- 1Vivian Smith Department of Neurosurgery, University of Texas Health Science Center at Houston, Houston, TX, USA
- 2Department of Computational and Applied Mathematics, Rice University, Houston, TX, USA
- 3Texas Medical Center, Mischer Neuroscience Institute, Memorial Hermann Hospital, Houston, TX, USA
Invasive intracranial EEG (icEEG) offers a unique opportunity to study human cognitive networks at an unmatched spatiotemporal resolution. To date, the contributions of icEEG have been limited to the individual-level analyses or cohorts whose data are not integrated in any way. Here we discuss how grouped approaches to icEEG overcome challenges related to sparse-sampling, correct for individual variations in response and provide statistically valid models of brain activity in a population. By the generation of whole-brain activity maps, grouped icEEG enables the study of intra and interregional dynamics between distributed cortical substrates exhibiting task-dependent activity. In this fashion, grouped icEEG analyses can provide significant advances in understanding the mechanisms by which cortical networks give rise to cognitive functions.
Introduction
The exponential growth in whole-brain neuroimaging studies has produced an overwhelming amount of data, and the conceptual frameworks for the neurobiology of human cognition have undergone tremendous change. These data have produced a consensus that complex cognitive functions—such as language—cannot be understood through the isolated study of specialized, cortical regions (Hagoort, 2014). Currently, a major focus of cognitive neuroscience is to understand how cognition emerges from transient, coordinated neural interactions in distributed large-scale cortical networks (Felleman and Van Essen, 1991; Bressler, 1995; Sporns et al., 2005; Martin, 2007; Patterson et al., 2007; Poeppel, 2014). Driven largely by fMRI, PET, and lesion-based analyses, significant advances have been made in identifying anatomical substrates that form the neural architecture of these distributed networks (Damasio et al., 2004; Dronkers and Ogar, 2004; Binder et al., 2009; Price, 2010; Friederici, 2011; Kanwisher, 2011). However, the limited temporal resolution of these neuroimaging modalities has hindered our understanding of how intra- and interregional cortical interactions give rise to cognition (Lachaux et al., 2003a; Jerbi et al., 2009; Friederici and Singer, 2015).
Introducing icEEG
A unique opportunity to study cognitive function is presented in patients undergoing intracranial EEG (icEEG) recordings as part of their pre-surgical evaluations for medically refractive focal epilepsy (Mukamel and Fried, 2012). In order to delineate their epileptogenic networks, these patients are implanted with either subdural electrodes that record from the cortical surface or penetrating depth electrodes that record from below the cortical surface, and in some case both (McGonigal et al., 2007; Van Gompel et al., 2008; Tandon et al., 2009). As such, icEEG recordings yield multi-lobar, high spatio-temporal resolution sampling of disseminated brain regions, providing optimal coverage and signal fidelity in comparison to the poor temporal resolution of fMRI/PET and poor spatial resolution of surface EEG/MEG (Jerbi et al., 2009; Lachaux et al., 2012). Importantly, high-frequency broadband gamma activity (BGA, 40–200 Hz) captured by icEEG yields precise estimates of task-related cortical activity, thereby permitting the study of local and long-distance networks at the millisecond time-scales relevant to neural processes (Jacobs and Kahana, 2010; Lachaux et al., 2012).
The Need for Grouped icEEG Analysis: The Sparse-sampling Problem
Despite its remarkable advantages, the widespread acceptance of icEEG by cognitive neuroscience has been hindered by difficulties in data representation and analyses at the individual and population-level (for review see Lachaux et al., 2003b; Conner et al., 2013; Kadipasaoglu et al., 2014; Chang, 2015). Relevant to the current discussion are the challenges arising from spatially variable and limited electrode coverage in patients- termed the sparse-sampling problem. This issue is unique to icEEG research, as electrode implantation, and therefore the sites where data are actually collected, are dictated solely by clinical criteria. Therefore, only a fraction of the total brain volume is sampled in any one patient (Halgren et al., 1998), precluding comprehensive investigation of cortical networks at the individual-level.
To address the sparse-sampling problem, and thereby develop icEEG for the study of large-scale, distributed networks, different methods for the grouped analysis of icEEG data have recently been proposed (Miller et al., 2009; Dykstra et al., 2012; Burke et al., 2013; Conner et al., 2013; Davidesco et al., 2013; Kadipasaoglu et al., 2014). Because of the discrete nature of recordings, icEEG activity will likely underestimate functional activity at the individual level. Therefore, a primary goal in all of these methods is to accurately combine data across large numbers of subjects to generate continuous brain activity maps, which leverage the spatiotemporal advantages of icEEG toward providing a more comprehensive view of cortical function. One such method—developed by our lab—employs topologically accurate representations of subdural electrode coverage and BGA on subject-specific cortical models. By integrating this approach with surface-based normalization to precisely align datasets across subjects (Argall et al., 2006; Saad and Reynolds, 2012), and a mixed effects multilevel analysis (MEMA) to correct for unsampled cortical regions (i.e., missing data) (Chen et al., 2011), we are able to perform statistically valid and topologically accurate grouped analyses of icEEG data. In this fashion, our surface-based MEMA (SB-MEMA) can generate continuous brain-activity maps to fully leverage the unique spatio-temporal properties of icEEG in the study of network function (Kadipasaoglu et al., 2014).
To illustrate how such grouped icEEG approaches can contribute to cognitive neuroscience, we discuss SB-MEMA in the context of cortical networks relating to visual object recognition and reading. We note here that the following analyses are intended only as illustrative examples. Therefore, we have not provided detailed experimental methods or statistical interpretations of our results. Furthermore, all results presented in this manuscript are intended solely to highlight the potential application of such grouped icEEG approaches. Importantly, these results will be elaborated in subsequent publications, and this manuscript is not the definitive representation of those analyses.
