angular Distribution Models
We found it realistic to successfully classify pixels of clear or cloudy land or ocean areas from the EPIC images, but not different cloud types (water or ice) or levels of cloud cover that are present in CERES ADMs. ADMs are also divided into different wind speeds for ocean and into different vegetation types for land. To exploit these, wind speeds on ocean pixels would need to be connected from weather models into our analysis together with up-to-date land cover information. We feel that this would complicate the albedo estimation too much at this stage and could be a topic for future development. We decided to emphasize more the interpolation of the scattering geometry inside the ADM models over having many ocean/land/cloud subtype ADMs.
The scattering geometry in the EPIC observations is such that the observation is always done close to backscattering. The phase angle between the Sun and the DSCOVR spacecraft, as seen from Earth, is below 12° except for few rare cases in years 2020–21. On average, phase angle has been 8.2°. After the break in the DSCOVR operations in 2019, the minimum phase angle has gradually started to decrease (see Figure 7). It was never below 4° before 2020, but now the minimum value is 1.8°.
While in terms of phase angle EPIC is observing close to backscattering, the local solar zenith angle on each Earth pixel varies between 0° and 90°. The ADM tables, on the other hand, have 10° bins for all the three angles (solar zenith, viewing zenith, azimuth). So, our backscattering geometry implies that we need ADM table values inside cells where the solar and viewing zenith angles are in the same bin and azimuth angle is in the bin 0–10°. Thus, the 3-dimensional (in angle) ADM tables will reduce for our purposes to 1-dimensional tables that can be parametrized with the solar zenith angle only.
We find the reduced 1-dimensional table binning of 10° too coarse as it would introduce inaccuracies when applied to pixels having the solar zenith angle anywhere between 0° and 90°. Therefore, we interpolate the reduced ADM tables over the 0° and 90° range using a cubic spline interpolation. We use these interpolated coefficients when converting the TOA radiances measured by EPIC into integrated TOA albedos. We find that the reduced 1-dimensional tables show relatively similar behavior for all clear ocean surfaces regardless of the wind speed, for all clear land surfaces, and for all cloud-covered areas regardless of the cloud type or coverage. Thus, our final set of ADM coefficients come from interpolated data on these three categories, averaged over their subtypes.
Results
The time series of the daily spherical shortwave albedo of the Earth is shown in Figure 1. One can see the evident annual cycle and the typical variation in the albedo with
The yearly annual cycle on albedo can be seen more clearly from Figure 2, where the years have been stacked together. In each year, there are two minima and two maxima. The minima are located close to the Northern and Southern equinoxes, and the maxima slightly before the Northern and Southern solstices. This indicates that the reflectivity of the Earth is at maximum, when either one of the pole areas are tilted more towards the Sun.
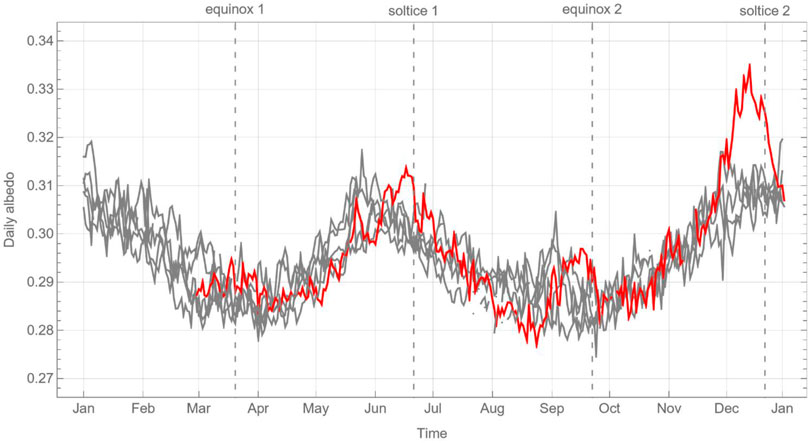
FIGURE 2. Daily averages of the Earth’s albedo with all years stacked together. Data from the year 2020 is highlighted with red color, years 2015–2019 and 2021 are shown in gray.
