- Department of Psychology, University of Alberta, Edmonton, AB, Canada
Introduction: The concept of animacy is often taken as a basic natural concept, in part I because most cases seem unambiguous. Most entities either are or are not animate. However, human animacy judgments do not reflect this binary classification. They suggest that there are borderline cases, such as virus, amoeba, fly, and imaginary beings (giant, dragon, god). Moreover, human roles (professor, mother, girlfriend) are consistently recognized as animate by far less than 100% of human judges.
Method: In this paper, I use computational modeling to identify features associated with human animacy judgments, modeling human animacy and living/non-living judgments using both bottom-up predictors (the principal components from a word embedding model) and top-down predictors (cosine distances from the names of animate categories).
Results: The results suggest that human animacy judgments may be relying on information obtained from imperfect estimates of category membership that are reflected in the word embedding models. Models using cosine distance from category names mirror human judgments in distinguishing strongly between humans (estimated lower animacy by the measure) and other animals (estimated higher animacy by the measure).
Discussion: These results are consistent with a family resemblance approach to the apparently categorical concept of animacy.
1. Introduction
The word animacy is defined in the Oxford English Dictionary (2022) as “The quality or condition of being alive or animate; animate existence; an instance of this.” This definition seems clear and unambiguous on its surface. However, when humans are asked to make judgments of animacy, they identify many intermediate or anomalous cases. The goal of the present paper is to use computational modeling to shed light on the lack of unanimous binary animacy decisions by English speakers for many words, by modeling the decisions for the 72 words rated for animacy in Radanović et al. (2016) and for 1,200 English words rated living/non-living from VanArsdall and Blunt (2022). I will consider two models with different set of predictors and synthesize their contributions to the understanding human animacy judgments at the end, by considering whether and why the models make the same kinds of errors that humans do.
As examples of the lack of agreement in animacy ratings, Radanović et al. (2016) reported that their university-student judges rated the animacy of giraffes or babies at about 50 (out of 100, where 0 = inanimate and 100 = animate), though we would normally think of the default state of these entities as living. This is approximately the same as the average ratings for balls (49.2) or snow (51.0), though we would not think of these entities as being alive. Other intermediate cases include imaginary beings such as ghosts (rated 41.7) and fairies (49.4); entities that imitate animate entities such as computers (52.2) and robots (33.1); and simple creatures such as amoebae (83.5) and viruses (69.4). Plants are a potentially ambiguous intermediate case, since they are animate by the Oxford English Dictionary’s definition, but we often interact with them in inanimate form. This may explain the lack of strong consensus about animacy in the ratings of words referring to plants such as cabbage (59.0), tomato (38.9), and orchid (59.0).
Languages that mark animacy grammatically can add additional complications within specific cultures. For example, in Cree, animal hides, trees (but not pieces of wood), and some (but not all) stones are marked grammatically as animate, perhaps (as suggested by Darnell and Vanek, 1976) reflecting that in Cree “a thing is classified as animate if it has power” (p. 164).
The role of animacy in semantic and lexical processing has been the focus of many studies (e.g., Cappa et al., 1998; Caramazza and Shelton, 1998; Grabowski et al., 1998; Mummery et al., 1998; Moore and Price, 1999; Tyler et al., 2000; Tyler and Moss, 2001; Radanović et al., 2016). Some studies have reported behavioral and/or neurological differences in response to animate and inanimate stimuli (Perani et al., 1995; Martin et al., 1996; Perani et al., 1999). Other studies have failed to replicate these findings (Devlin et al., 2002; Pilgrim et al., 2002; Tyler et al., 2003; Ilić et al., 2013). The linguistic encoding of animacy has been shown to affect many different aspects of psychological functioning, including the processing of relative clauses (Mak et al., 2002; Traxler et al., 2005; Gennari et al., 2012); attentional mechanisms (Bugaiska et al., 2019); the detection of semantic violations in language (Grewe et al., 2006; Szewczyk and Schriefers, 2011); the learning of artificial languages (Vihman et al., 2018); word recognition (Bonin et al., 2019) and the ability to recall words (Bonin et al., 2015; VanArsdall et al., 2015; Bugaiska et al., 2016; Popp and Serra, 2016, 2018; Nairne et al., 2017; Kazanas et al., 2020).
As Radanović et al. (2016) noted, one complication in studies using animacy is how stimuli are selected. Some studies have focused on only a few exemplars (i.e., tools versus animals, as in Perani et al., 1995, 1999; Martin et al., 1996). Others including a wider range of animate and inanimate stimuli.
Animacy ratings have been gathered in many languages (e.g., Serbian/English: Radanović et al., 2016; Portuguese: Félix et al., 2020; Persian: Mahjoubnavaz and Mokhtari, 2022; English: VanArsdall and Blunt, 2022). This study focuses on the two sets of English ratings in this list.
The first set was the set of 72 ratings from Radanović et al. (2016). As noted above, these were rated from 1 (inanimate) to 100 (animate). The authors reported that the English ratings were strongly correlated with independent Serbian ratings of the same words (r = 0.89, p < 0.001). They included a wide range of words. The ratings are summarized by into categories in Table 1. There is notable variation in ratings within categories of animate things. Contrary to the some claims (see discussion in Radanović et al., 2016, p. 17) human beings are rated as lower in animacy (Average [SD] rating: 60.0 [16.6]) than other animals (Average [SD] rating: 79.8 [22.6]; t(14.39) = 2.18; p = 0.046). Since human beings are certainly animate, this result is puzzling. I will consider it again in the conclusion section of this paper.