Visual Object Recognition
Visual object recognition is believed to be mediated by neural substrates in the ventral temporal cortex (VTC) capable of categorizing visual inputs within a few 100 ms (Thorpe et al., 1996; Grill-Spector and Kanwisher, 2005). Yet the role of the VTC in accomplishing these complex functional computations remains a mystery. Non-invasive neuroimaging studies have demonstrated a consistent relationship between cortical topology (and white matter connectivity) and functional representations in the VTC (Saygin et al., 2012; Pyles et al., 2013; Grill-Spector and Weiner, 2014; Gomez et al., 2015). Specifically, the mid-fusiform sulcus predicts transitions in the location of cyto-architectonic regions, receptor architectonics, and large-scale functional maps in the VTC (e.g., eccentricity bias/domain-specificity/animacy/real-world object size) (Grill-Spector and Weiner, 2014; Weiner et al., 2014; Gomez et al., 2015). This has led to a hypothesis that the VTC's anatomical organization is spatially optimized for the computational processes of the distinct functional networks subserving object recognition (Grill-Spector and Weiner, 2014). Grouped icEEG studies are uniquely suited to investigate such hypotheses, which require the differentiation of functional networks at millimeter resolution and millisecond time-scales.
To demonstrate this, we use SB-MEMA to investigate category-specific differences in the fusiform gyrus. We applied SB-MEMA to icEEG data collected in a large cohort (n = 27, left hemisphere only) as they performed visual confrontation naming of famous faces and places. Importantly, we were able to achieve comprehensive fusiform coverage using the precise inter-subject co-registration afforded by SB-MEMA (Figure 1, top). To focus on early perceptual processes, the analysis was constrained to window from 50 to 500 ms after stimulus presentation. Consistent with previously reported domain-specificity maps, significant BGA for faces and places was localized in a lateral-to-medial fashion, respectively, along the mid-fusiform sulcus (Figure 1, middle; Kanwisher et al., 1997; Epstein and Kanwisher, 1998; Nasr et al., 2011; Grill-Spector and Weiner, 2014).
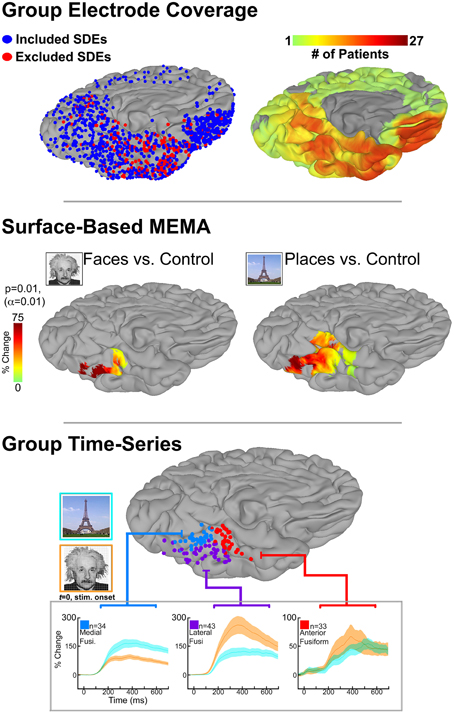
Figure 1. Top: icEEG data were collected in 27 patients, implanted with subdural electrodes (SDEs) in the left hemisphere, as they performed a visual confrontation naming of famous faces, places, and scrambled control images. Surface-based representations of SDE coverage and high-frequency broadband gamma activity (BGA; 60–120 Hz) were generated for each subject. We utilize cortical surface models that have been reconstructed from each subject's pre-implantation high-resolution anatomical MRI scans (Phillips Medical; T1-weighted, 1 mm isotropic resolution; using FreeSurfer software), and subsequently imported to the SUMA module of AFNI. Surface-based datasets of SDE coverage and BGA are generated with respect to each subject's cortical model using geodesic metrics to correct for local gyral and sulcal folding patterns. By spatially transforming data to the cortical surface, we integrate SUMA's surface-based normalization strategy to convert individual datasets to a standardized cortical surface (N27). To achieve this, SUMA resamples individual cortical models (and therefore their associated datasets) to a standardized mesh and enables a one-to-one correspondence between anatomical locations across subjects. Group maps for electrode (left) and surface-based coverage (right) are shown for the ventral temporal cortex. SDEs are modeled as spheres, with red spheres indicated SDEs that were excluded due to 60 Hz line noise or epileptiform activity. By grouping data in this fashion, comprehensive cortical coverage is obtained, and cognitive function can be critically evaluated at spatio-temporal scales relevant to neural processes. Middle: SB-MEMA derived significant grouped effects estimates by comparing composite BGA percent change (50–500 ms post-stim; with respect to pre-stimulus baseline of −700 to –200 ms) for each stimulus category against its scrambled control. Notably, BGA to faces was localized lateral to the mid-fusiform sulcus, while peak BGA to places was localized medially. Anterior to the mid-fusiform sulcus BGA for both conditions converged in magnitude and spatial extent. Bottom: Subject electrodes localized over the three regions in the fusiform (Fusi.) gyrus with significant activity to faces, places, or both stimuli as revealed by SB-MEMA (see B). SDEs are color-coded by region and displayed on a common brain surface (N27). Notably, SDEs are spatially arranged with respect to the mid-fusiform sulcus: laterally (purple), medially (blue), or anteriorly (red). Below, group time-series of percent change in BGA for face (orange) and place (cyan) stimuli can be seen. Of note, traces colored green indicate a region of activity overlap. Percent change is relative to a pre-stimulus baseline (−700 to −200 ms). Stimulus onset at 0 ms. Shading denotes 1 SEM. All figures display the ventro-medial aspect of the left hemisphere (N27 cortical surface model).
