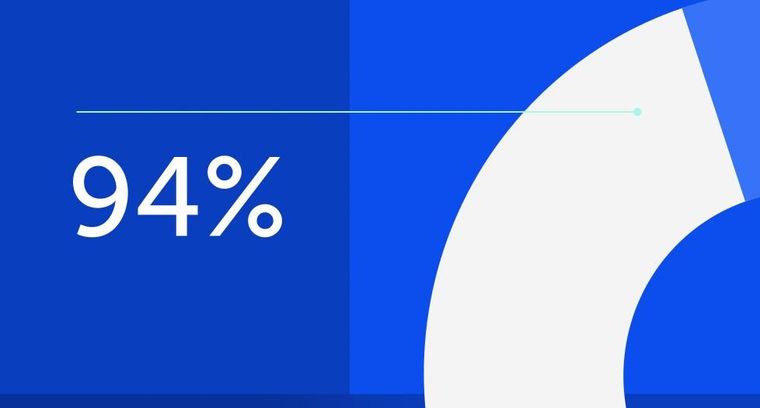
94% of researchers rate our articles as excellent or good
Learn more about the work of our research integrity team to safeguard the quality of each article we publish.
Find out more
ORIGINAL RESEARCH article
Front. Psychol., 22 March 2023
Sec. Psychopathology
Volume 14 - 2023 | https://doi.org/10.3389/fpsyg.2023.1153335
This article is part of the Research TopicAdvances of Neurobiological Basis and Psychopathological Mechanism for Mood DisordersView all 4 articles
Objective: Sleep disturbances (SD) are commonly found in patients with major depressive disorder (MDD). This study aims to explore the influence of SD symptoms on clinical characteristics in patients with MDD and to investigate the shared and distinct fractional amplitude of low-frequency fluctuation (fALFF) patterns in these patients with or without SD symptoms.
Methods: Twenty-four MDD patients with SD symptoms (Pa_s), 33 MDD patients without SD symptoms (Pa_ns) and 32 healthy controls (HCs) were included in this study. The fALFF and correlation analyses were applied to analyze the features of imaging and clinical data.
Results: Pa_s showed more severe anxiety and depression than Pa_ns. Compared with Pa_ns, Pa_s exhibited increased fALFF value in the left precuneus. Patients shared abnormal fALFF in the frontal-occipital brain regions. There was a positive correlation between fALFF values of the left precuneus and sleep disturbance scores (r = 0.607, p = 0.0000056734) in all patients in addition to a negative correlation between fALFF values of the left MOG/cuneus and HAMD-17 total scores (r = −0.595, p = 0.002141) in Pa_s. The receiver operating characteristic (ROC) results of the fALFF could be used to discriminate Pa_s from Pa_ns with a specificity of 72.73% and a sensitivity of 70.83%.
Conclusion: Pa_s displayed more serious anxiety and depression symptoms. Patients shared abnormal fALFF in the frontal-occipital brain regions, which may be a common characteristic for MDD. And increased fALFF value in the left precuneus might be a specific neuroimaging feature of MDD patients with SD symptoms.
Major depressive disorder (MDD) is a worldwide disabling disease which brings remarkable social and economic burden. Approximately over 300 million people around the world suffer depression and the prevalence is still rising (Friedrich, 2017). MDD is characterized by persistently depressive mood, anxiety, cognitive impairment, sleep disorders, suicidal thoughts and motivation alteration. About 60% patients with MDD will have recurrences with 10–20% risk of ineffective remission using current therapies in continuous episodes (Monroe & Harkness, 2011). Due to its early onset and frequent recurrences, MDD is one of the most important causes of social disability (CADTH, 2016).
Sleep disturbances (SD) are commonly reported in patients with persistent depression (Clark et al., 2009). Over 90% patients with MDD take SD as the chief complaint. The usual performance of SD includes difficulty of falling asleep, sleep interruption and early wake-up (Tsuno et al., 2005). SD could impair emotion, memory, attention and other executive functions (Liu et al., 2015). But SD often precedes depression rather than secondary to it, and constantly exists in the remission period. SD also affects the development, treatment response and prognosis of depressive disorder, and increases the risk of depression development (Lovato & Gradisar, 2014). Improvement of sleeping in patients with depression could remiss the depressive symptoms (Manber et al., 2008). A meta-analysis has suggested that people with insomnia have doubled risk of suffering depression than those without SD (Baglioni et al., 2011).
Apparently, there is a potentially close correlation between MDD and SD, which is not simply a cause-effect relationship but appears to be a complicated bidirectional association. Recently, neuroimaging has become a valuable way to investigate the pathogenesis and mechanisms of mental disorders. Amplitude of low-frequency fluctuation (ALFF) and fractional ALFF (fALFF), which are based on the blood oxygenation level-dependent (BOLD) fMRI signals, have been widely applied to identify the low-frequency fluctuation of spontaneous neural activity at rest (Biswal et al., 1995; Zhuo et al., 2019). In recent years, multiple studies have explored the underlying association between SD and MDD. A recent research has found that there was a correlation between the severity of insomnia in patients with MDD and increased ALFF values in the right inferior frontal gyrus (IFG)/anterior insula (Liu et al., 2018). And a positive correlation between fALFF values of the right superior parietal gyrus (SPG) and baseline sleep efficiency in patients with MDD was also reported (Chen et al., 2022). Another study has indicated that the SD scores of the 17-item Hamilton Depression Rating Scale for Depression (HAMD-17) could be predicted by a combination of gray matter density and fALFF values (Shi et al., 2021). And the smaller cortex surface area was found in frontoparietal cortices including the left inferior frontal gyrus pars triangularis, left frontal pole, right superior parietal cortex, and right supramarginal gyrus in patients with MDD with serious insomnia (Leerssen et al., 2020). Besides, functional connectivity between nucleus accumbens and default-mode network (DMN) was associated with the severity of insomnia, and nucleus accumbens-based functional connectivity in the reward network was correlated with depressive symptoms in patients with chronic insomnia (Gong et al., 2021). However, it remains unclear whether there were shared and differential brain spontaneous neural activities at rest in MDD with or without SD.
