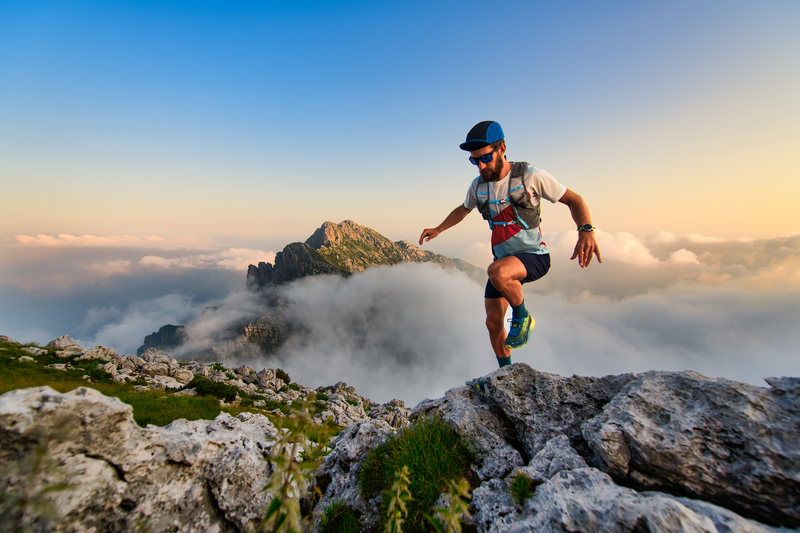
94% of researchers rate our articles as excellent or good
Learn more about the work of our research integrity team to safeguard the quality of each article we publish.
Find out more
ORIGINAL RESEARCH article
Front. Nutr. , 11 September 2024
Sec. Nutrition and Metabolism
Volume 11 - 2024 | https://doi.org/10.3389/fnut.2024.1425802
Background: Gut microbiota (GM) and metabolic alterations play pivotal roles in lung cancer (LC) development and host genetic variations are known to contribute to LC susceptibility by modulating the GM. However, the causal links among GM, metabolite, host genes, and LC remain to be fully delineated.
Method: Through bidirectional MR analyses, we examined the causal links between GM and LC, and utilized two-step mediation analysis to identify potential mediating blood metabolite. We employed diverse MR methods, including inverse-variance-weighted (IVW), weighted median, MR-Egger, weighted mode, and simple mode, to ensure a robust examination of the data. MR-Egger intercept test, Radial MR, MR-PRESSO, Cochran Q test and Leave-one-out (LOO) analysis were used for sensitivity analyses. Analyses were adjusted for smoking, alcohol intake frequency and air pollution. Linkage disequilibrium score regression and Steiger test were used to probe genetic causality. The study also explored the association between specific host genes and the abundance of gut microbes in LC patients.
Results: The presence of Bacteroides clarus was associated with an increased risk of LC (odds ratio [OR] = 1.07, 95% confidence interval [CI]: 1.03–1.11, p = 0.012), whereas the Eubacteriaceae showed a protective effect (OR = 0.82, 95% CI: 0.75–0.89, p = 0.001). These findings remained robust after False Discovery Rate (FDR) correction. Our mediator screening identified 13 blood metabolites that significantly influence LC risk after FDR correction, underscoring cystine and propionylcarnitine in reducing LC risk, while linking specific lipids and hydroxy acids to an increased risk. Our two-step mediation analysis demonstrated that the association between the bacterial pathway of synthesis of guanosine ribonucleotides and LC was mediated by Fructosyllysine, with mediated proportions of 11.38% (p = 0.037). LDSC analysis confirmed the robustness of these associations. Our study unveiled significant host genes ROBO2 may influence the abundance of pathogenic gut microbes in LC patients. Metabolic pathway analysis revealed glutathione metabolism and glutamate metabolism are the pathways most enriched with significant metabolites related to LC.
Conclusion: These findings underscore the importance of GM in the development of LC, with metabolites partly mediating this effect, and provide dietary and lifestyle recommendations for high-risk lung cancer populations.
Lung cancer (LC) remains a significant burden in contemporary societies. Recent global statistics indicate an incidence rate of 11.4% and a mortality rate of 18.0% in 2020 (1). Lung cancer is primarily divided into two main types: small-cell lung cancer (SCLC) and non-small cell lung cancer (NSCLC), with NSCLC constituting 80–85% of cases (2, 3). Currently, key risk factors for developing LC include smoking, exposure to air pollution, and certain dietary habits (4), among others. Understanding and exploring modifiable risk factors is essential in reducing lung cancer incidence, promoting early diagnosis and effective treatment.
Gut microbiota (GM) emerged as the collective term for the diverse and widespread community of microorganisms inhabiting the human gastrointestinal tract. Extensive research (5, 6) indicates that alterations in the composition, function, and host interaction of the gut microbiota are directly implicated in the pathogenesis of lung cancer. In stool samples from patients with NSCLC, there was a significant increase in the levels of Prevotella, Lactobacillus, Rikenellaceae, Streptococcus, Enterobacteriaceae, Oscillospira, and Bacteroides plebeius, in contrast to lower levels found in healthy individuals (7). Further research (8) has linked early-stage lung cancer with a significant reduction in the diversity of the gut microbiota and highlighted the elevation of specific microbes, including Bacillus and Akkermansia muciniphila, which may contribute to the development of LC. Furthermore, the gut microbiota’s role extends beyond its association with lung cancer pathogenesis to influencing the tumor microenvironment and treatment outcomes (9). A study on the gut microbiota of NSCLC patients undergoing anti-PD-1 therapy revealed significant differences in bacterial composition between responders and non-responders (10). Considering the significant correlation between gut microbiota and pulmonary pathology, researchers have introduced the “gut-lung axis” concept (11), underscoring the bidirectional communication between gut microbiota and lung immune cell recruitment, contributing to tumorigenesis and tumor progression (12). One of the crucial mechanisms is that different metabolites stimulate or inhibit the immune system to release various cytokines (13).
The human gut microbiota is influenced by diet, environmental factors, and lifestyle, affecting tumorigenesis. For example, a high-fat diet (HFD) can promote the expansion and colonization of potentially pathogenic bacteria, like Fusobacteria, reduce the intake of fermentable carbohydrates and the production of butyrate (14), shift colonocyte metabolism, increase host-derived reactive oxygen and nitrogen species (RONS), and ultimately lead to DNA damage and tumorigenesis (15). Exposure of A/J mice to a mixture of cigarette smoke carcinogens NNK and BaP triggered lung carcinogenesis, increased levels of Actinobacteria, Bifidobacterium, and Intestinimonas, and decreased levels of Alistipes, Odoribacter, and Acetatifactor (16). Despite advances in techniques like 16S rRNA sequencing and the continuous updating of data, the correlation between specific gut microbiota and lung cancer risk remains elusive due to multiple influencing factors.
The emergence of metabolomics has changed our understanding of disease mechanisms (17). By enabling metabolic reprogramming, tumor cells meet their unique bioenergetic and biosynthetic needs, resulting in alterations in the levels and types of metabolites, such as glucose, amino acids, and fatty acids (18, 19). Short-chain fatty acids (SCFAs), tryptophan metabolites, polyphenolic metabolites, and conjugated linoleic acids are known to play a protective role against CRC development (20, 21). In melanoma, SCFAs and inosine are strongly associated with disease stage and treatment efficacy (22, 23). Despite these findings, the crucial impact of microbial metabolites on lung cancer development has received only scarce attention. Existing research has indicated a significant synergistic relationship between the gut microbiome and the serum metabolic profile (24). In vivo studies on lung cancer by Hagihara et al. (25) have documented significant shifts in the gut microbiome and identified an inverse relationship between the lung and gut microbiomes in activating certain metabolic pathways, notably those related to retinol, fatty acids, and linoleic acid. In comparison to healthy individuals, lung cancer patients display distinct gut microbiome and serum metabolome profiles, with higher levels of certain glycerophospholipids and hypoxanthine in the serum (26), pointing to the possibility that metabolites are utilizable as biomarkers for lung cancer. But how do they participate in the regulation of the lungs, affect the development of lung cancer? The relevant mechanism of action remains to be systematically elucidated.
Unfortunately, the causal link between metabolites and LC remains elusive, clouded by observational study limitations like lifestyle changes post-diagnosis, medication effects, and tumor-induced metabolic changes. Ethical issues, observation periods, high financial costs, and other constraints further complicate the execution of Randomized Controlled Trials (RCTs). Additionally, the variability introduced by non-standardized patient cohorts and methodologies exacerbates the challenge of pinpointing risk-increasing metabolites. Mendelian randomization (MR), which utilizes genetic variants as instrumental variables (IVs), is a widely used technique in genetic epidemiology designed to minimize the impact of potential confounding factors (27, 28). By avoiding reverse causation bias, it supports stronger causal conclusions between exposures and clinical outcomes. Recent advancements in MR research methods have reinforced its effectiveness as a prime approach for gene-level investigations, offering a clear understanding of the links between exposures and outcomes. The role of some microorganisms in cancer development exert biological effects through various metabolic pathways. Hence, the relationship of gut miceobiota and metabolite needs to be explored (29). Mediation analysis can clearly decompose the total effect into direct and indirect effects, quantifying the correlation between GM and metabolites, thereby providing a deeper understanding of how gut microbiota influences lung cancer through metabolites. Therefore, we conducted a bidirectional MR study and two-step mediation analyses using summary statistics from the largest and most up-to-date genome-wide association studies (GWAS) of the GM, blood metabolites, and LC to dissect the associations between them.
Summary statistics on the GM, blood metabolite and LC were obtained from the respective consortia for the study design. We then explored the bidirectional two-sample MR analysis to probe the genetic causality and correlation between gut microbiota abundance and lung cancer (Figure 1). Additionally, we employed Multivariable Mendelian Randomization (MVMR) to adjust for potential confounders. And the approach we adopted for this MR analysis was grounded on 3 fundamental assumptions (Figure 1). Two-step Mediation analysis (30) was further conducted to assess the complex interactions between the GM, metabolites, and LC, pinpointing potential pathways through which gut microbiota and metabolites influence LC risk. Following this, linkage disequilibrium score regression (LDSC) was used to discern genetic associations and estimate sample overlap (31). Furthermore, instrumental variables (IVs) of GM with a direct causal association with LC were separately extracted for single nucleotide polymorphism (SNP) annotation. Finally, Significant blood metabolites related to lung cancer risk were also subject to metabolic pathway enrichment analysis. This study adhered to the reporting guidelines of the STROBE-MR (Supplementary material). Figure 1 illustrates the schematic diagram of the study design.