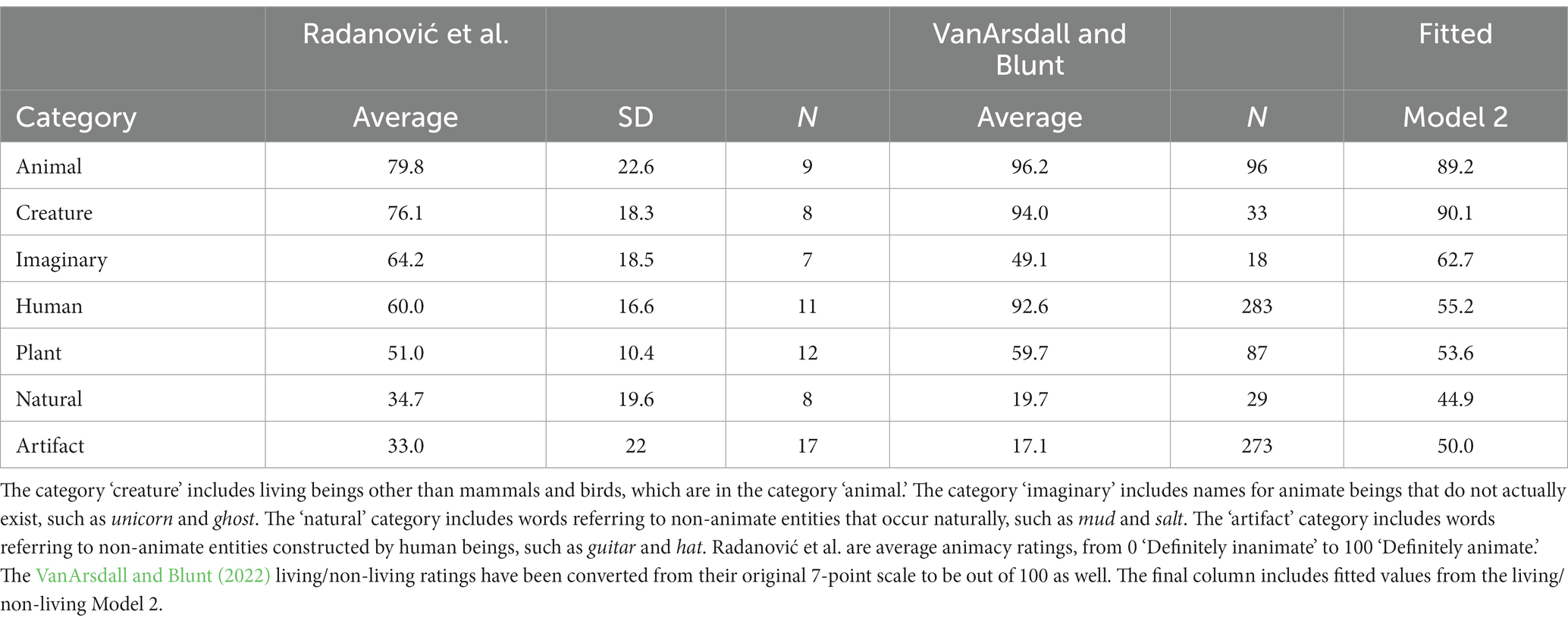
Table 1. Animacy ratings from Radanović et al. (2016), living/non-living ratings from VanArsdall and Blunt (2022), and model estimates of the latter, by category, ordered from most animate to least animate by human rating.
The other set is the recently released set of ratings from VanArsdall and Blunt (2022). They gathered living/non-living ratings from 1 to 7 for 1,200 English words. Each word was rated a minimum of 19 times (average [SD]: 25 [1.62]). The ratings are also summarized by category in Table 1.
These two sets of ratings are along slightly different dimensions. Some things that are clearly non-living (for example, unicorns and Santa Claus) might reasonably be judged animate. However, the larger set of ratings makes it possible to cross-validate the models, which is not possible with the small number of ratings from Radanović et al. (2016). Moreover, the ratings are correlated. The 50 words that appear in both data sets have animacy and living/non-living ratings that correlate at r = 0.60 (p < 0.0001).
The models use two different sources of data, to allow us to consider the issue from both a bottom-up perspective (to what degree is animacy encoded in semantics/patterns of language use?) and a top-down perspective (to what degree is animacy determined by membership in categories of animate entities?). One model uses the principal components of vector representations of words from a word embedding model (explained in more detail in the next section) to try to predict human ratings. This can give us an idea of the extent which animacy is encoded into language use, a bottom-up approach to animacy. The second model uses the similarity of a word’s vector to the vector of the names of definitely animate categories such as human, animal, and plant. This can give us an idea of the extent to which animacy is derivable from the goodness of its categorical membership. For example, though they are animals, humans are generally considered to be poor representatives of that class. It is possible that this is why humans are less likely to be judged as animate than other animals.
2. Model 1: introduction
The first model uses generalized additive models (GAMs) across the principal components (PCs) from a word-embedding model to predict human judgments. GAMs are able to capture non-linear relationships between predictors and a dependent measure but can also find linear relationships when they are the best fit for the data.
Word-embedding models are computational models that build vector representations of individual words that represent the average context in which that word appears in a large corpus of language. Perhaps the simplest way to do this is that used in the earliest model, Landauer and Dumais’s (1997) Latent Semantic Analysis (LSA), which built a word x document matrix in which the individual cells (prior to processing the matrix with singular value decomposition to reduce the dimensionality) recorded how often each word (rows) appeared in each document (columns). Since documents almost always have a semantic focus (they are usually about something), we might reasonably expect that words whose untransformed vectors were similar (say, vectors for the words pet and cat) are words that have similar semantics. Importantly, LSA does not directly measure whether cat and pet occur together in the same documents, which is what we call first-order co-occurrence. It measures whether the documents in which cat and pet appeared tended to contain the same words (a comparison of word context that we call second-order co-occurrence). It is possible for two words to have highly similar LSA vectors without ever appearing in the same document. For example, one can easily imagine that in some set of documents the informal word cat and the more formal word feline might never appear in the same document, but nevertheless would be likely to occur in documents that share many other words.
The basic principle of constructing vector representations of a word’s context continues in more recent word-embedding models, but the methods of constructing the vectors have been refined. There are two main differences. One difference is that most contemporary models do not construct their vectors from co-occurrence within documents, but rather from co-occurrence within some smaller moving window of text (which may be conceived of as very tiny documents, to keep the analogy with LSA precise). The second difference is that contemporary models do not merely count words but rather use more sophisticated computational methods to build the context vectors. In this paper I used a model called word2vec (Mikolov et al., 2013a,b,c). Skipping over some minor computational complications, word2vec models use a neural network with a single hidden layer (which is what is used as the vector representation of the word) to either predict the context of a target word (called CBOW, for continuous bag of words) or the inverse: to use context to try to predict a target word (skipgram, because the target word has been ‘skipped’ with context on either side). This paper uses the skipgram model with a 300-unit hidden layer and a context defined as two words on either side of the target word. Although these parameters are arbitrary, these values are commonly used in language research. For a corpus, I used the 150,000 most frequent words from a 100 billion words subset of the Google news corpus.1 To increase the chances that the results might have a clear interpretation, I applied principal components analysis (PCA) to this matrix, retaining all 300 principal components (PCs). The magnitude of the PC can thereby give us an estimate of how much variance in the matrix is accounted for.