In the present study, we aimed to determine the clinical characteristics of MDD patients with or without SD symptoms. Furthermore, we have employed fALFF to analyze the common and different alterations of brain spontaneous neural activities in these two groups in order to provide more insights to better understand correlation between MDD and SD.
The patients with MDD were recruited from the Second Xiangya Hospital, and the HCs were recruited from the local community. All participants were age-and education-matched and were Han Chinese and right-handed. The HCs would be ruled out if they: (1) had acute physical illness (here only included structural or organic diseases) or neurological illness, or a history of substance abuse; (2) had a history of brain injury resulting in loss of consciousness; (3) were pregnant or were unable to undergo MRI scans.” The diagnosis of MDD was based on the Diagnostic and Statistical Manual of Mental Disorders-Fifth Edition (DSM-5) by two psychiatrists independently. Patients with MDD were allocated to Pa_s group (patients with chief complaint of SD symptoms, and SD scores >4, n = 26) and Pa_ns group (patients without chief complaint of SD symptoms, and SD scores ≤4, n = 34) depending on the SD scores which were computed by adding scores of items 4, 5, and 6 of the 17-item Hamilton Rating Scale for Depression (HAMD-17) (Liu et al., 2018). Detailed demographic information was shown in Table 1. All patients had HAMD-17 scores >20 and had no history of major somatic diseases or other psychiatric disorders, no history of antidepressant treatment, substance abuse or electroconvulsive therapy. Pregnancy or incapacity of participating in brain MRI scan was also excluded.
The study was conducted according to the Helsinki Declaration and approved by the Medical Research Ethics Committee of the Second Xiangya Hospital, Central South University, Changsha, China. All participants signed a written informed consent.
The severity of depressive symptoms was assessed by the scores of HAMD-17. Anxiety/somatization severity was evaluated by items10-13, 15 and 17. Retardation symptoms were evaluated by items 1, 7, 8 and 14. Severity of cognitive disturbances was evaluated by items 2, 3 and 9. Severity of weight loss was evaluated by items 16. Anxiety state was assessed by the Beck anxiety inventory (BAI).
All participants received the rs-fMRI scanning on a 3.0 T scanner (General Electric, FairfieldConnecticut, USA). They were informed to lay supine in the scanner with heads fixed with a foam padding and belt, keeping motionless with eyes closed. Echo planar imaging (EPI) was employed to acquire the resting-state functional images with the following parameters: repetition time/echo time (TR/TE) = 2000/30 ms 33 axial slices, 64 × 64 matrix, 90° flip angle, 22 cm FOV, 4 mm section thickness, no slice gap, and 240 volumes.
Data were pre-processed using the Data Processing Assistant for Resting-State fMRI (DPARSF v5.2; DPARSF1) software (Chao-Gan & Yu-Feng, 2010). The first 10 images were deleted for MRI to achieve signal equilibrium and for the participants to adapt to the scanning noise. And the resting images were corrected of slice timing and head motion. We excluded participants with head motion exceeding 2 mm of displacement in the x-, y-, or z-axis or 2° angular motion in each axis. These images were normalized to the standard Montreal Neurological Institute (MNI) space and resampled with a resolution of 3 × 3 × 3 mm3. Spatial smoothing was conducted via a 4-mm Gaussian kernel of full width at half maximum (FWHM). Linear trend subtraction and temporal filtering (0.01–0.08 Hz) were performed on the time series of each voxel to reduce the effect of low-frequency drifts and physiological high frequency respiratory and cardiac noise for further analysis. Calculation of fALFF was refered to the previous study (Zou et al., 2008). Fast Fourier transform was applied to convert the time course of each voxel to the frequency domain to obtain the power spectrum. Then, the square root of the power spectrum was calculated, and the average was obtained across 0.01–0.08 Hz. The fALFF was calculated as the ratio of the sum of amplitude across 0.01–0.08 Hz to that across the complete frequency range. For standardization, the fALFF in each voxel was divided by the global mean fALFF value.
Difference in demographic, clinical and neuroimaging data across Pa_s, Pa_ns and HCs was compared. The continuous data were compared by Student’s t-test or one-way analysis of variance (ANOVA) and the categorical data were compared with chi-square test.
Analyses of covariance (ANCOVA), followed by post-hoc t-tests, was performed on fALFF maps of each participant across the three groups to discriminate the group differences. Age, sex, years of education and framewise displacement were applied as covariates. The results were FDR (false discovery rate) corrected at p < 0.05.
Correlation analysis was performed in the fALFF values of clusters with significant difference. Pearson or Spearman correlation analyses were used to assess the correlation between the extracted fALFF and scores of HAMD-17 and BAI scales, follwed by the Bonferroni correction to raise the inspection level.
The receiver operating characteristic (ROC) was used to discriminate Pa_s from Pa_ns. And the best cutoff which maxmized the sum of sensitivity and specificity was calculated.
We have recruited sixty first-episode patients with MDD at the Second Xiangya hospital, and 34 HCs from the local community. We, respectively, excluded 2, 1 and 2 participants from Pa_s, Pa_ns and HCs due to the head motion. Finally, 24 Pa_s, 33 Pa_ns and 32 HCs were included in the final analysis. The detailed data were shown in Table 1. No difference was found in age and years of education among the three groups except gender. And there was no difference of illness duration between Pa_s and Pa_ns. Meanwhile, Pa_s had higher scores in BAI scale, HAMD-17 scale and sleep disturbance than Pa_ns. All patients had higher scores in BAI scale, HAMD-17 scale and five other subscales than HCs.
The fALFF values were collected and compared with ANCOVA analyses and significant differences were found in the frontal, occipital, and parietal gyri among the three groups (Figure 1A).
