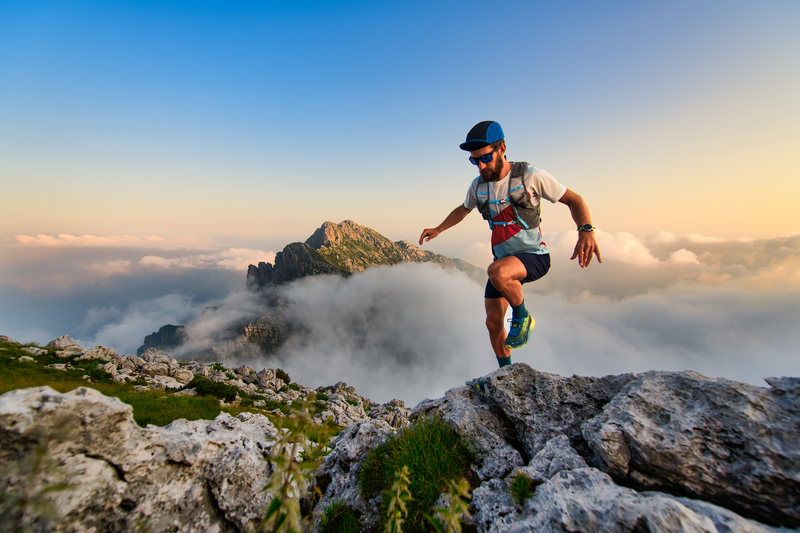
94% of researchers rate our articles as excellent or good
Learn more about the work of our research integrity team to safeguard the quality of each article we publish.
Find out more
ORIGINAL RESEARCH article
Front. Nutr. , 25 September 2024
Sec. Nutrigenomics
Volume 11 - 2024 | https://doi.org/10.3389/fnut.2024.1387514
This article is part of the Research Topic Addictions and Eating Behavior View all 9 articles
Objective: To test associations of candidate obesity-related single nucleotide polymorphisms (SNPs) and obesity polygenic risk scores (PRS) with neural reward reactivity to food cues.
Methods: After consuming a pre-load meal, 9–12-year-old children completed a functional magnetic resonance imaging (fMRI) paradigm with exposure to food and non-food commercials. Genetic exposures included FTO rs9939609, MC4R rs571312, and a pediatric-specific obesity PRS. A targeted region-of-interest (ROI) analysis for 7 bilateral reward regions and a whole-brain analysis were conducted. Independent associations between each genetic factor and reward responsivity to food cues in each ROI were evaluated using linear models.
Results: Analyses included 151 children (M = 10.9 years). Each FTO rs9939609 obesity risk allele was related to a higher food-cue-related response in the right lateral hypothalamus after controlling for covariates including the current BMI Z-score (p < 0.01), however, the association did not remain significant after applying the multiple testing correction. MC4R rs571312 and the PRS were not related to heightened food-cue-related reward responsivity in any examined regions. The whole-brain analysis did not identify additional regions of food-cue-related response related to the examined genetic factors.
Conclusion: Children genetically at risk for obesity, as indicated by the FTO genotype, may be predisposed to higher food-cue-related reward responsivity in the lateral hypothalamus in the sated state, which, in turn, could contribute to overconsumption.
Clinical trial registration:
Obesity currently affects approximately 20% of children and adolescents aged 2–19 years in the United States according to the National Health Statistics Report (1). Obesity often continues from childhood into adulthood (2), and obesity in adulthood is a known risk factor for the development of multiple comorbidities including type 2 diabetes, cardiovascular diseases, and cancer (3). The etiology of obesity is multifactorial, affected by genes, environment, and gene-environment interactions (4, 5).
Environmental food cues include the smell, taste, and sight of highly palatable food or food-related situations and play a crucial role in obesity development through physiological and psychological responses (6–9). They are often presented through media in the form of advertisements for highly palatable, nutrient-poor, and energy-dense foods and beverages (10). Marketing of highly palatable foods and beverages is often directed toward younger children, and these exposures promote the development of unhealthy food preferences and eating behavior patterns that may ultimately lead to childhood obesity (10–15).
Exposure to highly palatable food and beverage cues may activate a response in dopaminergic reward regions of the brain that are associated with increased food consumption (16–18) and weight gain (19–21). Previous functional magnetic resonance imaging (fMRI) studies have identified the brain regions involved in the corticolimbic reward circuitries related to food cues: nucleus accumbens, orbitofrontal cortex, amygdala, insula, lateral hypothalamus (LH), substantia nigra, and ventral tegmental area (22–28). Further, these regions have been related to food cravings and appetitive motivation (29–33).
Several genes, including the fat mass and obesity-associated gene (FTO) and melanocortin 4 receptor gene (MC4R), have been implicated in obesity risk. Research has shown that divergent central nervous system (CNS) mechanisms may drive overconsumption in those with FTO risk alleles. A rodent study has shown that FTO is present primarily in the hypothalamus, a region related to hunger/satiation control (34). A common genetic variant within the first intron of the FTO gene, rs9939609, is known to be associated with higher energy intake (35–38) and BMI (39–41) in children. A previous study from our group also found that FTO rs9939609 was associated with children’s food-cue-related neural reactivity in the left and right nucleus accumbens (42), a potential mediator of excess consumption and adiposity gain. Participants were only provided a light snack in that study to help control their hunger, so the association between FTO and food cue reactivity in the post-prandial state was not examined.
Melanocortin 4 receptors are also expressed in the hypothalamus as part of the leptin-melanocortin pathway and play a crucial role in regulating appetite, energy balance, and body weight (43–45). MC4R rs571312, a common near-MC4R variant, has been related to higher caloric intake (46) and greater BMI or obesity (47–49). Another study found a strong association between rs12970134 and obesogenic eating behaviors including greater food responsiveness and less satiety responsiveness in children (50). However, no studies to date have examined the effect of common MC4R polymorphisms on food-cue-related neural reward reactivity.
Though some individuals may have obesity due to a rare mutation in a single gene, most individuals with obesity have numerous polymorphisms that jointly affect their adiposity (51). A comprehensive genetic obesity risk can be summarized through an obesity polygenic risk score (PRS) that is constructed based on the effects of variants observed in genome-wide association studies (GWAS). Richardson et al. (52) created a 295 SNP PRS to predict adiposity in early life. Though previous studies in children have demonstrated an association between weight status and food-cue-related neural response to food cues (21, 53–55), the relationship between comprehensive genetic risk for obesity, characterized by a PRS, and food-cue-related neural responsivity has not yet been examined.