Four words of the 1,200 words from VanArsdall and Blunt (2022) were eliminated from this study. The word bluejay was eliminated because it is confounded with the name of Canada’s favorite baseball team and appears only in capitalized form in the Google news corpus. The word is also problematic since the name of the bird is normally not considered a compound word but is rather composed of two words. Similarly, the word hornet appeared in the Google news corpus only in capitalized form (though it contained the plural form hornets), presumably referring to the name of the Marvel comic character. The word ghoul did not appear in the corpus, although ghouls did. The word sphinx did appear in the corpus, but only in capitalized form. The remaining dataset was randomly split into two sets of 598 words, with one half used for model development, and the other for cross-validation.
2.1. Model 1: method
All reported analyses were conducted in R 4.2.2 (R Core Team, 2022) using R Studio (2022.12.0 + 353; Posit Software, 2022) for macOS. The GAMs were analyzed using the mgcv package (v. 1.8–41, Wood, 2022; see also Wood, 2017).
Ninety-seven of the 300 PCs were significantly (p < = 0.05) correlated by GAM (i.e., possibly non-linearly) with the human animacy ratings from Radanović et al. (2016). This included 13 of the first 20 PCs (but not PC1). Since this provided more reliable predictors than there are data points, I used the 21 PCs that had a GAM whose output correlated with the human estimates at p < = 0.001 to construct a full GAM model. All predictors were entered initially. Those with the highest value of p were removed one by one until all remaining predictors entered with p < 0.05.
Ninety-two of the of the 300 PCs were significantly (p < = 0.05) correlated by GAM with the human living/non-living ratings from VanArsdall and Blunt (2022). This also provided more predictors than datapoints, because each smooth in the GAM has nine parameters using the default rank value (number of possible turning points, or knots) of 10 (the tenth is eliminated by centering the predictors). I therefore used the same method as above, initially entering only the 29 PCs that had a GAM whose output correlated with the human judgments at p < = 0.001. This included four of the first 20 PCs, but again, not PC1.
2.2. Model 1: result
Only two PCs entered the model of the animacy ratings: PC123 and PC246. Together these PCs accounted for 56.6% of the variance in those ratings (p < 0.00001; see Figure 1).
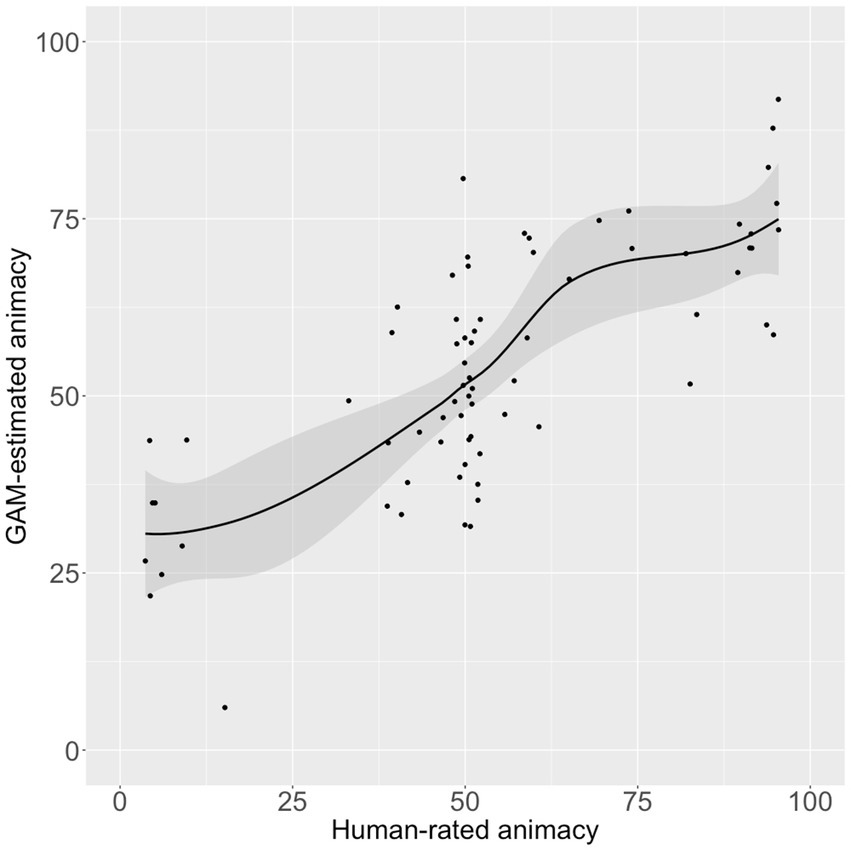
Figure 1. Human-rated (X-axis) versus GAM-estimated (Y-axis) animacy, using word2vec PCs as predictors. The gray-shaded area is the 95% confidence interval.
I constructed a dictionary by taking the 75,000 most frequent words from Shaoul and Westbury, 2006. I eliminated words that did not appear in the Google news matrix, which does not include closed class words, as well as compounds words (or phrases) with spaces in them. The final dictionary contains 67,717 words. Applying the GAM to this dictionary suggested that the model may be over-fit to the small data set, since the words estimated most highly animate were not clearly exemplars of any animate category. The top 10 words were disclaims, threes, clientless, fouling, republication, desegregation, effigies, barriers, reflate, and mineralization.
Four PCs entered the living/non-living model: PC30, PC138, PC248, and PC225 (see Table 2). Together these PCs accounted for 12.5% of the variance in the human ratings (p < 0.00001). The model did not cross-validate successfully. Its predictions were unreliably correlated (r = −0.02, p = 0.61) with the human living/non-living ratings in the validation set.
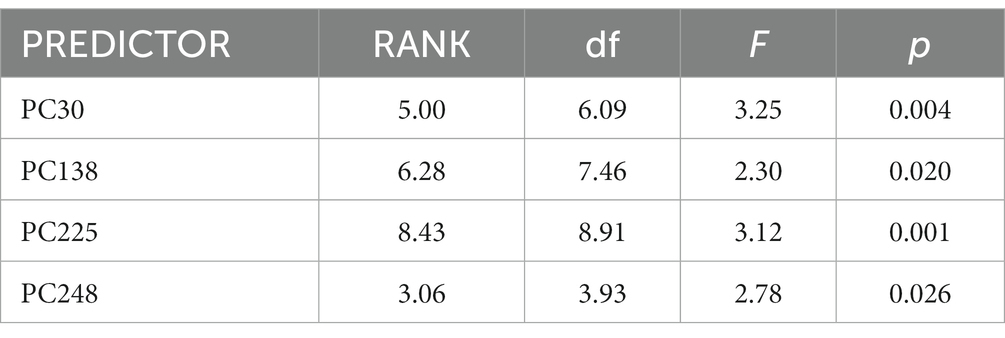
Table 2. Best GAM model to predict the living/non-living ratings from VanArsdall and Blunt (2022), using word-embedding PCs as predictors.
