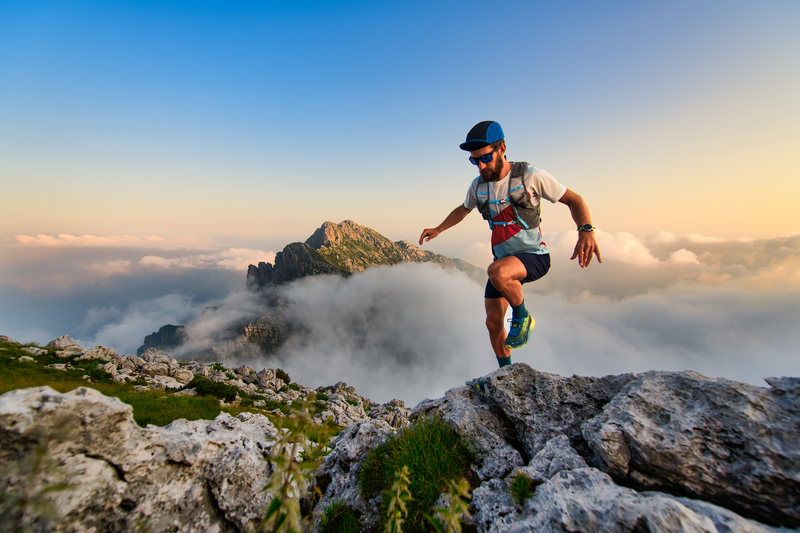
94% of researchers rate our articles as excellent or good
Learn more about the work of our research integrity team to safeguard the quality of each article we publish.
Find out more
SYSTEMATIC REVIEW article
Front. Nutr. , 24 October 2023
Sec. Clinical Nutrition
Volume 10 - 2023 | https://doi.org/10.3389/fnut.2023.1274592
Objective: Recent studies have reported inconsistent results regarding the association between geriatric nutritional risk index (GNRI) and clinical outcomes in patients with hematologic malignancies (HMs). We performed a meta-analysis to evaluate the effect of low GNRI on the overall survival (OS) and progression-free survival (PFS) in patients with HMs.
Research Methods and Procedures: We conducted the research via PubMed, Embase, and Cochrane Library databases to identify trials. Exploring the association between GNRI and prognosis in patients with HMs. A meta-analysis of OS and PFS was performed. Quality In Prognostic Studies instrument and Newcastle–Ottawa quality assessment Scale were used to assess the quality of included trials.
Results: Fourteen studies enrolling 3,524 patients with HMs were included. Low GNRI was associated with shorter OS (Hazard ratio (HR) = 1.77; 95% CI = 1.44–2.18, p < 0.01) and PFS (HR = 1.63; 95% CI = 1.17–2.27, p < 0.01) in patients with HMs. In the subgroup analysis, GNRI was not significantly associated with prognosis in Chinese patients with HMs (OS, HR =1.33; 95% CI = 0.89–1.98, p = 0.16; PFS, HR = 1.70; 95% CI = 0.72–4.01, p = 0.23). For the subgroup with a GNRI cutoff value less than 98, there was no significant difference in PFS (HR = 1.34; 95% CI = 0.98–1.83, p = 0.06).
Conclusion: Low GNRI negatively impacted on the prognosis in patients with HMs. Prospective studies to identify the best cut-off value for GNRI are required.
Hematologic malignancies (HMs), also called blood cancers, are a diverse group of cancers originating from blood-forming tissue or cells of the immune system. There are three main types of hematologic tumors, lymphoma, myeloma, and leukemia. In 2022, there were approximately 184,130 new cases and 57,810 new deaths of hematologic malignancies in the US estimated by the North American Association of Central Cancer Registries and US mortality data (1). Even though much progress has been made in personalized therapy and many new drugs have been developed for HMs in recent years (2), the prognosis of HMs is still poor for some patients, with a 5-year survival ranging from 24 to 86% (3). Immunotherapies, including checkpoint inhibitors and therapeutic vaccines, have also been applied to HMs to improve patient survival (4, 5). However, chemotherapy, radiotherapy and immunotherapies do not achieve satisfactory therapeutic effects for all cancer types and patients with HMs (4). Various prognostic factors based on clinical and pathologic features such as stage of disease, histological subtype, positron emission tomography (PET) imaging, tissue and circulating biomarkers and gene expression profiling have been widely used to predict hematologic malignancies survival (6–9). However, those prognostic factors are not enough for predicting prognosis precisely. In addition, detecting genetic markers is costly and is not readily available in clinical practice in the low-income group.
Multiple studies have confirmed that baseline nutrition status is a common factor associated with decreased overall survival time in patients with HMs undergoing chemotherapy and allogeneic hematopoietic cell transplantation (HCT) (10–14). Moreover, it has been proposed that nutritional treatment would improve the prognosis in patients with HMs before HCT (15). Nevertheless, there are no consensus diagnostic criteria for malnutrition in patients with HMs. Hence, it is crucial to identify an effective nutrition index to predict the prognosis of patients with HMs.
GNRI is a simple and accurate nutritional index invented to predict morbidity and mortality in hospitalized elderly patients (16). GNRI consists of serum albumin levels and the ideal body weight, calculated using the Lorentz formula [1.489 × serum albumin (g/L)] + [41.7 × (current body weight/ideal body weight)]. The height and sex-specific formulas were used to calculate ideal body weight. For GNRI, studies have confirmed its good predictability of clinical outcomes for patients with acute ischemic stroke (17), polytrauma (18), heart failure (19), undergoing emergency surgery (20), and receiving hemodialysis (21). Subsequent studies have verified the prognostic value of GNRI of patients with solid cancers (22–24). Recently, emerging evidence has reported the prognostic significance of pretreatment GNRI in patients with HMs undergoing chemotherapy, immunotherapy and HCT. However, those studies have garnered inconsistent results (25–27). Some studies showed that low GNRI was associated with poorer prognosis in patients with diffuse large B-cell lymphoma (DLBCL), myelodysplastic syndrome (MDS) and acute myeloid leukemia (AML) (28, 29). However, Li’s study suggested that GNRI was not an independent predictor in patients with DLBCL (30). Two previously published meta-analyzes, which reviewed the predictive role of GNRI in DLBCL, both found that low GNRI was associated with shorter survival. However, only seven articles were included. In the results analyzing the relationship between GNRI and PFS, only 2 and 3 studies were included, respectively, which would lead to inaccurate results (31, 32). To date, we found that other five new studies (27, 29, 33–36) also explored the relationship between GNRI and survival outcomes in patients with HMs. To our knowledge, no meta-analyzes have been conducted on the potential prognostic value of GRNI in patients with non-DLBCL HMs. Therefore, we conducted a meta-analysis to summarize emerging evidence regarding the prognostic value of GRNI in patients with HMs.