Given that SB-MEMA computes grouped effects estimates by summing BGA over time, a temporal smoothing of the data is still present. This precludes the evaluation of certain cortical response properties (e.g., onset latency/response duration), which may otherwise provide valuable insight into a given region's functional role (Lachaux et al., 2012). To evaluate the temporal profile of BGA, time-series representations of averaged BGA can be generated from all electrodes contributing to significant loci seen in SB-MEMA (Figure 1, bottom; Conner et al., 2013; Kadipasaoglu et al., 2014). In contrast to summing BGA over a time window, time-series representations instead compute percent change in BGA at each data point (which can be on the order of ms, depending on sampling rate) and plot these changes over time (Yoshor et al., 2007; Kadipasaoglu et al., 2014). Alternatively, data from time-series representations can be spatially transformed back onto the cortical surface to generate 4-dimensional, whole brain representations of cortical activity (Movie 1). Such visualization of time-varying BGA (shown as cortical surface heat maps) relative to cortical anatomy facilitates insights into dynamic network behavior that may not be readily appreciable in static images, and is complementary to SB-MEMA.
Once cortical regions of interest have been identified, more sophisticated measures for assessing functional connectivity and information flow can then be applied to understand how these regions interact during cognitive operations (Bruns et al., 2000; Canolty et al., 2006; Nir et al., 2008; Korzeniewska et al., 2011; Vidal et al., 2012; Watrous et al., 2013; Flinker et al., 2015). To illustrate this, we discuss one such connectivity measure—the short time direct directed transfer function (SdDTF) (Korzeniewska et al., 2008, 2011)—in the context of cortical reading networks. Of note, the electrodes for this example were identified using SB-MEMA for the evaluation of a word-completion task (not shown).
Network Dynamics of Reading
The neural substrates that comprise the reading network include cortical areas traditionally associated with language production (e.g., Broca's area), as well a ventrally positioned region in the fusiform gyrus, which demonstrates preferential responses to visually presented words and pseudowords (w-FG) (McCandliss et al., 2003). Cognitive approaches are divided on connectivity patterns during word reading that facilitate the visual processing of orthographic stimuli (Carreiras et al., 2014). While it is agreed upon that w-FG is crucial to word reading, some models predict strictly feed-forward connectivity patterns accompany word reading while other models stress the presence of bi-directional interactions between ventral visual and higher-level frontal cortex (Price and Devlin, 2011; Carreiras et al., 2014). Given that the anatomical sources and temporal evolution of top-down control are not well-established, a data-driven connectivity measure, such as SdDTF, is necessary to investigate the timing and directionality of information transmission during word reading. SdDTF quantifies connectivity across multi-dimensional networks, and can derive directed information flow between any two network nodes, while controlling for the contributions from all other sources (Korzeniewska et al., 2008, 2011). Applied to our icEEG data, patient-specific information flows were computed for subsets of task-relevant electrodes identified through SB-MEMA. It is important to note that connectivity between any two regions can only be derived in patients with electrodes recording simultaneously from both regions. In other words, connectivity measures must first be performed within subject, before individual connectivity estimates can be combined across subjects to yield a grouped connectivity estimate. In this fashion, flows derived from SdDTF were averaged over patient and region, and were able to isolate top-down information flow from Pars Triangularis to w-FG during a word completion task (Figure 2).
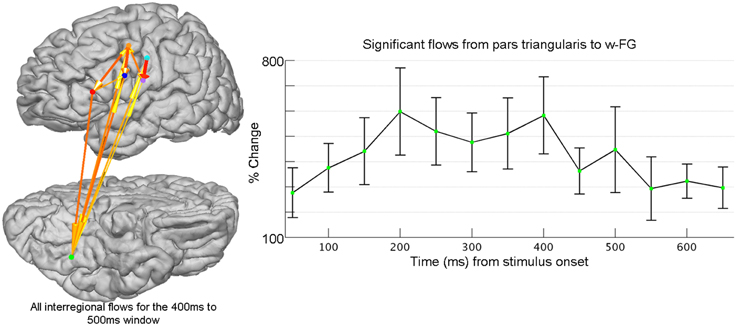
Figure 2. Frontal-ventral temporal interactions are evaluated using grouped icEEG collected during a word-completion task. Connectivity is evaluated using the Short-time direct Directed Transfer Function (SdDTF). Post-stimulus interregional flows were determined across post-stimulus windows (100 ms long, 50 ms shift) for high-frequency broadband gamma activity (60–120 Hz) and were compared to pre-stimulus flows computed over one pre-stimulus, baseline window (−700 ms to −200 ms). After normalizing across all patients, all post-stimulus interregional flows were tested for significance (FDR-corrected with a significance level of p = 0.05). Shown at right is the time course of percent change of flows (±1 standard error of the mean) from pars triangularis to word-preferential areas in fusiform gyrus (w-FG) that achieved significance. Electrodes for each region (colored spheres) have been identified using SB-MEMA (not shown). The cortical model to the left (lateral view at top, ventral view at bottom; left hemisphere) provides a snapshot of significant flows for the cortical reading network at 400 ms after stimulus onset (w-FG is shown in green and pars triangularis is shown in red). The ability to study long-distance cortical network interactions at millisecond resolution is a unique advantage of grouped icEEG, and enables the critical evaluation of hypotheses regarding functional network dynamics.