Figure 1. Different fALFF values and brain regions with significantly different fALFF values across three groups. The color bar indicates the F values based on ANCOVA (A). Brain regions with fALFF difference between Pa_s and Pa_ns (B). Brain regions with fALFF difference between Pa_s and HCs (C). Brain regions with fALFF difference between Pa_ns and HCs (D). For panels (B–D), the color bar indicates the t values from post-hoc t-tests. Red and blue colors, respectively, represent increased and decreased fALFF. fALFF, fractional amplitude of low-frequency fluctuation; ANCOVA, analysis of covariance. Pa_s, major depressive disorder with sleep disturbance; Pa_ns, major depressive disorder without sleep disturbance. HCs, healthy controls.
Compared with Pa_ns, Pa_s showed increased fALFF values in the left precuneus (Figure 1B, Table 2). Besides, Pa_s exhibited higher fALFF values in the bilateral superior MPFC/SMA but lower values in the right middle occipital gyrus (MOG) /fusiform gyrus and left MOG/cuneus relative to HCs (Figure 1C, Table 2). In addition, increased fALFF values were found in the right inferior frontal gyrus (IFG) and bilateral superior MPFC/SMA in Pa_ns compared to HCs. And Pa_ns showed decreased fALFF values in the right MOG/inferior occipital gyrus (IOG), left MOG/IOG and bilateral MOG/cuneus than HCs (Figure 1D, Table 2).
For all patients, there was a positive correlation between fALFF values of the left precuneus and BAI scores (r = 0.308, p = 0.023) in addition to fALFF values of the left precuneus and sleep disturbance scores (r = 0.607, p = 0.0000056734) (Figure 2A). But the correlation between fALFF values of the left precuneus and BAI scores failed to survive the Bonferroni correction.
Figure 2. Correlations between fALFF values and clinical variables. For all patients with MDD, there was a positive correlation between fALFF values of the left precuneus and BAI scores (A). For Pa_s, there was a negative correlation between fALFF values of the left middle occipital gyrus/cuneus and HAMD-17 total scores (B). fALFF, fractional amplitude of low-frequency fluctuation; MDD, major depressive disorder; BAI, Beck anxiety inventory; Pa_s, major depressive disorder with sleep disturbance; HAMD-17, 17-item Hamilton Rating Scale for Depression.
For Pa_s, the fALFF values of the right MOG/fusion gyrus and HAMD-17 total scores (r = −0.406, p = 0.049), fALFF values of the left MOG/cuneus and HAMD-17 total scores (r = −0.595, p = 0.002141) (Figure 2B) apart from fALFF values of the left MOG/cuneus and cognitive disturbance scores (r = −0.515, p = 0.010) displayed inverse correlations. But only the correlation between fALFF values of the left MOG/cuneus and HAMD-17 total scores survived the Bonferroni correction.
For Pa_ns, positive correlations were found between weight lost scores and fALFF values of the bilateral MOG/cuneus (r = 0.379, p = 0.030) or the left MOG/IOG (r = 0.492, p = 0.004) or the right MOG/IOG (r = 0.426, p = 0.013). But all these correlations did not pertain after the Bonferroni correction.
The fALFF values in the left precuneus of Pa_s and Pa_ns were further analysed with the receiver operating characteristic (ROC). The results indicated that fALFF values in the left precuneus could be used to differentiate Pa_n from Pa_ns with a satisfactory specificity of 72.73% and a sensitivity of 70.83% (Figure 3). The area under the curve (AUC) was 0.8169 for the ROC results.
Figure 3. Receiver operating characteristic (ROC) results indicated that fALFF values in the left precuneus could discriminate Pa_s from Pa_ns. Pa_s, major depressive disorder with sleep disturbance; Pa_ns, major depressive disorder without sleep disturbance.
In this study, we found that Pa_s had more severe anxiety and depression than Pa_ns, which could be reflected with the higher scores of BAI scale and HAMD-17 scale Pa_s obtained. This suggested that SD symptoms negatively influenced MDD patients. In addition, patients shared abnormal fALFF in the frontal-occipital brain regions, which may be a common characteristic for MDD. Moreover, Pa_s showed increased fALFF values in the left precuneus than Pa_ns, which could be used to discriminate the two groups according to the ROC results with a specificity of 72.73% and a sensitivity of 70.83% (Figure 3).
This study also showed that Pa_s presented higher scores of BAI scale and HAMD-17 scale relative to Pa_ns. But there was no significant difference in the scores of anxiety/somatization, retardation symptoms, weight loss and cognitive disturbances between these two groups. Despite Pa_s did not have a higher score of anxiety/somatization in HAMD-17, they did score higher in BAI scale, which may be due to the discrepant abilities to detect statistical differences in anxiety. BAI scale has a total of 21 items to assess anxiety severity. It focuses on somatic symptoms of anxiety and is applied to acquire purer measure of anxiety which discriminates from depression (Julian, 2011). While anxiety/somatization factor in HAMD-17 is unstable and is weakly correlated to Hamilton Anxiety Scale (HAMA), which may not be enough to measure anxiety severity in depressive patients (Goldberger et al., 2011). This means BAI scores of anxiety could be more reliable relative to anxiety/somatization scores in HAMD-17. These results suggested that Pa_s had a more severe total disease state, which indicated that SD was related to higher levels of anxiety and depression. As we know, SD is a common symptom in MDD which is used to help MDD diagnosis in DSM-5(Spiegel et al., 2013). SD is also considered to be a main risk factor and predictor of depression (Baglioni et al., 2011). Recently, mounting evidence has demonstrated that SD occurs prior to depression (Jaussent et al., 2011). An early cross-sectional study suggested that young adults who had persistent insomnia presented more severe major depression and generalized anxiety (Vollrath et al., 1989). In addition, depressive patients with SD usually had more difficulties in treatment and continuous insomnia was thought to be an important predictor of depression relapse (Vollrath et al., 1989). All these implied that there may be a bidirectional relationship between SD and MDD. Increasing evidence has shown that elevated levels of inflammatory cytokines like IL-6 and TNFα were found in adults with MDD and people with sleep disorders (Irwin et al., 2006; Dowlati et al., 2010). SD could result in increased inflammation whereas antagonism of endogenous inflammation would improve depressive symptoms (Irwin et al., 2006, 2010; Raison et al., 2013). But the exact interaction between them is still unknown. Besides, twin studies suggest that MDD and SD are all heritable and other research demonstrated that they overlap significantly (Lind et al., 2015, 2017; Fang et al., 2019). Due to the close association between SD and MDD, improved SD would be beneficial to the treatment and prognosis of MDD.