2.3. Model 1: discussion
Although the models did not generalize well to the full dictionary or to a validation dataset, we can draw some tentative conclusions from this initial model.
The lack of good generalization and the lack of concordance between the two models suggests that one conclusion we can draw is that little of the variance in animacy or living/non-living judgments can be derived from the PCs in a word embedding model. The failure of these ‘bottom-up’ models suggests that animacy or being alive are not strongly encoded in patterns of word use. More speculatively, we can conclude that animacy is not a basic component of lexical semantics, since many components considered to be basic can be well-estimated from the PCs e.g., (see Hollis and Westbury, 2016; Hollis et al., 2017; Westbury and Hollis, 2019).
However, that said, the second conclusion is that animacy may be correlated with other aspects of semantics, since a large number of individual PC GAMs produced estimates that were reliably correlated with the human animacy ratings. The Radanović et al. (2016) are reliably correlated with the extrapolated estimates of human judgments of valence, dominance, and arousal from Hollis et al. (2017). Higher animacy ratings are associated with lower valence (r = −0.29, p = 0.01), higher arousal (r = 0.35, p = 0.003), and lower dominance (r = −0.33, p = 0.005). The negative correlation with dominance reflects the fact that many small (i.e., low dominance) living things such as amoeba (83/100), bacteria (83/100), squirrel (95/100), worm (92/100), and spider (94/100) receive high animacy ratings. The animacy ratings are also positively correlated (r = 0.31, p = 0.007) with the measure of self-relevance (how strongly a word is associated with the first-person singular word I) that was defined in Westbury and Wurm (2022), where it was shown to strong predictor of the value of early PC values across a large dictionary. A GAM developed with all these values to predict the animacy ratings allowed in only arousal and self-relevance with p < 0.05. Together these two measures accounted for 21.2% of the variance in the ratings.
A third conclusion is that (tautologically) most of the error in predicting animacy is seen for words of ambiguous animacy. In Figure 1 there is a wide range of model estimates for words that were rated the mid-range of animacy by humans in Radanović et al. (2016).
3. Model 2: introduction
Word2vec vectors for category names (such as the vector representing the word animal) usually (though not necessarily) serve as centroids for the category they name. This means that words with vectors that are similar (by cosine distance) to the vector for a category name are often members of that category. For example, the twenty vectors most similar to the vector of the word vegetable are the vectors for the words tomato, potato, tomatoes, broccoli, sweet_potato, onion, onions, cauliflower, mango (oops!), kale, potatoes, mangos, cabbage, and melons. We may perhaps forgive the model for sometimes confusing vegetables and fruit, since we ourselves routinely refer to the tomato fruit, the avocado fruit, the olive fruit, the cucumber fruit, the zucchini fruit, and several other fruits (strictly speaking, plants in which the edible part develops from a flower) as vegetables. If humans discuss fruits as if they were vegetables, we must expect that word embedding models will reflect that. Of course, fruits and vegetables also do correctly both belong to many other categories: plant products, things we eat, things that cannot thrive in freezing weather, domesticated products, things you will find at the grocery store, everyday objects, things that can be composted, and so on. A word-embedding model of categorization may be influenced by all these categories simultaneously since it can only induce the categories from the similarity of the contexts of words as encoded in the words’ vectors. It is possible that a super-ordinate category could be better captured by patterns of word use than a more focal category, if people used language in a way that better reflected that super-ordinate category.
In the second model, this categorizing feature of word embedding models is used, by building models of the human ratings based on the distance from the vectors of the names of categories of unambiguously animate things.
3.1. Model 2: method
For predictors I used the cosine similarity of each word that had been classified by humans to five main category names of definitely animate and living things: plant, animal, insect, human, and bacteria. Of the five taxonomic kingdoms, three are captured by these categories (plant, animal, and bacteria/~monera). The other two (funghi and protista) are less relevant kingdoms when it comes to animacy. Insects and humans are broken out of the animalia kingdom to which they belong because they are regularly incorrectly classified as non-animate.
3.2. Model 2: results
The Pearson correlations between all the predictors and the ratings from Radanović et al. (2016) are shown in Figure 2. The correlations between the human ratings for each word and the cosine distance of their word2vec vectors from the vectors of the category labels were reliable at p < 0.001 for all categories except plant (r = 0.13, p > 0.05).
The best GAM model to predict the Radanović et al. (2016) ratings included only two predictors that entered with p < 0.05, insect and human. This model is summarized in Table 3. It accounted for 28.8% of the variance in the human ratings. The relationship between the predictors and the model estimates for are shown graphically in Figure 3.
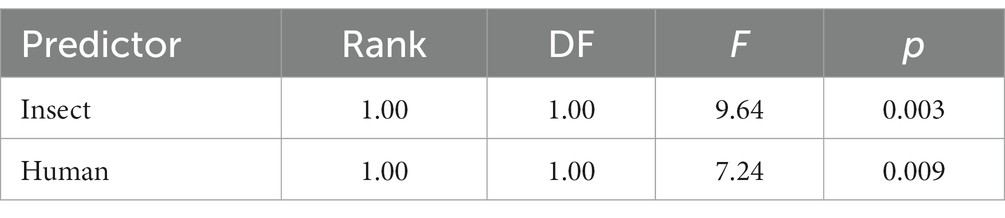
Table 3. Best GAM model to predict the animacy ratings from Radanović et al. (2016), using cosine distance to category label vectors as predictors.