The methods of the present meta-analysis were performed following the Meta-Analyzes (PRISMA) statement. We searched the PubMed, Embase, and Cochrane Library databases for all references from January 2005 to July 2023. The keywords and MeSH terms “hematologic malignancies,” “Geriatric Nutritional Risk Index” and other related words were used in the search process. The search strategy was [GNRI(All Fields) OR “Geriatric nutritional risk index” (All Fields)] AND [“leukemia” (All Fields) OR “lymphoma” (All Fields) OR “myeloma” (All Fields) OR “hematologic malignancy” (All Fields) OR “hematopoietic malignancy” (All Fields) OR “hematopoietic neoplasms” (All Fields) OR “hematological malignancy” (All Fields) OR “hematological neoplasms” (All Fields) OR “hematologic neoplasms” (All Fields) OR “multiple myeloma” (All Fields) OR “diffuse large B cell lymphoma” (All Fields)]. Two independent researchers searched twice to avoid omissions in the literature search. The references and conference articles were also reviewed.
Studies were included if (1) the study was designed as a prospective cohort study, a randomized controlled trial, or a retrospective study; (2) the subjects were patients diagnosed with any hematologic malignancies; (3) GNRI was calculated before or during the treatment, (4) the outcomes included OS and PFS. Studies published in abstract form, case reports, and non-English literature were excluded.
Two authors extracted data independently from eligible articles; another independently checked all extracted data. The following details were included: the name of the first author, the year of publication, characteristics of study participants, numbers of participants, the cut-off value of GNRI, treatment methods for hematologic malignancies, median OS, and outcomes.
The quality assessment of included trials was performed independently using the Quality In Prognostic Studies tool by two reviewers. This tool consists of six domains: study participation, study attrition, prognostic factor measurement, outcome measurement, study confounding, and statistical analysis and reporting. If more than four of these six domains are at low risk of bias, the overall risk of bias of the literature is low; if two or more than two domains are at high risk of bias, the overall evaluation of literature quality is high, and the remaining studies were classified as the moderate risk of bias (37). The Newcastle–Ottawa quality assessment Scale consists of eight components divided into three areas. Each research received a maximum of nine points. The tool classifies articles with a total score of 6 or more as high-quality, articles with a score of 3 to 6 as intermediate, and articles with a score of less than 3 as poor quality.
The Stata software (version 14) was used for statistical analysis. Cochran’s Q and I squared tests were performed to identify between-study heterogeneity for each analysis. I2 < 25% was defined as no heterogeneity, I2 = 25 ~ 50% moderate heterogeneity, and I2 > 50% considerable heterogeneity. When heterogeneity across studies was considerable, the random-effects model was chosen to calculate the pooled hazard ratio (HR) and the corresponding 95% CIs. Otherwise, the fixed-effects model would be used. HR was defined as risk of outcome in low GNRI group/ risk of outcome in high GNRI group. Most of the adjusted HRs values were extracted from multivariate Cox regression analyzes. If the multivariate analysis was not carried out in the study, the outcomes from univariate Cox regression were used for analysis. A few HRs and 95% CIs were recalculated from Kaplan–Meier curves. A predefined subgroup analysis based on the country, cut-off value, the number of participants, median age, type of HMs and median OS was performed to explore the prognostic role of GNRI further. To further explore the prognostic value of GNRI on lymphoma, a separate pooled analysis and meta regression were performed for studies in which all the participants were lymphoma. The sex (% of males), age (% of median age < 70), stage (% of stage I – II), Eastern Cooperative Oncology Group performance status score (ECGO PS) (% of 0–1), B symptoms (% of absent), lactic dehydrogenase (LDH) (% of normal), country, cut-off value (% of >98), the number of participants (% of number > 200), and median OS(% of OS <48 month) were used as potential moderators to conduct the meta-regression using univariate model. Potential publication bias was judged by funnel plots and the Egger test.
The selection process was summarized in a PRISMA flowchart (Figure 1). Firstly, 47 potential studies were obtained from databases and other sources during the search process. Seventeen studies were excluded due to duplication. Another nine articles were excluded after screening titles and abstracts. Then 21 studies were examined by reviewing the full texts, and seven studies were excluded since they had no interesting results or incomplete data. Ultimately, 14 studies with 15 comparisons met the eligibility criteria and were included.
The baseline characteristics of the included studies are shown in Table 1. Thirteen studies were retrospective and one study was prospective (35). The number of participants in the included studies ranged from 86 to 615. A total of 3,524 patients were enrolled in the 14 studies. Participants in 10 included studies were patients with DLBCL (25, 26, 28, 30, 35, 36, 38–41). Participants in two studies were diagnosed with lymphoma (33, 34), and participants in the other two were diagnosed with myeloid leukemia (27, 29). The cut-off value of GNRI ranged from 92 to 106.26.
The details of the risk of bias are listed in supplemental file, accessed by the QUIPS tools. Three trials had an overall low risk of bias, and one studies had an overall high risk of bias. The rest had an overall moderate risk of bias. While based on the score of Newcastle–Ottawa quality assessment Scale, there were none trials for poor quality, 11 trails for intermediates, and three trials for high quality.