Pa_s showed higher fALFF values in the left precuneus compared with Pa_ns. And for all patients with MDD, there was a positive correlation between fALFF values in the left precuneus and SD scores. These results indicate that the left precuneus might be closely associated with SD symptoms in MDD. The precuneus plays an important role in highly integrated cognitive tasks including attention, conscious perception, visuospatial imagery, episodic and working memory retrieval (Lundstrom et al., 2005; Cavanna & Trimble, 2006). And precuneus is a key component of the default-mode network (DMN) which mainly spans medial prefrontal cortex, medial temporal cortex and posterior cingulate cortex (Fox & Raichle, 2007; Buckner et al., 2008). It is reported that the DMN is related to information collection, self-referential mental activity, consciousness, adaption, mind wandering or daydreaming, emotion and anxiety (Gusnard et al., 2001a, b; Simpson et al., 2001; Cabeza et al., 2002; Mason et al., 2007; Andrews-Hanna et al., 2010; Wilson et al., 2010). When brain is resting, DMN is activated to maintain internal mental state. Plentiful studies have suggested that patients with sleep disorders have functional alteration in the DMN. For example, Luo et al. have found increased functional connectivity in the left precuneus of patients with sleep disorders and mild cognitive impairment (Luo et al., 2022). In addition, decreased functional connectvity is concerned with dysfunctional cognition in the DMN at rest and enhanced functional connectivity may be compensatory for the impaired cognition (Luo et al., 2022). There was no significant difference in cognitive disturbance between Pa_s and Pa_ns. Therefore, the abnormally increased fALFF values in the left precuneus might reflect SD-associated impairment in cognition along with other functions and an adaptive compensation in the DMN (Chen et al., 2016).
Compared to HCs, the Pa_s and Pa_ns patients presented increased fALFF values of the bilateral superior MPFC/SMA. MPFC is of great importance in attention, working memory, long-term memory and emotional and inhibitory control (Bittar & Labonté, 2021) and when dysfunctional, may lead to the depressive-like behaviors. MPFC has been considered to be closely related with MDD (Belleau et al., 2019). In other studies, MPFC of patients with MDD showed increased ALFF values (Gong et al., 2020) and the altered values were positively associated with glutamate concentration in the MPFC (Zhang et al., 2016). The raised levels of glutamate in prefrontal cortex of patients with MDD were confirmed in post-mortem studies (Hashimoto et al., 2007). In addition, numerous evidence has suggested that dysregulated glutamate-glutamine cycling in the MPFC is related to MDD. Inhibition of glutamine synthase which could convert glutamate to glutamine in MPFC would cause depressive behavior (Hashimoto et al., 2007; Lee et al., 2013). Apart from changes in glutamate concentration, decreased GABA levels in MPFC were found in patients with MDD which could be elevated by effective treatment (Dubin et al., 2016; Brennan et al., 2017). Furthermore, the reduced GABA levels in MPFC are correlated with treatment resistance in MDD (Price et al., 2009; Levinson et al., 2010). Several meta-analyses have reported that patients with MDD displayed reduced MPFC volume. And the reduction of volumes is more significant when depression could not remit (Frodl et al., 2008). Thus, these findings may suggest that the abnormally increased fALFF value in the MPFC is a potential marker for MDD.
Compared with HCs, Pa_s showed lower fALFF values in the right MOG/fusiform gyrus and the left MOG/cuneus. Pa_ns also displayed lower fALFF values in the right MOG/IOG, left MOG/IOG and bilateral MOG/cuneus. Besides, fALFF values of the left MOG/cuneus in Pa_s were negatively correlated to total HAMD-17 scores. Various research has indicated that the occipital cortex may be associated with MDD (Bhagwagar et al., 2007; Furey et al., 2013). Zhao et al. have found thicker gray matter in the left fusiform and right lateral occipital cortex and thinner gray matter in the bilateral lingual cortex and left cuneus of patients with MDD (Zhao et al., 2017). Lee et al. and Na et al. found thinner left occipital cortex and bilateral fusiform gyrus in patients with MDD (Na et al., 2016; Lee et al., 2021). Moreover, occipital bending that occipital cortex wraps around other brain areas might be a characteristic of MDD which often exists in treatment resistant patients with MDD (Siebert, 2015). Patients with MDD showed frequent structural abnormalities in regions of visual recogniton network heavily involved in facial emotional processing. And these structural alteration within the visual recognition network might be related to damaged selective attention in MDD (Desseilles et al., 2009; Tao et al., 2013; Zhao et al., 2017). Negative attention bias in the information processing is of great importance in depression episode which could contribute directly to depression and serve as a risk factor (Koster et al., 2009; Disner et al., 2011; Foland-Ross & Gotlib, 2012). Our previous research and Teng et al. respectively found decreased ALFF values of the occipital cortex and left-MOG in patients with MDD, indicating that visual processing was disturbed in MDD (Guo et al., 2012; Teng et al., 2018). Together with these studies, the low activity in the occipital cortex was possibly associated with dysfunctional visual emotional information processing in patients with MDD.
Pa_ns showed increased fALFF values in the right IFG relative to HCs. IFG is associated with emotion regulation and cognition control (Jastorff et al., 2016; Urgesi et al., 2016). Abnormal recruitment of IFG may be involved in emotional stimulus processing in adults with MDD (Disner et al., 2011). Our study included more females in Pa_ns. The increased fALFF values in the right IFG may be a result caused by the gender bias. It’s reported that MDD female patients showed greater hyperactivity in the right IFG than the left part during facial emotion processing. And this laterality was associated with patients’ performance (Briceño et al., 2013). There were many studies indicating that structurally altered IFG was related to MDD. For example, IFG volumes of patients with MDD were smaller than healthy people and had significant relation to depressive severity (Kandilarova et al., 2019; Dai et al., 2020). Thus, IFG may have some specific association with MDD about emotion processing, which shall be warranted in future studies.