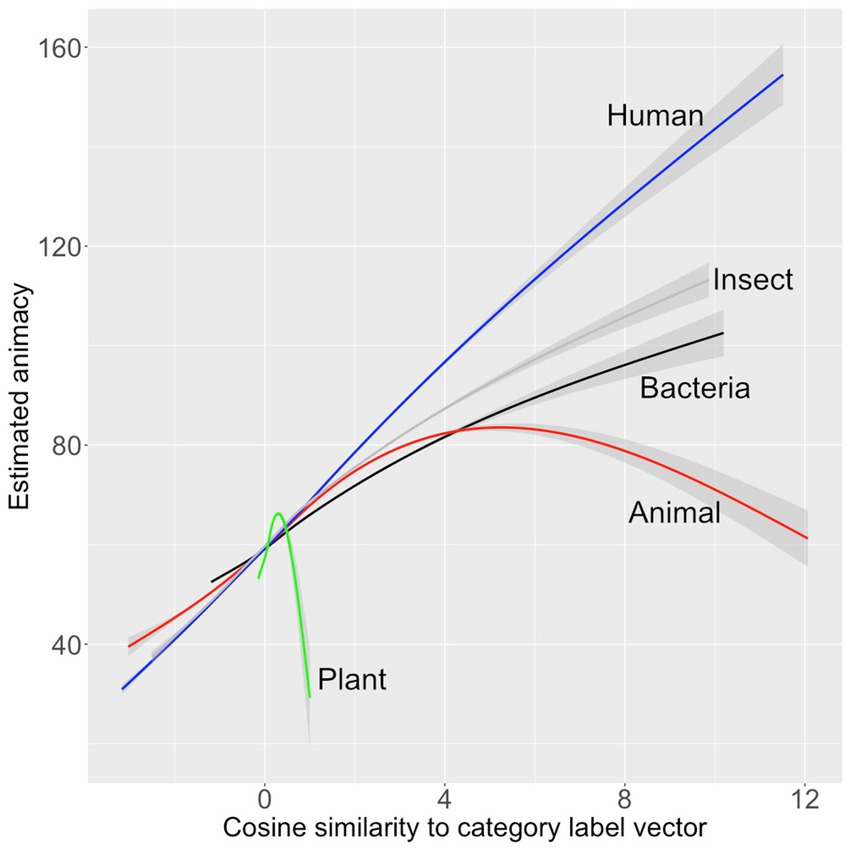
Figure 3. Model 2’s estimates of animacy (y-axis) graphed against the normalized cosine similarity of the vectors for the category labels of 67,717 words (x-axis) The gray-shaded area is the 95% confidence interval.
When the model was applied to the full dictionary, the 10 words estimated most animate were almost all insects: beetle, aphid, beetles, moth, pests, pest, aphids, wasps, wasp, and fungus.
The living/non-living judgments from VanArsdall and Blunt (2022) were modeled in the same way. Four predictors entered with p < 0.05: animal, bacteria, insect and plant (see Table 4). The model accounted for 26.7% of the variance in the human ratings in the development set and 23.7% of the variance in the validation set. The model was applied to the full dictionary. The 10 words estimated most animate were animal, insect, rodent, animals, owl, bird, reptile, critter, feline, and elephant. This list has high face validity, both because it only includes only words that name living things and because it includes many high-level living-thing category names. The relationship between the predictors and the living/nonliving judgments are shown graphically in Figure 4.
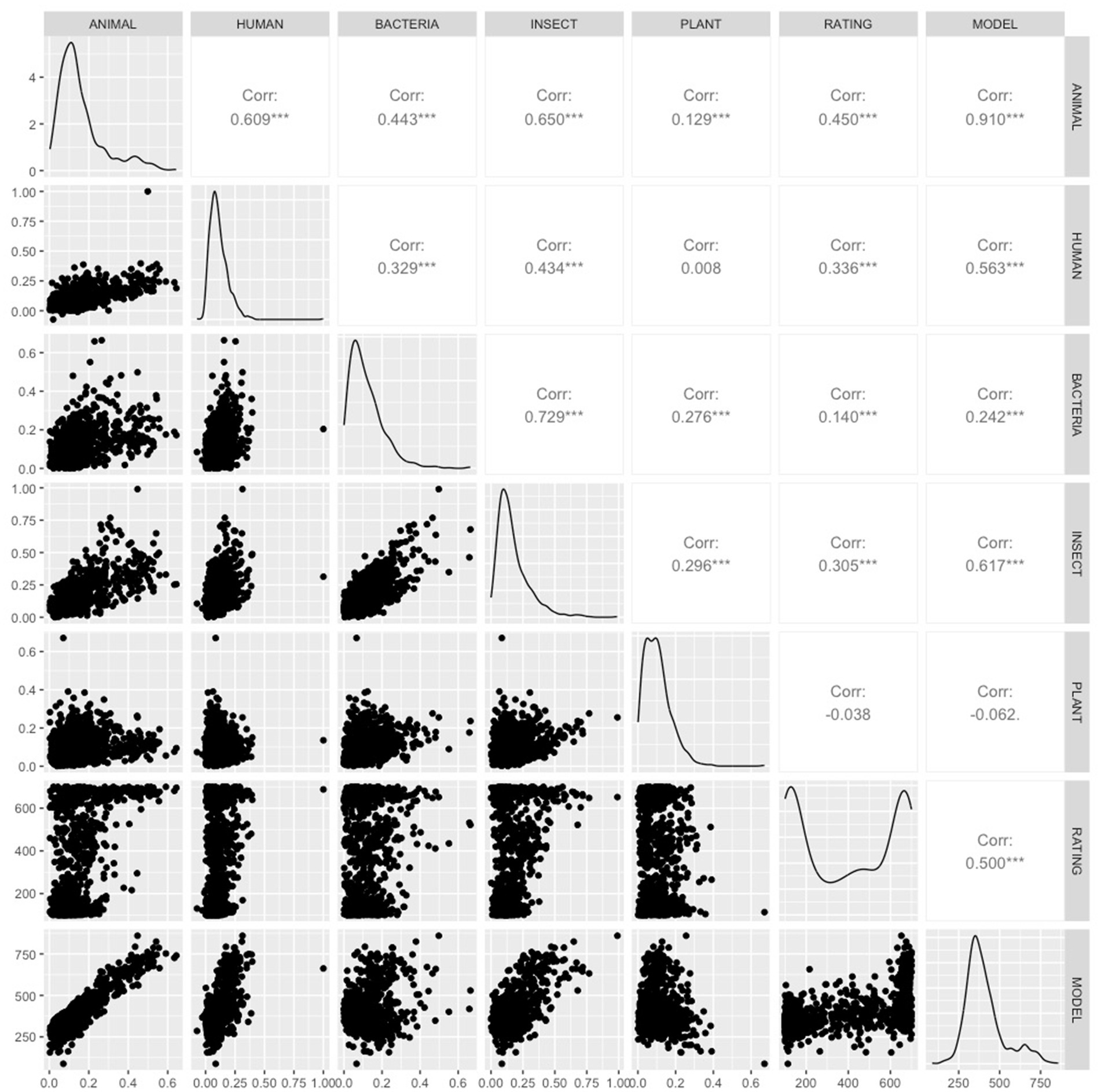
Figure 4. Relationships between human living/non-living ratings, Model 2’s estimates of those ratings, and vector cosine similarity of each word’s vector to five animate category labels.
The predictions from this model are broken down into categories in the rightmost column of Table 1. The seven average categorical predictions from the model are highly correlated with the average categorical human ratings of both animacy (r = 0.91, p = 0.002 one-sided) and living/non-living (r = 0.81, p = 0.01 one-sided) (Table 4).