Figure 1. Assumptions and design of the bidirectional and mediation Mendelian randomization (MR) analyses. Firstly, a two-sample bidirectional MR was performed to investigate the causal relationships between gut microbiota and lung cancer. Then, we conducted a two-step mediation analysis to detect potential mediating metabolites (Step 1, the effect of metabolites on lung cancer; Step 2, the effect of significant gut microbiota on significant metabolites). The images for gut microbiota, blood metabolites, and lung cancer were adapted from BioRender.com.
The prospective cohort study LifeLines recruited 7,738 participants from the Dutch Microbiome Project (32). The summary statistics offered the most detailed insight into the genetic impact on human gut microbiota and bacterial pathways so far, identifying a comprehensive list of 207 taxonomies (comprising 5 phyla, 10 classes, 13 orders, 26 families, 48 genera, and 105 species) along with 205 gut microbial metabolic pathways. To ensure data quality, the majority of cohorts employed similar methods for interpolation and subsequent filtering. The full GWAS summary statistical data are available for download at NHGRI-EBI GWAS Catalog.1 Genetic data for blood metabolites also were accessed from the GWAS Catalog (see Footnote 1). Notably, this genetic data was conducted by Chen et al. (33), which was the most comprehensive analysis of genetic loci for blood metabolites thus far, identifying associations with 1,091 metabolites and 309 metabolite ratios through Genome-wide association scans with high-throughput metabolic profiling. The study encompassed a cohort of 8,299 unrelated European subjects from The Canadian Longitudinal Study of Aging (CLSA), excluding 203 European individuals identified as having first- and second-degree relatives. Among the 1,091 metabolites, 241 were defined as unknown due to as yet poorly defined chemical properties. Another 850 metabolites were chemically authenticated and allocated to eight super pathways, including lipids, amino acids, xenobiotics, nucleotides, cofactors and vitamins, carbohydrates, peptides, and energy. The analysis not only expanded the spectrum of known metabolites but also provided an in-depth exploration of metabolite ratios, which have been scarcely studied.
To bolster statistical power, validate research findings, and foster the exploration of potential new associations, summary-level GWAS data for the association analysis between the gut microbiota, metabolite and LC were obtained from two large GWAS meta-analyses. One GWAS encompassed 29,863 cases and 55,586 controls of European descent from the Transdisciplinary Research in Cancer of Lung team (TRICL) based on OncoArray and other Illumina genome-wide arrays. TRICL is a member of an organization focused on Genetic Associations and Mechanisms in Oncology (GAME-ON consortium). The data was downloaded from GWAS Catalog (see Footnote 1) (34). The other was a primary meta-analysis of the Lung Cancer Cohort Consortium (LC3), International Agency for Research on Cancer (IARC), MD Anderson Cancer Center (MDACC), St. Luke’s Radiation Research Institute (SLRI), The Institute of Cancer Research (ICR), Harvard, National Cancer Institute (NCI), Germany and deCODE, which encompassed 85,716 individuals of European descent (29,266 patients and 56,450 controls) (35). Characteristics of corresponding GWAS data sources are described in Table 1.
This study utilized data that is accessible to the public. Each study involved in the GWAS was approved by the appropriate Institutional Review Board, and consent was obtained from the participants or their legal representatives, guardians, or proxies.
The criteria for selecting IVs were determined through a methodical process: (1) Threshold Adjustment: Guided by research (36, 37), we adjusted the threshold to p < 1 × 10−5 for locus-wide significance; (2) Performing a linkage disequilibrium (LD) analysis with an r2 < 0.001 and a clumping window of 10,000 kb; (3) Excluding palindromic sequences and SNPs with allelic discrepancies between samples; (4) Removing SNPs with an F value <10 to reduce bias, using R2 and F statistics to assess instrument quality; (5) Identified LC’s main risk factors—smoking, alcohol use, and air pollution—through literature review (4, 38), we then used the PhenoScanner database (39) to remove confounders. This culminated in an MR analysis of gut microbiota and metabolites associated with at least two SNPs. Given the evidence from previous studies highlighting the adverse effects of unhealthy lifestyles, such as smoking and drinking, along with air pollution on the respiratory system, we conducted an MVMR analysis (40) to adjust for genetic liability to the aforementioned risk factors, using the same IV screening procedures and criteria as mentioned above.
To explore the link between GM, metabolites, and LC, we employed five methods: inverse variance weighted (IVW), MR-Egger, Weighted Median (WM), Simple Model, and Weighted Model. IVW combines Wald estimates across loci for multi-SNP analysis, assuming no horizontal pleiotropy (41). MR-Egger addresses pleiotropy under the InSIDE assumption, correcting biases via weighted regression (42). The WM offers reliable causal effect estimates, especially when valid IVs contribute over 50% of the weight (43), by prioritizing larger effect sizes for consistency even with less valid SNPs. The Simple Model posits a direct causal link between genetic variants and outcomes (44), minimizing biases from complex MR methods. IVW is the primary method, with the rest providing supportive results. Consistency across methods suggests reliability. We applied an FDR correction for multiple comparisons to reduce false positives, indicating that non-significant post-correction results may still suggest a potential causal link to lung cancer.
Sensitivity analysis is vital for addressing horizontal pleiotropy and heterogeneity, which can affect MR estimates significantly. Horizontal pleiotropy occurs when IVs influence the outcome through unrelated pathways. We employed a quartet of methods to identify and adjust for heterogeneity and pleiotropy: MR-Egger intercept, Radial MR, MR-PRESSO, and the Cochran Q test (45). MR-Egger intercept identifies directional pleiotropy and biases from invalid IVs. Radial MR spots outliers, allowing for reanalysis without them. MR-PRESSO assesses for heterogeneous SNPs, while Cochran Q test, with a significance threshold of p < 0.05 (46), evaluates result heterogeneity. Additionally, a leave-one-out sensitivity test determined the influence of each IV on the causal estimation. In summary, our detailed analysis of GM and blood metabolites’ potential causal links to LC followed several key criteria: (1) Primary analysis significance (IVW p < 0.05 post-FDR correction); (2) Consistent direction and magnitude across five MR methods; (3) No heterogeneity or horizontal pleiotropy in MR findings; (4) Little influence of any single SNP on MR estimates. Additionally, we calculated the statistical power of our estimates using the results_binary function, incorporating Sample Size, Significance Level, Proportion of Variance Explained, Case–Control Ratio, and Odds Ratio (47).
To further assess the causal relationship between gut microbiota and lung cancer, we conducted reverse MR analysis considering LC as the exposure and GM or metabolite as the outcome, using the same methods and settings as the forward analysis.
In MR studies, causal interpretations can be skewed by genetic links between the studied exposure and outcome. Although we excluded SNPs with direct lung cancer associations from our IVs selection, indirect SNP associations could still influence LC’s genetic structure. To confirm that observed causal effects were not muddled by genetic overlap between exposure and lung cancer, we applied LDSC regression. The threshold for genetic correlation (rg) was set at 0.05, and Genetic correlation is considered significant if the p-value is less than 0.05; otherwise, it is not significant. This method helps accurately assess genetic correlations and sample overlap, essential for verifying that our findings on gut microbiota, metabolites, and lung cancer stem from genuine causal connections, free from confounding by genetic coheritability.
Given that dysbiosis of the GM can promote the onset and progression of cancer by producing harmful metabolites (20, 48), we adopted two-step Mendelian randomization to investigate whether blood metabolites play a mediating role between the GM and LC (49). The GWAS data of blood metabolites are available in large-scale consortia or cohorts with no sample overlap with the GWASs of exposures and outcomes. Four inclusion criteria were established for each candidate mediator. First, the mediator should be causally associated with LC. Second, the mediator should have a direct causal effect on LC independently of GM. Third, GM should be causally associated with the mediator, but not vice versa. Fourth, the association of GM with the mediator and the association of the mediator with LC should be in the same direction (50). We assessed the causal impact of GM on potential mediators (β1) and the effect of these mediators on LC (β2), with the total effect (β3) representing the comprehensive impact of GM on LC. The magnitude of the mediated effect is calculated as the product of indirect effects (β1*β2), and its proportion is derived by dividing this product by the total effect, which quantifies the relative contribution or degree of influence of the gut microbiota on lung cancer through the specific mediator. We also applied the Delta method to calculate the indirect effect’s standard error (SE) (51).
To annotate SNPs, this study employed the advanced bioinformatics tool g: SNPense,2 specifically designed to map human SNP rs-codes to corresponding gene names. This tool not only provides chromosomal coordinates of SNPs but also predicts potential variant effects. Notably, g: SNPense facilitates mapping only for variants overlapping with at least one protein-coding Ensembl gene, ensuring the accuracy and relevance of the annotation. All foundational data were sourced from the Ensembl genome database and WormBase ParaSite database, providing a robust and comprehensive genomic background for our SNP annotations.
To explore how blood metabolites might influence LC, we conducted metabolic pathway analyses with MetaboAnalyst 6.0 (52, 53),3 aiming to uncover LC pathogenesis. Functional enrichment and pathway analysis modules helped identify relevant metabolite groups or pathways. The Kyoto Encyclopedia of Genes and Genomes (KEGG) and the Rapid Metabolite and Pathway Mapping (RaMP) database supported our research. We set the pathway analysis significance threshold at 0.10.
All statistical analyses were conducted using the R software, version 4.3.1. For our Mendelian randomization approach, we utilized the packages “TwoSampleMR,” “MR-PRESSO,” and “PhenoScanner” which are available in R (54). Visualization of results was performed using the packages “ggplot2” and “ComplexHeatmap” also available in R.