There are still a few limitations which should be noticed in this study. First, our sample size was small and we did not classify different SD symptoms in detail to explore the fALFF or score distinction among patients with distinct SD performance. Second, this was a cross-sectional study which could not analyze cause-effect relationship thus we cannot define whether the abnormal fALFF values were the consequence or causation of SD in MDD. Third, our findings were limited in Han Chinese, and further studies in different ethnic groups are essential to validate this research.
Our study has revealed that SD symptoms may produce negative effects in MDD patients. In addition, the shared and different fALFF changes in patients with MDD with or without SD symptoms were also demonstrated. Patients shared abnormal fALFF in the frontal-occipital brain regions, which may be a common characteristic for MDD. Pa_s showed increased fALFF value in the left precuneus compared with Pa_ns, which could be used to discriminate MDD patients with or without SD symptoms, indicating a potential abnormal activity within this region in MDD patients with SD symptoms.
The original contributions presented in the study are included in the article/supplementary material, further inquiries can be directed to the corresponding authors.
The studies involving human participants were reviewed and approved by Medical Research Ethics Committee of the Second Xiangya Hospital. The patients/participants provided their written informed consent to participate in this study.
WG and BL designed the research. YO carried out the experiments and analyzed the data. WG, BL, and NZ wrote the paper. HL, FL, GX, and PL contributed to the MRI data acquisition. All authors contributed to the article and approved the submitted version.
This study was supported by grants from the National Natural Science Foundation of China (Grant Numbers: 82171508 and 82071507).
The authors declare that the research was conducted in the absence of any commercial or financial relationships that could be construed as a potential conflict of interest.
All claims expressed in this article are solely those of the authors and do not necessarily represent those of their affiliated organizations, or those of the publisher, the editors and the reviewers. Any product that may be evaluated in this article, or claim that may be made by its manufacturer, is not guaranteed or endorsed by the publisher.
Andrews-Hanna, J. R., Reidler, J. S., Sepulcre, J., Poulin, R., and Buckner, R. L. (2010). Functional-anatomic fractionation of the brain’s default network. Neuron 65, 550–562. doi: 10.1016/j.neuron.2010.02.005
Baglioni, C., Battagliese, G., Feige, B., Spiegelhalder, K., Nissen, C., Voderholzer, U., et al. (2011). Insomnia as a predictor of depression: a meta-analytic evaluation of longitudinal epidemiological studies. J. Affect. Disord. 135, 10–19. doi: 10.1016/j.jad.2011.01.011
Belleau, E. L., Treadway, M. T., and Pizzagalli, D. A. (2019). The impact of stress and major depressive disorder on hippocampal and medial prefrontal cortex morphology. Biol. Psychiatry 85, 443–453. doi: 10.1016/j.biopsych.2018.09.031
Bhagwagar, Z., Wylezinska, M., Jezzard, P., Evans, J., Ashworth, F., Sule, A., et al. (2007). Reduction in occipital cortex gamma-aminobutyric acid concentrations in medication-free recovered unipolar depressed and bipolar subjects. Biol. Psychiatry 61, 806–812. doi: 10.1016/j.biopsych.2006.08.048
Biswal, B., Yetkin, F. Z., Haughton, V. M., and Hyde, J. S. (1995). Functional connectivity in the motor cortex of resting human brain using echo-planar MRI. Magn. Reson. Med. 34, 537–541. doi: 10.1002/mrm.1910340409
Bittar, T. P., and Labonté, B. (2021). Functional contribution of the medial prefrontal circuitry in major depressive disorder and stress-induced depressive-like behaviors. Front. Behav. Neurosci. 15:699592. doi: 10.3389/fnbeh.2021.699592
Briceño, E. M., Weisenbach, S. L., Rapport, L. J., Hazlett, K. E., Bieliauskas, L. A., Haase, B. D., et al. (2013). Shifted inferior frontal laterality in women with major depressive disorder is related to emotion-processing deficits. Psychol. Med. 43, 1433–1445. doi: 10.1017/S0033291712002176
Brennan, B. P., Admon, R., Perriello, C., LaFlamme, E. M., Athey, A. J., Pizzagalli, D. A., et al. (2017). Acute change in anterior cingulate cortex GABA, but not glutamine/glutamate, mediates antidepressant response to citalopram. Psychiatry Res. Neuroimaging 269, 9–16. doi: 10.1016/j.pscychresns.2017.08.009
Buckner, R. L., Andrews-Hanna, J. R., and Schacter, D. L. (2008). The brain’s default network: anatomy, function, and relevance to disease. Ann. N. Y. Acad. Sci. 1124, 1–38. doi: 10.1196/annals.1440.011
Cabeza, R., Dolcos, F., Graham, R., and Nyberg, L. (2002). Similarities and differences in the neural correlates of episodic memory retrieval and working memory. NeuroImage 16, 317–330. doi: 10.1006/nimg.2002.1063
CADTH, (2016). Aripiprazole (Abilify): Depression, Major Depressive Disorder (MDD). Ottawa (ON):Canadian Agency for Drugs and Technologies in Health.