In Figure 3, we show the derived spherical albedo values on the map. The values are averaged over the months of the equinoxes (March and September) and solstices (June and December). These maps can give further insight of the input of different surface types and the annual variation in the albedo. The polar areas show large contributions during albedo maxima. Interestingly, the Indonesia region in the equator shows high albedos throughout the year. The clouds over the ocean areas are high in albedo, but because of their transient nature, only the most constantly cloud-covered areas show up in the monthly averaged maps.
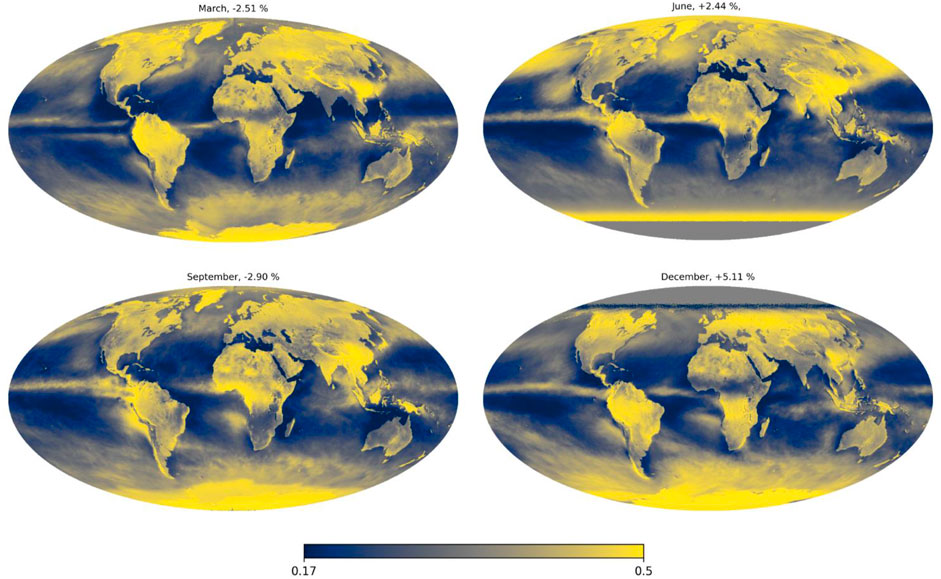
FIGURE 3. The spherical albedo of the Earth. The monthly averages are presented for the months of equinoxes (March and September) and solstices (June and December). Darker and bluer values indicate lower albedo, brighter and more yellow values higher albedo.
The year 2020 is highlighted with red color in Figure 2 because of the anomaly in the second annual maximum. In the EPIC data, there are observations of four other Northern solstice maxima, but the one in 2020 is significantly higher. We can verify the anomaly in December 2020 statistically by studying the monthly averages for each year. The combined monthly averages are shown in Figure 4, plotted as variations from the overall average albedo in a box-and-whiskers chart. The one-way analysis-of-variance test of the differences in the mean monthly values over several years can find significant (p-value less than 0.1%) differences for all months. However, by far the smallest p-value, and therefore the largest difference, is for December, formally less than 10−29.
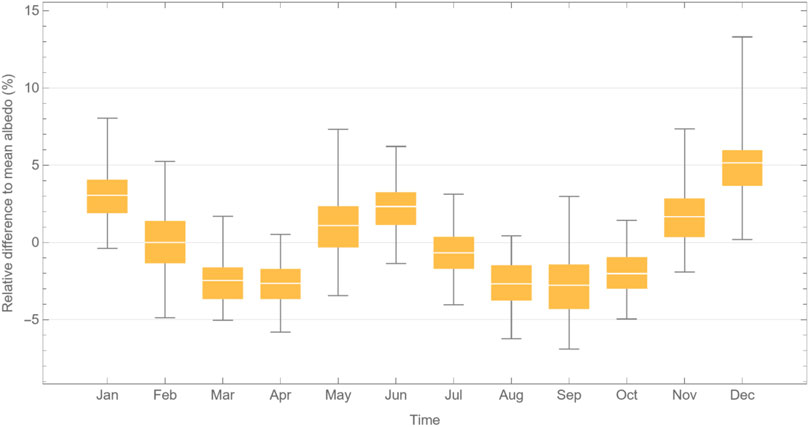
FIGURE 4. Monthly variation in the daily averages of the Earth’s spherical albedo. Values are relative differences from the overall mean albedo of 0.295. The horizontal white line shows the average over the month, the box indicates the range from the first quartile to the third quartile, and the lines the range from the minimum to the maximum.