In total, 13 studies reported HRs for OS of patients with HMs. As significant heterogeneity was detected, the random effects model was used (I2 = 67.8%). Patients with low pretreatment GNRI tended to have a shorter OS than those with high GNRI (HR = 1.77; 95% CI = 1.44–2.18, p < 0.01; Figure 2).
Figure 2. The forest plot for the association between GNRI and overall survival. The boxes and the horizontal lines through the boxes illustrate the estimated hazard ratios and the length of the confidence interval. The diamond and horizontal line through the diamond show the pooled hazard ratio and confidence interval.
Subgroup analysis was performed to comprehensively evaluate the relationship between GNRI and OS in patients with HMs. In the subgroup analysis based on the type of HMs, the observed significant survival time reduction was observed in patients with DLBCL (11 studies, HR =1.70; 95% CI = 1.37–2.12, p < 0.01; I2 = 68.9%) and patients with non-DLBCL (3 studies, HR = 2.33; 95% CI = 1.15–4.71, p = 0.02; I2 = 68.7%). In the subgroup analysis based on the region, low GNRI was not associated with poor OS in patients from China (4 studies, HR =1.33; 95% CI = 0.89–1.98, p = 0.16; I2 = 41.7%). In subgroups other than those above, all the pooled data indicated an association between low GNRI and shorter OS in patients with HMs (Table 2).
Table 2. Subgroup analysis of the association between GNRI and the overall survival of patients with HMs.
Nine studies were included in the meta-analysis on the predictive value of GNRI on PFS. Patients with low GNRI had poorer PFS than patients with high GNRI (HR = 1.63; 95% CI = 1.17–2.27, p < 0.01), and significant heterogeneity was detected (I2 = 86.3%; Figure 3).
Figure 3. The forest plot for the association between GNRI and progression-free survival. The boxes and the horizontal lines through the boxes illustrate the estimated hazard ratios and the length of the confidence interval. The diamond and horizontal line through the diamond show the pooled hazard ratio and confidence interval.
No significant association between PFS and GNRI was observed in the subgroup of patients from China (2 studies, HR = 1.70; 95% CI = 0.72–4.01, p = 0.23) and Japan (3 studies, HR = 1.27; 95% CI = 0.91–1.77, p = 0.17). For the subgroup with a cutoff value less than 98, there was no significant difference in PFS (5 studies, HR = 1.34; 95% CI = 0.98–1.83, p = 0.06). In the subgroup with non-DLBCL, no significant difference in PFS was observed for low GNRI vs. high GNRI (2 studies, HR = 1.08; 95% CI = 0.88–1.34, p = 0.46; Table 3).
Table 3. Subgroup analysis of the association between GNRI and the progression-free survival of patients with HMs.
Eleven studies and 12 comparisons were included in the meta-analysis on the predictive value of GNRI on OS for lymphoma. Patients with low GNRI had shorter OS than those with high GNRI (HR = 1.79; 95% CI = 1.42–2.27, p < 0.01), with high heterogeneity (I2 = 72.4%; Figure 4). Meta-regression analyzes showed that no significant part of the variance could be explained by confounders in the heterogeneity including sex, age, stage, ECGO PS, B symptoms, LDH, country, cut-off value, the number of participants, and median OS.
Figure 4. The forest plot for the association between GNRI and overall survival in patients with lymphoma. The boxes and the horizontal lines through the boxes illustrate the estimated hazard ratios and the length of the confidence interval. The diamond and horizontal line through the diamond show the pooled hazard ratio and confidence interval.
Both funnel plot (Figure 5) and Egger’s publication bias plot (Figure 6) were used to evaluate the potential publication bias for the association between GNRI and OS/PFS. Significant publication bias was detected by the Egger’s test (Egger’s test for OS: p = 0.045; Egger’s test for PFS: p = 0.014).
To verify the robustness of the results, a sensitivity analysis was performed. The sensitivity analysis results showed that the overall pooled data of OS and PFS did not change significantly when either included study was omitted (Figure 7).
Since GNRI’s prognostic role has been demonstrated in HMs research, we conducted the meta-analysis to systematically evaluate the relationship between the GNRI and the clinical outcome in patients with HMs. The finding of our meta-analysis showed that low GNRI was associated with shorter OS and PFS. However, the negative effect of low GNRI on the survival was not significant in the subgroup analysis stratified by country. No significant benefit on PFS was not observed in the subgroup analysis based on cut-off value less than 98 and patients with non-DLBCL.
GNRI is an easily obtained, objective simple, efficient, and applicable tool to evaluate nutrition statues in the clinical practice. Currently, nutritional status in hospitalized patients is generally evaluated by systematic nutritional assessment methods, such as subjective global assessment (SGA), malnutrition universal screening tool (MUST), mini nutritional assessment (MNA) and Global Leadership Initiative on Malnutrition (GLIM). These measures include several components, such as weight loss history, anthropometry, and dietary intake change (42–45). In some cases, these approaches are difficult to implement completely. Previous studies have attempted to compare systematic nutritional assessment methods with GNRI. In a study conducted in Egypt, GNRI demonstrated a higher prognostic value for malnutrition-related clinical outcomes in hospitalized patients than MNA (46). The relationship between GNRI and SGA was also well-defined in elderly patients on hemodialysis (47). For GNRI, the serum albumin level can be easily obtained by routine examination of peripheral blood. As problems occurred in measuring the usual bodyweight of the elderly, the GNRI index replaces usual body weight with the ideal body weight (48). In addition, low GNRI predicted poor outcome in many cancers. In Wang’s study, GNRI was identified as a significant prognostic factor for OS and PFS in cervical cancer (49). A meta-analysis with 11 studies also showed that GNRI has good prognostic ability on OS (HR 1.96) in patients with non-small cell lung cancer (50). Our results demonstrated that pretreatment GNRI was an independent prognostic risk factor for patients with HMs.