Conclusion
The study of icEEG has been able to generate novel insights into a wide range of cognitive functions (Jacobs and Kahana, 2010; Lachaux et al., 2012). Within the past decade alone, it has significantly advanced diverse areas of neuroscience research, including cognitive control (Wessel et al., 2013), working and episodic memory (Fell et al., 2001; Axmacher et al., 2007; Watrous et al., 2013), sensorimotor integration (Brovelli et al., 2005; Hermes et al., 2011; Bouchard et al., 2013), brain-machine interfaces (Leuthardt et al., 2004, 2011; Miller et al., 2007), perception (Allison et al., 1994, 1999; McCarthy et al., 1999; Privman et al., 2007, 2011; Fisch et al., 2009; Liu et al., 2009; Engell and McCarthy, 2010, 2014; Vidal et al., 2010; Chan et al., 2011; Davidesco et al., 2014; Ghuman et al., 2014), and language processing (Crone et al., 2001; Sahin et al., 2009; Chang et al., 2011; Mesgarani and Chang, 2012; Conner et al., 2013; Flinker et al., 2015). With the development of robust techniques for grouped analysis, icEEG analyses are provided a powerful new tool to investigate the architecture and interregional dynamics of distributed cortical networks. Yet despite its significant advantages, grouped approaches to icEEG still suffer from a number of limitations. Most notably, group-size, and degree of cortical coverage limit the applicability of methods like SB-MEMA. As mentioned earlier, the discrete nature of the recordings may underrepresent functional activity. A failure to find significant effects may be due to the absence of such effects in a given region (true negative) or the lack of sufficient coverage in that region (false negative). Furthermore, as discussed in Section Network Dynamics of Reading, connectivity measures are also dependent on individual with coverage in all regions of interest. For these reasons, it is critical that population-level analyses continue to be supported by data at the individual level.
A final concern that arises with any icEEG study is whether the results found in these patient populations are applicable to the normal human brain. Such concerns are generally addressed using a variety of inclusion criteria, both for patients as well as the data analyzed (e.g., data free of electrophysiological abnormalities, or which arise from pathological cortex) (Halgren et al., 1998; Lachaux et al., 2003b; Crone et al., 2006; Jerbi et al., 2009). The development of grouped icEEG provides a new environment in which to voice these concerns, but also a new opportunity to resolve them. Work from our lab has previously compared patient fMRI and icEEG recordings against fMRI obtained in healthy volunteers, under identical task conditions (Conner et al., 2013). Critically, we identified no significant difference in activity, further validating the reliability of such icEEG recordings. Additionally, work from other groups has begun to investigate the potential of multi-modal analyses by critically investigating grouped icEEG and grouped fMRI analyses from the same patient populations (Mukamel et al., 2005; Privman et al., 2007; He et al., 2008; Conner et al., 2011; Esposito et al., 2012). In doing so, these studies have hoped to better understand the electrophysiological basis of the BOLD signal. Such multi-modal approaches also provide a method for resolving concerns arising from the lack of global coverage in grouped icEEG studies. By integrating data from grouped fMRI and icEEG analyses, it could be confirmed that all relevant components of a given cognitive network have indeed been sampled within the icEEG cohort, prior to subjecting these data to a population-level analysis.
Author Contributions
NT designed research; CK, CC, MR, VB acquired data; CK, MW, KF, CC, VB performed research; CK, KF, MW, CC analyzed data; CK, MW, and NT wrote the paper.
Conflict of Interest Statement
The authors declare that the research was conducted in the absence of any commercial or financial relationships that could be construed as a potential conflict of interest.
Acknowledgments
Research reported in this publication was supported by the National Center for Advancing Translational Sciences of the National Institutes of Health (5TL1TR000369-07), the Clinical and Translational Award from the National Center for Research Resources (K12-KL2 RR0224149), the National Institute of Biomedical Imaging and Bioengineering (NIBIB; T32EB006350 to CC), the Keck Center for Interdisciplinary Bioscience Training of the Gulf Coast Consortia (Grant No. T15 LM007093), the Vivian Smith Foundation for Neurologic Research, the Keck Center of the Gulf Coast Consortium, and the Memorial Hermann Foundation. We thank Bartlett Moore IV, Suganya Karunakaran, Eleonora Bartoli, and Kamin Kim for their comments on earlier drafts of the manuscript. We are especially grateful to all the patients who participated in this study, the neurologists at the Texas Comprehensive Epilepsy Program (Jeremy Slater, Giridhar Kalamangalam, Omotola Hope and Melissa Thomas) who participated in the care of these patients, Vips Patel, and all of the nurses and technicians in the Epilepsy Monitoring Unit at Memorial Hermann Hospital who helped make this research possible.
Supplementary Material
The Supplementary Material for this article can be found online at: http://journal.frontiersin.org/article/10.3389/fpsyg.2015.01008
Movie 1. Intracranial recordings provide unparalleled insights into rapidly evolving patterns of cortical activity across distributed neural substrates. Electrocorticographic movies of grouped percent change in high-frequency broadband gamma activity (BGA; 60–120 Hz; n = 27 subject) during a face and place-naming task are visualized on the N27 cortical surface model. Hotter colors denote an increase in mid-gamma band power, while cooler colors denote a decrease (color-scale ranges from −50 to 50% change). The movie begins 100 ms before stimulus onset and continues until 700 ms after stimulus onset, in 5 ms steps (stimulus onset at 0 ms). Notably, for face stimuli (left surface) increases in BGA are localized to the lateral aspect of the mid-fusiform sulcus. In contrast, place stimuli produce more widespread activations in the medial aspects of the fusiform gyrus.
References
Allison, T., Ginter, H., McCarthy, G., Nobre, A. C., Puce, A., Luby, M., et al. (1994). Face recognition in human extrastriate cortex. J. Neurophysiol. 71, 821–825.