In this study, we aimed to test whether some children are genetically predisposed to heightened food-cue-related neural reward reactivity in the post-prandial period. We hypothesized that the genetic risk of obesity, defined by FTO rs9939609, MC4R rs571312, and a pediatric PRS, would relate to greater differential activation in brain reward regions in response to food advertisements. Additionally, we conducted a hypothesis-generating whole-brain exploratory analysis to identify additional regions that may be related to the associations between genetic exposures and post-prandial responsivity to food cues. This study builds upon previous work by examining a greater range of obesity-related factors and by examining how these genetic factors affect neural food cue reactivity when children are in the sated state.
The data in this paper are from a larger study measuring the genetic associations with children’s neural reward reactivity and eating in the absence of hunger in response to food cues. The study enrolled 189 pre-adolescent children from the Northern New England community. Seven participants were excluded after genotyping quality control, and 31 participants were excluded after MRI scanning quality control. Scan data from 31 participants were excluded due to: refusal to be scanned (n = 12); excessive movement in the scanner (n = 9); and technical issues (n = 10). The final analysis included 151 children (86 of whom were male) between the ages of 9 and 12 [mean (SD) = 10.9 (1.16) y]. Dartmouth College’s Committee for the Protection of Human Subjects approved all study protocols.
Participants attended a study visit alongside a parent or guardian. Visits were scheduled at either lunchtime (11:00 am–1:00 pm) or dinnertime (4:00 pm–6:00 pm). A trained research staff member collected children’s saliva samples for genetic analysis, measured height and weight, and administered questionnaires to the parents. The parent-reported child’s physical activity, date of birth, biological sex, race, ethnicity, annual household income, and parent education level. Participant height was measured to the nearest 0.1 cm using Seca 264 Stadiometer (Hamburg, Germany), and weight was measured to the nearest 0.01 kg using a Seca 703 Medical Scale (Hamburg, Germany). Children consumed a standardized pre-load meal consisting of macaroni and cheese, apple sauce, corn, milk, and water. Satiety level was measured prior to the scan using the Freddy Fullness scale (56), a validated visual analog scale for estimating fullness in children. The fullness scale was reported across a range of 0-150 mm and converted into percentages (out of 150 mm); higher scores indicated greater fullness.
DNA extracted from saliva samples was genotyped for >600,000 single nucleotide polymorphisms (SNPs) with the Illumina Global Screening Array 24 v1.0 or v3.0 (57). Pre-specified quality control thresholds were applied to generate genotype calls using GenomeStudio software (58) with downstream quality control steps and determination of European or non-European ancestry with principal components, as previously described (59, 60). Using the Michigan Imputation Server, haplotype-based imputation was performed with a quality score threshold of R2 > 0.8 selected for SNPs with high-quality imputation (61, 62). Seven children were excluded for failing genotype quality control.
As primary exposures of genetic risk, two single SNPs were considered (FTO rs9939609 and MC4R rs571312), and a pediatric-specific PRS with 265 of 295 SNPs available in the data (52), the “Pediatric PRS.” In additional exploratory analyses, we also analyzed three PRS previously associated with adult BMI. These included a 97 SNPs PRS (63), 557 SNP PRS (52), and a ~ 2 million SNP PRS (64), henceforth referred to as the “Adult 97 PRS,” “Adult 557 PRS,” and “Adult 2M PRS,” respectively. Each PRS was computed as the product of the dosage of each risk allele (0, 1, or 2) and the published effect size, summed and standardized into Z-scores.
Using E-Prime (65), children were presented with a series of videos that were designed to replicate a typical television show. The stimuli included three 5 min segments of a popular science show (MythBusters) interspersed with four 5 min commercial breaks.
Four functional runs were conducted in each scan. Additional functional runs were collected as part of the larger study after the experimental paradigm of this study, but are not relevant to this presented analysis. Each functional run began and ended with a 15 s presentation of a fixation cross. For each run, 5 food and 5 non-food TV commercials were presented which alternated in an AB pattern (66, 67). The block pattern for each run was randomized between participants (AB or BA) and which commercials were played during each block were also randomized between participants. Each commercial ran for approximately 15 s, and each functional run was approximately 5 min in length. The total duration of the scan was approximately 1 h.
Age-appropriate food and non-food commercials that were included in this study were rated by children for interest and excitement (42). There was no overall difference in interest and excitement between the food and non-food commercials.
Scans were conducted using a 3.0 T Siemens MAGNETOM Prisma MRI scanner equipped with a 32-channel head coil. For T1-weighted structural scans (MPRAGE), the following parameters were employed: echo time (TE) of 2.32 ms, repetition time (TR) of 2,300 ms, flip angle of 8 degrees, matrix size of 256 × 256 mm, field of view of 240 × 240 mm, 192 slices with a slice thickness of 0.9 mm, and voxel size of 0.9 × 0.9 × 0.9 mm. Functional imaging utilized a T2*-weighted echo planar imaging (EPI) sequence with TE = 33 ms, TR = 1,250 ms, flip angle = 64 degrees, matrix size = 96 × 96, field of view = 240 × 240 mm, 56 slices with a slice thickness = 2.5 mm, and voxel dimensions of 2.5 × 2.5 × 2.5 mm. Four functional runs of 144 volumes were included in the analysis for each participant.
BMI was calculated based on participants’ height and weight using the U.S. Center for Disease Control and Prevention (CDC) 2020 age-and sex-specific distributions (68). A missing value for physical activity (N = 1) was imputed with the most frequently reported value. A missing value for the fullness measure (N = 1) was imputed with the median value. One fullness value of 180 mm was imputed with the median due to researcher measurement error.
Anatomical data preprocessing and functional data preprocessing were performed using fMRIPrep 1.2.5 (69, 70), which is based on Nipype 1.1.6 (71, 72). The pipeline and protocol used are described in detail as a template provided by fMRIPrep in a previously published article (73).