When conducting t-tests for year pairs inside a month, we find that the only year which differs from all the other years when using the Bonferroni-corrected p-value limit of 0.001/mi, where mi is the number of yearly data sets on month i, is the year 2020 and months August and December. Bonferroni correction is a method to counteract the bias when doing multiple statistical tests on the same phenomena. From these analyses, we conclude that the largest yearly variations in a month are in December, and that is mainly due to year 2020.
We can conclude on the results on temporal variation on Earth’s spherical albedo by summarizing that there are two maxima and two minima. The main maximum occurs in December–January during the Southern Hemisphere summer and the Southern solstice, and the secondary maximum in June during the Northern Hemisphere summer and the Northern solstice. The minimum values are in March–April, and on September, around equinoxes. The coefficient of variation, that is the standard deviation divided by mean, of the daily average values over a year is 2.7%, and the range of minimum-to-maximum deviation of the daily averages from annual average is 0.031 (10.3 percentage points in scale relative to the mean annual albedo). In December 2020, the daily albedo values were up to 0.023 (7.2%, on December 13) larger than the average daily value over all the years.
Discussion
Annual Albedo Variation
The annual variation of the Earth’s spherical (Bond) albedo is described for the first time in detail. Previous investigations mainly describe the variations on the incident solar flux due to the Earth’s elliptic orbit (see Figure 5 in Kandel and Viollier, 2010), as for us, this effect is considered and properly accounted for Su et al. (2018), Su et al. (2020) are using also EPIC images, but they do not reduce the albedo but produce outgoing fluxes. The accurate annual albedo behavior enables the detailed analysis of local geographic and atmospheric effects on the albedo during a year.
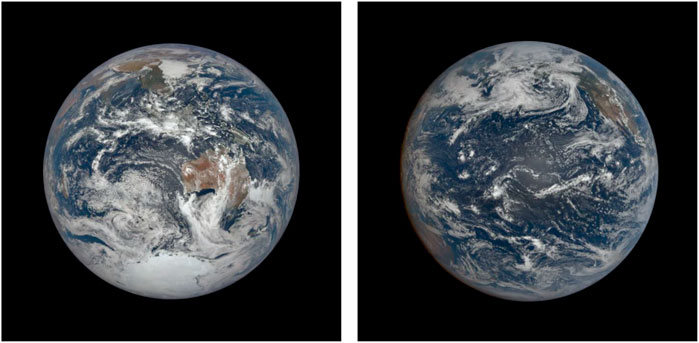
FIGURE 5. EPIC natural color constructed images, taken at 2020-12-13 at 04:40 UCT (left), and 2020-08-04 at 22:25 UCT (right). The image on the left has one of the largest albedos in 2020 (0.350), and the image on the right has one of the lowest (0.253). Image credits: NASA EPIC team, https://epic.gsfc.nasa.gov/.
The Earth contains regions of low albedo (e.g., cloud-free ocean areas, vegetated land areas) and high albedo (e.g., clouds, ice and snow surfaces). Diurnal and annual variations in the albedo time series (Figure 1) result from a modulation of these two aspects, depending on the apparent longitude (diurnal variations) and latitude (annual variations). Especially, the cloud-covered ocean areas increase the albedo. The daily cloud-covered ocean fraction, estimated from the EPIC images, has the correlation coefficient value of 86% with the daily albedo value (see Figure 7).