Allison, T., Puce, A., Spencer, D. D., and McCarthy, G. (1999). Electrophysiological studies of human face perception. I: potentials generated in occipitotemporal cortex by face and non-face stimuli. Cereb. Cortex 9, 415–430. doi: 10.1093/cercor/9.5.415
Argall, B. D., Saad, Z. S., and Beauchamp, M. S. (2006). Simplified intersubject averaging on the cortical surface using SUMA. Hum. Brain Mapp. 27, 14–27. doi: 10.1002/hbm.20158
Axmacher, N., Mormann, F., Fernandez, G., Cohen, M. X., Elger, C. E., and Fell, J. (2007). Sustained neural activity patterns during working memory in the human medial temporal lobe. J. Neurosci. 27, 7807–7816. doi: 10.1523/JNEUROSCI.0962-07.2007
Binder, J. R., Desai, R. H., Graves, W. W., and Conant, L. L. (2009). Where is the semantic system? A critical review and meta-analysis of 120 functional neuroimaging studies. Cereb. Cortex 19, 2767–2796. doi: 10.1093/cercor/bhp055
Bouchard, K. E., Mesgarani, N., Johnson, K., and Chang, E. F. (2013). Corrigendum: functional organization of human sensorimotor cortex for speech articulation. Nature 495, 327–332. doi: 10.1038/nature12182
Bressler, S. L. (1995). Large-scale cortical networks and cognition. Brain Res. Brain Res. Rev. 20, 288–304. doi: 10.1016/0165-0173(94)00016-I
Brovelli, A., Lachaux, J. P., Kahane, P., and Boussaoud, D. (2005). High gamma frequency oscillatory activity dissociates attention from intention in the human premotor cortex. Neuroimage 28, 154–164. doi: 10.1016/j.neuroimage.2005.05.045
Bruns, A., Eckhorn, R., Jokeit, H., and Ebner, A. (2000). Amplitude envelope correlation detects coupling among incoherent brain signals. Neuroreport 11, 1509–1514. doi: 10.1097/00001756-200005150-00028
Burke, J. F., Zaghloul, K. A., Jacobs, J., Williams, R. B., Sperling, M. R., Sharan, A. D., et al. (2013). Synchronous and asynchronous theta and gamma activity during episodic memory formation. J. Neurosci. 33, 292–304. doi: 10.1523/JNEUROSCI.2057-12.2013
Canolty, R. T., Edwards, E., Dalal, S. S., Soltani, M., Nagarajan, S. S., Kirsch, H. E., et al. (2006). High gamma power is phase-locked to theta oscillations in human neocortex. Science 313, 1626–1628. doi: 10.1126/science.1128115
Carreiras, M., Armstrong, B. C., Perea, M., and Frost, R. (2014). The what, when, where, and how of visual word recognition. Trends Cogn. Sci. 18, 90–98. doi: 10.1016/j.tics.2013.11.005
Chan, A. M., Baker, J. M., Eskandar, E., Schomer, D., Ulbert, I., Marinkovic, K., et al. (2011). First-pass selectivity for semantic categories in human anteroventral temporal lobe. J. Neurosci. 31, 18119–18129. doi: 10.1523/JNEUROSCI.3122-11.2011
Chang, E. F. (2015). Towards large-scale, human-based, mesoscopic neurotechnologies. Neuron 86, 68–78. doi: 10.1016/j.neuron.2015.03.037
Chang, E. F., Rieger, J. W., Johnson, K., Berger, M. S., Barbaro, N. M., and Knight, R. T. (2011). Categorical speech representation in human superior temporal gyrus. Nat. Neurosci. 13, 1428–1432. doi: 10.1038/nn.2641
Chen, G., Saad, Z. S., Nath, A. R., Beauchamp, M. S., and Cox, R. W. (2011). FMRI group analysis combining effect estimates and their variances. Neuroimage 60, 747–765. doi: 10.1016/j.neuroimage.2011.12.060
Conner, C. R., Chen, G., Pieters, T. A., and Tandon, N. (2013). Category specific spatial dissociations of parallel processes underlying visual naming. Cereb. Cortex 24, 2741–2750. doi: 10.1093/cercor/bht130
Conner, C. R., Ellmore, T. M., Pieters, T. A., Disano, M. A., and Tandon, N. (2011). Variability of the relationship between electrophysiology and BOLD-fMRI across cortical regions in humans. J. Neurosci. 31, 12855–12865. doi: 10.1523/JNEUROSCI.1457-11.2011
Crone, N. E., Hao, L., Hart, B. J., Boatman, D., Lesser, R. P., Irizarry, R., et al. (2001). Electrocorticographic gamma activity during word production in spoken and sign language. Neurology 57, 2045–2053. doi: 10.1212/WNL.57.11.2045
Crone, N. E., Sinai, A., and Korzeniewska, A. (2006). High-frequency gamma oscillations and human brain mapping with electrocorticography. Prog. Brain Res. 159, 275–295. doi: 10.1016/S0079-6123(06)59019-3
Damasio, H., Tranel, D., Grabowski, T., Adolphs, R., and Damasio, A. (2004). Neural systems behind word and concept retrieval. Cognition 92, 179–229. doi: 10.1016/j.cognition.2002.07.001
Davidesco, I., Harel, M., Ramot, M., Kramer, U., Kipervasser, S., Andelman, F., et al. (2013). Spatial and object-based attention modulates broadband high-frequency responses across the human visual cortical hierarchy. J. Neurosci. 33, 1228–1240. doi: 10.1523/JNEUROSCI.3181-12.2013
Davidesco, I., Zion-Golumbic, E., Bickel, S., Harel, M., Groppe, D. M., Keller, C. J., et al. (2014). Exemplar selectivity reflects perceptual similarities in the human fusiform cortex. Cereb. Cortex 24, 1879–1893. doi: 10.1093/cercor/bht038
Dronkers, N., and Ogar, J. (2004). Brain areas involved in speech production. Brain 127, 1461–1462. doi: 10.1093/brain/awh233