To start with, we examined the causal relationship between genetically determined gut microbiota and the risk of lung cancer. After rigorous quality control of IVs, we identified 3,760 significant SNPs closely associated with gut microbiota. Our MR analysis then included 200 gut microbiota. The characteristics of IVs for microbial taxa and LC are detailed in Supplementary Table S1. The F statistics for all SNPs related to GM exceeded 10, demonstrating the strong power of our IVs. After excluding confounding factors (Supplementary Table S12), we investigated the associations between specific gut microbiota taxa and LC utilizing MR. Using the IVW method, we identified 17 causal relationships between GM and LC, as shown in Table 2, Figure 2A, and Supplementary Table S3. Among these 17 microbial communities, we found suggestive evidence for a causal association between genetically predicted increases in Bacteroides_faecis (p = 0.010), Dorea (p = 0.029), Holdemania (p = 0.024), Bacteroidaceae_clarus (p = 1.80E-4), Adlercreutzia/Adlercreutzia_equolifaciens (p = 0.017), Bacteroides_xylanisolvens (p = 0.003), Negativicutes/Negativicutes_Selenomonadales (p = 0.025) and Lachnospiraceae (p = 0.025) and higher risk of LC, while Eubacteriaceae/Eubacterium (p = 7.43E-06), Alistipes_putredinis (p = 0.042), Ruminococcus_lactaris (p = 0.036), Veillonellaceae (p = 0.009), Eggerthella (p = 0.022) and Roseburia (p = 0.013) were deemed negatively correlated with LC risk. After FDR correction, Bacteroides_clarus (OR = 1.07, 95% CI: 1.03–1.11, p = 0.012) remained significant. Eubacteriaceae/Eubacterium illustrated a protective effect on LC (OR = 0.82, 95% CI: 0.75–0.89, p = 0.001). Certain microbiota traits like Enterobacteriales, Adlercreutzia and Negativicutes shared identical IVs and were categorized similarly. Bacteroides_clarus persisted as positive (p = 0.000703) even after adjusting for relevant factors, including smoking, alcohol intake and air pollution, through MVMR analysis (Supplementary Table S4). The MR-Egger, Weighted model, Weighted median and Simple model yielded causal effect estimates with magnitudes and directions comparable to those obtained from the IVW method (see Supplementary Table S3). The variability in LC explained by these taxa, as represented by R2 values, ranged from 1.58 to 3.54%, while the estimated effect sizes spanned from 57.88 to 99.96%. Importantly, our findings confirmed no heterogeneity or pleiotropy, with sensitivity analyses like MR Egger reinforcing the main results’ consistency. MR PRESSO further validated the absence of pleiotropy, as detailed in Table 2, Supplementary Table S7, and Supplementary Figures S1, S2.
Table 2. Mendelian randomization analyses of the causal effects between gut microbiota and lung cancer.
Figure 2. Circular heat map of lung cancer with an MR IVW p less than 0.05. (A) is the gut microbiota abundance; (B) is the blood metabolites.
A comprehensive set of 205 pathways was examined to determine the key targets. When solely considering the p value of the IVW, 30 functional pathways emerged as potential initial findings (see Supplementary Table S3). After FDR corrections, only the degradation of acetylene (OR = 1.22, 95% CI: 1.10–1.35, p = 0.012) and fucose (OR = 1.15, 95% CI: 1.07–1.24, p = 0.013) showed a positive correlation with LC incidence. In contrast, Engineered Biosynthesis of Taxadiene (OR = 0.93, 95% CI: 0.89–0.97, p = 0.013) and Synthesis of Guanosine Ribonucleotides (OR = 0.90, 95% CI: 0.85–0.96, p = 0.026) were negatively correlated with LC occurrence after stringent corrections (Figure 3A and Table 2). Among these, the degradation of acetylene and Synthesis of Guanosine Ribonucleotides remained significant (p = 0.0306, p = 0.0176, respectively) after MVMR validation (see Supplementary Table S4). We also performed a reverse MR analysis to explore if LC causally affects significant bacteria, following the same MR process. No significant results were found (Supplementary Table S10).
Figure 3. Mendelian randomization results of causal effects on lung cancer risk (p < 0.05). (A) Gut microbiome; (B) metabolites. ADP, Adenosine 5′-diphosphate; AMP, Adenosine 5′-monophosphate; OLG, Oleoyl-linoleoyl-glycerol; LAG, linoleoyl-arachidonoyl-glycerol.
In our study aimed at identifying potential mediators, we initially selected 1,400 metabolites to investigate their effects on LC. After performing initial filtering and completely excluding confounder-related SNPs, we identified a total of 25,879 significant SNPs. The detailed characteristics of IVs of metabolite were summarized in Supplementary Table S2. In the IVW analysis, we identified correlations between the risk of LC and 13 metabolites after rigorous FDR correction, including 10 with known chemical identities and 3 with unknown chemical identities, which were shown in Figures 2B, 3B. The 13 known metabolites were chemically assigned to the amino acid, carbohydrate, dipeptide, lipid, nucleotide and xenobiotics. They were as follows: Propionylcarnitine (OR 0.93, 95% CI: 0.90–0.97, p = 0.00092), 2-hydroxy-3-methylvalerate (OR 1.09, 95% CI: 1.04–1.14, p = 0.00034), 1-palmitoyl-2-stearoyl-gpc (16:0/18:0) (OR 1.08, 95% CI: 1.04–1.13, p = 4.69E-05), N-palmitoyl-sphingadienine (d18:2/16:0) (OR 1.10, 95% CI: 1.05–1.16, p = 0.00026), 1-stearoyl-2-arachidonoyl-gpc (18:0/20:4) (OR 1.06, 95% CI: 1.03–1.09, p = 0.00013), 3-(3-amino-3-carboxypropyl)uridine (OR 0.88, 95% CI: 0.83–0.94, p = 0.00022), 5alpha-androstan-3beta,17alpha-diol disulfate (OR 1.08, 95% CI: 1.03–1.12, p = 0.00064), Fructosyllysine (OR 1.09, 95% CI: 1.04–1.14, p = 0.00032), Gamma-glutamylcitrulline (OR 0.91, 95% CI: 0.87–0.96, p = 0.00010), Cystine (OR 0.88, 95% CI: 0.82–0.93, p = 2.63E-05), X-14056 (OR 1.12, 95% CI: 1.05–1.18, p = 0.000189), X-22509 (OR 0.89, 95% CI: 0.84–0.94, p = 2.73E-05) and X-24757 (OR 1.09, 95% CI: 1.04–1.14, p = 0.00036). Additionally, we observed correlations between the risk of LC incidence and the ratios of 5 pairs of metabolites after strict FDR corrrection (Figures 2B, 3B), and they are Adenosine 5′-diphosphate (ADP) to Adenosine 5′-monophosphate (AMP) ratio (OR 1.08, 95% CI: 1.04–1.12, p = 4.14E-05), AMP to arginine ratio (OR 0.88, 95% CI: 0.83–0.94, p = 0.00012), phosphate to 2′-deoxyuridine ratio (OR 0.93, 95% CI: 0.89–0.97, p = 0.00024), Oleoyl-linoleoyl-glycerol (OLG) (18:1/18:2) to linoleoyl-arachidonoyl-glycerol (LAG) (18:2/20:4) [1] ratio (OR 0.94 95% CI: 0.90–0.97, p = 0.00074) and OLG (18:1/18:2) to LAG (18:2/20:4) [2] ratio (OR 1.09, 95% CI: 0.92–0.98, p = 0.00070). In summary, IVW-derived estimates were significant (p < 0.05), with the direction and magnitude of IVW, MR-Egger, WM, and both weighted and simple models exhibiting consistency (see Supplementary Table S5 and Supplementary Figure S2). After the exclusion of outliers, the MR-PRESSO results further negated the presence of heterogeneous SNPs (Supplementary Table S7). The Cochran Q test (p > 0.05) and MR-Egger intercept test (p > 0.05) corroborated the absence of heterogeneity and pleiotropy (Supplementary Table S7). LOO analysis substantiated that no single SNP significantly contributed to bias in MR estimation (Supplementary Figure S4). The statistical power for all estimates exceeded 99.00% (Supplementary Table S2). The above results were obtained after excluding confounding factors (Supplementary Table S12). We also conducted a reverse MR analysis to determine if LC has a causal impact on the significant metabolites identified. Using the same MR methodology, no significant associations were found, as detailed in Supplementary Table S11. Therefore, these blood metabolites and metabolite ratios are identified as promising candidates for further investigation.
Following our examination of the influence of metabolites on LC, we selected strictly screened microbial communities and microbial pathways and further explored the potential mediation effects of GM exposures on these significant mediators (refer to Figure 4). Our analysis yielded that the bacterial pathway involving the de novo biosynthesis of guanosine ribonucleotides was found to influence LC through its impact on Fructosyllysine, with indirect effect sizes of −0.012 (p = 0.0367). The detailed MR results can be seen in Supplementary Table S6. After identifying significant mediators influencing lung cancer and the subsequent effects of exposure on mediation, we assessed the mediation effect proportions. Specifically, as Figure 4 shows, the de novo biosynthesis of guanosine ribonucleotides demonstrated an 11.38% mediation effect on LC outcomes, mediated through Fructosyllysine. These results highlight the complex relationships between GM exposures and specific blood metabolites in influencing LC, providing a deeper understanding of the pathways involved. No significant heterogeneity or pleiotropy was observed in the analysis (see Table 3 and Supplementary Table S7).
Figure 4. Mediating effects of gut microbiota on lung cancer. β1 signifies the causal influence of gut microbiota on potential mediators. β2 depicts the causal effect of these metabolite mediators on lung cancer. β3 represents the cumulative causal impact of gut microbiota on lung cancer.
Table 3. Mendelian randomization analyses of the causal effects between blood metabolites and lung cancer.
LDSC analysis was conducted on the GM and metabolites identified as having significant causal relationships through MR methods. The results of the LDSC analysis unveiled the genetic correlation (rg) between various GM, metabolites, and LC (Supplementary Table S7), with Bacteroides_clarus showing a significant correlation with LC (Rg = −0.467, Se = 0.201, p = 0.020). In contrast, other gut microbiota did not exhibit significant correlations. Furthermore, LDSC-based estimates indicated a minimal genetic correlation between LC and the following metabolites: Propionylcarnitine (Rg = −0.082, Se = 0.054, p = 0.127), 3-(3-amino-3-carboxypropyl) uridine (Rg = 0.031, Se = 0.068, p = 0.652), 2-hydroxy-3-methylvalerate (Rg = 0.048, Se = 0.060, p = 0.419), among others. This implies that MR estimates are unaffected by shared genetic components. Additionally, the SNP-heritability of metabolites was determined based on LDSC. The SNP-heritability (proportion of variance explained by genome-wide SNPs) of these metabolites ranged from 0.0094 to 0.1681 (Supplementary Table S8). The application of the Steiger test further confirmed that the observed causal relationships between GM, genetically proxied metabolites, and LC were not influenced by reverse causation, as detailed in Supplementary Table S9.
We annotated the SNPs at a locus-wide significance level of the three intestinal flora and identified 10 host genes that may be related to pathogenic intestinal microflora in LC patients (Table 4). In this study, the SNPs corresponding to the family Eubacteriaceae and the genus Eubacterium were identical, leading to the annotation of the same genes for both taxonomic categories.
Based on the analysis of eight known metabolites, our study identified five metabolic pathways potentially implicated in the pathogenesis of LC, as detailed in Table 5 and Figure 5. These pathways include the metabolism of leucine, isoleucine, and valine; glutathione metabolism; amino acid metabolism in triple-negative breast cancer cells; cysteine metabolism and glutamate metabolism. Importantly, glutathione metabolism and glutamate metabolism appear to be closely related to all other pathways.