In annual albedo variations, the main albedo maximum occurs in December, around Southern solstice, when the Antarctic ice sheet, sea ice, and snow cover are visible entirely. At this time, cloud formations of the mid-latitude cyclones over the Southern Ocean are well pronounced. Albedo is further enhanced by the shallow convective cloud cover over the subtropical oceans and the relatively small areas of cloud-free ocean areas visible, on average, at this time of the year.
The secondary albedo maximum occurs in June, around Northern solstice, when the Greenland ice sheet and sea ice in the Arctic Ocean are well exposed. Cloud formations of the mid-latitude cyclones of the North Atlantic and Pacific storm tracks are active, although reduced from their winter maxima. Deep convective clouds of the inter-tropical convergence zone are on the Northern Hemisphere at this time of the year. Again, relatively little cloud-free ocean areas are visible.
The main albedo minimum precedes the main maximum in August–September when neither of the Polar regions is visible. At this time of year, there are relatively few shallow convective clouds over the subtropical Northern Atlantic and Pacific, as well as over the Mediterranean. North Atlantic and Pacific storm track activities are near to their minima. Also, vegetation is at its maximum extent in the Sahel region. Monsoon is in active phase over the Indian peninsula and South-East Asia, thus enhancing the albedo. The secondary minimum precedes the secondary maximum in March–April.
The solar energy input at the Earth’s average distance from the Sun is 1361
Exceptional Albedo Maximum in December 2020
An exceptional albedo occurred on 13 December 2020 and persisted for about a week before and after the peak value. This anomaly coincides with the main annual maximum in December. Our main explanation is a short-lived and exceptional maximum in the amount of shallow convective clouds over the subtropical Indian Ocean and the Pacific east of Australia, see Figure 5. The development of exceptionally wide shallow cloud cover coincides and is favoured by a constellation of several mature mid-latitude cyclones, feeding moist air from the subtropics towards the Antarctic. At the time of the maximum albedo, all relevant large-scale atmosphere-ocean indicators were in close-to-neutral stages4: the Madden-Julian oscillation (MJO) phase centre was in the Oceanic continent, but the amplitude was weak; the Indian Ocean Dipole (IOD) was neutral; El Niño–Southern Oscillation (ENSO) was in a weak La Niña phase (El Niño 3.4 index) and returning to neutral.
In December 2020, the EPIC-derived daily albedo was elevated to 0.334 from its typical December value of 0.312 in 2015–2019. Assuming these to represent well the planetary reflectivity, the emission temperature of the Earth would decrease by 2.1 K through a 7.8
Effect of Phase Angle on Albedo
The phase angle of EPIC observations varies between 2° and 12°. After the DSCOVR operations break in 2019, the phase angle variation has increased, and the minimum phase angle has decreased from previous 4°–1.8° (Marshak et al., 2021). These decreasing phase angles introduce a possible source of bias in our albedo estimation. The resolution in the binned data of the ADM models that we use is 10° in all three angles, indicating that we do not have exact information on how possible backscattering effects (self-shadowing, coherent backscattering) are behaving on small phase angles inside this bin.
Currently, we can only estimate the possible bias resulting from small phase angles. We can do this by computing the deviations of daily albedo values from the average-year albedo values, and by modeling how they are changing as a function of the observation phase angle. From Figure 6 we can see that there is an obvious correlation between these, the linear correlation coefficient being −0.59. Decreasing phase angles are introducing a positive bias, on average, into the albedo values. A second-degree linear fit has the p-values of all the model coefficients significant below 0.1% level, and a slightly better value (0.37) of adjusted
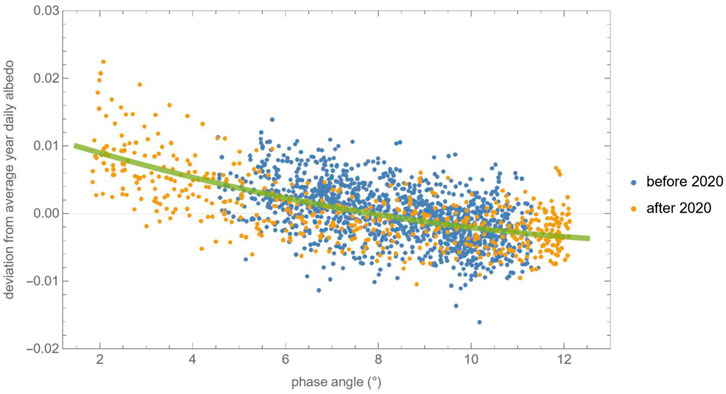
FIGURE 6. Differences between daily albedo values and corresponding values from an average year, as a function of the phase angle of the observation. The solid line shows the second-degree linear fit to the data. The data before and after the DSCOVR operations break in 2019–2020 is shown with different colors, but the model is fitted to both sets together.