Dykstra, A. R., Chan, A. M., Quinn, B. T., Zepeda, R., Keller, C. J., Cormier, J., et al. (2012). Individualized localization and cortical surface-based registration of intracranial electrodes. Neuroimage 59, 3563–3570. doi: 10.1016/j.neuroimage.2011.11.046
Engell, A. D., and McCarthy, G. (2010). Selective attention modulates face-specific induced gamma oscillations recorded from ventral occipitotemporal cortex. J. Neurosci. 30, 8780–8786. doi: 10.1523/JNEUROSCI.1575-10.2010
Engell, A. D., and McCarthy, G. (2014). Face, eye, and body selective responses in fusiform gyrus and adjacent cortex: an intracranial EEG study. Front. Hum. Neurosci. 8:642. doi: 10.3389/fnhum.2014.00642
Epstein, R., and Kanwisher, N. (1998). A cortical representation of the local visual environment. Nature 392, 598–601. doi: 10.1038/33402
Esposito, F., Singer, N., Podlipsky, I., Fried, I., Hendler, T., and Goebel, R. (2012). Cortex-based inter-subject analysis of iEEG and fMRI data sets: application to sustained task-related BOLD and gamma responses. Neuroimage 66C, 457–468. doi: 10.1016/j.neuroimage.2012.10.080
Fell, J., Klaver, P., Lehnertz, K., Grunwald, T., Schaller, C., Elger, C. E., et al. (2001). Human memory formation is accompanied by rhinal-hippocampal coupling and decoupling. Nat. Neurosci. 4, 1259–1264. doi: 10.1038/nn759
Felleman, D. J., and Van Essen, D. C. (1991). Distributed hierarchical processing in the primate cerebral cortex. Cereb. Cortex 1, 1–47. doi: 10.1093/cercor/1.1.1
Fisch, L., Privman, E., Ramot, M., Harel, M., Nir, Y., Kipervasser, S., et al. (2009). Neural “ignition”: enhanced activation linked to perceptual awareness in human ventral stream visual cortex. Neuron 64, 562–574. doi: 10.1016/j.neuron.2009.11.001
Flinker, A., Korzeniewska, A., Shestyuk, A. Y., Franaszczuk, P. J., Dronkers, N. F., Knight, R. T., et al. (2015). Redefining the role of Broca's area in speech. Proc. Natl. Acad. Sci. U.S.A. 112, 2871–2875. doi: 10.1073/pnas.1414491112
Friederici, A. D. (2011). The brain basis of language processing: from structure to function. Physiol. Rev. 91, 1357–1392. doi: 10.1152/physrev.00006.2011
Friederici, A. D., and Singer, W. (2015). Grounding language processing on basic neurophysiological principles. Trends Cogn. Sci. 19, 329–338. doi: 10.1016/j.tics.2015.03.012
Ghuman, A. S., Brunet, N. M., Li, Y., Konecky, R. O., Pyles, J. A., Walls, S. A., et al. (2014). Dynamic encoding of face information in the human fusiform gyrus. Nat. Commun. 5, 5672. doi: 10.1038/ncomms6672
Gomez, J., Pestilli, F., Witthoft, N., Golarai, G., Liberman, A., Poltoratski, S., et al. (2015). Functionally defined white matter reveals segregated pathways in human ventral temporal cortex associated with category-specific processing. Neuron 85, 216–227. doi: 10.1016/j.neuron.2014.12.027
Grill-Spector, K., and Kanwisher, N. (2005). Visual recognition: as soon as you know it is there, you know what it is. Psychol. Sci. 16, 152–160. doi: 10.1111/j.0956-7976.2005.00796.x
Grill-Spector, K., and Weiner, K. S. (2014). The functional architecture of the ventral temporal cortex and its role in categorization. Nat. Rev. Neurosci. 15, 536–548. doi: 10.1038/nrn3747
Hagoort, P. (2014). Nodes and networks in the neural architecture for language: broca's region and beyond. Curr. Opin. Neurobiol. 28, 136–141. doi: 10.1016/j.conb.2014.07.013
Halgren, E., Marinkovic, K., and Chauvel, P. (1998). Generators of the late cognitive potentials in auditory and visual oddball tasks. Electroencephalogr. Clin. Neurophysiol. 106, 156–164. doi: 10.1016/S0013-4694(97)00119-3
He, B. J., Snyder, A. Z., Zempel, J. M., Smyth, M. D., and Raichle, M. E. (2008). Electrophysiological correlates of the brain's intrinsic large-scale functional architecture. Proc. Natl. Acad. Sci. U.S.A. 105, 16039–16044. doi: 10.1073/pnas.0807010105
Hermes, D., Miller, K. J., Vansteensel, M. J., Aarnoutse, E. J., Leijten, F. S., and Ramsey, N. F. (2011). Neurophysiologic correlates of fMRI in human motor cortex. Hum. Brain Mapp. 33, 1689–1899. doi: 10.1002/hbm.21314
Jacobs, J., and Kahana, M. J. (2010). Direct brain recordings fuel advances in cognitive electrophysiology. Trends Cogn. Sci. 14, 162–171. doi: 10.1016/j.tics.2010.01.005
Jerbi, K., Ossandon, T., Hamame, C. M., Senova, S., Dalal, S. S., Jung, J., et al. (2009). Task-related gamma-band dynamics from an intracerebral perspective: review and implications for surface EEG and MEG. Hum. Brain Mapp. 30, 1758–1771. doi: 10.1002/hbm.20750
Kadipasaoglu, C. M., Baboyan, V. G., Conner, C. R., Chen, G., Saad, Z. S., and Tandon, N. (2014). Surface-based mixed effects multilevel analysis of grouped human electrocorticography. Neuroimage 101, 215–224. doi: 10.1016/j.neuroimage.2014.07.006
Kanwisher, N. B. J. (2011). “The functional architecture of the face system: integrating evidence from fmri and patient studies,” in The Oxford Handbook of Face Perception, Vol. 1, ed N. B. J. Kanwisher (New York, NY: Oxford University Press), 111–129.
Kanwisher, N., McDermott, J., and Chun, M. M. (1997). The fusiform face area: a module in human extrastriate cortex specialized for face perception. J. Neurosci. 17, 4302–4311.