Microbiome and metabolomics studies have significantly advanced our understanding of lung cancer’s pathogenic mechanisms (55, 56). The gut microbiota’s impact on lung cancer risk through metabolite interactions highlight the lung-gut axis as a critical area of research (57, 58). Through this comprehensive large-scale MR analysis, we have, for the first time, established a causal and mediating relationship between gut microbiota, metabolites, and lung cancer, addressing the gaps left by prior research constrained by confounders and reverse causation.
Our findings indicate that the s_Bacteroides clarus is a risk factor for LC, whereas f_Eubacteriaceae serves as a protective element against this disease. Significantly, Bacteroides clarus, a species within the g_Bacteroides, is a Gram-negative anaerobic bacterium that can decompose complex polysaccharides in the diet, and regulate the host immune system (59). Bacteroides has been proven to be a significant microbial biomarker for the non-invasive screening of colorectal neoplasms in asymptomatic individuals (60). And a notable augmentation in Bacteroides abundance was observed in the follow-up samples relative to the baseline samples among subjects who experienced a recurrence of adenomas (61). Moreover, Bacteroides clarus can coexist with other bacteria such as Fusobacterium nucleatum and Peptostreptococcus anaerobius in the intestinal epithelium, playing an important role in carcinogenesis by inducing tumor proliferation (62), enhancing inflammation (63), triggering DNA damage (64) and provide protection to tumor from immune attack (65). Indeed, the equilibrium between Bacteroidetes and Firmicutes seems to be important in the prevalence of CRC (66). The balance and composition of Eubacteriaceae in the gut microbiome are considered indicators of the host’s health status, with deviations linked to a range of conditions such as inflammatory bowel disease, obesity, and cancer. A research focusing on cholangiocarcinoma in mice involved high-throughput sequencing of prokaryotic 16S ribosomal DNA (67) revealed a decrease in the abundance of Eubacteriaceae. In contrast, the observed increase in Eubacteriaceae abundance in post-liver transplantation patients (68) underscores the microbiome’s recovery and the positive impact of the transplant on the patient’s gut flora. These contrasting situations emphasize the presence and balance of Eubacteriaceae are crucial for health. It is important to note that despite the slightly different results after MVMR adjustment, which may be due to reduced statistical power or weakened instrumental variable effects (69), the potential protective role of Eubacteriaceae cannot be denied.
Beyond the GM, our findings demonstrate the complex interaction between bacterial pathways and LC risk. Recent research indicates that two acetylene alcohols inhibited phosphorylation of IGF-1Rβ as well as reduced its target signaling molecules IRS-1 and PDK1, allowing inhibition of pro-survival signaling and showed anti-tumor effects (70), suggesting the gut bacterial acetylene degradation pathway may increase LC risks. The abundance of gut bacterial pathway for acetylene degradation is closely related to Proteobacteria and Bacteroidetes. It has been reported that α-, β-, γ-, and δ- subclasses of Proteobacteria all have the ability to degrade acetylene (71). Multiple clinical studies have indicated an increase in pathogens within Proteobacteria in fecal samples from lung cancer patients (72, 73). Understanding these dynamics is key to developing strategies that support microbiome health, thereby aiding in tumor treatment.
In the current work, we finally determined that genetically determined high levels of Cystine, Propionylcarnitine, Gamma-glutamylcitrulline and so on are associated with lower LC risk, while genetically predisposition to high levels of N-palmitoyl-sphingadienine (d18:2/16:0), 1-stearoyl-2-arachidonoyl-gpc (18:0/20:4), Fructosyllysine and so on increased risk of LC. Among the metabolites that reduce the risk of LC, cystine stands out for its antioxidative role and potential in modulating cancer progression (74). Studies show (75) that cystine supplementation rebalances redox states, enhances glutathione production, and counters drug-resistant lung cancer cell growth by reversing P-glycoprotein upregulation. These findings, combined with our research, underlining the significance of targeting the uptake and metabolic pathways of cystine in cancer therapy. In the study of propionylcarnitine, the prediction model constructed by Zhang et al. (76) proved that reduced propionylcarnitine content is significantly related to the risk of LC, which is consistent with our study results. Gamma-glutamylcitrulline, a plasma metabolite significantly correlated with glucose and lipid fluxes (77), has been previously associated with LC, along with citrulline. A study on Polish NSCLC patients found (78) that decreased level of citrulline is a clear marker of cancer. Further research (79) indicates that monitoring citrulline levels can help evaluate gut health and predict the efficacy of immune checkpoint inhibitors for lung cancer patients. Among the metabolites that contribute to the increased risk of LC, we focus on the key metabolites identified by the highest OR and the lowest p-values. Despite limited research on N-palmitoyl-sphingadienine (palmitoyl ceramide), evidence shows it promotes cell apoptosis, linking it to endothelial damage and LC risk (80). Ceramide was found to trigger apoptosis in lung adenocarcinoma cells by affecting the Txnip/Trx1 complex (81), which appears to contradict our results, suggesting a complex role for ceramide species. 1-palmitoyl-2-stearoyl-gpc (16:0/18:0) and 1-stearoyl-2-arachidonoyl-gpc (18:0/20:4), all belong to Phosphatidylcholine (PC). Studies have found that (82) PC isomers were associated with a shorter recurrence-free period and a greater likelihood of progressed T-factor and pleural invasion in post-surgery lung adenocarcinoma (ADC) patients who are smokers. Our study further refines the subclasses of PC, this detailed classification facilitates deeper investigation into its role in cancer progression.
The connection between microbial traits and plasma metabolic traits underscores the significant role of microbe-metabolite interactions in the carcinogenesis process (83). Building on this, our MR analysis also provided genetic evidence for the association between GM and blood metabolites. For instance, fructosyllysine (FL) is not only associated with an increased risk of LC but was also confirmed in our study to act as a mediator in the pathway of de novo biosynthesis of guanosine ribonucleotides, through which the gut microbiota influences LC risk. This pathway, crucial for guanosine synthesis (84)—a key molecule in RNA synthesis and LC development—impacts cancer through the ABCG transporter, reducing the proportion of side population (SP) cells in cancer (85). Additionally, activation of guanosine leads to cell cycle arrest, apoptosis, and inhibits metastasis in LC mice models (86). Understanding this mechanism is vital for creating targeted treatments, particularly for patients with KRAS mutations (87). Fructosyllysine, a key Amadori rearrangement product (ARPs) from lysine and glucose, is significantly metabolized by the gut microbiota, a factor linked to its high detection in infant feces (88). Evidence shows ARPs, including FL, trigger oxidative stress and inflammation, promoting cancer progression (89). Research by Raupbach et al. (90) on FL’s effects on colon cancer cells highlights its structure-dependent pro-inflammatory impact, underscoring the need to further study the gut microbiota’s role in FL metabolism.
A growing area of investigation in microbiome studies relevant to cancer focuses on whether dietary components mediate their beneficial health effects via the regulation of gut microbiota and metabolite. Bacteroides, originally studied for its role in causing human inflammatory diarrhoea, has emerged as a CRC-promoting bacterium (91). Secretion of active B. fragilis toxin can be suppressed by fermentable carbohydrates that are derived from plant-based diets and are constituents of healthy colonic mucus (92). In the diet of rural Africans with African Americans who consume different diets, a lower occurrence of Bacteroides rural Africans as compared to African Americans has been noticed. As rural Americans consume more diets based on low fiber and high fat and animal protein (93). In addition, supplementation of Wild melon (Cucumis melo var. agrestis) seed oil in the diet remarkably increased the production of fecal SCFAs and favorably altered the relative abundances of Eubacteriaceae at a family level (94).
Besides, environmental factors and lifestyle are also influential factors that cannot be ignored, such as air pollution. The gut is exposed to particulate matter (PM) as most of the inhaled particles are removed from the lungs to the gastrointestinal (GI) tract via mucociliary clearance. This represents a concrete manifestation of the lung-gut axis. Air pollution, particularly benzo [a] pyrene (BaP), is highly carcinogenic (95). A study explored the impact of BaP oral exposure on the gut microbiome in C57BL/6 mice (96). In the feces, compared with the control group, the relative abundance of Bacteroides was increased. Air pollution also induces changes in lipid metabolism and the redox lipidome, both of which may influence tumor development and progression (97).
Influencing GM and metabolite levels through dietary and lifestyle adjustments could be an effective prevention strategy. We suggest that lung cancer patients increase their intake of foods rich in dietary fiber, such as fruits, vegetables, and whole grains, to promote the growth of beneficial bacteria like the Eubacteriaceae family. These foods help produce protective metabolites such as SCFAs, which can reduce lung cancer risk (98, 99). At the same time, reducing high-sugar diets, such as candies, chocolates, smoked foods, and instant foods, and using cooking methods like moist heat can reduce the accumulation of harmful metabolites like fructosyllysine (100). Avoiding smoking may prevent the increase of harmful bacteria like Bacteroides clarus (101, 102), thereby reducing lung cancer risk. For individuals at high risk of lung cancer, personalized dietary and lifestyle interventions can be developed through genomic and metabolomic analyses (103) to modulate gut microbiota and reduce LC risk.
Considering the influence of the aforementioned factors, we conducted additional analyses to ensure the robustness of our results and further explored mechanisms related to host genetics. Our LDSC analysis found only Bacteroides clarus genetically correlated with LC, with no significant correlation for other microbes or metabolites. This is primarily because LDSC’s focus on genetic background of two triats (104) and the low heritability of gut microbiomes and metabolites (h2 p > 0.05), which are heavily influenced by diet, lifestyle, and medication. However, this does not dismiss a potential connection between GM, metabolites, and LC, but rather emphasizes the role of genetic and environmental factors in shaping the gut microbiome and plasma metabolites (105, 106). Future microbiome-wide association studies and metabolite-focused research may elucidate the impact of the environment on the host, thereby yielding valuable insights.
We also conducted an initial exploration of the key genes and pathways involved in the causal link between GM, blood metabolites, and LC. Our study identified 8 metabolic pathways linked to LC development, with glutathione and glutamate metabolism playing key roles (107, 108). Abnormal glutathione metabolism expression affects oxidative stress, apoptosis, and ferroptosis in NSCLC cells (109, 110). Recent studies have pointed out that the glutathione metabolism core SMS gene was highly enriched in M2 Macrophages in lung adenocarcinoma (111). Furthermore, glutamine metabolism is involved in biosynthesis and redox reactions and has been proven to be the key metabolic pathway contributing to the chemo-immunotherapy response in advanced NSCLC patients (112). Single-cell RNA-seq data analysis revealed that the glutamine metabolism gene scores of tumor cells were significantly higher than those of CD8T cells, and glutamine metabolism inhibitor could promote the proliferation of CD8T cells (113).