Following pre-processing, participants’ individual fMRI data were analyzed using the NLTools Python package (74). A general linear model (GLM) was conducted for subject-level analysis for each participant. This included constructing a design matrix, convolving with the hemodynamic response function (HRF), incorporating nuisance variables such as intercepts, linear and quadratic trends, motion covariates (comprising 24 parameters: six demeaned realignment parameters, their squares, derivatives, and squared derivatives), and identifying motion spikes (defined as spikes between successive TRs and global spikes exceeding an intensity change threshold of 2.5 standard deviations from the mean). The data underwent spatial smoothing using a Gaussian kernel with a full-width at half maximum (FWHM) of 6 mm. We conducted a standard visual inspection of scans with a frame-wise displacement of 1 or greater, and one of four functional runs was excluded for 17 participants (~11%) due to such visual inspections (73). Additionally, we examined functional runs for extreme head motion defined as >25% motion spikes (> 36 spikes) of the scan volumes; however, no functional run was excluded from further analyses. To generate the food-specific regression coefficient maps for individuals, the coefficients in each voxel were averaged across functional runs for food and non-food ad conditions, separately, and then the within-subject difference between the two conditions was computed to create contrast maps. All individual-level contrast maps were used in the targeted region of interest (ROI) and whole brain analyses. Multiple comparison correction using the false discovery rate (FDR) was applied to the p-values across 7 bilateral ROIs with significance set at q < 0.05.
For the a priori ROI analysis, seven bilateral ROIs were selected as candidate reward regions based on previous literature (25): the nucleus accumbens (NAcc), orbitofrontal cortex (OFC), amygdala, insula, lateral hypothalamus (LH), ventral tegmental area (VTA), and substantia nigra (SN). The masks of the bilateral NAcc, OFC, amygdala, and insula were extracted for each participant using FreeSurfer’s autosegmentation.1 The final group-level ROI masks were created by including those voxels that are counted in the individual-level masks for at least 75% of participants. As FreeSurfer does not include autosegmentation of the lateral hypothalamus and SN, masks of these regions were generated using the anatomical atlas of the human hypothalamic regions (75). The mask of the ventral tegmental area was defined by the sphere with a radius of 5 mm centered at the MNI coordinate [±4, −16, −10] (76). The ROI masks are shown in Figure 1. Beta values were then averaged across each mask and analyzed using R (77). We investigated the Pearson correlation between corresponding lateral reward ROIs as well as across reward ROIs.
Figure 1. Axial view of masks used in the regions-of-interest (ROI) analysis. (A) Orbitofrontal cortex. (B) Blue: nucleus accumbens. Light green: lateral hypothalamus. Red: amygdala. Dark green: substantia nigra. Orange: ventral tegmental area. (C) Insula.
Child age, biological sex, BMI Z-score, satiety level post preload-meal, and European ancestry were selected as covariates a priori for all adjusted models given potential relationships with genetic exposures and/or neural response to stimuli, for all adjusted models. After examining the bivariate relationships between the other potential covariates and the neural response in any ROIs using a threshold of p < 0.1, physical activity and annual household income were added into adjusted models. Linear models were used to test the independent associations between the genetic factors (MC4R rs571312, FTO rs9939609, Pediatric PRS) and the neural response in the bilateral ROIs. In an exploratory analysis, the linear models were repeated with the secondary genetic exposures of 3 adult obesity PRS. Given that the pediatric and adult obesity PRS measures were trained on individuals of European ancestry, the distribution of each genotype and PRS in participants with European and non-European ancestry was explored in Supplementary Table S1, and the regression models of the four PRS measures were repeated restricting the sample to participants with European ancestry in a sensitivity analysis.
A whole-brain analysis was conducted using the individual beta maps as input to a group-level analysis to test the unadjusted and adjusted linear relationships between food-cue-related response and genotypes. To determine significance at the group level, an initial voxel-wise significance threshold of p-value <0.001 was applied and was then cluster-corrected using a threshold of a cluster size of k = 90 for an overall p-value <0.05. Clustering parameters were based on 10,000 Monte-Carlo simulations determined using 3dClustSim from AFNI.
Seven participants were excluded after genotype quality control, and 31 participants were excluded after MRI quality control, leaving 151 participants in the analysis (Table 1). Most participants were white (94.7%) and non-Hispanic (93.4%). The average (SD) BMI Z-score was 0.468 (0.95), and 30% of participants were categorized as either having overweight or obesity. The average (SD) caloric consumption of the standardized preload meal was 449 (171 kcal). Examining the distribution of MC4R rs571312 in our sample, 4.6% were highest-risk (AA) children (N = 7), 41.1% were heterozygotes (AC) children (N = 62), and 54.3% were homozygous low-risk (CC) participants (N = 82). Due to the limited number of participants in the highest-risk group, the MC4R AA and AC genotypes were collapsed into one category and analyzed with a dominant model (AA and AC vs. CC). In our sample, the distribution of FTO rs9939609 was 10.6% with the highest obesity risk (AA) (N = 16), 48.3% with moderate risk (AT) children (N = 73), and 41.1% with the lowest risk (TT) children (N = 62). The additive model of the FTO genotype (AA vs. AT vs. TT) was used in further analyses.
The correlations between the responses of food > non-food contrast maps in 14 bilateral reward regions are shown in Figure 2. In general, all bilateral reward regions tested were positively and significantly correlated ranging from 0.93 for the OFC and 0.62 for the LH (Supplementary Figure S1). The right LH exhibited correlations with three other ROIs, and the bilateral VTA exhibited positive correlations with five ROIs. The ROI analysis examining the associations between each genetic exposure and neural response to food cues in the full cohort is presented in Table 2. Children with the FTO rs9939609 risk allele had a significantly higher food-related neural response in the right LH in models adjusted for covariates even after controlling for current adiposity (t = 2.6, p = 0.01) (Figure 3). However, the association did not remain significant after applying the FDR correction. The association between FTO rs9939609 risk alleles and food-related response in the left LH did not reach statistical significance (t = 1.7, p = 0.08). The MC4R genotype and Pediatric PRS were not significantly associated with the food-related reward reactivity in any of the explored ROIs (Table 2).