This same bias can also be roughly estimated from the small upturns in daily albedo values in November 2020 and March 2021, see Figures 1, 7. These upturns are simultaneous with local phase angle minima of about 2°. Graphical estimation of possible excess in albedo values in these upturns also suggests a value of 0.01 at most.
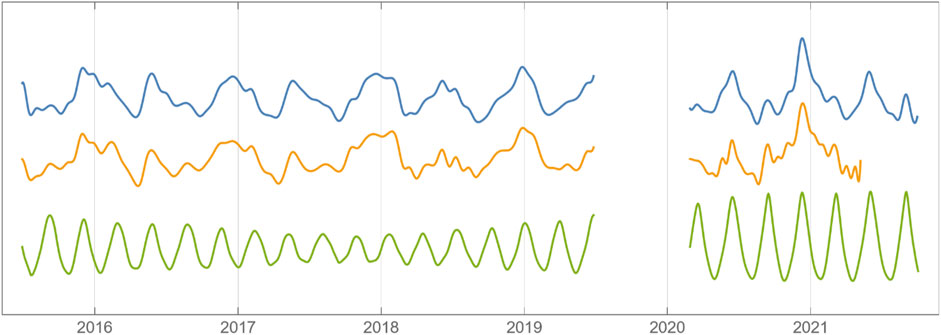
FIGURE 7. The daily average albedo values (blue lines), the daily average fraction of clouds over oceans (orange lines), and the scattering angle (complement of the Sun-Earth-vehicle angle) of the EPIC observations (green lines). The curves have different vertical scales and have been shifted in vertical direction and rescaled for improved visualization.
The exceptional albedo values in December 2020 were at most 0.023 larger than the average December value. This exceptional value was received at the time of local phase angle minima of the DSCOVR spacecraft, 2.1°. We conclude that the effect of the phase angle can explain, at maximum, about half of the difference, and that December 2020 is exceptional in our albedo time series even when taking the possible phase angle effect into account.
Conclusion
We introduce here a novel method for following the spherical shortwave albedo of Earth. The albedo values are updated daily and in almost real time with only a few days delay. The data processing pipeline is fully automated and the estimated albedo values are publicly available. The average yearly albedo estimated with our method, 0.295±0.008, agrees well with the previous works on the albedo. With the albedo time series presented here from 2015 up to date we can study the seasonal variations in albedo, and we find an annual trend with two maxima and two minima, approximately associated with the solstice and the equinox dates. We can also find one anomalous feature with the unusually large yearly maximum in December 2020.
The variations in the albedo can support studies of the radiation budget of Earth and its temporal variation. As the method can also map the albedo values geographically one can relate better the varying local weather and climate conditions with the variations in the albedo.
This method of deriving the spherical shortwave albedo from the EPIC imagery is presented here for the first time. There are several aspects in this method with prospects for further studies and improvements. The cloud classification could probably be improved by taking the local solar zenith angle into account in the model. The CERES ADMs have editions with more detailed angular binning (Edition 4, Su et al., 2015a; Su et al., 2015b). They are not publicly available as they are much larger in size and coupled with the CERES software libraries, however, with adequate resources they could be employed. The increased variation in the phase angle of the EPIC observations after 2020 might call for modeling the effect of the phase angle in the albedo estimation. Finally, a detailed comparison of our albedo estimate to other estimates of albedo or outgoing flux would be very interesting.