Studies have shown that GM and host gene expression vary during the disease process, with certain microbes stimulating oncogene transcription in NSCLC cells (114), highlighting the importance of GM-host gene interactions in lung cancer development. We linked 10 host genes, including ROBO2, to gut microbe abundance in LC, with studies (115) showing a correlation between ROBO2 and oral microbiota levels. During tumor formation, advanced sequencing techniques suggest ROBO2’s immunosuppressive function may influence the tumor microenvironment, contributing to the progression of various cancers (116–118). Interestingly, further research indicates that ROBO family-related signaling pathways may be involved in macrophage immune responses by inducing cytoskeletal changes in macrophages (119). These findings suggest that immune cells and the TME are critical factors in the disease process (120), and advanced methods like the scPagwas method (121), combined with immune cell scRNA-seq data, could be used for further exploration.
Our research stands out for its comprehensive approach. First, we analyzed 412 gut microbiotas and 1,400 blood metabolites, alongside integrating data from over 170,000 individuals from two lung cancer databases for enhanced statistical solidity. Second, to overcome limitations like reverse causality, we conducted strict MR analyses, employing SNPs as IVs, with consistency checks across five MR estimates and sensitivity analyses. Besides the host’s genetic characteristics, we considered the impact of confounding factors and applied MVMR to ensure the robustness of the results. Moreover, we employed Two-sample MR and mediation analysis to explore linear and possible nonlinear relationships. Third, apart from MR analysis, we assessed the heritability of IVs through LDSC, explored metabolite pathways related to lung cancer risk, and conducted a preliminary exploration of the gut microbiome genome.
Nonetheless, the current MR study has limitations, including the exclusive inclusion of European participants. Differences in diet, lifestyle, environmental exposures, and genetic backgrounds can influence the genetic architecture and prevalence of specific GM and metabolites across ethnicities. These differences could result in distinct causal pathways and interactions between GM, metabolites, and LC risk in non-European populations. Additionally, although we identified gene-gut microbiome associations via SNP annotation, the diagnostic and prognostic relevance of these lung cancer-specific links requires further clinical validation due to the scarcity of existing studies. Furthermore, we were unable to include detailed dietary data. The lack of comprehensive dietary information prevents us from accounting for the potential effects of various dietary patterns on gut microbiota composition and metabolism. Lastly, the inability to dynamically capture the changes in GM, metabolites, and LC development limits our understanding of how these relationships change over time and prevents us from accurately identifying the specific time points at which they interact during disease progression.
Future studies should include a more diverse range of participants to capture the full spectrum of genetic and environmental factors affecting these relationships. Additionally, if sufficient sample sizes are available, we plan to explore different lung cancer subtypes, such as small cell lung cancer, lung adenocarcinoma, and others, to offer more precise prevention and treatment strategies. We also suggest considering the collection of detailed dietary data to better understand the impact of dietary patterns on gut microbiota and their metabolites. And we plan to collect longitudinal data, including regular fecal and blood samples from participants, to monitor the dynamic changes in gut microbiota, metabolite levels, and lung cancer development. Furthermore, we will consider incorporating more environmental and lifestyle factors, such as medicine usage and exercise, through questionnaires and community collaborations. This comprehensive approach aims to elucidate how the relationships between gut microbiota, blood metabolites, and lung cancer evolve over time and in response to external factors.
Our MR and genetic analysis revealed causal links between three microbial communities, four GM pathways, thirteen metabolites, and LC, also investigating how metabolites mediate the relationship between the GM and LC. This study highlights the importance of considering gut microbiota and their metabolites in the clinical prevention and treatment of lung cancer, and proposes that interventions related to gut microbiota and metabolites could include promoting healthy lifestyles such as avoiding smoking, regulating diet by increasing the intake of foods rich in dietary fiber and reducing high-sugar diets, and taking precautions in environments with poor air quality, such as wearing masks.
The datasets presented in this study can be found in online repositories. The names of the repository/repositories and accession number(s) can be found in the article/Supplementary material.
YD: Conceptualization, Data curation, Formal analysis, Investigation, Methodology, Project administration, Resources, Software, Supervision, Validation, Visualization, Writing – original draft, Writing – review & editing. QW: Data curation, Formal analysis, Funding acquisition, Investigation, Project administration, Supervision, Writing – review & editing. ZZ: Formal analysis, Funding acquisition, Project administration, Supervision, Writing – review & editing. HZ: Formal analysis, Writing – review & editing. YH: Supervision, Writing – review & editing. AQ: Supervision, Writing – review & editing. LJ: Supervision, Writing – review & editing. YG: Formal analysis, Funding acquisition, Project administration, Supervision, Writing – review & editing.
The author(s) declare that financial support was received for the research, authorship, and/or publication of this article. This study was supported by the National Natural Science Foundation of China (no. 82474588, 82104948, 82074339), Shanghai Clinical Research Center of Traditional Chinese Medicine Oncology, Science and Technology Commission of Shanghai Municipality (no. 21MC1930500), the Project of Shanghai Municipal Public Health Bureau (no. 20214Y0177).
The authors thank participants and researchers from the GWAS study, IEU OpenGWAS project, TRICL Catalog, and related GWAS datasets for their valuable contributions and commitment to data sharing.
The authors declare that the research was conducted in the absence of any commercial or financial relationships that could be construed as a potential conflict of interest.
All claims expressed in this article are solely those of the authors and do not necessarily represent those of their affiliated organizations, or those of the publisher, the editors and the reviewers. Any product that may be evaluated in this article, or claim that may be made by its manufacturer, is not guaranteed or endorsed by the publisher.
The Supplementary material for this article can be found online at: https://www.frontiersin.org/articles/10.3389/fnut.2024.1425802/full#supplementary-material
1. Sung, H, Ferlay, J, Siegel, RL, Laversanne, M, Soerjomataram, I, Jemal, A, et al. Global Cancer statistics 2020: GLOBOCAN estimates of incidence and mortality worldwide for 36 cancers in 185 countries. CA Cancer J Clin. (2021) 71:209–49. doi: 10.3322/caac.21660
2. Travis, WD, Brambilla, E, Burke, AP, Marx, A, and Nicholson, AG. Introduction to the 2015 World Health Organization classification of tumors of the Lung, pleura, Thymus, and heart. J Thorac Oncol. (2015) 10:1240–2. doi: 10.1097/JTO.0000000000000663
3. Schabath, MB, and Cote, ML. Cancer Progress and priorities: Lung Cancer. Cancer Epidemiol Biomarkers Prev. (2019) 28:1563–79. doi: 10.1158/1055-9965.EPI-19-0221
4. Bade, BC, and Dela Cruz, CS. Lung Cancer 2020: epidemiology, etiology, and prevention. Clin Chest Med. (2020) 41:1–24. doi: 10.1016/j.ccm.2019.10.001
5. Lo, BC, Chen, GY, Núñez, G, and Caruso, R. Gut microbiota and systemic immunity in health and disease. Int Immunol. (2021) 33:197–209. doi: 10.1093/intimm/dxaa079
6. Zhao, Y, Liu, Y, Li, S, Peng, Z, Liu, X, Chen, J, et al. Role of lung and gut microbiota on lung cancer pathogenesis. J Cancer Res Clin Oncol. (2021) 147:2177–86. doi: 10.1007/s00432-021-03644-0
7. Botticelli, A, Vernocchi, P, Marini, F, Quagliariello, A, Cerbelli, B, Reddel, S, et al. Gut metabolomics profiling of non-small cell lung cancer (NSCLC) patients under immunotherapy treatment. J Transl Med. (2020) 18:49. doi: 10.1186/s12967-020-02231-0
8. Zheng, Y, Fang, Z, Xue, Y, Zhang, J, Zhu, J, Gao, R, et al. Specific gut microbiome signature predicts the early-stage lung cancer. Gut Microbes. (2020) 11:1030–42. doi: 10.1080/19490976.2020.1737487
9. Zhu, X, Li, K, Liu, G, Wu, R, Zhang, Y, Wang, S, et al. Microbial metabolite butyrate promotes anti-PD-1 antitumor efficacy by modulating T cell receptor signaling of cytotoxic CD8 T cell. Gut Microbes. (2023) 15:2249143. doi: 10.1080/19490976.2023.2249143