Korzeniewska, A., Crainiceanu, C. M., Kus, R., Franaszczuk, P. J., and Crone, N. E. (2008). Dynamics of event-related causality in brain electrical activity. Hum. Brain Mapp. 29, 1170–1192. doi: 10.1002/hbm.20458
Korzeniewska, A., Franaszczuk, P. J., Crainiceanu, C. M., Kus, R., and Crone, N. E. (2011). Dynamics of large-scale cortical interactions at high gamma frequencies during word production: event related causality (ERC) analysis of human electrocorticography (ECoG). Neuroimage 56, 2218–2237. doi: 10.1016/j.neuroimage.2011.03.030
Lachaux, J. P., Axmacher, N., Mormann, F., Halgren, E., and Crone, N. E. (2012). High-frequency neural activity and human cognition: past, present and possible future of intracranial EEG research. Prog. Neurobiol. 98, 279–301. doi: 10.1016/j.pneurobio.2012.06.008
Lachaux, J. P., Chavez, M., and Lutz, A. (2003a). A simple measure of correlation across time, frequency and space between continuous brain signals. J. Neurosci. Methods 123, 175–188. doi: 10.1016/S0165-0270(02)00358-8
Lachaux, J. P., Rudrauf, D., and Kahane, P. (2003b). Intracranial EEG and human brain mapping. J. Physiol. Paris 97, 613–628. doi: 10.1016/j.jphysparis.2004.01.018
Leuthardt, E. C., Gaona, C., Sharma, M., Szrama, N., Roland, J., Freudenberg, Z., et al. (2011). Using the electrocorticographic speech network to control a brain-computer interface in humans. J. Neural Eng. 8:036004. doi: 10.1088/1741-2560/8/3/036004
Leuthardt, E. C., Schalk, G., Wolpaw, J. R., Ojemann, J. G., and Moran, D. W. (2004). A brain-computer interface using electrocorticographic signals in humans. J. Neural Eng. 1, 63–71. doi: 10.1088/1741-2560/1/2/001
Liu, H., Agam, Y., Madsen, J. R., and Kreiman, G. (2009). Timing, timing, timing: fast decoding of object information from intracranial field potentials in human visual cortex. Neuron 62, 281–290. doi: 10.1016/j.neuron.2009.02.025
Martin, A. (2007). The representation of object concepts in the brain. Annu. Rev. Psychol. 58, 25–45. doi: 10.1146/annurev.psych.57.102904.190143
McCandliss, B. D., Cohen, L., and Dehaene, S. (2003). The visual word form area: expertise for reading in the fusiform gyrus. Trends Cogn. Sci. 7, 293–299. doi: 10.1016/S1364-6613(03)00134-7
McCarthy, G., Puce, A., Belger, A., and Allison, T. (1999). Electrophysiological studies of human face perception. II: response properties of face-specific potentials generated in occipitotemporal cortex. Cereb. Cortex 9, 431–444. doi: 10.1093/cercor/9.5.431
McGonigal, A., Bartolomei, F., Regis, J., Guye, M., Gavaret, M., Trebuchon-Da Fonseca, A., et al. (2007). Stereoelectroencephalography in presurgical assessment of MRI-negative epilepsy. Brain 130, 3169–3183. doi: 10.1093/brain/awm218
Mesgarani, N., and Chang, E. F. (2012). Selective cortical representation of attended speaker in multi-talker speech perception. Nature 485, 233–236. doi: 10.1038/nature11020
Miller, K. J., Dennijs, M., Shenoy, P., Miller, J. W., Rao, R. P., and Ojemann, J. G. (2007). Real-time functional brain mapping using electrocorticography. Neuroimage 37, 504–507. doi: 10.1016/j.neuroimage.2007.05.029
Miller, K. J., Weaver, K. E., and Ojemann, J. G. (2009). Direct electrophysiological measurement of human default network areas. Proc. Natl. Acad. Sci. U.S.A. 106, 12174–12177. doi: 10.1073/pnas.0902071106
Mukamel, R., and Fried, I. (2012). Human intracranial recordings and cognitive neuroscience. Annu. Rev. Psychol. 63, 511–537. doi: 10.1146/annurev-psych-120709-145401
Mukamel, R., Gelbard, H., Arieli, A., Hasson, U., Fried, I., and Malach, R. (2005). Coupling between neuronal firing, field potentials, and FMRI in human auditory cortex. Science 309, 951–954. doi: 10.1126/science.1110913
Nasr, S., Liu, N., Devaney, K. J., Yue, X., Rajimehr, R., Ungerleider, L. G., et al. (2011). Scene-selective cortical regions in human and nonhuman primates. J. Neurosci. 31, 13771–13785. doi: 10.1523/JNEUROSCI.2792-11.2011
Nir, Y., Mukamel, R., Dinstein, I., Privman, E., Harel, M., Fisch, L., et al. (2008). Interhemispheric correlations of slow spontaneous neuronal fluctuations revealed in human sensory cortex. Nat. Neurosci. 11, 1100–1108. doi: 10.1038/nn.2177
Patterson, K., Nestor, P. J., and Rogers, T. T. (2007). Where do you know what you know? The representation of semantic knowledge in the human brain. Nat. Rev. Neurosci. 8, 976–987. doi: 10.1038/nrn2277
Poeppel, D. (2014). The neuroanatomic and neurophysiological infrastructure for speech and language. Curr. Opin. Neurobiol. 28, 142–149. doi: 10.1016/j.conb.2014.07.005
Price, C. J. (2010). The anatomy of language: a review of 100 fMRI studies published in 2009. Ann. N.Y. Acad. Sci. 1191, 62–88. doi: 10.1111/j.1749-6632.2010.05444.x
Price, C. J., and Devlin, J. T. (2011). The interactive account of ventral occipitotemporal contributions to reading. Trends Cogn. Sci. 15, 246–253. doi: 10.1016/j.tics.2011.04.001