To our knowledge, the mechanisms underlying linking between GNRI and cancer prognosis could be explained as follows. Firstly, GNRI index directly reflected nutritional status in hospitalized patients. Malnutrition is common in patients with HMs (51, 52). Different from malnutrition caused by simply insufficient energy intake, one of the leading causes of cancer-associated malnutrition is metabolic derangements caused by tumors (53). In addition, chemotherapy-induced nausea, vomiting symptoms, and decreased appetite further lead to a decrease in food intake, exacerbating malnutrition severity (54). A prospective multicenter cohort study has found that the pretreatment malnutrition based on GLIM criteria led to an increase in mortality for patients with non-Hodgkin’s lymphoma (52) and even affected the efficacy of immunotherapy (14). Malnourished patients with HMs are also at high risk of sarcopenia. Sarcopenic patients faced higher chemotherapy toxicity and poorer tolerance to oncological treatments (55). Recent meta-analyzes have also suggested that sarcopenia predicts impaired OS in patients with DLBCL (56). Cancer cachexia refers to weight loss due to sarcopenia and cancer-related inflammation (57). Cancer cachexia has also been proven to affect the clinical outcomes in patients with HMs negatively (58). Secondly, GNRI is also considered an immune nutritional parameter because albumin is closely related to nutrition status and inflammation. Decreased serum albumin levels are the result of inflammation and malnutrition (59). As a nutritional parameter, serum albumin level is a potent predictor for clinical outcomes in patients with acute myeloid leukemia and lymphoma (60, 61).
Another critical feature of albumin is its capability to mediate inflammation. Albumin could bind lipopolysaccharide, lipoteichoic acid, and peptidoglycan to cause inflammation through Toll-like receptor 4 (62). The serum albumin level could be regulated by inflammatory cytokines such as interleukin-1, interleukin-6, and tumor necrosis factor-alpha (63–65). The role of inflammation in the prognosis of HMs should not be ignored. In the included studies, different inflammatory conditions in different patients also influenced the predictive effect of GNRI. Studies have proven that the tumor microenvironment is largely orchestrated by inflammatory cells (66). Local immune response and systemic inflammation both promote the development of tumors and contribute to cancer migration. Cancer-related inflammation also regulates the efficacy of anti-cancer therapies (67, 68), slows the clearance of anticancer drugs and increases the side effects of treatment (69). Although GNRI also reflects the level of inflammation in the body to a certain extent, it is not enough to reflect the level of systemic inflammation in the body. Unfortunately, extracting relevant information for further analysis in meta-analysis is impossible. Studies have proven that the tumor microenvironment is largely orchestrated by inflammatory cells (66). In addition, malnutrition was also associated with inflammation. Weight loss (the so-called B symptoms) was also recognized as a sustained and systemic reaction to cancer (70). Cancer cachexia syndrome was considered as the most extreme result of systemic inflammation (71, 72). The inflammation may be another explanation for predictive role of GNRI on prognosis in HMs.
Notably, we did not observe differences in OS/PFS between low and high GNRI in our meta-analysis results in Chinese patients with HMs. It may be explained by the different study population selected in the included studies. In Li’s study, patients with low GNRI had short survival time, but the significance did not remain after adjusting NCCN- international prognostic index in the multivariate analyzes (30). The situation is less straightforward for studies using a GNRI cutoff less than 98. There was no significant difference in PFS, which suggested that the results may be influenced by the different cutoff values. Different from the previous two published meta-analyzes (31, 32), our study provides more evidence and information on the role of GNRI in predicting PFS in HMs.
Several other articles that explored the predictive values of GNRI cannot be ignored. One study reported that GNRI was significantly associated with ICU mortality for HM patients with acute respiratory failure treated in ICU (73). In Takuji Matsuo’s study, GNRI score < 82 correlated with poor 5-year OS (74). Another study also demonstrated statistical differences in GNRI values between patients who completed complete six or eight cycles of the standard regimens and those who did not (75).
Our meta-analysis is not free of limitations. First, most of the included studies were retrospective. Second, the potential heterogeneity among articles would lead to bias. There was considerable heterogeneity in the meta-analysis as a result of using different GNRI cut-off values. The GNRI cut-off value varied between 92 to 106.3 for OS. While, no moderators reached statistical significance in our meta-regression for lymphoma. Unfortunately, other moderators such as BMI, hemoglobin, extranodal involvement and CNS/liver/bone marrow invasion cannot be obtained from all the included studies. These factors could be significant parts of the variance in the heterogeneity. Third, eleven of the fourteen included studies were conducted in East Asia countries, which may affect the accuracy of the results and cause bias. In addition, the distribution of pathological types, treatment and prognosis of HMs are also different in different regions. Therefore, we were unable to make a partial generalization with limited information. Further research needs to confirm the prognostic effect of GNRI in patients with HMs from other countries. Fourth, the outcome reported in one article was TTF, which we also incorporated in the meta-analysis of PFS. It would cause bias. Fifth, one study included patients with myelodysplastic syndromes in addition to leukemia, considering that myelodysplastic syndromes frequently progress to acute myeloid leukemia, and the American Cancer Society also considered myelodysplastic syndromes as a type of cancer, so we included this study in the meta-analysis. This may also contribute to heterogeneity among studies.
In summary, for patients with HMs, Low GNRI predicted shorter OS and PFS. Identifying the best cut-off value for GNRI applied to patients with different features of HMs and prospective studies to validate the prognostic significance of GNRI in patients with HMs are areas for further research.
The original contributions presented in the study are included in the article/Supplementary material, further inquiries can be directed to the corresponding authors.
QY: Conceptualization, Formal analysis, Writing – original draft. MT: Formal analysis, Writing – review & editing. GP: Data curation, Formal analysis, Writing – review & editing. YJ: Writing – review & editing. XJ: Conceptualization, Formal analysis, Writing – original draft.
The author(s) declare that no financial support was received for the research, authorship, and/or publication of this article.
We would like to thank Yu Qian for great editing.
The authors declare that the research was conducted in the absence of any commercial or financial relationships that could be construed as a potential conflict of interest.
All claims expressed in this article are solely those of the authors and do not necessarily represent those of their affiliated organizations, or those of the publisher, the editors and the reviewers. Any product that may be evaluated in this article, or claim that may be made by its manufacturer, is not guaranteed or endorsed by the publisher.