10. Vernocchi, P, Gili, T, Conte, F, Del Chierico, F, Conta, G, Miccheli, A, et al. Network analysis of gut microbiome and metabolome to discover Microbiota-linked biomarkers in patients affected by non-small cell Lung Cancer. Int J Mol Sci. (2020) 21:8730. doi: 10.3390/ijms21228730
11. Dang, AT, and Marsland, BJ. Microbes, metabolites, and the gut-lung axis. Mucosal Immunol. (2019) 12:843–50. doi: 10.1038/s41385-019-0160-6
12. Schuijt, TJ, Lankelma, JM, Scicluna, BP, De Sousa, EMF, Jjth, R, De Boer, JD, et al. The gut microbiota plays a protective role in the host defence against pneumococcal pneumonia. Gut. (2016) 65:575–83. doi: 10.1136/gutjnl-2015-309728
13. Zhang, D, Li, S, Wang, N, Tan, H-Y, Zhang, Z, and Feng, Y. The cross-talk between gut Microbiota and lungs in common Lung diseases. Front Microbiol. (2020) 11:301. doi: 10.3389/fmicb.2020.00301
14. Lee, J-Y, Cevallos, SA, Byndloss, MX, Tiffany, CR, Olsan, EE, Butler, BP, et al. High-fat diet and antibiotics cooperatively impair mitochondrial bioenergetics to trigger Dysbiosis that exacerbates pre-inflammatory bowel disease. Cell Host Microbe. (2020) 28:273–284.e6. doi: 10.1016/j.chom.2020.06.001
15. Greten, FR, and Grivennikov, SI. Inflammation and Cancer: triggers, mechanisms, and consequences. Immunity. (2019) 51:27–41. doi: 10.1016/j.immuni.2019.06.025
16. Qu, Z, Zhang, L, Hou, R, Ma, X, Yu, J, Zhang, W, et al. Exposure to a mixture of cigarette smoke carcinogens disturbs gut microbiota and influences metabolic homeostasis in a/J mice. Chem Biol Interact. (2021) 344:109496. doi: 10.1016/j.cbi.2021.109496
17. Sánchez-Castillo, A, Heylen, E, Hounjet, J, Savelkouls, KG, Lieuwes, NG, Biemans, R, et al. Targeting serine/glycine metabolism improves radiotherapy response in non-small cell lung cancer. Br J Cancer. (2023) 130:568–84. doi: 10.1038/s41416-023-02553-y
18. Pavlova, NN, and Thompson, CB. The emerging hallmarks of Cancer metabolism. Cell Metab. (2016) 23:27–47. doi: 10.1016/j.cmet.2015.12.006
19. Martínez-Reyes, I, and Chandel, NS. Cancer metabolism: looking forward. Nat Rev Cancer. (2021) 21:669–80. doi: 10.1038/s41568-021-00378-6
20. Li, J, Zhang, A-H, Wu, F-F, and Wang, X-J. Alterations in the gut Microbiota and their metabolites in colorectal Cancer: recent Progress and future prospects. Front Oncol. (2022) 12:841552. doi: 10.3389/fonc.2022.841552
21. Qu, R, Zhang, Y, Ma, Y, Zhou, X, Sun, L, Jiang, C, et al. Role of the gut Microbiota and its metabolites in tumorigenesis or development of colorectal Cancer. Adv Sci (Weinh). (2023) 10:e2205563. doi: 10.1002/advs.202205563
22. Spencer, CN, McQuade, JL, Gopalakrishnan, V, McCulloch, JA, Vetizou, M, Cogdill, AP, et al. Dietary fiber and probiotics influence the gut microbiome and melanoma immunotherapy response. Science. (2021) 374:1632–40. doi: 10.1126/science.aaz7015
23. Vandoni, G, D’Amico, F, Fabbrini, M, Mariani, L, Sieri, S, Casirati, A, et al. Gut Microbiota, metabolome, and body composition signatures of response to therapy in patients with advanced melanoma. Int J Mol Sci. (2023) 24:11611. doi: 10.3390/ijms241411611
24. Chen, Y, Yan, H, Yan, L, Wang, X, Che, X, Hou, K, et al. Hypoxia-induced ALDH3A1 promotes the proliferation of non-small-cell lung cancer by regulating energy metabolism reprogramming. Cell Death Dis. (2023) 14:617–1. doi: 10.1038/s41419-023-06142-y
25. Hagihara, M, Kato, H, Yamashita, M, Shibata, Y, Umemura, T, Mori, T, et al. Lung cancer progression alters lung and gut microbiomes and lipid metabolism. Heliyon. (2024) 10:e23509. doi: 10.1016/j.heliyon.2023.e23509
26. Zhao, F, An, R, Wang, L, Shan, J, and Wang, X. Specific gut microbiome and serum metabolome changes in Lung Cancer patients. Front Cell Infect Microbiol. (2021) 11:725284. doi: 10.3389/fcimb.2021.725284
27. Lawlor, DA, Harbord, RM, Sterne, JAC, Timpson, N, and Davey, SG. Mendelian randomization: using genes as instruments for making causal inferences in epidemiology. Stat Med. (2008) 27:1133–63. doi: 10.1002/sim.3034
28. Davey Smith, G, and Hemani, G. Mendelian randomization: genetic anchors for causal inference in epidemiological studies. Hum Mol Genet. (2014) 23:R89–98. doi: 10.1093/hmg/ddu328
29. Scott, AJ, Alexander, JL, Merrifield, CA, Cunningham, D, Jobin, C, Brown, R, et al. International Cancer microbiome consortium consensus statement on the role of the human microbiome in carcinogenesis. Gut. (2019) 68:1624–32. doi: 10.1136/gutjnl-2019-318556
30. Zeng, P, Shao, Z, and Zhou, X. Statistical methods for mediation analysis in the era of high-throughput genomics: current successes and future challenges. Comput Struct Biotechnol J. (2021) 19:3209–24. doi: 10.1016/j.csbj.2021.05.042
31. Pettit, RW, and Amos, CI. Linkage disequilibrium score statistic regression for identifying novel trait associations. Curr Epidemiol Rep. (2022) 9:190–9. doi: 10.1007/s40471-022-00297-6
32. Lopera-Maya, EA, Kurilshikov, A, van der Graaf, A, Hu, S, Andreu-Sánchez, S, Chen, L, et al. Effect of host genetics on the gut microbiome in 7,738 participants of the Dutch microbiome project. Nat Genet. (2022) 54:143–51. doi: 10.1038/s41588-021-00992-y
33. Chen, Y, Lu, T, Pettersson-Kymmer, U, Stewart, ID, Butler-Laporte, G, Nakanishi, T, et al. Genomic atlas of the plasma metabolome prioritizes metabolites implicated in human diseases. Nat Genet. (2023) 55:44–53. doi: 10.1038/s41588-022-01270-1
34. Buniello, A, MacArthur, JAL, Cerezo, M, Harris, LW, Hayhurst, J, Malangone, C, et al. The NHGRI-EBI GWAS catalog of published genome-wide association studies, targeted arrays and summary statistics 2019. Nucleic Acids Res. (2019) 47:D1005–12. doi: 10.1093/nar/gky1120
35. McKay, JD, Hung, RJ, Han, Y, Zong, X, Carreras-Torres, R, Christiani, DC, et al. Large-scale association analysis identifies new lung cancer susceptibility loci and heterogeneity in genetic susceptibility across histological subtypes. Nat Genet. (2017) 49:1126–32. doi: 10.1038/ng.3892
36. Li, Y, Wang, K, Zhang, Y, Yang, J, Wu, Y, and Zhao, M. Revealing a causal relationship between gut microbiota and lung cancer: a Mendelian randomization study. Front Cell Infect Microbiol. (2023) 13:1200299. doi: 10.3389/fcimb.2023.1200299
37. Yun, Z, Guo, Z, Li, X, Shen, Y, Nan, M, Dong, Q, et al. Genetically predicted 486 blood metabolites in relation to risk of colorectal cancer: a Mendelian randomization study. Cancer Med. (2023) 12:13784–99. doi: 10.1002/cam4.6022
38. Marshall, EA, Ng, KW, Kung, SHY, Conway, EM, Martinez, VD, Halvorsen, EC, et al. Emerging roles of T helper 17 and regulatory T cells in lung cancer progression and metastasis. Mol Cancer. (2016) 15:67. doi: 10.1186/s12943-016-0551-1
39. Yang, M, Wan, X, Zheng, H, Xu, K, Xie, J, Yu, H, et al. No evidence of a genetic causal relationship between ankylosing spondylitis and gut Microbiota: a two-sample Mendelian randomization study. Nutrients. (2023) 15:1057. doi: 10.3390/nu15041057
40. Burgess, S, Daniel, RM, Butterworth, AS, and Thompson, SGEPIC-InterAct Consortium. Network Mendelian randomization: using genetic variants as instrumental variables to investigate mediation in causal pathways. Int J Epidemiol. (2015) 44:484–95. doi: 10.1093/ije/dyu176
41. Burgess, S, Dudbridge, F, and Thompson, SG. Combining information on multiple instrumental variables in Mendelian randomization: comparison of allele score and summarized data methods. Stat Med. (2016) 35:1880–906. doi: 10.1002/sim.6835
42. Burgess, S, and Thompson, SG. Interpreting findings from Mendelian randomization using the MR-egger method. Eur J Epidemiol. (2017) 32:377–89. doi: 10.1007/s10654-017-0255-x
43. Bowden, J, Davey Smith, G, Haycock, PC, and Burgess, S. Consistent estimation in Mendelian randomization with some invalid instruments using a weighted median estimator. Genet Epidemiol. (2016) 40:304–14. doi: 10.1002/gepi.21965
44. Wang, L, and Ding, C. Major depression disorder may causally associate with the increased atrial fibrillation risk: evidence from two-sample mendelian randomization analyses. BMC Med Genet. (2023) 16:144. doi: 10.1186/s12920-023-01565-0
45. Verbanck, M, Chen, C-Y, Neale, B, and Do, R. Detection of widespread horizontal pleiotropy in causal relationships inferred from Mendelian randomization between complex traits and diseases. Nat Genet. (2018) 50:693–8. doi: 10.1038/s41588-018-0099-7
46. Cohen, JF, Chalumeau, M, Cohen, R, Korevaar, DA, Khoshnood, B, and Bossuyt, PMM. Cochran’s Q test was useful to assess heterogeneity in likelihood ratios in studies of diagnostic accuracy. J Clin Epidemiol. (2015) 68:299–306. doi: 10.1016/j.jclinepi.2014.09.005
47. Brion, M-JA, Shakhbazov, K, and Visscher, PM. Calculating statistical power in Mendelian randomization studies. Int J Epidemiol. (2013) 42:1497–501. doi: 10.1093/ije/dyt179
48. Fan, Y, and Pedersen, O. Gut microbiota in human metabolic health and disease. Nat Rev Microbiol. (2021) 19:55–71. doi: 10.1038/s41579-020-0433-9
49. Carter, AR, Sanderson, E, Hammerton, G, Richmond, RC, Davey Smith, G, Heron, J, et al. Mendelian randomisation for mediation analysis: current methods and challenges for implementation. Eur J Epidemiol. (2021) 36:465–78. doi: 10.1007/s10654-021-00757-1
50. Ye, C-J, Liu, D, Chen, M-L, Kong, L-J, Dou, C, Wang, Y-Y, et al. Mendelian randomization evidence for the causal effect of mental well-being on healthy aging. Nat Hum Behav. (2024). doi: 10.1038/s41562-024-01905-9