Privman, E., Fisch, L., Neufeld, M. Y., Kramer, U., Kipervasser, S., Andelman, F., et al. (2011). Antagonistic relationship between gamma power and visual evoked potentials revealed in human visual cortex. Cereb. Cortex 21, 616–624. doi: 10.1093/cercor/bhq128
Privman, E., Nir, Y., Kramer, U., Kipervasser, S., Andelman, F., Neufeld, M. Y., et al. (2007). Enhanced category tuning revealed by intracranial electroencephalograms in high-order human visual areas. J. Neurosci. 27, 6234–6242. doi: 10.1523/JNEUROSCI.4627-06.2007
Pyles, J. A., Verstynen, T. D., Schneider, W., and Tarr, M. J. (2013). Explicating the face perception network with white matter connectivity. PLoS ONE 8:e61611. doi: 10.1371/journal.pone.0061611
Saad, Z. S., and Reynolds, R. C. (2012). Suma. Neuroimage 62, 768–773. doi: 10.1016/j.neuroimage.2011.09.016
Sahin, N. T., Pinker, S., Cash, S. S., Schomer, D., and Halgren, E. (2009). Sequential processing of lexical, grammatical, and phonological information within Broca's area. Science 326, 445–449. doi: 10.1126/science.1174481
Saygin, Z. M., Osher, D. E., Koldewyn, K., Reynolds, G., Gabrieli, J. D., and Saxe, R. R. (2012). Anatomical connectivity patterns predict face selectivity in the fusiform gyrus. Nat. Neurosci. 15, 321–327. doi: 10.1038/nn.3001
Sporns, O., Tononi, G., and Kotter, R. (2005). The human connectome: a structural description of the human brain. PLoS Comput. Biol. 1:e42. doi: 10.1371/journal.pcbi.0010042
Tandon, N., Alexopoulos, A. V., Warbel, A., Najm, I. M., and Bingaman, W. E. (2009). Occipital epilepsy: spatial categorization and surgical management. J. Neurosurg. 110, 306–318. doi: 10.3171/2008.4.17490
Thorpe, S., Fize, D., and Marlot, C. (1996). Speed of processing in the human visual system. Nature 381, 520–522. doi: 10.1038/381520a0
Van Gompel, J. J., Worrell, G. A., Bell, M. L., Patrick, T. A., Cascino, G. D., Raffel, C., et al. (2008). Intracranial electroencephalography with subdural grid electrodes: techniques, complications, and outcomes. Neurosurgery 63, 498–505; discussion 505–496. doi: 10.1227/01.NEU.0000324996.37228.F8
Vidal, J. R., Freyermuth, S., Jerbi, K., Hamame, C. M., Ossandon, T., Bertrand, O., et al. (2012). Long-distance amplitude correlations in the high gamma band reveal segregation and integration within the reading network. J. Neurosci. 32, 6421–6434. doi: 10.1523/JNEUROSCI.4363-11.2012
Vidal, J. R., Ossandon, T., Jerbi, K., Dalal, S. S., Minotti, L., Ryvlin, P., et al. (2010). Category-specific visual responses: an intracranial study comparing gamma, beta, alpha, and ERP response selectivity. Front. Hum. Neurosci. 4:195. doi: 10.3389/fnhum.2010.00195
Watrous, A. J., Tandon, N., Conner, C. R., Pieters, T., and Ekstrom, A. D. (2013). Frequency-specific network connectivity increases underlie accurate spatiotemporal memory retrieval. Nat. Neurosci. 16, 349–356. doi: 10.1038/nn.3315
Weiner, K. S., Golarai, G., Caspers, J., Chuapoco, M. R., Mohlberg, H., Zilles, K., et al. (2014). The mid-fusiform sulcus: a landmark identifying both cytoarchitectonic and functional divisions of human ventral temporal cortex. Neuroimage 84, 453–465. doi: 10.1016/j.neuroimage.2013.08.068
Wessel, J. R., Conner, C. R., Aron, A. R., and Tandon, N. (2013). Chronometric electrical stimulation of right inferior frontal cortex increases motor braking. J. Neurosci. 33, 19611–19619. doi: 10.1523/JNEUROSCI.3468-13.2013
Keywords: icEEG, ECoG, cortical network dynamics, distributed cortical networks, ventral temporal cortex, face perception, fusiform face area (FFA), parahippocampal place area (PPA)
Citation: Kadipasaoglu CM, Forseth K, Whaley M, Conner CR, Rollo MJ, Baboyan VG and Tandon N (2015) Development of grouped icEEG for the study of cognitive processing. Front. Psychol. 6:1008. doi: 10.3389/fpsyg.2015.01008
Received: 03 May 2015; Accepted: 06 July 2015;
Published: 21 July 2015.
Edited by:
Timothy Michael Ellmore, The City College of New York, USAReviewed by:
Jean-Philippe Lachaux, Centre de Recherche en Neurosciences de Lyon, FranceJohn M. Zempel, Washington University, USA
Xiao Liu, National Institute of Neurological Disorders and Stroke, National Institutes of Health, USA
Copyright © 2015 Kadipasaoglu, Forseth, Whaley, Conner, Rollo, Baboyan and Tandon. This is an open-access article distributed under the terms of the Creative Commons Attribution License (CC BY). The use, distribution or reproduction in other forums is permitted, provided the original author(s) or licensor are credited and that the original publication in this journal is cited, in accordance with accepted academic practice. No use, distribution or reproduction is permitted which does not comply with these terms.
*Correspondence: Nitin Tandon, University of Texas Health Science Center at Houston, 6431 Fannin Street Suite G.550D, Houston, TX 77030, USA, nitin.tandon@uth.tmc.edu