Figure 2. Pearson correlations between the reward regions are shown. Only correlations statistically significant at the p < 0.05 are numbered. NAcc, nucleus accumbens; OFC, orbitofrontal cortex; VTA, ventral tegmental area; SN, substantia nigra; LH, lateral hypothalamus.
Table 2. Associations between genetic exposures and food-related response in the region-of-interest (ROI) after eating a meal to satiety (N = 151).
Figure 3. Adjusted association between FTO rs9939609 and neural response in the right lateral hypothalamus after eating a meal to satiety (N = 151). Adjusted linear regression model was conducted and adjusted for age, biological sex, BMI Z-score, satiety level post preload-meal, European ancestry, physical activity, and annual household income. The sample size of FTO rs9939609 for lowest-risk group (TT) is 62, heterozygotes (AT) is 73, and highest-risk group (AA) is 16. FTO rs9939609 was added as an additive model (TT vs. AT vs. AA).
In the exploratory analysis that tested three additional adult obesity PRS associations, the 97 PRS with food cue-related activity in the left insula did not reach statistical significance (t = 1.9, p = 0.06) (Supplementary Table S2). In the sensitivity analyses of the PRS measures restricting the sample to participants with European ancestry (N = 136), the adult 97 and 557 PRS with food-related response in the LH did not reach statistical significance (t = 1.8, p = 0.08; t = 1.7, p = 0.08, respectively) (Supplementary Table S3).
No additional relationships between genetic factors and food-related responsivity in the whole brain analysis.
In this study of 151 children aged 9–12 years old, we found that the genetic risk of obesity was associated with greater brain activation in response to food advertisements in the lateral hypothalamus after eating a meal to satiety. Specifically, each risk allele of the FTO rs9939609 genotype was associated with heightened food-related responsivity in the right lateral hypothalamus.
In the brain, FTO is highly expressed in the hypothalamus, a region involved in the regulation of central energy homeostasis to control body energy balance, energy expenditure, and food intake (78). Many studies have reported the connection between FTO SNPs and obesity-related traits such as BMI, body fat mass, waist circumference, hip circumference, and energy intake (79–81). In addition, FTO risk alleles have been related to fat or carbohydrate intake, reduced satiety, overeating, and loss of control over eating (39, 82, 83). The lateral hypothalamus, as part of the hypothalamus, receives a high level of melanocortinergic inputs from the arcuate nucleus of the hypothalamus. Animal studies have identified that the LH is specifically involved in food-seeking behaviors, reward behaviors, and autonomic function (84–86). Our study finding that FTO is related to greater food-related reward activity in the lateral hypothalamus when children are in a sated state highlights a potential biological mechanism that may mediate the association between genetic obesity risk and excess weight gain. As the understanding of the LH’s precise functions and mechanisms remains limited, further research is needed to understand if the higher food-related reward activity corresponds to cued non-homeostatic caloric intake in the post-prandial period.
In our previous study with an independent study cohort of the same age group, we observed a heightened reward response in the bilateral nucleus accumbens in response to food vs. non-food TV commercials among children with at least one FTO risk allele compared to those with no risk alleles (42). In that study, children were provided a light snack prior to imaging, rather than a full meal eaten to satiety. Together, these studies suggest that the FTO genotype is related to the heightened reward response to food cues in different brain regions at different states of satiety.
In our current study, we did not find significant neural reward reactivity to food cues associated with the MC4R genotype nor four PRS measures. Given the composite nature of PRS, they may have limited utility in clarifying the biological mechanisms underlying the genetic-reward activity relationship.
This study provides evidence that the FTO genotype is related to neural reward reactivity to food cues in children in the post-prandial period. Nevertheless, it is important to acknowledge the limitations of our study. Given the distinct role of the lateral hypothalamus in food-seeking and reward behaviors within the hypothalamus, we aimed to study this specific region. While we used a mask, future research could use manual segmentation of the lateral hypothalamus to potentially provide more accurate segmentation. Our sample predominantly consisted of individuals of a white, non-Hispanic population and relatively higher socioeconomic status. Additionally, the additive effect of the MC4R genotype was not explored due to the limited number the high-risk (AA) individuals. Due to limitations in sample size, the analysis did not extend to additional candidate genes associated with obesity. Future research should aim to explore the genetic effects on heightened neural reward responsivity in a larger and more diverse population, allowing for broader generalization of the findings.
Our findings indicate that some children possess a genetic predisposition towards heightened food-cue-related neural reward reactivity in the post-prandial period. Given the prevalence of extensive media exposure among children which often includes the promotion of a variety of unhealthy food products, it is crucial to understand the genetic influences on food-related neural responses in children and mitigate exposure that may contribute to excess consumption. Longitudinal studies are needed to understand whether this heightened reward response to food cues leads to greater cued consumption and, ultimately, to excess weight gain.
The original contributions presented in the study are publicly available. This data can be found at: https://www.ncbi.nlm.nih.gov/gap/, phs003550.v1.
The studies involving humans were approved by Institutional Review Board at Dartmouth College. The studies were conducted in accordance with the local legislation and institutional requirements. Written informed consent for participation in this study was provided by the participants’ legal guardians/next of kin.
DY: Formal analysis, Writing – original draft, Writing – review & editing. TR: Writing – review & editing. DC: Data curation, Project administration, Writing – review & editing. GB: Data curation, Project administration, Writing – review & editing. RLa: Data curation, Project administration, Writing – review & editing. MM: Writing – review & editing, Validation. RLo: Conceptualization, Writing – review & editing. JE: Conceptualization, Formal analysis, Investigation, Validation, Writing – review & editing. TM: Conceptualization, Investigation, Methodology, Supervision, Writing – review & editing. DG-D: Conceptualization, Funding acquisition, Investigation, Methodology, Project administration, Supervision, Writing – review & editing.
The author(s) declare that financial support was received for the research, authorship, and/or publication of this article. This study was supported by the National Institutes of Health (5R01HD092604; NCT03766191), Dartmouth Cancer Center Genomics Shared Resource Grants (5P30CA023108; P20GM130454), and National Cancer Institute (T32CA260262).
The authors declare that the research was conducted in the absence of any commercial or financial relationships that could be construed as a potential conflict of interest.
All claims expressed in this article are solely those of the authors and do not necessarily represent those of their affiliated organizations, or those of the publisher, the editors and the reviewers. Any product that may be evaluated in this article, or claim that may be made by its manufacturer, is not guaranteed or endorsed by the publisher.