Cavanna, A. E., and Trimble, M. R. (2006). The precuneus: a review of its functional anatomy and behavioural correlates. Brain 129, 564–583. doi: 10.1093/brain/awl004
Chen, T., Yang, M., Liu, B., Liu, Y. T., Zhang, H. X., Liu, C. C., et al. (2016). The resting-state functional connectivity of the default mode networks in patients with obstructive sleep apnea-hypopnea syndrome. CNS Neurol. Disord. Drug Targets
Chen, T., Zhao, W., Zhang, Y., Yu, J., Wang, T., Zhang, J., et al. (2022). Neural mechanism of the relationship between sleep efficiency and clinical improvement in major depressive disorder: a longitudinal functional magnetic resonance imaging study. Front. Psych. 13:1027141. doi: 10.3389/fpsyt.2022.1027141
Chao-Gan, Y., and Yu-Feng, Z. (2010). DPARSF: a MATLAB toolbox for “pipeline” data analysis of resting-state fMRI. Front. Syst. Neurosci. 4:13. doi: 10.3389/fnsys.2010.00013
Clark, L., Chamberlain, S. R., and Sahakian, B. J. (2009). Neurocognitive mechanisms in depression: implications for treatment. Annu. Rev. Neurosci. 32, 57–74. doi: 10.1146/annurev.neuro.31.060407.125618
Dai, D., Lacadie, C. M., Holmes, S. E., Cool, R., Anticevic, A., Averill, C., et al. (2020). Ketamine normalizes the structural alterations of inferior frontal gyrus in depression. Chronic Stress 4, 1–10. doi: 10.1177/2470547020980681
Desseilles, M., Balteau, E., Sterpenich, V., Dang-Vu, T. T., Darsaud, A., Vandewalle, G., et al. (2009). Abnormal neural filtering of irrelevant visual information in depression. J. Neurosci. 29, 1395–1403. doi: 10.1523/JNEUROSCI.3341-08.2009
Disner, S. G., Beevers, C. G., Haigh, E. A., Beevers, C. G., Haigh, E. A., and Beck, A. T. (2011). Neural mechanisms of the cognitive model of depression. Nat. Rev. Neurosci. 12, 467–477. doi: 10.1038/nrn3027
Dowlati, Y., Herrmann, N., Swardfager, W., Liu, H., Sham, L., Reim, E. K., et al. (2010). A meta-analysis of cytokines in major depression. Biol. Psychiatry 67, 446–457. doi: 10.1016/j.biopsych.2009.09.033
Dubin, M. J., Mao, X., Banerjee, S., Goodman, Z., Lapidus, K. A. B., Kang, G., et al. (2016). Elevated prefrontal cortex GABA in patients with major depressive disorder after TMS treatment measured with proton magnetic resonance spectroscopy. J. Psychiatry Neurosci. 41, E37–E45. doi: 10.1503/jpn.150223
Fang, H., Tu, S., Sheng, J., and Shao, A. (2019). Depression in sleep disturbance: a review on a bidirectional relationship, mechanisms and treatment. J. Cell. Mol. Med. 23, 2324–2332. doi: 10.1111/jcmm.14170
Foland-Ross, L. C., and Gotlib, I. H. (2012). Cognitive and neural aspects of information processing in major depressive disorder: an integrative perspective. Front. Psychol. 3:489. doi: 10.3389/fpsyg.2012.00489
Fox, M. D., and Raichle, M. E. (2007). Spontaneous fluctuations in brain activity observed with functional magnetic resonance imaging. Nat. Rev. Neurosci. 8, 700–711. doi: 10.1038/nrn2201
Friedrich, M. J. (2017). Depression is the leading cause of disability around the world. JAMA 317:1517. doi: 10.1001/jama.2017.3828
Frodl, T. S., Koutsouleris, N., Bottlender, R., Born, C., Jäger, M., Scupin, I., et al. (2008). Depression-related variation in brain morphology over 3 years: effects of stress? Arch. Gen. Psychiatry 65, 1156–1165. doi: 10.1001/archpsyc.65.10.1156
Furey, M. L., Drevets, W. C., Hoffman, E. M., Frankel, E., Speer, A. M., and Zarate, C. A. (2013). Potential of pretreatment neural activity in the visual cortex during emotional processing to predict treatment response to scopolamine in major depressive disorder. JAMA Psychiat. 70, 280–290. doi: 10.1001/2013.jamapsychiatry.60
Goldberger, C., Guelfi, J. D., and Sheehan, D. V. (2011). Assessment of anxiety in clinical trials with depressed patients using the Hamilton depression rating scale. Psychopharmacol. Bull. 44, 34–50.
Gong, J., Wang, J., Qiu, S., Chen, P., Luo, Z., Wang, J., et al. (2020). Common and distinct patterns of intrinsic brain activity alterations in major depression and bipolar disorder: voxel-based meta-analysis. Transl. Psychiatry 10:353. doi: 10.1038/s41398-020-01036-5
Gong, L., Yu, S., Xu, R., Liu, D., Dai, X., Wang, Z., et al. (2021). The abnormal reward network associated with insomnia severity and depression in chronic insomnia disorder. Brain Imaging Behav. 15, 1033–1042. doi: 10.1007/s11682-020-00310-w
Guo, W. B., Liu, F., Xue, Z. M., Xu, X. J., Wu, R. R., Ma, C. Q., et al. (2012). Alterations of the amplitude of low-frequency fluctuations in treatment-resistant and treatment-response depression: a resting-state fMRI study. Prog. Neuro-Psychopharmacol. Biol. Psychiatry 37, 153–160. doi: 10.1016/j.pnpbp.2012.01.011
Gusnard, D. A., Akbudak, E., Shulman, G. L., and Raichle, M. E. (2001a). Medial prefrontal cortex and self-referential mental activity: relation to a default mode of brain function. Proc. Natl. Acad. Sci. U. S. A. 98, 4259–4264. doi: 10.1073/pnas.071043098
Gusnard, D. A., Raichle, M. E., and Raichle, M. E. (2001b). Searching for a baseline: functional imaging and the resting human brain. Nat. Rev. Neurosci. 2, 685–694. doi: 10.1038/35094500
Hashimoto, K., Sawa, A., and Iyo, M. (2007). Increased levels of glutamate in brains from patients with mood disorders. Biol. Psychiatry 62, 1310–1316. doi: 10.1016/j.biopsych.2007.03.017