The Supplementary material for this article can be found online at: https://www.frontiersin.org/articles/10.3389/fnut.2023.1274592/full#supplementary-material
1. Siegel, RL, Miller, KD, Fuchs, HE, and Jemal, A. Cancer statistics, 2022. CA Cancer J Clin. (2022) 72:7–33. doi: 10.3322/caac.21708
2. Sochacka-Cwikla, A, Maczynski, M, and Regiec, A. FDA-approved drugs for hematological malignancies-the last decade review. Cancers (Basel). (2021) 14:87. doi: 10.3390/cancers14010087
3. Centers for Disease Control and Prevention. Hematologic Cancer incidence, survival, and prevalence. USCS data brief, No. 30. Atlanta, GA: Centers for Disease Control and Prevention, US Department of Health and Human Services (2022).
4. Palanca-Wessels, MC, and Press, OW. Advances in the treatment of hematologic malignancies using immunoconjugates. Blood. (2014) 123:2293–301. doi: 10.1182/blood-2013-10-492223
5. Lanier, OL, Perez-Herrero, E, Andrea, APD, Bahrami, K, Lee, E, Ward, DM, et al. Immunotherapy approaches for hematological cancers. iScience. (2022) 25:105326. doi: 10.1016/j.isci.2022.105326
6. Vaidya, R, and Witzig, TE. Prognostic factors for diffuse large B-cell lymphoma in the R(X)CHOP era. Ann Oncol. (2014) 25:2124–33. doi: 10.1093/annonc/mdu109
7. Cramer, P, and Hallek, M. Prognostic factors in chronic lymphocytic leukemia-what do we need to know? Nat Rev Clin Oncol. (2011) 8:38–47. doi: 10.1038/nrclinonc.2010.167
8. Cuccaro, A, Bartolomei, F, Cupelli, E, Galli, E, Giachelia, M, and Hohaus, S. Prognostic factors in hodgkin lymphoma. Mediterr J Hematol Infect Dis. (2014) 6:e2014053. doi: 10.4084/MJHID.2014.053
9. Rubio-Jurado, B, Sosa-Quintero, LS, Carrasco-Martinez, IL, Norato-Delgado, A, Garcia-Luna, E, Guzman-Silahua, S, et al. New biomarkers in non-Hodgkin lymphoma and acute leukemias. Adv Clin Chem. (2020) 96:19–53. doi: 10.1016/bs.acc.2019.11.002
10. Zhang, L, Chen, S, Wang, W, Wang, Y, and Liang, Y. Inflammatory and nutritional scoring system for predicting prognosis in patients with newly diagnosed multiple myeloma. J Inflamm Res. (2023) 16:7–17. doi: 10.2147/JIR.S390279
11. Zhang, L, Chen, S, Huang, M, Wang, W, Liang, Y, and Wang, Y. Prognostic value of the nutritional risk index in patients with newly diagnosed multiple myeloma. Ann Hematol. (2023) 102:125–32. doi: 10.1007/s00277-022-05059-4
12. Orvain, C, Byelykh, M, Othus, M, Sandmaier, BM, Schoch, G, Davis, C, et al. Relationship between Pretransplantation nutritional status and outcome in adults with acute myelogenous leukemia undergoing allogeneic hematopoietic cell transplantation. Transplant Cell Ther. (2022) 28:846.e1–9. doi: 10.1016/j.jtct.2022.09.023
13. Yilmaz, M, Atilla, FD, Sahin, F, and Saydam, G. The effect of malnutrition on mortality in hospitalized patients with hematologic malignancy. Support Care Cancer. (2020) 28:1441–8. doi: 10.1007/s00520-019-04952-5
14. Park, S, Han, B, Cho, JW, Woo, SY, Kim, S, Kim, SJ, et al. Effect of nutritional status on survival outcome of diffuse large B-cell lymphoma patients treated with rituximab-CHOP. Nutr Cancer. (2014) 66:225–33. doi: 10.1080/01635581.2014.867065
15. McMillen, KK, Coghlin-Dickson, T, and Adintori, PA. Optimization of nutrition support practices early after hematopoietic cell transplantation. Bone Marrow Transplant. (2021) 56:314–26. doi: 10.1038/s41409-020-01078-9
16. Bouillanne, O, Morineau, G, Dupont, C, Coulombel, I, Vincent, JP, Nicolis, I, et al. Geriatric nutritional risk index: a new index for evaluating at-risk elderly medical patients. Am J Clin Nutr. (2005) 82:777–83. doi: 10.1093/ajcn/82.4.777
17. Kang, MK, Kim, TJ, Kim, Y, Nam, KW, Jeong, HY, Kim, SK, et al. Geriatric nutritional risk index predicts poor outcomes in patients with acute ischemic stroke - automated undernutrition screen tool. PLoS One. (2020) 15:e0228738. doi: 10.1371/journal.pone.0228738
18. Yeh, CH, Wu, SC, Chou, SE, Su, WT, Tsai, CH, Li, C, et al. Geriatric nutritional risk index as a tool to evaluate impact of malnutrition risk on mortality in adult patients with Polytrauma. Int J Environ Res Public Health. (2020) 17:233. doi: 10.3390/ijerph17249233
19. Li, H, Cen, K, Sun, W, and Feng, B. Prognostic value of geriatric nutritional risk index in elderly patients with heart failure: a meta-analysis. Aging Clin Exp Res. (2021) 33:1477–86. doi: 10.1007/s40520-020-01656-3
20. Jia, Z, El Moheb, M, Nordestgaard, A, Lee, JM, Meier, K, Kongkaewpaisan, N, et al. The geriatric nutritional risk index is a powerful predictor of adverse outcome in the elderly emergency surgery patient. J Trauma Acute Care Surg. (2020) 89:397–404. doi: 10.1097/TA.0000000000002741
21. Yamada, S, Yamamoto, S, Fukuma, S, Nakano, T, Tsuruya, K, and Inaba, M. Geriatric nutritional risk index (GNRI) and creatinine index equally predict the risk of mortality in hemodialysis patients: J-DOPPS. Sci Rep. (2020) 10:5756. doi: 10.1038/s41598-020-62720-6
22. Sasaki, M, Miyoshi, N, Fujino, S, Ogino, T, Takahashi, H, Uemura, M, et al. The geriatric nutritional risk index predicts postoperative complications and prognosis in elderly patients with colorectal cancer after curative surgery. Sci Rep. (2020) 10:10744. doi: 10.1038/s41598-020-67285-y