The Supplementary material for this article can be found online at: https://www.frontiersin.org/articles/10.3389/fnut.2024.1387514/full#supplementary-material
BMI, Body mass index; PRS, Polygenic risk score; SNP, Single nucleotide polymorphism; EER, Estimated daily energy requirement.
1. Bryan, S, Afful, J, Carroll, MD, Chen, T-C, Davy, O, Fink, S, et al. NHSR 158. National Health and Nutrition Examination Survey 2017—March 2020 pre-pandemic data files National Center for Health Statistics (U.S.) (2021). Available at: https://stacks.cdc.gov/view/cdc/106273
2. Singh, AS, Mulder, C, Twisk, JWR, van Mechelen, W, and Chinapaw, MJM. Tracking of childhood overweight into adulthood: a systematic review of the literature. Obes Rev. (2008) 9:474–88. doi: 10.1111/j.1467-789X.2008.00475.x
3. Maury, E, and Brichard, SM. Adipokine dysregulation, adipose tissue inflammation and metabolic syndrome. Mol Cell Endocrinol. (2010) 314:1–16. doi: 10.1016/j.mce.2009.07.031
4. Hill, JO, Wyatt, HR, and Melanson, EL. Genetic and environmental contributions to obesity. Med Clin North Am. (2000) 84:333–46. doi: 10.1016/s0025-7125(05)70224-8
5. Bray, MS . Implications of gene-behavior interactions: prevention and intervention for obesity. Obesity. (2008) 16:S72–8. doi: 10.1038/oby.2008.522
6. Jansen, A . A learning model of binge eating: cue reactivity and cue exposure. Behav Res Ther. (1998) 36:257–72. doi: 10.1016/s0005-7967(98)00055-2
7. Prinsen, S, de Ridder, DTD, and de Vet, E. Eating by example. Effects of environmental cues on dietary decisions. Appetite. (2013) 70:1–5. doi: 10.1016/j.appet.2013.05.023
8. van den Akker, K, Stewart, K, Antoniou, EE, Palmberg, A, and Jansen, A. Food cue reactivity, obesity, and impulsivity: are they associated? Curr Addict Rep. (2014) 1:301–8. doi: 10.1007/s40429-014-0038-3
9. Hayashi, D, Edwards, C, Emond, JA, Gilbert-Diamond, D, Butt, M, Rigby, A, et al. What is food noise? A conceptual model of food cue reactivity. Nutrients. (2023) 15:4809. doi: 10.3390/nu15224809
10. Harris, JL, Pomeranz, JL, Lobstein, T, and Brownell, KD. A crisis in the marketplace: how food marketing contributes to childhood obesity and what can be done. Annu Rev Public Health. (2009) 30:211–25. doi: 10.1146/annurev.publhealth.031308.100304
11. Ustjanauskas, AE, Harris, JL, and Schwartz, MB. Food and beverage advertising on children’s web sites. Pediatr Obes. (2013) 9:362–72. doi: 10.1111/j.2047-6310.2013.00185.x
12. Harris, JL, and Kalnova, SS. Food and beverage TV advertising to young children: measuring exposure and potential impact. Appetite. (2018) 123:49–55. doi: 10.1016/j.appet.2017.11.110
13. Coates, AE, Hardman, CA, Halford, JCG, Christiansen, P, and Boyland, EJ. Social media influencer marketing and children’s food intake: a randomized trial. Pediatrics. (2019) 143:e20182554. doi: 10.1542/peds.2018-2554
14. Bragg, M, Lutfeali, S, Greene, T, Osterman, J, and Dalton, M. How food marketing on Instagram shapes adolescents’ food preferences: online randomized trial. J Med Internet Res. (2021) 23:e28689. doi: 10.2196/28689
15. Packer, J, Russell, SJ, Siovolgyi, G, McLaren, K, Stansfield, C, Viner, RM, et al. The impact on dietary outcomes of celebrities and influencers in marketing unhealthy foods to children: a systematic review and meta-analysis. Nutrients. (2022) 14:434. doi: 10.3390/nu14030434
16. Lawrence, NS, Hinton, EC, Parkinson, JA, and Lawrence, AD. Nucleus accumbens response to food cues predicts subsequent snack consumption in women and increased body mass index in those with reduced self-control. NeuroImage. (2012) 63:415–22. doi: 10.1016/j.neuroimage.2012.06.070
17. Masterson, TD, Stein, WM, Beidler, E, Bermudez, M, English, LK, and Keller, KL. Brain response to food brands correlates with increased intake from branded meals in children: an fMRI study. Brain Imaging Behav. (2019) 13:1035–48. doi: 10.1007/s11682-018-9919-8
18. Gearhardt, AN, Yokum, S, Harris, JL, Epstein, LH, and Lumeng, JC. Neural response to fast food commercials in adolescents predicts intake. Am J Clin Nutr. (2020) 111:493–502. doi: 10.1093/ajcn/nqz305
19. Stice, E, Yokum, S, Bohon, C, Marti, N, and Smolen, A. Reward circuitry responsivity to food predicts future increases in body mass: moderating effects of DRD2 and DRD4. NeuroImage. (2010) 50:1618–25. doi: 10.1016/j.neuroimage.2010.01.081
20. Demos, KE, Heatherton, TF, and Kelley, WM. Individual differences in nucleus accumbens activity to food and sexual images predict weight gain and sexual behavior. J Neurosci. (2012) 32:5549–52. doi: 10.1523/jneurosci.5958-11.2012
21. Gearhardt, AN, Yokum, S, Stice, E, Harris, JL, and Brownell, KD. Relation of obesity to neural activation in response to food commercials. Soc Cogn Affect Neurosci. (2014) 9:932–8. doi: 10.1093/scan/nst059
22. Berridge, KC . ‘Liking’ and ‘wanting’ food rewards: brain substrates and roles in eating disorders. Physiol Behav. (2009) 97:537–50. doi: 10.1016/j.physbeh.2009.02.044