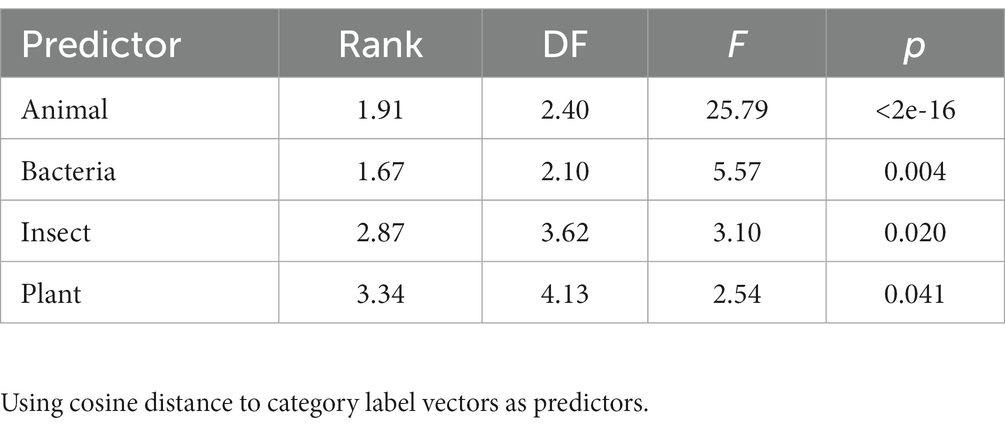
Table 4. Best GAM model to predict the living/non-living ratings from VanArsdall and Blunt (2022).
3.3. Model 2: discussion
The results from all models to predict human animacy and living/non-living ratings are summarized in Table 5. There are two main findings.
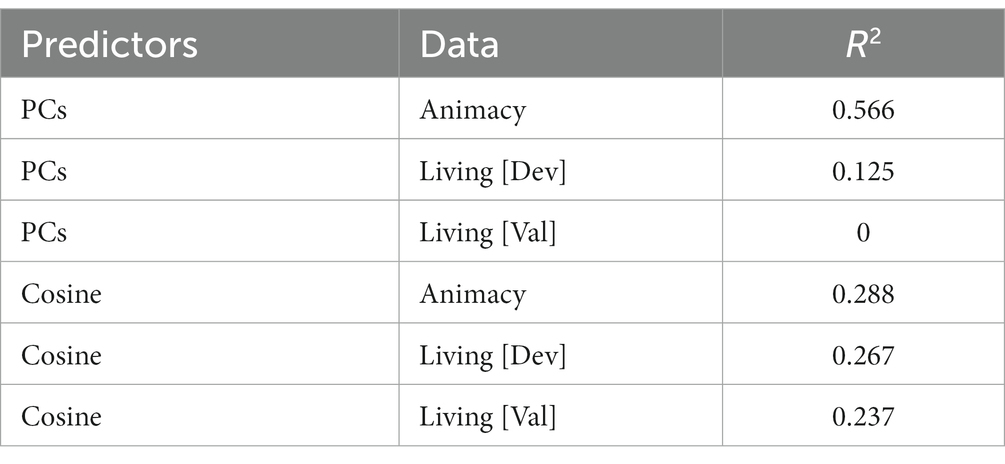
Table 5. Results for predicting human animacy ratings, using GAM with PCs (Model 1) or cosine similarity to the vectors for label names (Model 2).
One is that modeling human animacy and living/non-living judgments using distance from category names is more successful than modeling them using word2vec PCs. Although the word2vec PCs predicted the 72 animacy judgments relatively well (R2 = 0.57), that model had very low face validity when extended to the whole dictionary. Those word2vec vectors were also poor at predicting the living/non-living judgments. The best model accounted for only 12.5% of the variance and failed to cross-validate at all. In contrast, the model using distances from category names accounted for roughly the same variance in the animacy (28.8%) and living judgments (26.7%), although of course there are many more living judgments. That model cross-validated relatively well, accounting for 23.7% of the variance in the living/non-living judgment validation dataset. It also had high face validity when applied to a larger set of words.
The other finding of interest is that neither of models using categorical distance included distance from the category human. This is noteworthy because (as shown in Table 1 and discussed above) human categories tend to be rated low by humans on both animacy and (to a lesser extent) living/non-living judgments, where they received an average rating of 92.6/100, compared to 94.0/100 for mammals and birds and 94.0/100 for other living creatures.
4. General discussion
Of course, if we provided a model of animacy with categorical information, it would achieve perfect classification, since the five categories of plant, animal, insects, humans (which are of course also animals, but we generally do not speak of them this way), and bacteria cover the superordinate category of the animate almost perfectly. The fact that humans are not unanimous about their decisions suggests that human beings must not be relying on categorical information, which we already knew from their failure to accept members of these categories as animate with perfect accuracy.
The fact that the pattern of errors in the models is similar to the pattern of errors seen in humans suggests that human may be making animacy decisions based on contextual information (or the categories that may be derived from that information) rather than on category membership.
The model which used cosine distance from category labels performed much better at classifying words as being animate than the analogous model that used PCs. We can roughly conceive of the models as being bottom-up (PC predictors) versus top-down (category label predictors). These results therefore suggest that animacy is unlike valence or arousal, which are usually conceived as being components of semantics (Osgood et al., 1957). It is rather more like being expensive or being soft, an objectively grounded top-down classification that we learn from experience.
The second noteworthy finding supports this. That is the fact that the models built on cosine distance from the category name vectors make one of the same errors that humans do: they tend to rate humans as lower in animacy than animals. Table 1 shows that human beings rated human words (such as mother, boy, and professor) as animate at 60/100, compared to 79.8 for animal names. Similarly, the model rates humans at 55.2, compared to 89.2 for animals. This may reflect that humans are not generally conceived of (or, at least, written about) as animate.
The model also replicates humans in (erroneously) classifying plants as moderately animate. Humans rated plants at 51/100 (Table 1). The model rates them at 53.6/100.
The top 200 most animate words according to the final model are reproduced in Supplementary Appendix 1. Animacy ratings for the full dictionary of 67,717 words are available at https://osf.io/k3cn9/.
It is obvious that humans do not make animacy decisions using category membership. If they did their animacy ratings would be unanimously high or low for many words that get intermediate ratings. The success of the category vector distance models at modeling human ratings suggests that humans are instead making animacy judgments by making rough animate category membership judgments (without considering the category of humans, according the best model discussed above). This may have implications for studies looking at animacy effects. The repeated finding that humans and living things outside of the animalia kingdom are poorly classified as animate by models using cosine distance from the vectors of category names suggests that language use does not present humans and living things outside of the animalia kingdom in contexts that highlight their animacy. These results suggest that humans make animacy ratings not by considering the category of each item, but rather by making family resemblance judgments to animate categories. The nature and direction of those judgments are reflected in word-embedding models.