23. Nakayama, M, Gosho, M, Adachi, M, Ii, R, Matsumoto, S, Miyamoto, H, et al. The geriatric nutritional risk index as a prognostic factor in patients with advanced head and neck Cancer. Laryngoscope. (2021) 131:E151–6. doi: 10.1002/lary.28587
24. Kang, HW, Seo, SP, Kim, WT, Yun, SJ, Lee, SC, Kim, WJ, et al. A low geriatric nutritional risk index is associated with aggressive pathologic characteristics and poor survival after nephrectomy in clear renal cell carcinoma: a multicenter retrospective study. Nutr Cancer. (2020) 72:88–97. doi: 10.1080/01635581.2019.1621357
25. Yan, D, Shen, Z, Zhang, S, Hu, L, Sun, Q, Xu, K, et al. Prognostic values of geriatric nutritional risk index (GNRI) and prognostic nutritional index (PNI) in elderly patients with diffuse large B-cell lymphoma. J Cancer. (2021) 12:7010–7. doi: 10.7150/jca.62340
26. Atas, U, Sozel, H, Iltar, U, Yucel, OK, Salim, O, and Undar, L. The prognostic impact of pretreatment geriatric nutritional risk index in patients with diffuse large B-cell lymphoma. Nutr Cancer. (2023) 75:591–8. doi: 10.1080/01635581.2022.2142248
27. Nagata, A, Otsuka, Y, Konuma, R, Adachi, H, Wada, A, Kishida, Y, et al. Weight-adjusted urinary creatinine excretion predicts transplant outcomes in adult patients with acute myeloid leukemia in complete remission. Leuk Lymphoma. (2022) 63:3117–27. doi: 10.1080/10428194.2022.2109334
28. Chuang, TM, Liu, YC, Hsiao, HH, Wang, HC, Du, JS, Yeh, TJ, et al. Low geriatric nutritional risk index is associated with poorer prognosis in elderly diffuse large b-cell lymphoma patients unfit for intensive anthracycline-containing therapy: a real-world study. Nutrients. (2021) 13:3243. doi: 10.3390/nu13093243
29. Nishiyama-Fujita, Y, Nakazato, T, Kamiya, T, Mizuno, K, Ito, C, Ogura, S, et al. The geriatric nutritional risk index predicts the early death and survival in patients with myelodysplastic syndrome and acute myeloid leukemia with myelodysplasia-related changes treated with azacitidine. Hematol Oncol. (2020) 38:611–3. doi: 10.1002/hon.2760
30. Li, Z, Guo, Q, Wei, J, Jin, J, and Wang, J. Geriatric nutritional risk index is not an independent predictor in patients with diffuse large B-cell lymphoma. Cancer Biomark. (2018) 21:813–20. doi: 10.3233/CBM-170754
31. Cao, D, and Zhang, Z. Prognostic and clinicopathological role of geriatric nutritional risk index in patients with diffuse large B-cell lymphoma: a meta-analysis. Frontiers. Oncology. (2023) 13:13. doi: 10.3389/fonc.2023.1169749
32. Yan, C, Xie, Y, Hua, Y, Li, S, Fu, H, Cheng, Z, et al. Prognostic value of geriatric nutritional risk index in patients with diffuse large B-cell lymphoma: a meta-analysis. Clin Transl Oncol. (2023) 2023:3271. doi: 10.1007/s12094-023-03271-w
33. Mizuno, K, Nakazato, T, Ito, C, Fujita, Y, Ogura, S, Kamiya, T, et al. The prognostic value of geriatric nutritional risk index in patients with follicular lymphoma. Ann Hematol. (2019) 98:1777–9. doi: 10.1007/s00277-019-03703-0
34. Kikuchi, K, Sawada, M, Takeda, G, Watanabe, T, Souma, T, and Sato, H. Influence of geriatric nutritional risk index on occurrence of adverse events and duration of treatment in patients with lymphoma receiving R-CHOP therapy. In Vivo. (2023) 37:1297–303. doi: 10.21873/invivo.13208
35. Juliette, P, Hélène, L, Caroline, T, Anne-Lise, M, Vincent, C, Aspasia, S, et al. Prognostic relevance of sarcopenia, geriatric, and nutritional assessments in older patients with diffuse large B-cell lymphoma: results of a multicentric prospective cohort study. Ann Hematol. (2023) 102:1811–23. doi: 10.1007/s00277-023-05200-x
36. Yagi, Y, Kanemasa, Y, Sasaki, Y, Goto, S, Yamamura, Y, Ishimine, K, et al. Utility of the frailty score for predicting prognosis and individualizing treatment intensity in elderly patients with diffuse large B cell lymphoma. Ann Hematol. (2023) 102:1485–500. doi: 10.1007/s00277-023-05233-2
37. Foroutan, F, Guyatt, GH, O’Brien, K, Bain, E, Stein, M, Bhagra, S, et al. Prognosis after surgical replacement with a bioprosthetic aortic valve in patients with severe symptomatic aortic stenosis: systematic review of observational studies. BMJ. (2016) 354:i5065. doi: 10.1136/bmj.i5065
38. Go, SI, Kim, HG, Kang, MH, Park, S, and Lee, GW. Prognostic model based on the geriatric nutritional risk index and sarcopenia in patients with diffuse large B-cell lymphoma. BMC Cancer. (2020) 20:439. doi: 10.1186/s12885-020-06921-2
39. Lee, S, Fujita, K, Morishita, T, Negoro, E, Oiwa, K, Tsukasaki, H, et al. Prognostic utility of a geriatric nutritional risk index in combination with a comorbidity index in elderly patients with diffuse large B cell lymphoma. Br J Haematol. (2021) 192:100–9. doi: 10.1111/bjh.16743
40. Matsukawa, T, Suto, K, Kanaya, M, Izumiyama, K, Minauchi, K, Yoshida, S, et al. Validation and comparison of prognostic values of GNRI, PNI, and CONUT in newly diagnosed diffuse large B cell lymphoma. Ann Hematol. (2020) 99:2859–68. doi: 10.1007/s00277-020-04262-5