51. Alevizakos, V, and Koukouvinos, C. An asymptotic confidence interval for the process capability index Cpm. Commun. Stat. - Theory Methods. (2019) 48:5138–44. doi: 10.1080/03610926.2018.1508713
52. Chong, J, and Xia, J. Using MetaboAnalyst 4.0 for metabolomics data analysis, interpretation, and integration with other omics data. Methods Mol Biol. (2020) 2104:337–60. doi: 10.1007/978-1-0716-0239-3_17
53. Pang, Z, Lu, Y, Zhou, G, Hui, F, Xu, L, Viau, C, et al. MetaboAnalyst 6.0: towards a unified platform for metabolomics data processing, analysis and interpretation. Nucleic Acids Res. (2024) 52:W398–406. doi: 10.1093/nar/gkae253
54. Hemani, G, Zheng, J, Elsworth, B, Wade, KH, Haberland, V, Baird, D, et al. The MR-base platform supports systematic causal inference across the human phenome. eLife. (2018) 7:e34408. doi: 10.7554/eLife.34408
55. Li, Z, Chen, S, He, X, Gong, S, Sun, L, and Weng, L. SLC3A2 promotes tumor-associated macrophage polarization through metabolic reprogramming in lung cancer. Cancer Sci. (2023) 114:2306–17. doi: 10.1111/cas.15760
56. Lin, Z, Li, J, Zhang, J, Feng, W, Lu, J, Ma, X, et al. Metabolic reprogramming driven by IGF2BP3 promotes acquired resistance to EGFR inhibitors in non-small cell Lung Cancer. Cancer Res. (2023) 83:2187–207. doi: 10.1158/0008-5472.CAN-22-3059
57. Zhu, Z, Cai, J, Hou, W, Xu, K, Wu, X, Song, Y, et al. Microbiome and spatially resolved metabolomics analysis reveal the anticancer role of gut Akkermansia muciniphila by crosstalk with intratumoral microbiota and reprogramming tumoral metabolism in mice. Gut Microbes. (2023) 15:2166700. doi: 10.1080/19490976.2023.2166700
58. Guo, Y, Chen, X, Gong, P, Li, G, Yao, W, and Yang, W. The gut–organ-Axis concept: advances the application of gut-on-Chip Technology. Int J Mol Sci. (2023) 24:4089. doi: 10.3390/ijms24044089
59. Kim, Y, Kim, G, Kim, S, Cho, B, Kim, S-Y, Do, E-J, et al. Fecal microbiota transplantation improves anti-PD-1 inhibitor efficacy in unresectable or metastatic solid cancers refractory to anti-PD-1 inhibitor. Cell Host Microbe. (2024) 32:1380–1393.e9. doi: 10.1016/j.chom.2024.06.010
60. Liang, JQ, Wong, SH, Szeto, CH, Chu, ES, Lau, HC, Chen, Y, et al. Fecal microbial DNA markers serve for screening colorectal neoplasm in asymptomatic subjects. J Gastroenterol Hepatol. (2021) 36:1035–43. doi: 10.1111/jgh.15171
61. Long, Y, Tang, L, Zhou, Y, Zhao, S, and Zhu, H. Causal relationship between gut microbiota and cancers: a two-sample Mendelian randomisation study. BMC Med. (2023) 21:66. doi: 10.1186/s12916-023-02761-6
62. Yang, Y, Weng, W, Peng, J, Hong, L, Yang, L, Toiyama, Y, et al. Fusobacterium nucleatum increases proliferation of colorectal Cancer cells and tumor development in mice by activating toll-like receptor 4 signaling to nuclear factor-κB, and up-regulating expression of MicroRNA-21. Gastroenterology. (2017) 152:851–866.e24. doi: 10.1053/j.gastro.2016.11.018
63. Chung, L, Orberg, ET, Geis, AL, Chan, JL, Fu, K, DeStefano Shields, CE, et al. Bacteroides fragilis toxin coordinates a pro-carcinogenic inflammatory Cascade via targeting of colonic epithelial cells. Cell Host Microbe. (2018) 23:421. doi: 10.1016/j.chom.2018.02.004
64. Rubinstein, MR, Wang, X, Liu, W, Hao, Y, Cai, G, and Han, YW. Fusobacterium nucleatum promotes colorectal carcinogenesis by modulating E-cadherin/β-catenin signaling via its FadA Adhesin. Cell Host Microbe. (2013) 14:195–206. doi: 10.1016/j.chom.2013.07.012
65. Long, X, Wong, CC, Tong, L, Chu, ESH, Ho Szeto, C, Go, MYY, et al. Peptostreptococcus anaerobius promotes colorectal carcinogenesis and modulates tumour immunity. Nat Microbiol. (2019) 4:2319–30. doi: 10.1038/s41564-019-0541-3
66. Sinha, R, Ahn, J, Sampson, JN, Shi, J, Yu, G, Xiong, X, et al. Fecal Microbiota, fecal metabolome, and colorectal Cancer interrelations. PLoS One. (2016) 11:e0152126. doi: 10.1371/journal.pone.0152126
67. Plieskatt, JL, Deenonpoe, R, Mulvenna, JP, Krause, L, Sripa, B, Bethony, JM, et al. Infection with the carcinogenic liver fluke Opisthorchis viverrini modifies intestinal and biliary microbiome. FASEB J. (2013) 27:4572–84. doi: 10.1096/fj.13-232751
68. Sun, L-Y, Yang, Y-S, Qu, W, Zhu, Z-J, Wei, L, Ye, Z-S, et al. Gut microbiota of liver transplantation recipients. Sci Rep. (2017) 7:3762. doi: 10.1038/s41598-017-03476-4
69. Burgess, S, and Thompson, SG. Multivariable Mendelian randomization: the use of pleiotropic genetic variants to estimate causal effects. Am J Epidemiol. (2015) 181:251–60. doi: 10.1093/aje/kwu283
70. Zovko, A, Novak, M, Hååg, P, Kovalerchick, D, Holmlund, T, Färnegårdh, K, et al. Compounds from the marine sponge Cribrochalina vasculum offer a way to target IGF-1R mediated signaling in tumor cells. Oncotarget. (2016) 7:50258–76. doi: 10.18632/oncotarget.10361
71. Spormann, AM, and Widdel, F. Metabolism of alkylbenzenes, alkanes, and other hydrocarbons in anaerobic bacteria. Biodegradation. (2000) 11:85–105. doi: 10.1023/a:1011122631799
72. Jiang, H, Zeng, W, Zhang, X, Li, Y, Wang, Y, Peng, A, et al. Gut microbiota and its metabolites in non-small cell lung cancer and brain metastasis: from alteration to potential microbial markers and drug targets. Front Cell Infect Microbiol. (2023) 13:1211855. doi: 10.3389/fcimb.2023.1211855
73. Lu, X, Xiong, L, Zheng, X, Yu, Q, Xiao, Y, and Xie, Y. Structure of gut microbiota and characteristics of fecal metabolites in patients with lung cancer. Front Cell Infect Microbiol. (2023) 13:1170326. doi: 10.3389/fcimb.2023.1170326
74. Zhang, K-R, Zhang, Y-F, Lei, H-M, Tang, Y-B, Ma, C-S, Lv, Q-M, et al. Targeting AKR1B1 inhibits glutathione de novo synthesis to overcome acquired resistance to EGFR-targeted therapy in lung cancer. Sci Transl Med. (2021) 13:eabg6428. doi: 10.1126/scitranslmed.abg6428
75. Li, S, Cao, B, Lu, Z, Sun, R, Guo, S, Xie, Y, et al. Cystine supplementation rebalances the redox homeostasis of microenvironment in non-small cell lung cancer cells and reverses their resistance to docetaxel. Acta Pharmacol Sin. (2021) 42:2132–43. doi: 10.1038/s41401-020-00610-3
76. Zhang, X, Wang, C, Li, C, and Zhao, H. Development and internal validation of nomograms based on plasma metabolites to predict non-small cell lung cancer risk in smoking and nonsmoking populations. Thorac Cancer. (2023) 14:1719–31. doi: 10.1111/1759-7714.14917
77. Hartstra, AV, de Groot, PF, Mendes Bastos, D, Levin, E, Serlie, MJ, Soeters, MR, et al. Correlation of plasma metabolites with glucose and lipid fluxes in human insulin resistance. Obes Sci Pract. (2020) 6:340–9. doi: 10.1002/osp4.402
78. Klupczynska, A, Dereziński, P, Dyszkiewicz, W, Pawlak, K, Kasprzyk, M, and Kokot, ZJ. Evaluation of serum amino acid profiles’ utility in non-small cell lung cancer detection in polish population. Lung Cancer. (2016) 100:71–6. doi: 10.1016/j.lungcan.2016.04.008
79. Ocáriz-Díez, M, Cruellas, M, Gascón, M, Lastra, R, Martínez-Lostao, L, Ramírez-Labrada, A, et al. Microbiota and Lung Cancer. Opportunities and challenges for improving immunotherapy efficacy. Front Oncologia. (2020) 10:568939. doi: 10.3389/fonc.2020.568939
80. Petrache, I, Pujadas, E, Ganju, A, Serban, KA, Borowiec, A, Babbs, B, et al. Marked elevations in lung and plasma ceramide in COVID-19 linked to microvascular injury. JCI Insight. (2023) 8:e156104. doi: 10.1172/jci.insight.156104
81. Shi, Y, Jin, Y, Liu, F, Jiang, J, Cao, J, Lu, Y, et al. Ceramide induces the apoptosis of non-small cell lung cancer cells through the Txnip/Trx1 complex. Int J Mol Med. (2021) 47:85. doi: 10.3892/ijmm.2021.4918
82. Takanashi, Y, Kahyo, T, Hayakawa, T, Sekihara, K, Kawase, A, Kondo, M, et al. Lipid biomarkers that reflect postoperative recurrence risk in lung cancer patients who smoke: a case–control study. Lipids Health Dis. (2023) 22:15. doi: 10.1186/s12944-023-01778-3
83. Gao, R, Wu, C, Zhu, Y, Kong, C, Zhu, Y, Gao, Y, et al. Integrated analysis of colorectal Cancer reveals cross-cohort gut microbial signatures and associated serum metabolites. Gastroenterology. (2022) 163:1024–1037.e9. doi: 10.1053/j.gastro.2022.06.069
84. Huang, F, Huffman, KE, Wang, Z, Wang, X, Li, K, Cai, F, et al. Guanosine triphosphate links MYC-dependent metabolic and ribosome programs in small-cell lung cancer. J Clin Invest. (2021) 131:139929. doi: 10.1172/JCI139929
85. Scharenberg, CW, Harkey, MA, and Torok-Storb, B. The ABCG2 transporter is an efficient Hoechst 33342 efflux pump and is preferentially expressed by immature human hematopoietic progenitors. Blood. (2002) 99:507–512. doi: 10.1182/blood.v99.2.507
86. AboYoussef, AM, Khalaf, MM, Malak, MN, and Hamzawy, MA. Repurposing of sildenafil as antitumour; induction of cyclic guanosine monophosphate/protein kinase G pathway, caspase-dependent apoptosis and pivotal reduction of nuclear factor kappa light chain enhancer of activated B cells in lung cancer. J Pharm Pharmacol. (2021) 73:1080–91. doi: 10.1093/jpp/rgab049
87. Negri, F, Bottarelli, L, de’Angelis, GL, and Gnetti, L. KRAS: a Druggable target in Colon Cancer patients. Int J Mol Sci. (2022) 23:4120. doi: 10.3390/ijms23084120
88. Filipp, L, Bausch, F, Neuhaus, LS, Flade, J, and Henle, T. Metabolization of the Amadori product N-ε-Fructosyllysine by probiotic Bacteria. J Agric Food Chem. (2024) 72:2718–26. doi: 10.1021/acs.jafc.3c07927