Data availability statement
The original contributions presented in the study are included in the article/Supplementary material, further inquiries can be directed to the corresponding author.
Ethics statement
The studies involving human participants were reviewed and approved by the University of Alberta Research Ethics Board. The patients/participants provided their written informed consent to participate in this study.
Author contributions
The author confirms being the sole contributor of this work and has approved it for publication.
Funding
This work was funded by the Natural Sciences and Engineering Research Council of Canada, grant#RGPIN-2018-04679.
Acknowledgments
Thanks to the editor and reviewers for their helpful feedback on an earlier version of this manuscript.
Conflict of interest
The author declares that the research was conducted in the absence of any commercial or financial relationships that could be construed as a potential conflict of interest.
Publisher’s note
All claims expressed in this article are solely those of the authors and do not necessarily represent those of their affiliated organizations, or those of the publisher, the editors and the reviewers. Any product that may be evaluated in this article, or claim that may be made by its manufacturer, is not guaranteed or endorsed by the publisher.
Supplementary material
The Supplementary material for this article can be found online at: https://www.frontiersin.org/articles/10.3389/fpsyg.2023.1145289/full#supplementary-material
Footnotes
References
Bonin, P., Gelin, M., Dioux, V., and Méot, A. (2019). “It is alive!” evidence for animacy effects in semantic categorization and lexical decision. Appl. Psycholinguist. 40, 965–985. doi: 10.1017/S0142716419000092
Bonin, P., Gelin, M., Laroche, B., Méot, A., and Bugaiska, A. (2015). The “how” of animacy effects in episodic memory. Exp. Psychol. 62, 371–384. doi: 10.1027/1618-3169/a000308
Bugaiska, A., Grégoire, L., Camblats, A. M., Gelin, M., Méot, A., and Bonin, P. (2019). Animacy and attentional processes: evidence from the stroop task. Q. J. Exp. Psychol. 72, 882–889. doi: 10.1177/1747021818771514
Bugaiska, A., Méot, A., and Bonin, P. (2016). Do healthy elders, like young adults, remember animates better than inanimates? An adaptive view. Exp. Aging Res. 42, 447–459. doi: 10.1080/0361073X.2016.1224631
Cappa, S. F., Perani, D., Schnur, T., Tettamanti, M., and Fazio, F. (1998). The effects of semantic category and knowledge type on lexical-semantic access: a PET study. NeuroImage 8, 350–359. doi: 10.1006/nimg.1998.0368
Caramazza, A., and Shelton, J. R. (1998). Domain-specific knowledge systems in the brain the animate-inanimate distinction. J. Cogn. Neurosci. 10, 1–34. doi: 10.1162/089892998563752
Darnell, R., and Vanek, A. L. (1976). The semantic basis of the animate/inanimate distinction in Cree. Res. Lang. Soc. Interact. 9, 159–180.
Devlin, J. T., Russell, R. P., Davis, M. H., Price, C. J., Moss, H. E., Fadili, M. J., et al. (2002). Is there an anatomical basis for category-specificity? Semantic memory studies in PET and fMRI. Neuropsychologia 40, 54–75. doi: 10.1016/S0028-3932(01)00066-5
Félix, S. B., Pandeirada, J., and Nairne, J. S. (2020). Animacy norms for 224 European Portuguese concrete words. Análise Psicológica 38, 257–269. doi: 10.14417/ap.1690
Gennari, S. P., Mirković, J., and MacDonald, M. C. (2012). Animacy and competition in relative clause production: a cross-linguistic investigation. Cogn. Psychol. 65, 141–176. doi: 10.1016/j.cogpsych.2012.03.002
Grabowski, T. J., Damasio, H., and Damasio, A. R. (1998). Premotor and prefrontal correlates of category-related lexical retrieval. NeuroImage 7, 232–243. doi: 10.1006/nimg.1998.0324
Grewe, T., Bornkessel, I., Zysset, S., Wiese, R., von Cramon, D. Y., and Schlesewsky, M. (2006). Linguistic prominence and Broca’s area: the influence of animacy as a linearization principle. NeuroImage 32, 1395–1402. doi: 10.1016/j.neuroimage.2006.04.213
Hollis, G., and Westbury, C. (2016). The principals of meaning: extracting semantic dimensions from co-occurrence models of semantics. Psychon. Bull. Rev. 23, 1744–1756. doi: 10.3758/s13423-016-1053-2
Hollis, G., Westbury, C., and Lefsrud, L. (2017). Extrapolating human judgments from skip-gram vector representations of word meaning. Q. J. Exp. Psychol. 70, 1603–1619. doi: 10.1080/17470218.2016.1195417
Ilić, O., Ković, V., and Styles, S. J. (2013). In the absence of animacy: superordinate category structure affects subordinate label verification. PLoS One 8:e83282. doi: 10.1371/journal.pone.0083282
Kazanas, S. A., Altarriba, J., and O’Brien, E. G. (2020). Paired-associate learning, animacy, and imageability effects in the survival advantage. Mem. Cogn. 48, 244–255. doi: 10.3758/s13421-019-01007-2
Landauer, T. K., and Dumais, S. T. (1997). A solution to Plato's problem: the latent semantic analysis theory of acquisition, induction, and representation of knowledge. Psychol. Rev. 104, 211–240. doi: 10.1037/0033-295X.104.2.211
Mahjoubnavaz, F., and Mokhtari, S. (2022). Animacy in Persian language: Animacy norms for 401 Persian words. J. Neurodevelop. Cogn. 5, 21–37.
Mak, W. M., Vonk, W., and Schriefers, H. (2002). The influence of animacy on relative clause processing. J. Mem. Lang. 47, 50–68. doi: 10.1006/jmla.2001.2837
Martin, A., Wiggs, C. L., Ungerleider, L. G., and Haxby, J. V. (1996). Neural correlates of category-specific knowledge. Nature 379, 649–652. doi: 10.1038/379649a0
Mikolov, T., Chen, K., Corrado, G., and Dean, J. (2013a). Efficient estimation of word representations in vector space. ICLR. Available at: https://arxiv.org/abs/1301.3781.
Mikolov, T., Sutskever, I., Chen, K., Corrado, G. S., and Dean, J. (2013b). Distributed representations of words and phrases and their compositionality. Advances in neural information processing systems. 26:3111–3119.