23. García-García, I, Narberhaus, A, Marqués-Iturria, I, Garolera, M, Rădoi, A, Segura, B, et al. Neural responses to visual food cues: insights from functional magnetic resonance imaging: brain and sight of food. Eur Eat Disorders Rev. (2013) 21:89–98. doi: 10.1002/erv.2216
24. Kelley, A, Baldo, B, Pratt, W, and Will, M. Corticostriatal-hypothalamic circuitry and food motivation: integration of energy, action and reward. Physiol Behav. (2005) 86:773–95. doi: 10.1016/j.physbeh.2005.08.066
25. Kenny, PJ . Reward mechanisms in obesity: new insights and future directions. Neuron. (2011) 69:664–79. doi: 10.1016/j.neuron.2011.02.016
26. Killgore, WDS, Young, AD, Femia, LA, Bogorodzki, P, Rogowska, J, and Yurgelun-Todd, DA. Cortical and limbic activation during viewing of high-versus low-calorie foods. NeuroImage. (2003) 19:1381–94. doi: 10.1016/S1053-8119(03)00191-5
27. Sheng, Z, Santiago, AM, Thomas, MP, and Routh, VH. Metabolic regulation of lateral hypothalamic glucose-inhibited orexin neurons may influence midbrain reward neurocircuitry. Mol Cell Neurosci. (2014) 62:30–41. doi: 10.1016/j.mcn.2014.08.001
28. van der Laan, LN, de Ridder, DTD, Viergever, MA, and Smeets, PAM. The first taste is always with the eyes: a meta-analysis on the neural correlates of processing visual food cues. NeuroImage. (2011) 55:296–303. doi: 10.1016/j.neuroimage.2010.11.055
29. Pelchat, ML, Johnson, A, Chan, R, Valdez, J, and Ragland, JD. Images of desire: food-craving activation during fMRI. NeuroImage. (2004) 23:1486–93. doi: 10.1016/j.neuroimage.2004.08.023
30. Siep, N, Roefs, A, Roebroeck, A, Havermans, R, Bonte, M, and Jansen, A. Fighting food temptations: the modulating effects of short-term cognitive reappraisal, suppression and up-regulation on mesocorticolimbic activity related to appetitive motivation. NeuroImage. (2012) 60:213–20. doi: 10.1016/j.neuroimage.2011.12.067
31. Dietrich, A, Hollmann, M, Mathar, D, Villringer, A, and Horstmann, A. Brain regulation of food craving: relationships with weight status and eating behavior. Int J Obes. (2016) 40:982–9. doi: 10.1038/ijo.2016.28
32. Kahathuduwa, CN, Davis, T, O’Boyle, M, and Binks, M. Do scores on the food craving inventory and three-factor eating questionnaire correlate with expected brain regions of interest in people with obesity? Physiol Behav. (2018) 188:1–10. doi: 10.1016/j.physbeh.2018.01.018
33. Contreras-Rodríguez, O, Cano, M, Vilar-López, R, Rio-Valle, JS, Verdejo-Román, J, Navas, JF, et al. Visceral adiposity and insular networks: associations with food craving. Int J Obes. (2019) 43:503–11. doi: 10.1038/s41366-018-0173-3
34. Olszewski, PK, Fredriksson, R, Olszewska, AM, Stephansson, O, Alsiö, J, Radomska, KJ, et al. Hypothalamic FTO is associated with the regulation of energy intake not feeding reward. BMC Neurosci. (2009) 10:129. doi: 10.1186/1471-2202-10-129
35. Timpson, NJ, Emmett, PM, Frayling, TM, Rogers, I, Hattersley, AT, McCarthy, MI, et al. The fat mass- and obesity-associated locus and dietary intake in children. Am J Clin Nutr. (2008) 88:971–8. doi: 10.1093/ajcn/88.4.971
36. Wardle, J, Llewellyn, C, Sanderson, S, and Plomin, R. The FTO gene and measured food intake in children. Int J Obes. (2008) 33:42–5. doi: 10.1038/ijo.2008.174
37. Gilbert-Diamond, D, Emond, JA, Lansigan, RK, Rapuano, KM, Kelley, WM, Heatherton, TF, et al. Television food advertisement exposure and FTO rs9939609 genotype in relation to excess consumption in children. Int J Obes. (2016) 41:23–9. doi: 10.1038/ijo.2016.163
38. Ranzenhofer, LM, Mayer, LES, Davis, HA, Mielke-Maday, HK, McInerney, H, Korn, R, et al. The FTO gene and measured food intake in 5-to 10-year-old children without obesity. Obesity. (2019) 27:1023–9. doi: 10.1002/oby.22464
39. Cecil, JE, Tavendale, R, Watt, P, Hetherington, MM, and Palmer, CNA. An obesity-associated FTO gene variant and increased energy intake in children. N Engl J Med. (2008) 359:2558–66. doi: 10.1056/nejmoa0803839
40. Liu, G, Zhu, H, Lagou, V, Gutin, B, Stallmann-Jorgensen, IS, Treiber, FA, et al. FTO variant rs9939609 is associated with body mass index and waist circumference, but not with energy intake or physical activity in European-and African-American youth. BMC Med Genet. (2010) 11:57. doi: 10.1186/1471-2350-11-57
41. Lourenço, BH, Qi, L, Willett, WC, and Cardoso, MAACTION Study Team. FTO genotype, vitamin D status, and weight gain during childhood. Diabetes. (2014) 63:808–14. doi: 10.2337/db13-1290
42. Rapuano, KM, Zieselman, AL, Kelley, WM, Sargent, JD, Heatherton, TF, and Gilbert-Diamond, D. Genetic risk for obesity predicts nucleus accumbens size and responsivity to real-world food cues. Proc Natl Acad Sci USA. (2017) 114:160–5. doi: 10.1073/pnas.1605548113
43. Balthasar, N, Dalgaard, LT, Lee, CE, Yu, J, Funahashi, H, Williams, T, et al. Divergence of melanocortin pathways in the control of food intake and energy expenditure. Cell. (2005) 123:493–505. doi: 10.1016/j.cell.2005.08.035