89. Yamagishi, S . Role of advanced glycation end products (AGEs) and receptor for AGEs (RAGE) in vascular damage in diabetes. Exp Gerontol. (2011) 46:217–24. doi: 10.1016/j.exger.2010.11.007
90. Raupbach, J, Müller, SK, Schnell, V, Friedrich, S, Hellwig, A, Grune, T, et al. The effect of free and protein-bound Maillard reaction products N-ε-Carboxymethyllysine, N-ε-Fructosyllysine, and Pyrraline on Nrf2 and NFκB in HCT 116 cells. Mol Nutr Food Res. (2023) 67:e2300137. doi: 10.1002/mnfr.202300137
91. Sears, CL, Geis, AL, and Housseau, F. Bacteroides fragilis subverts mucosal biology: from symbiont to colon carcinogenesis. J Clin Invest. (2014) 124:4166–72. doi: 10.1172/JCI72334
92. Casterline, BW, Hecht, AL, Choi, VM, and Bubeck, WJ. The Bacteroides fragilis pathogenicity island links virulence and strain competition. Gut Microbes. (2017) 8:374–83. doi: 10.1080/19490976.2017.1290758
93. Ou, J, Carbonero, F, Zoetendal, EG, DeLany, JP, Wang, M, Newton, K, et al. Diet, microbiota, and microbial metabolites in colon cancer risk in rural Africans and African Americans. Am J Clin Nutr. (2013) 98:111–20. doi: 10.3945/ajcn.112.056689
94. Hao, W, Zhu, H, Chen, J, Kwek, E, He, Z, Liu, J, et al. Wild melon seed oil reduces plasma cholesterol and modulates gut Microbiota in Hypercholesterolemic hamsters. J Agric Food Chem. (2020) 68:2071–81. doi: 10.1021/acs.jafc.9b07302
95. Baan, R, Grosse, Y, Straif, K, Secretan, B, El Ghissassi, F, Bouvard, V, et al. A review of human carcinogens—part F: chemical agents and related occupations. Lancet Oncol. (2009) 10:1143–4. doi: 10.1016/S1470-2045(09)70358-4
96. Ribière, C, Peyret, P, Parisot, N, Darcha, C, Déchelotte, PJ, Barnich, N, et al. Oral exposure to environmental pollutant benzo[a]pyrene impacts the intestinal epithelium and induces gut microbial shifts in murine model. Sci Rep. (2016) 6:31027. doi: 10.1038/srep31027
97. Feng, J, Cavallero, S, Hsiai, T, and Li, R. Impact of air pollution on intestinal redox lipidome and microbiome. Free Radic Biol Med. (2020) 151:99–110. doi: 10.1016/j.freeradbiomed.2019.12.044
98. den Besten, G, van Eunen, K, Groen, AK, Venema, K, Reijngoud, D-J, and Bakker, BM. The role of short-chain fatty acids in the interplay between diet, gut microbiota, and host energy metabolism. J Lipid Res. (2013) 54:2325–40. doi: 10.1194/jlr.R036012
99. De Filippo, C, Cavalieri, D, Di Paola, M, Ramazzotti, M, Poullet, JB, Massart, S, et al. Impact of diet in shaping gut microbiota revealed by a comparative study in children from Europe and rural Africa. Proc Natl Acad Sci USA. (2010) 107:14691–6. doi: 10.1073/pnas.1005963107
100. Uribarri, J, Woodruff, S, Goodman, S, Cai, W, Chen, X, Pyzik, R, et al. Advanced glycation end products in foods and a practical guide to their reduction in the diet. J Am Diet Assoc. (2010) 110:911–916.e12. doi: 10.1016/j.jada.2010.03.018
101. Biedermann, L, Zeitz, J, Mwinyi, J, Sutter-Minder, E, Rehman, A, Ott, SJ, et al. Smoking cessation induces profound changes in the composition of the intestinal microbiota in humans. PLoS One. (2013) 8:e59260. doi: 10.1371/journal.pone.0059260
102. Ma, W, Zhang, W, Shen, L, Liu, J, Yang, F, Maskey, N, et al. Can smoking cause differences in urine microbiome in male patients with bladder Cancer? A retrospective study. Front Oncol. (2021) 11:677605. doi: 10.3389/fonc.2021.677605
103. Wang, Z, Klipfell, E, Bennett, BJ, Koeth, R, Levison, BS, Dugar, B, et al. Gut flora metabolism of phosphatidylcholine promotes cardiovascular disease. Nature. (2011) 472:57–63. doi: 10.1038/nature09922
104. Ning, Z, Pawitan, Y, and Shen, X. High-definition likelihood inference of genetic correlations across human complex traits. Nat Genet. (2020) 52:859–64. doi: 10.1038/s41588-020-0653-y
105. Grieneisen, L, Dasari, M, Gould, TJ, Björk, JR, Grenier, J-C, Yotova, V, et al. Gut microbiome heritability is nearly universal but environmentally contingent. Science. (2021) 373:181–6. doi: 10.1126/science.aba5483
106. Drouard, G, Hagenbeek, FA, Whipp, AM, Pool, R, Hottenga, JJ, Jansen, R, et al. (2023). Longitudinal multi-omics study reveals common etiology underlying association between plasma proteome and BMI trajectories in adolescent and young adult twins. BMC Medicine. 21:508. doi: 10.1186/s12916-023-03198-7
107. Zhang, J, Pavlova, NN, and Thompson, CB. Cancer cell metabolism: the essential role of the nonessential amino acid, glutamine. EMBO J. (2017) 36:1302–15. doi: 10.15252/embj.201696151
108. Pan, T, Han, D, Xu, Y, Peng, W, Bai, L, Zhou, X, et al. LC-MS based metabolomics study of the effects of EGCG on A549 cells. Front Pharmacol. (2021) 12:732716. doi: 10.3389/fphar.2021.732716
109. Song, G-Q, Wu, P, Dong, X-M, Cheng, L-H, Lu, H-Q, and Lin, Y-Y. Elemene induces cell apoptosis via inhibiting glutathione synthesis in lung adenocarcinoma. J Ethnopharmacol. (2023) 311:116409. doi: 10.1016/j.jep.2023.116409
110. Cao, P, Gu, J, Liu, M, Wang, Y, Chen, M, Jiang, Y, et al. BRMS1L confers anticancer activity in non-small cell lung cancer by transcriptionally inducing a redox imbalance in the GPX2-ROS pathway. Transl Oncol. (2024) 41:101870. doi: 10.1016/j.tranon.2023.101870
111. Qiu, J, Wang, Z, Yu, Y, Zheng, Y, Li, M, and Lin, C. Prognostic and immunological implications of glutathione metabolism genes in lung adenocarcinoma: a focus on the core gene SMS and its impact on M2 macrophage polarization. Int Immunopharmacol. (2024) 132:111940. doi: 10.1016/j.intimp.2024.111940
112. Mei, L, Zhang, Z, Li, X, Yang, Y, and Qi, R. Metabolomics profiling in prediction of chemo-immunotherapy efficiency in advanced non-small cell lung cancer. Front Oncol. (2022) 12:1025046. doi: 10.3389/fonc.2022.1025046
113. Chen, J, Wang, R, Liu, Z, Fan, J, Liu, S, Tan, S, et al. Unbalanced glutamine partitioning between CD8T cells and Cancer cells accompanied by immune cell dysfunction in hepatocellular carcinoma. Cells. (2022) 11:3924. doi: 10.3390/cells11233924
114. Kim, TD . Bacterial hormone-sensitive lipases (bHSLs): emerging enzymes for biotechnological applications. J Microbiol Biotechnol. (2017) 27:1907–15. doi: 10.4014/jmb.1708.08004
115. Richter, GM, Kruppa, J, Keceli, HG, Ataman-Duruel, ET, Graetz, C, Pischon, N, et al. Epigenetic adaptations of the masticatory mucosa to periodontal inflammation. Clin Epigenetics. (2021) 13:203. doi: 10.1186/s13148-021-01190-7
116. Le, TM, Nguyen, HDT, Lee, E, Lee, D, Choi, YS, Cho, J, et al. Transcriptomic immune profiles can represent the tumor immune microenvironment related to the tumor budding histology in uterine cervical Cancer. Genes (Basel). (2022) 13:1405. doi: 10.3390/genes13081405
117. Liu, Y, Han, Y-S, Wang, J-F, Pang, Z-Q, Wang, J-S, Zhang, L, et al. A new immune-related gene signature predicts the prognosis and immune escape of bladder cancer. Cancer Biomark. (2023) 38:567–81. doi: 10.3233/CBM-230190
118. Geraldo, LH, Xu, Y, Jacob, L, Pibouin-Fragner, L, Rao, R, Maissa, N, et al. SLIT2/ROBO signaling in tumor-associated microglia and macrophages drives glioblastoma immunosuppression and vascular dysmorphia. J Clin Invest. (2021) 131:e141083. doi: 10.1172/JCI141083
119. Bhosle, VK, Mukherjee, T, Huang, Y-W, Patel, S, Pang, BWF, Liu, G-Y, et al. SLIT2/ROBO1-signaling inhibits macropinocytosis by opposing cortical cytoskeletal remodeling. Nat Commun. (2020) 11:4112. doi: 10.1038/s41467-020-17651-1
120. Ma, Y, Qiu, F, Deng, C, Li, J, Huang, Y, Wu, Z, et al. Integrating single-cell sequencing data with GWAS summary statistics reveals CD16+monocytes and memory CD8+T cells involved in severe COVID-19. Genome Med. (2022) 14:16. doi: 10.1186/s13073-022-01021-1
Keywords: lung cancer, gut microbiota, metabolite, Mendelian randomization, gene, mediation analysis
Citation: Du Y, Wang Q, Zheng Z, Zhou H, Han Y, Qi A, Jiao L and Gong Y (2024) Gut microbiota influence on lung cancer risk through blood metabolite mediation: from a comprehensive Mendelian randomization analysis and genetic analysis. Front. Nutr. 11:1425802. doi: 10.3389/fnut.2024.1425802
Received: 30 April 2024; Accepted: 26 August 2024;
Published: 11 September 2024.
Edited by:
Jingyuan Xiong, Sichuan University, ChinaReviewed by:
Guang-Liang Chen, Fudan University, ChinaCopyright © 2024 Du, Wang, Zheng, Zhou, Han, Qi, Jiao and Gong. This is an open-access article distributed under the terms of the Creative Commons Attribution License (CC BY). The use, distribution or reproduction in other forums is permitted, provided the original author(s) and the copyright owner(s) are credited and that the original publication in this journal is cited, in accordance with accepted academic practice. No use, distribution or reproduction is permitted which does not comply with these terms.
*Correspondence: Lijing Jiao, amxqOTY5QDE2My5jb20=; Yabin Gong, Z29uZ3lhYmluQGhvdG1haWwuY29t
†These authors have contributed equally to this work
Disclaimer: All claims expressed in this article are solely those of the authors and do not necessarily represent those of their affiliated organizations, or those of the publisher, the editors and the reviewers. Any product that may be evaluated in this article or claim that may be made by its manufacturer is not guaranteed or endorsed by the publisher.
Research integrity at Frontiers
Learn more about the work of our research integrity team to safeguard the quality of each article we publish.