Mikolov, T., Yih, W. T., and Zweig, G. (2013c). “Linguistic regularities in continuous space word representations,”in Proceedings of the 2013 conference of the North American chapter of the Association for Computational Linguistics: Human language technologies. 746–751.
Moore, C. J., and Price, C. J. (1999). A functional neuroimaging study of the variables that generate category-specific object processing differences. Brain 122, 943–962. doi: 10.1093/brain/122.5.943
Mummery, C. J., Patterson, K., Hodges, J. R., and Price, C. J. (1998). Functional neuroanatomy of the semantic system: divisible by what? J. Cogn. Neurosci. 10, 766–777. doi: 10.1162/089892998563059
Nairne, J. S., VanArsdall, J. E., and Cogdill, M. (2017). Remembering the living: episodic memory is tuned to animacy. Curr. Dir. Psychol. Sci. 26, 22–27. doi: 10.1177/0963721416667711
Oxford English Dictionary (2022). Oxford University Press, September 2022. Available at: www.oed.com/view/Entry/248721 (Accessed December 1, 2022).
Osgood, C. E., Suci, G. J., and Tannenbaum, P. H. (1957). The measurement of meaning. Chicago, USA: University of Illinois Press.
Perani, D., Cappa, S. F., Bettinardi, V., Bressi, S., Gorno-Tempini, M., Matarrese, M., et al. (1995). Different neural systems for the recognition of animals and man-made tools. Neuroreport 6, 1637–1641. doi: 10.1097/00001756-199508000-00012
Perani, D., Schnur, T., Tettamanti, M., Gorno, M., Cappa, S. F., and Fazio, F. (1999). Word and picture matching: a PET study of semantic category effects. Neuropsychologia 37, 293–306. doi: 10.1016/S0028-3932(98)00073-6
Pilgrim, L. K., Fadili, J., Fletcher, P., and Tyler, L. K. (2002). Overcoming confounds of stimulus blocking: an event-related fMRI design of semantic processing. NeuroImage 16, 713–723. doi: 10.1006/nimg.2002.1105
Popp, E. Y., and Serra, M. J. (2016). Adaptive memory: animacy enhances free recall but impairs cued recall. J. Exp. Psychol. Learn. Mem. Cogn. 42, 186–201. doi: 10.1037/xlm0000174
Popp, E. Y., and Serra, M. J. (2018). The animacy advantage for free-recall performance is not attributable to greater mental arousal. Memory 26, 89–95. doi: 10.1080/09658211.2017.1326507
R Core Team (2022). R: A language and environment for statistical computing. R Foundation for Statistical Computing, Vienna, Austria.
Radanović, J., Westbury, C., and Milin, P. (2016). Quantifying semantic animacy: how much are words alive? Appl. Psycholinguist. 37, 1477–1499. doi: 10.1017/S0142716416000096
Shaoul, C., and Westbury, C. (2006) USENET orthographic frequencies for 111,627 English words. (2005–2006). Edmonton, AB: University of Alberta.
Szewczyk, J. M., and Schriefers, H. (2011). Is animacy special?: ERP correlates of semantic violations and animacy violations in sentence processing. Brain Res. 1368, 208–221. doi: 10.1016/j.brainres.2010.10.070
Traxler, M. J., Williams, R. S., Blozis, S. A., and Morris, R. K. (2005). Working memory, animacy, and verb class in the processing of relative clauses. J. Mem. Lang. 53, 204–224. doi: 10.1016/j.jml.2005.02.010
Tyler, L. K., Bright, P., Dick, E., Tavares, P., Pilgrim, L., Fletcher, P., et al. (2003). Do semantic categories activate distinct cortical regions? Evidence for a distributed neural semantic system. Cogn. Neuropsychol. 20, 541–559. doi: 10.1080/02643290244000211
Tyler, L., and Moss, H. (2001). Towards a distributed account of conceptual knowledge. Trends Cogn. Sci. 5, 244–252. doi: 10.1016/S1364-6613(00)01651-X
Tyler, L. K., Moss, H. E., Durrant-Peatfield, M. R., and Levy, J. P. (2000). Conceptual structure and the structure of concepts: a distributed account of category-specific deficits. Brain Lang. 75, 195–231. doi: 10.1006/brln.2000.2353
VanArsdall, J. E., and Blunt, J. R. (2022). Analyzing the structure of animacy: exploring relationships among six new animacy and 15 existing normative dimensions for 1,200 concrete nouns. Mem. Cogn. 50, 997–1012. doi: 10.3758/s13421-021-01266-y
VanArsdall, J. E., Nairne, J. S., Pandeirada, J. N., and Cogdill, M. (2015). Adaptive memory: Animacy effects persist in paired-associate learning. Memory 23, 657–663. doi: 10.1080/09658211.2014.916304
Vihman, V. A., Nelson, D., and Kirby, S. (2018). Animacy distinctions arise from iterated learning. Open Linguist. 4, 552–565. doi: 10.1515/opli-2018-0027
Westbury, C., and Hollis, G. (2019). Conceptualizing syntactic categories as semantic categories: unifying part-of-speech identification and semantics using co-occurrence vector averaging. Behav. Res. Methods 51, 1371–1398. doi: 10.3758/s13428-018-1118-4
Westbury, C., and Wurm, L. H. (2022). Is it you you’re looking for? Personal relevance as a principal component of semantics. Ment. Lexicon 17, 1–33. doi: 10.1075/ml.20031.wes
Wood, S. (2017). Generalized additive models: An introduction with R, 2nd. London, England: Chapman and Hall/CRC.
Wood, S. (2022). mgcv: Mixed GAM computation vehicle with automatic smoothness estimation. Available at: https://cran.r-project.org/web/packages/mgcv/index.htm
Keywords: animacy, word embedding (word2vec), computational modeling methods, human judgment, taxonomy, classification
Citation: Westbury C (2023) Why are human animacy judgments continuous rather than categorical? A computational modeling approach. Front. Psychol. 14:1145289. doi: 10.3389/fpsyg.2023.1145289
Edited by:
Michael J. Serra, Texas Tech University, United StatesReviewed by:
Johannes Schultz, University Hospital Bonn, GermanySara B. Felix, University of Aveiro, Portugal
Aurélia Bugaiska, Université de Bourgogne, France
Copyright © 2023 Westbury. This is an open-access article distributed under the terms of the Creative Commons Attribution License (CC BY). The use, distribution or reproduction in other forums is permitted, provided the original author(s) and the copyright owner(s) are credited and that the original publication in this journal is cited, in accordance with accepted academic practice. No use, distribution or reproduction is permitted which does not comply with these terms.
*Correspondence: Chris Westbury, Y2hyaXN3QHVhbGJlcnRhLmNh