44. Tao, YX . Molecular mechanisms of the neural melanocortin receptor dysfunction in severe early onset obesity. Mol Cell Endocrinol. (2005) 239:1–14. doi: 10.1016/j.mce.2005.04.012
45. Garfield, AS, Li, C, Madara, JC, Shah, BP, Webber, E, Steger, JS, et al. A neural basis for melanocortin-4 receptor-regulated appetite. Nat Neurosci. (2015) 18:863–71. doi: 10.1038/nn.4011
46. McCaffery, JM, Jablonski, KA, Franks, PW, Delahanty, LM, Aroda, V, Marrero, D, et al. Replication of the association of BDNF and MC4R variants with dietary intake in the diabetes prevention program. Psychosom Med. (2017) 79:224–33. doi: 10.1097/PSY.0000000000000380
47. Grant, SFA, Bradfield, JP, Zhang, H, Wang, K, Kim, CE, Annaiah, K, et al. Investigation of the locus near MC4R with childhood obesity in Americans of European and African ancestry. Obesity. (2009) 17:1461–5. doi: 10.1038/oby.2009.53
48. Apalasamy, YD, Ming, MF, Rampal, S, Bulgiba, A, and Mohamed, Z. Association of melanocortin-4 receptor gene polymorphisms with obesity-related parameters in Malaysian Malays. Ann Hum Biol. (2013) 40:102–6. doi: 10.3109/03014460.2012.720709
49. Kvaløy, K, Kulle, B, Romundstad, P, and Holmen, TL. Sex-specific effects of weight-affecting gene variants in a life course perspective—the HUNT study, Norway. Int J Obes. (2013) 37:1221–9. doi: 10.1038/ijo.2012.220
50. Wang, S, Song, J, Yang, Y, Chawla, NV, Ma, J, and Wang, H. Rs12970134 near MC4R is associated with appetite and beverage intake in overweight and obese children: a family-based association study in Chinese population. PLoS One. (2017) 12:e0177983. doi: 10.1371/journal.pone.0177983
51. Joffe, YT, and Houghton, CA. A novel approach to the nutrigenetics and nutrigenomics of obesity and weight management. Curr Oncol Rep. (2016) 18:43. doi: 10.1007/s11912-016-0529-6
52. Richardson, TG, Sanderson, E, Elsworth, B, Tilling, K, and Davey, SG. Use of genetic variation to separate the effects of early and later life adiposity on disease risk: Mendelian randomisation study. BMJ. (2020):m1203. doi: 10.1136/bmj.m1203
53. Bruce, AS, Lepping, RJ, Bruce, JM, Cherry, JBC, Martin, LE, Davis, AM, et al. Brain responses to food logos in obese and healthy weight children. J Pediatr. (2013) 162:759–764.e2. doi: 10.1016/j.jpeds.2012.10.003
54. Masterson, TD, Bermudez, MA, Austen, M, Lundquist, E, Pearce, AL, Bruce, AS, et al. Food commercials do not affect energy intake in a laboratory meal but do alter brain responses to visual food cues in children. Appetite. (2019) 132:154–65. doi: 10.1016/j.appet.2018.10.010
55. Richter, M, Widera, S, Malz, F, Goltermann, J, Steinmann, L, Kraus, A, et al. Higher body weight-dependent neural activation during reward processing. Brain Imaging Behav. (2023) 17:414–24. doi: 10.1007/s11682-023-00769-3
56. Keller, KL, Assur, SA, Torres, M, Lofink, HE, Thornton, JC, Faith, MS, et al. Potential of an analog scaling device for measuring fullness in children: development and preliminary testing. Appetite. (2006) 47:233–43. doi: 10.1016/j.appet.2006.04.004
57. Illumina . (2020). Infinium™ global screening array-24 v3.0 BeadChip. Available at: https://www.illumina.com/content/dam/illumina-marketing/documents/products/datasheets/infinium-global-screening-array-data-sheet-370-2016-016.pdf (Accessed February 01, 2023).
58. Illumina . (2022). GenomeStudio software. Available at: https://www.illumina.com/techniques/microarrays/array-data-analysis-experimental-design/genomestudio.html (Accessed February 1, 2023).
59. Renier, TJ, Yeum, D, Emond, JA, Lansigan, RK, Ballarino, GA, Carlson, DD, et al. Elucidating pathways to pediatric obesity: a study evaluating obesity polygenic risk scores related to appetitive traits in children. Int J Obes. (2023) 48:71–7. doi: 10.1038/s41366-023-01385-3
60. Meyer, H . meyer-lab-cshl/plinkQC: plinkQC 0.3.2 (v0.3.2). Zenodo. (2020). doi: 10.5281/ZENODO.3934294
61. Michigan Imputation Server . Getting started. (2021). Available at: https://imputationserver.readthedocs.io/en/latest/getting-started/#quality-control (Accessed February 1, 2023).
62. Das, S, Forer, L, Schönherr, S, Sidore, C, Locke, AE, Kwong, A, et al. Next-generation genotype imputation service and methods. Nat Genet. (2016) 48:1284–7. doi: 10.1038/ng.3656
63. Locke, AE, Kahali, B, Berndt, SI, Justice, AE, Pers, TH, Day, FR, et al. Genetic studies of body mass index yield new insights for obesity biology. Nature. (2015) 518:197–206. doi: 10.1038/nature14177
64. Khera, AV, Chaffin, M, Wade, KH, Zahid, S, Brancale, J, Xia, R, et al. Polygenic prediction of weight and obesity trajectories from birth to adulthood. Cell. (2019) 177:587–596.e9. doi: 10.1016/j.cell.2019.03.028
65. E-Prime® . (2023). Psychology software tools, solutions for research, assessment, and education. Available at: https://pstnet.com/products/e-prime/ (Accessed December 19, 2023).