41. Kanemasa, Y, Shimoyama, T, Sasaki, Y, Hishima, T, and Omuro, Y. Geriatric nutritional risk index as a prognostic factor in patients with diffuse large B cell lymphoma. Ann Hematol. (2018) 97:999–1007. doi: 10.1007/s00277-018-3273-1
42. Da Silva, FJ, Daniel de Mello, P, and Daniel de Mello, E. Subjective global assessment of nutritional status - a systematic review of the literature. Clin Nutr. (2015) 34:785–92. doi: 10.1016/j.clnu.2014.12.014
43. Donini, LM, Poggiogalle, E, Molfino, A, Rosano, A, Lenzi, A, Rossi Fanelli, F, et al. Mini-nutritional assessment, malnutrition universal screening tool, and nutrition risk screening tool for the nutritional evaluation of older nursing home residents. J Am Med Dir Assoc. (2016) 17:959.e11–8. doi: 10.1016/j.jamda.2016.06.028
44. Stratton, RJ, Hackston, A, Longmore, D, Dixon, R, Price, S, Stroud, M, et al. Malnutrition in hospital outpatients and inpatients: prevalence, concurrent validity and ease of use of the ‘malnutrition universal screening tool’ (’MUST’) for adults. Br J Nutr. (2004) 92:799–808. doi: 10.1079/bjn20041258
45. Cederholm, T, Jensen, GL, Correia, M, Gonzalez, MC, Fukushima, R, Higashiguchi, T, et al. GLIM criteria for the diagnosis of malnutrition - a consensus report from the global clinical nutrition community. Clin Nutr. (2019) 38:1–9. doi: 10.1016/j.clnu.2018.08.002
46. Abd-El-Gawad, WM, Abou-Hashem, RM, El Maraghy, MO, and Amin, GE. The validity of geriatric nutrition risk index: simple tool for prediction of nutritional-related complication of hospitalized elderly patients. Comparison with Mini nutritional assessment. Clin Nutr. (2014) 33:1108–16. doi: 10.1016/j.clnu.2013.12.005
47. Spatola, L, Finazzi, S, Santostasi, S, Angelini, C, and Badalamenti, S. Geriatric nutritional risk index is predictive of subjective global assessment and Dialysis malnutrition scores in elderly patients on hemodialysis. J Ren Nutr. (2019) 29:438–43. doi: 10.1053/j.jrn.2019.01.012
48. Abd Aziz, NAS, Teng, N, Abdul Hamid, MR, and Ismail, NH. Assessing the nutritional status of hospitalized elderly. Clin Interv Aging. (2017) 12:1615–25. doi: 10.2147/CIA.S140859
49. Wang, HB, Xu, XT, Tian, MX, Ding, CC, Tang, J, Qian, Y, et al. Prognostic values of the prognostic nutritional index, geriatric nutritional risk index, and systemic inflammatory indexes in patients with stage IIB-III cervical cancer receiving radiotherapy. Front Nutr. (2023) 10:1000326. doi: 10.3389/fnut.2023.1000326
50. Shen, F, Ma, Y, Guo, W, and Li, F. Prognostic value of geriatric nutritional risk index for patients with non-small cell lung Cancer: a systematic review and Meta-analysis. Lung. (2022) 200:661–9. doi: 10.1007/s00408-022-00567-6
51. Wang, F, Dong, Q, Yu, K, Li, RR, Fu, J, Guo, JY, et al. Nutrition risk screening and related factors analysis of non-hospitalized Cancer survivors: a Nationwide online survey in China. Front Nutr. (2022) 9:920714. doi: 10.3389/fnut.2022.920714
52. Zou, Y, Xu, H, Lyu, Q, Weng, M, Cui, J, Shi, H, et al. Malnutrition diagnosed by GLIM criteria better predicts long-term outcomes for patients with non-Hodgkin’s lymphoma: a prospective multicenter cohort study. Hematol Oncol. (2022) 41:371–9. doi: 10.1002/hon.3107
53. Arends, J. Struggling with nutrition in patients with advanced cancer: nutrition and nourishment-focusing on metabolism and supportive care. Ann Oncol. (2018) 29:ii27. doi: 10.1093/annonc/mdy093
54. Morton, M, Patterson, J, Sciuva, J, Perni, J, Backes, F, Nagel, C, et al. Malnutrition, sarcopenia, and cancer cachexia in gynecologic cancer. Gynecol Oncol. (2023) 175:142–55. doi: 10.1016/j.ygyno.2023.06.015
55. Bozzetti, F. Forcing the vicious circle: sarcopenia increases toxicity, decreases response to chemotherapy and worsens with chemotherapy. Ann Oncol. (2017) 28:2107–18. doi: 10.1093/annonc/mdx271
56. Xu, XT, He, DL, Tian, MX, Wu, HJ, and Jin, X. Prognostic value of sarcopenia in patients with diffuse large B-cell lymphoma treated with R-CHOP: a systematic review and Meta-analysis. Front Nutr. (2022) 9:816883. doi: 10.3389/fnut.2022.816883
57. Meza-Valderrama, D, Marco, E, Davalos-Yerovi, V, Muns, MD, Tejero-Sanchez, M, Duarte, E, et al. Sarcopenia, malnutrition, and Cachexia: adapting definitions and terminology of nutritional disorders in older people with Cancer. Nutrients. (2021) 13:761. doi: 10.3390/nu13030761
58. Go, SI, Park, MJ, Park, S, Kang, MH, Kim, HG, Kang, JH, et al. Cachexia index as a potential biomarker for cancer cachexia and a prognostic indicator in diffuse large B-cell lymphoma. J Cachexia Sarcopenia Muscle. (2021) 12:2211–9. doi: 10.1002/jcsm.12837
59. Don, BR, and Kaysen, G. Serum albumin: relationship to inflammation and nutrition. Semin Dial. (2004) 17:432–7. doi: 10.1111/j.0894-0959.2004.17603.x
60. Bairey, O, Shacham-Abulafia, A, Shpilberg, O, and Gurion, R. Serum albumin level at diagnosis of diffuse large B-cell lymphoma: an important simple prognostic factor. Hematol Oncol. (2016) 34:184–92. doi: 10.1002/hon.2233
61. Yokus, O, Sunger, E, Cinli, TA, Goze, H, and Serin, I. Serum albumin and ferritin levels: a practical indicator of prognosis in acute myeloid leukemia over 50 years of age? Am J Blood Res. (2022) 12:97–104.