Data Availability Statement
The raw image data supporting the conclusion of this article is available at the NASA EarthData site at https://asdc.larc.nasa.gov/data/DSCOVR/EPIC/. The produced albedo data is available at https://albedo.physics.helsinki.fi/.
Author Contributions
The method and algorithm were designed by AP, KM OI, EU, MV, GX, and OW. The algorithm was coded by AP, OI, EU, MV, and OW. The web service was designed by AP, KM, EU, MV, and coded by AP and MV. The data in this article was prepared by AP, as well as the statistical analysis on the data. The article was written by AP, KM, EU, MG, and HJ. All authors provided input and comments to the article.
Funding
Funding is received from Academy of Finland projects 298137, 325805, and 333034.
Conflict of Interest
Author OI was employed by the company VTT Technical Research Centre of Finland Ltd.
The remaining authors declare that the research was conducted in the absence of any commercial or financial relationships that could be construed as a potential conflict of interest.
Publisher’s Note
All claims expressed in this article are solely those of the authors and do not necessarily represent those of their affiliated organizations, or those of the publisher, the editors and the reviewers. Any product that may be evaluated in this article, or claim that may be made by its manufacturer, is not guaranteed or endorsed by the publisher.
Acknowledgments
We acknowledge that computational resources are provided by CSC—IT Center for Science Ltd., Finland.
Footnotes
1http://albedo.physics.helsinki.fi.
2https://ceres.larc.nasa.gov/data/angular-distribution-models/.
4Bureau of Meteorology, climate monitoring, http://www.bom.gov.au/climate/. Accessed 31 January 2022.
References
Coddington, O., Lean, J. L., Lindholm, D., Pilewskie, P., and Snow, M.NOAA CDR Program (2015). NOAA Climate Data Record (CDR) of Solar Spectral Irradiance (SSI), NRLSSI Version 2. NOAA National Centers for Environmental Information. accessed 2020-10-21. doi:10.7289/V51J97P6
Geogdzhayev, I. V., and Marshak, A. (2018). Calibration of the DSCOVR EPIC Visible and NIR Channels Using MODIS Terra and Aqua Data and EPIC Lunar Observations. Atmos. Meas. Tech. 11, 359–368. doi:10.5194/amt-11-359-2018
Goode, P. R., Pallé, E., Shoumko, A., Shoumko, S., Montañes‐Rodriguez, P., and Koonin, S. E. (2021). Earth's Albedo 1998-2017 as Measured from Earthshine. Geophys. Res. Lett. 48, e2021GL094888. doi:10.1029/2021GL094888
Goode, P. R., Qiu, J., Yurchyshyn, V., Hickey, J., Chu, M.-C., Kolbe, E., et al. (2001). Earthshine Observations of the Earth's Reflectance. Geophys. Res. Lett. 28 (9), 1671–1674. doi:10.1029/2000GL012580
Hartmann, D. (2015). Global Physical Climatology. 2nd Edition. Elsevier Science, 498. ISBN: 9780123285317.