66. Maus, B, van Breukelen, GJP, Goebel, R, and Berger, MPF. Optimization of blocked designs in fMRI studies. Psychometrika. (2010) 75:373–90. doi: 10.1007/s11336-010-9159-3
67. Smith, S, Jenkinson, M, Beckmann, C, Miller, K, and Woolrich, M. Meaningful design and contrast estimability in FMRI. NeuroImage. (2007) 34:127–36. doi: 10.1016/j.neuroimage.2006.09.019
68. Wei, R, Ogden, CL, Parsons, VL, Freedman, DS, and Hales, CM. A method for calculating BMI z-scores and percentiles above the 95th percentile of the CDC growth charts. Ann Hum Biol. (2020) 47:514–21. doi: 10.1080/03014460.2020.1808065
69. Esteban, O, Blair, R, Markiewicz, CJ, Berleant, SL, Moodie, C, Ma, F, et al. poldracklab/fmriprep: 1.0.0-rc5. Zenodo. (2017). doi: 10.5281/zenodo.996169
70. Esteban, O, Markiewicz, CJ, Blair, RW, Moodie, CA, Isik, AI, Erramuzpe, A, et al. fMRIPrep: a robust preprocessing pipeline for functional MRI. Nat Methods. (2019) 16:111–6. doi: 10.1038/s41592-018-0235-4
71. Gorgolewski, K, Burns, CD, Madison, C, Clark, D, Halchenko, YO, Waskom, ML, et al. Nipype: a flexible, lightweight and extensible neuroimaging data processing framework in python. Front Neuroinform. (2011) 5:13. doi: 10.3389/fninf.2011.00013
72. Esteban, O, Markiewicz, CJ, Burns, C, Goncalves, M, Jarecka, D, Ziegler, E, et al. nipy/nipype: 1.7.1. Zenodo. (2022). doi: 10.5281/ZENODO.596855
73. Yeum, D, Jimenez, CA, Emond, JA, Meyer, ML, Lansigan, RK, Carlson, DD, et al. Differential neural reward reactivity in response to food advertising medium in children. Front Neurosci. (2023) 17:1052384. doi: 10.3389/fnins.2023.1052384
74. Chang, L, Jolly, E, Cheong, JH, Burnashev, A, and Chen, A. cosanlab/nltools: 0.3.11. Genève: Zenodo. (2018) 10.
75. Neudorfer, C, Germann, J, Elias, GJB, Gramer, R, Boutet, A, and Lozano, AM. A high-resolution in vivo magnetic resonance imaging atlas of the human hypothalamic region. Sci Data. (2020) 7:305. doi: 10.1038/s41597-020-00644-6
76. Carter, RM . Activation in the VTA and nucleus accumbens increases in anticipation of both gains and losses. Front Behav Neurosci. (2009) 3:2009. doi: 10.3389/neuro.08.021.2009
77. R Core Team . R: a language and environment for statistical computing R Foundation for Statistical Computing (2020) Available at: https://www.R-project.org/.
78. Gerken, T, Girard, CA, Tung, YCL, Webby, CJ, Saudek, V, Hewitson, KS, et al. The obesity-associated FTO gene encodes a 2-oxoglutarate-dependent nucleic acid demethylase. Science. (2007) 318:1469–72. doi: 10.1126/science.1151710
79. Dina, C, Meyre, D, Gallina, S, Durand, E, Körner, A, Jacobson, P, et al. Variation in FTO contributes to childhood obesity and severe adult obesity. Nat Genet. (2007) 39:724–6. doi: 10.1038/ng2048
80. Frayling, TM, Timpson, NJ, Weedon, MN, Zeggini, E, Freathy, RM, Lindgren, CM, et al. A common variant in the FTO gene is associated with body mass index and predisposes to childhood and adult obesity. Science. (2007) 316:889–94. doi: 10.1126/science.1141634
81. Scuteri, A, Sanna, S, Chen, WM, Uda, M, Albai, G, Strait, J, et al. Genome-wide association scan shows genetic variants in the FTO gene are associated with obesity-related traits. PLoS Genet. (2007) 3:e115. doi: 10.1371/journal.pgen.0030115
82. Sonestedt, E, Roos, C, Gullberg, B, Ericson, U, Wirfält, E, and Orho-Melander, M. Fat and carbohydrate intake modify the association between genetic variation in the FTO genotype and obesity. Am J Clin Nutr. (2009) 90:1418–25. doi: 10.3945/ajcn.2009.27958
83. Tanofsky-Kraff, M, Han, JC, Anandalingam, K, Shomaker, LB, Columbo, KM, Wolkoff, LE, et al. The FTO gene rs9939609 obesity-risk allele and loss of control over eating. Am J Clin Nutr. (2009) 90:1483–8. doi: 10.3945/ajcn.2009.28439
84. Cui, H, Sohn, JW, Gautron, L, Funahashi, H, Williams, KW, Elmquist, JK, et al. Neuroanatomy of melanocortin-4 receptor pathway in the lateral hypothalamic area. J Comp Neurol. (2012) 520:4168–83. doi: 10.1002/cne.23145
85. Jennings, JH, Ung, RL, Resendez, SL, Stamatakis, AM, Taylor, JG, Huang, J, et al. Visualizing hypothalamic network dynamics for appetitive and consummatory behaviors. Cell. (2015) 160:516–27. doi: 10.1016/j.cell.2014.12.026
Keywords: food cues, fMRI, polygenic risk score, children, genotype, reward
Citation: Yeum D, Renier TJ, Carlson DD, Ballarino GA, Lansigan RK, Meyer ML, Loos RJF, Emond JA, Masterson TD and Gilbert-Diamond D (2024) Genetic associations with neural reward responsivity to food cues in children. Front. Nutr. 11:1387514. doi: 10.3389/fnut.2024.1387514
Received: 19 February 2024; Accepted: 10 September 2024;
Published: 25 September 2024.
Edited by:
Robert Fred Clark, St. Jude Children’s Research Hospital, United StatesReviewed by:
Carine Lampert, The University of Alabama at Birmingham, United StatesCopyright © 2024 Yeum, Renier, Carlson, Ballarino, Lansigan, Meyer, Loos, Emond, Masterson and Gilbert-Diamond. This is an open-access article distributed under the terms of the Creative Commons Attribution License (CC BY). The use, distribution or reproduction in other forums is permitted, provided the original author(s) and the copyright owner(s) are credited and that the original publication in this journal is cited, in accordance with accepted academic practice. No use, distribution or reproduction is permitted which does not comply with these terms.
*Correspondence: Dabin Yeum, ZGFiaW55ZXVtQGdtYWlsLmNvbQ==
Disclaimer: All claims expressed in this article are solely those of the authors and do not necessarily represent those of their affiliated organizations, or those of the publisher, the editors and the reviewers. Any product that may be evaluated in this article or claim that may be made by its manufacturer is not guaranteed or endorsed by the publisher.
Research integrity at Frontiers
Learn more about the work of our research integrity team to safeguard the quality of each article we publish.