Irwin, M. R., Wang, M., Campomayor, C. O., Collado-Hidalgo, A., and Cole, S. (2006). Sleep deprivation and activation of morning levels of cellular and genomic markers of inflammation. Arch. Intern. Med. 166, 1756–1762. doi: 10.1001/archinte.166.16.1756
Irwin, M. R., Carrillo, C., and Olmstead, R. (2010). Sleep loss activates cellular markers of inflammation: sex differences. Brain Behav. Immun. 24, 54–57. doi: 10.1016/j.bbi.2009.06.001
Jastorff, J., De Winter, F. L., Van den Stock, J., Vandenberghe, R., Giese, M. A., and Vandenbulcke, M. (2016). Functional dissociation between anterior temporal lobe and inferior frontal gyrus in the processing of dynamic body expressions: insights from behavioral variant frontotemporal dementia. Hum. Brain Mapp. 37, 4472–4486. doi: 10.1002/hbm.23322
Jaussent, I., Bouyer, J., Ancelin, M. L., Akbaraly, T., Peres, K., Ritchie, K., et al. (2011). Insomnia and daytime sleepiness are risk factors for depressive symptoms in the elderly. Sleep 34, 1103–1110. doi: 10.5665/SLEEP.1170
Julian, L. J. (2011). Measures of anxiety: state-trait anxiety inventory (STAI), Beck anxiety inventory (BAI), and hospital anxiety and depression scale-anxiety (HADS-A). Arthritis Care Res. 63 0 11, S467–S472. doi: 10.1002/acr.20561
Kandilarova, S., Stoyanov, D., Sirakov, N., Maes, M., and Specht, K. (2019). Reduced grey matter volume in frontal and temporal areas in depression: contributions from voxel-based morphometry study. Acta Neuropsychiatr. 31, 252–257. doi: 10.1017/neu.2019.20
Koster, E. H., Fox, E., and Macleod, C. (2009). Introduction to the special section on cognitive bias modification in emotional disorders. J. Abnorm. Psychol. 118, 1–4. doi: 10.1037/a0014379
Leerssen, J., Blanken, T. F., Pozzi, E., Jahanshad, N., Aftanas, L., Andreassen, O. A., et al. (2020). Brain structural correlates of insomnia severity in 1053 individuals with major depressive disorder: results from the ENIGMA MDD working group. Transl. Psychiatry 10:425. doi: 10.1038/s41398-020-01109-5
Lee, J. S., Kang, W., Kang, Y., Kim, A., Han, K. M., Tae, W. S., et al. (2021). Alterations in the occipital cortex of drug-Naïve adults with major depressive disorder: a surface-based analysis of surface area and cortical thickness. Psychiatry Investig. 18, 1025–1033. doi: 10.30773/pi.2021.0099
Lee, Y., Son, H., Kim, G., Kim, S., Lee, D. H., Roh, G. S., et al. (2013). Glutamine deficiency in the prefrontal cortex increases depressive-like behaviours in male mice. J. Psychiatry Neurosci. 38, 183–191. doi: 10.1503/jpn.120024
Levinson, A. J., Fitzgerald, P. B., Favalli, G., Blumberger, D. M., Daigle, M., and Daskalakis, Z. J. (2010). Evidence of cortical inhibitory deficits in major depressive disorder. Biol. Psychiatry 67, 458–464. doi: 10.1016/j.biopsych.2009.09.025
Lind, M. J., Aggen, S. H., Kirkpatrick, R. M., Kendler, K. S., and Amstadter, A. B. (2015). A longitudinal twin study of insomnia symptoms in adults. Sleep 38, 1423–1430. doi: 10.5665/sleep.4982
Lind, M. J., Hawn, S. E., Sheerin, C. M., Aggen, S. H., Kirkpatrick, R. M., Kendler, K. S., et al. (2017). An examination of the etiologic overlap between the genetic and environmental influences on insomnia and common psychopathology. Depress. Anxiety 34, 453–462. doi: 10.1002/da.22587
Liu, C. H., Guo, J., Lu, S. L., Tang, L. R., Fan, J., Wang, C. Y., et al. (2018). Increased salience network activity in patients with insomnia complaints in major depressive disorder. Front. Psych. 9:93. doi: 10.3389/fpsyt.2018.00093
Liu, X., Yan, Z., Wang, T., Yang, X., Feng, F., Fan, L., et al. (2015). Connectivity pattern differences bilaterally in the cerebellum posterior lobe in healthy subjects after normal sleep and sleep deprivation: a resting-state functional MRI study. Neuropsychiatr. Dis. Treat. 11, 1279–1289. doi: 10.2147/NDT.S84204
Lovato, N., and Gradisar, M. (2014). A meta-analysis and model of the relationship between sleep and depression in adolescents: recommendations for future research and clinical practice. Sleep Med. Rev. 18, 521–529. doi: 10.1016/j.smrv.2014.03.006
Lundstrom, B. N., Ingvar, M., and Petersson, K. M. (2005). The role of precuneus and left inferior frontal cortex during source memory episodic retrieval. NeuroImage 27, 824–834. doi: 10.1016/j.neuroimage.2005.05.008
Luo, Y., Qiao, M., Liang, Y., Chen, C., Zeng, L., Wang, L., et al. (2022). Functional brain connectivity in mild cognitive impairment with sleep disorders: a study based on resting-state functional magnetic resonance imaging. Front. Aging Neurosci. 14:812664. doi: 10.3389/fnagi.2022.812664
Manber, R., Edinger, J. D., Gress, J. L., Pedro-Salcedo, M. G. S., Kuo, T. F., and Kalista, T. (2008). Cognitive behavioral therapy for insomnia enhances depression outcome in patients with comorbid major depressive disorder and insomnia. Sleep 31, 489–495. doi: 10.1093/sleep/31.4.489
Mason, M. F., Norton, M. I., Van Horn, J. D., Wegner, D. M., Grafton, S. T., and Macrae, C. N. (2007). Wandering minds: the default network and stimulus-independent thought. Science 315, 393–395. doi: 10.1126/science.1131295
Monroe, S. M., and Harkness, K. L. (2011). Recurrence in major depression: a conceptual analysis. Psychol. Rev. 118, 655–674. doi: 10.1037/a0025190
Na, K. S., Won, E., Kang, J., Chang, H. S., Yoon, H. K., Tae, W. S., et al. (2016). Brain-derived neurotrophic factor promoter methylation and cortical thickness in recurrent major depressive disorder. Sci. Rep. 6:21089. doi: 10.1038/srep21089