Herman, J. R., Huang, L., McPeters, R. D., Ziemke, J., Cede, A., and Blank, K. (2018). Synoptic Ozone, Cloud Reflectivity, and Erythemal Irradiance from Sunrise to sunset for the Whole Earth as Viewed by DSCOVR Spacecraft from the Earth-Sun Lagrange-1. Atmos. Meas. Tech. 11, 1–18. doi:10.5194/amt-11-177-2018
Kandel, R., and Viollier, M. (2010). Observation of the Earth's Radiation Budget from Space. Comptes Rendus Geosci. 342 (4), 286–300. doi:10.1016/j.crte.2010.01.005
Marshak, A., Delgado-Bonal, A., and Knyazikhin, Y. (2021). Effect of Scattering Angle on Earth Reflectance. Front. Remote Sens. 2. doi:10.3389/frsen.2021.719610
Marshak, A., Herman, J., Adam, S., Karin, B., Carn, S., Cede, A., et al. (2018). Earth Observations from DSCOVR EPIC Instrument. Bull. Am. Meteorol. Soc. 99 (9), 1829–1850. doi:10.1175/BAMS-D-17-0223.1
Ohtake, M., Matsunaga, T., Yokota, Y., Yamamoto, S., Ogawa, Y., Morota, T., et al. (2010). Deriving the Absolute Reflectance of Lunar Surface Using SELENE (Kaguya) Multiband Imager Data. Space Sci. Rev. 154, 57–77. doi:10.1007/s11214-010-9689-0
Ohtake, M., Pieters, C. M., Isaacson, P., Besse, S., Yokota, Y., Matsunaga, T., et al. (2013). One Moon, many Measurements 3: Spectral Reflectance. Icarus 226, 364–374. doi:10.1016/j.icarus.2013.05.010
Stephens, G. L., O'Brien, D., Webster, P. J., Pilewski, P., Kato, S., and Li, J.-l. (2015). The Albedo of Earth. Rev. Geophys. 53 (1), 141–163. doi:10.1002/2014RG000449
Su, W., Corbett, J., Eitzen, Z., and Liang, L. (2015a). Next-generation Angular Distribution Models for Top-Of-Atmosphere Radiative Flux Calculation from CERES Instruments: Methodology. Atmos. Meas. Tech. 8, 611–632. doi:10.5194/amt-8-611-2015
Su, W., Corbett, J., Eitzen, Z., and Liang, L. (2015b). Next-generation Angular Distribution Models for Top-Of-Atmosphere Radiative Flux Calculation from CERES Instruments: Validation. Atmos. Meas. Tech. 8, 3297–3313. doi:10.5194/amt-8-3297-2015
Su, W., Liang, L., Doelling, D. R., Minnis, P., Duda, D. P., Khlopenkov, K., et al. (2018). Determining the Shortwave Radiative Flux from Earth Polychromatic Imaging Camera. J. Geophys. Res. Atmos. 123 (20), 479–511. doi:10.1029/2018JD029390
Su, W., Minnis, P., Liang, L., Duda, D. P., Khlopenkov, K., Thieman, M. M., et al. (2020). Determining the Daytime Earth Radiative Flux from National Institute of Standards and Technology Advanced Radiometer (NISTAR) Measurements. Atmos. Meas. Tech. 13 (2), 429–443. doi:10.5194/amt-13-429-2020
Keywords: albedo, EPIC camera, DSCOVR, shortwave radiation, radiation budget
Citation: Penttilä A, Muinonen K, Ihalainen O, Uvarova E, Vuori M, Xu G, Näränen J, Wilkman O, Peltoniemi J, Gritsevich M, Järvinen H and Marshak A (2022) Temporal Variation of the Shortwave Spherical Albedo of the Earth. Front. Remote Sens. 3:790723. doi: 10.3389/frsen.2022.790723
Received: 07 October 2021; Accepted: 07 February 2022;
Published: 11 March 2022.
Edited by:
Hartmut Boesch, University of Leicester, United KingdomReviewed by:
Bing Lin, National Aeronautics and Space Administration (NASA), United StatesYves Julien, University of Valencia, Spain
Copyright © 2022 Penttilä, Muinonen, Ihalainen, Uvarova, Vuori, Xu, Näränen, Wilkman, Peltoniemi, Gritsevich, Järvinen and Marshak. This is an open-access article distributed under the terms of the Creative Commons Attribution License (CC BY). The use, distribution or reproduction in other forums is permitted, provided the original author(s) and the copyright owner(s) are credited and that the original publication in this journal is cited, in accordance with accepted academic practice. No use, distribution or reproduction is permitted which does not comply with these terms.
*Correspondence: A. Penttilä, antti.i.penttila@helsinki.fi