62. Arroyo, V, Garcia-Martinez, R, and Salvatella, X. Human serum albumin, systemic inflammation, and cirrhosis. J Hepatol. (2014) 61:396–407. doi: 10.1016/j.jhep.2014.04.012
63. Moshage, HJ, Janssen, JA, Franssen, JH, Hafkenscheid, JC, and Yap, SH. Study of the molecular mechanism of decreased liver synthesis of albumin in inflammation. J Clin Invest. (1987) 79:1635–41. doi: 10.1172/JCI113000
64. Huang, Y, Shinzawa, H, Togashi, H, Takahashi, T, Kuzumaki, T, Otsu, K, et al. Interleukin-6 down-regulates expressions of the aldolase B and albumin genes through a pathway involving the activation of tyrosine kinase. Arch Biochem Biophys. (1995) 320:203–9. doi: 10.1016/0003-9861(95)90001-2
65. McCarthy, ET, Sharma, R, Sharma, M, Li, JZ, Ge, XL, Dileepan, KN, et al. TNF-alpha increases albumin permeability of isolated rat glomeruli through the generation of superoxide. J Am Soc Nephrol. (1998) 9:433–8. doi: 10.1681/ASN.V93433
66. Coussens, LM, and Werb, Z. Inflammation and cancer. Nature. (2002) 420:860–7. doi: 10.1038/nature01322
67. Grivennikov, SI, Greten, FR, and Karin, M. Immunity, inflammation, and cancer. Cells. (2010) 140:883–99. doi: 10.1016/j.cell.2010.01.025
68. Crusz, SM, and Balkwill, FR. Inflammation and cancer: advances and new agents. Nat Rev Clin Oncol. (2015) 12:584–96. doi: 10.1038/nrclinonc.2015.105
69. Slaviero, KA, Clarke, SJ, and Rivory, LP. Inflammatory response: an unrecognised source of variability in the pharmacokinetics and pharmacodynamics of cancer chemotherapy. Lancet Oncol. (2003) 4:224–32. doi: 10.1016/s1470-2045(03)01034-9
70. Diakos, CI, Charles, KA, McMillan, DC, and Clarke, SJ. Cancer-related inflammation and treatment effectiveness. Lancet Oncol. (2014) 15:e493–503. doi: 10.1016/S1470-2045(14)70263-3
71. Peixoto da Silva, S, Santos, JMO, Costa e Silva, MP, Gil da Costa, RM, and Medeiros, R. Cancer cachexia and its pathophysiology: links with sarcopenia, anorexia and asthenia. J Cachexia Sarcopenia Muscle. (2020) 11:619–35. doi: 10.1002/jcsm.12528
72. MacDonald, N. Terminology in cancer cachexia: importance and status. Curr Opin Clin Nutr Metab Care. (2012) 15:220–5. doi: 10.1097/MCO.0b013e328352a895
73. Kundu, R, Seeger, R, Elfassy, MD, Rozenberg, D, Ahluwalia, N, Detsky, ME, et al. The association between nutritional risk index and ICU outcomes across hematologic malignancy patients with acute respiratory failure. Ann Hematol. (2023) 102:439–45. doi: 10.1007/s00277-022-05064-7
74. Matsuo, T, Tashiro, H, Shirasaki, R, Sumiyoshi, R, Yamamoto, T, Saito, S, et al. Serum high-density lipoprotein cholesterol level has a significant prognostic impact on outcomes of follicular lymphoma patients. Medicine (United States). (2022) 101:E29541. doi: 10.1097/MD.0000000000029541
Keywords: geriatric nutritional risk index, diffuse large B-cell lymphoma, hematologic malignancies, overall survival, meta-analysis
Citation: Yu Q, Tian M, Pi G, Jia Y and Jin X (2023) Geriatric nutritional risk index as a predictor of prognosis in hematologic malignancies: a systematic review and meta-analysis. Front. Nutr. 10:1274592. doi: 10.3389/fnut.2023.1274592
Received: 08 August 2023; Accepted: 29 September 2023;
Published: 24 October 2023.
Edited by:
Harriët Jager-Wittenaar, Hanze University of Applied Sciences, NetherlandsReviewed by:
Barbara Van Der Meij, HAN University of Applied Sciences, NetherlandsCopyright © 2023 Yu, Tian, Pi, Jia and Jin. This is an open-access article distributed under the terms of the Creative Commons Attribution License (CC BY). The use, distribution or reproduction in other forums is permitted, provided the original author(s) and the copyright owner(s) are credited and that the original publication in this journal is cited, in accordance with accepted academic practice. No use, distribution or reproduction is permitted which does not comply with these terms.
*Correspondence: Yegui Jia, anlnMjE4QHNpbmEuY29t; Xin Jin, amlueGlucmRAYWx1bW5pLmh1c3QuZWR1LmNu
†These authors have contributed equally to this work
Disclaimer: All claims expressed in this article are solely those of the authors and do not necessarily represent those of their affiliated organizations, or those of the publisher, the editors and the reviewers. Any product that may be evaluated in this article or claim that may be made by its manufacturer is not guaranteed or endorsed by the publisher.
Research integrity at Frontiers
Learn more about the work of our research integrity team to safeguard the quality of each article we publish.