Price, R. B., Shungu, D. C., Mao, X., Nestadt, P., Kelly, C., Collins, K. A., et al. (2009). Amino acid neurotransmitters assessed by proton magnetic resonance spectroscopy: relationship to treatment resistance in major depressive disorder. Biol. Psychiatry 65, 792–800. doi: 10.1016/j.biopsych.2008.10.025
Raison, C. L., Rutherford, R. E., Woolwine, B. J., Shuo, C., Schettler, P., Drake, D. F., et al. (2013). A randomized controlled trial of the tumor necrosis factor antagonist infliximab for treatment-resistant depression: the role of baseline inflammatory biomarkers. JAMA Psychiat. 70, 31–41. doi: 10.1001/2013.jamapsychiatry.4
Shi, Y., Zhang, L., He, C., Yin, Y., Song, R., Chen, S., et al. (2021). Sleep disturbance-related neuroimaging features as potential biomarkers for the diagnosis of major depressive disorder: a multicenter study based on machine learning. J. Affect. Disord. 295, 148–155. doi: 10.1016/j.jad.2021.08.027
Simpson, J. J., Drevets, W. C., Snyder, A. Z., Gusnard, D. A., and Raichle, M. E. (2001). Emotion-induced changes in human medial prefrontal cortex: II. During anticipatory anxiety. Proc. Natl. Acad. Sci. U. S. A. 98, 688–693. doi: 10.1073/pnas.98.2.688
Spiegel, D., Lewis-Fernández, R., Lanius, R., Vermetten, E., Simeon, D., and Friedman, M. (2013). Dissociative disorders in DSM-5. Annu. Rev. Clin. Psychol. 9, 299–326. doi: 10.1146/annurev-clinpsy-050212-185531
Tao, H., Guo, S., Ge, T., Kendrick, K. M., Xue, Z., Liu, Z., et al. (2013). Depression uncouples brain hate circuit. Mol. Psychiatry 18, 101–111. doi: 10.1038/mp.2011.127
Teng, C., Zhou, J., Ma, H., Tan, Y., Wu, X., Guan, C., et al. (2018). Abnormal resting state activity of left middle occipital gyrus and its functional connectivity in female patients with major depressive disorder. BMC Psychiatry 18:370. doi: 10.1186/s12888-018-1955-9
Tsuno, N., Besset, A., and Ritchie, K. (2005). Sleep and depression. J. Clin. Psychiatry 66, 1254–1269. doi: 10.4088/JCP.v66n1008
Urgesi, C., Mattiassi, A. D., Buiatti, T., Mattiassi, A. D., Buiatti, T., and Marini, A. (2016). Tell it to a child! A brain stimulation study of the role of left inferior frontal gyrus in emotion regulation during storytelling. NeuroImage 136, 26–36. doi: 10.1016/j.neuroimage.2016.05.039
Vollrath, M, and Wicki, W, Angst J. The Zurich study. VIII,. (1989) Insomnia: association with depression, anxiety, somatic syndromes, and course of insomnia. Eur. Arch. Psychiatry Neurol. Sci. 239, 113–124. doi: 10.1007/BF01759584
Wilson, C. R., Gaffan, D., Browning, P. G., and Baxter, M. G. (2010). Functional localization within the prefrontal cortex: missing the forest for the trees? Trends Neurosci. 33, 533–540. doi: 10.1016/j.tins.2010.08.001
Zhang, X., Tang, Y., Maletic-Savatic, M., Sheng, J., Zhang, X., Zhu, Y., et al. (2016). Altered neuronal spontaneous activity correlates with glutamate concentration in medial prefrontal cortex of major depressed females: an fMRI-MRS study. J. Affect. Disord. 201, 153–161. doi: 10.1016/j.jad.2016.05.014
Zhao, Y., Chen, L., Zhang, W., Xiao, Y., Shah, C., Zhu, H., et al. (2017). Gray matter abnormalities in non-comorbid medication-naive patients with major depressive disorder or social anxiety disorder. EBioMedicine 21, 228–235. doi: 10.1016/j.ebiom.2017.06.013
Zhuo, C., Li, G., Lin, X., Jiang, D., Xu, Y., Tian, H., et al. (2019). The rise and fall of MRI studies in major depressive disorder. Transl. Psychiatry 9:335. doi: 10.1038/s41398-019-0680-6
Keywords: fractional amplitude of low-frequency fluctuation, major depressive diorders, sleep disturbance, MRI, correlation
Citation: Zheng N, Ou Y, Li H, Liu F, Xie G, Li P, Lang B and Guo W (2023) Shared and differential fractional amplitude of low-frequency fluctuation patterns at rest in major depressive disorders with or without sleep disturbance. Front. Psychol. 14:1153335. doi: 10.3389/fpsyg.2023.1153335
Received: 29 January 2023; Accepted: 01 March 2023;
Published: 22 March 2023.
Edited by:
Ranji Cui, Second Affiliated Hospital of Jilin University, ChinaReviewed by:
Jinhong Meng, University College London, United KingdomCopyright © 2023 Zheng, Ou, Li, Liu, Xie, Li, Lang and Guo. This is an open-access article distributed under the terms of the Creative Commons Attribution License (CC BY). The use, distribution or reproduction in other forums is permitted, provided the original author(s) and the copyright owner(s) are credited and that the original publication in this journal is cited, in accordance with accepted academic practice. No use, distribution or reproduction is permitted which does not comply with these terms.
*Correspondence: Bing Lang, YmluZy5sYW5nQGNzdS5lZHUuY24=; Wenbin Guo, Z3Vvd2VuYmluNzZAY3N1LmVkdS5jbg==
†These authors have contributed equally to this work
Disclaimer: All claims expressed in this article are solely those of the authors and do not necessarily represent those of their affiliated organizations, or those of the publisher, the editors and the reviewers. Any product that may be evaluated in this article or claim that may be made by its manufacturer is not guaranteed or endorsed by the publisher.
Research integrity at Frontiers
Learn more about the work of our research integrity team to safeguard the quality of each article we publish.