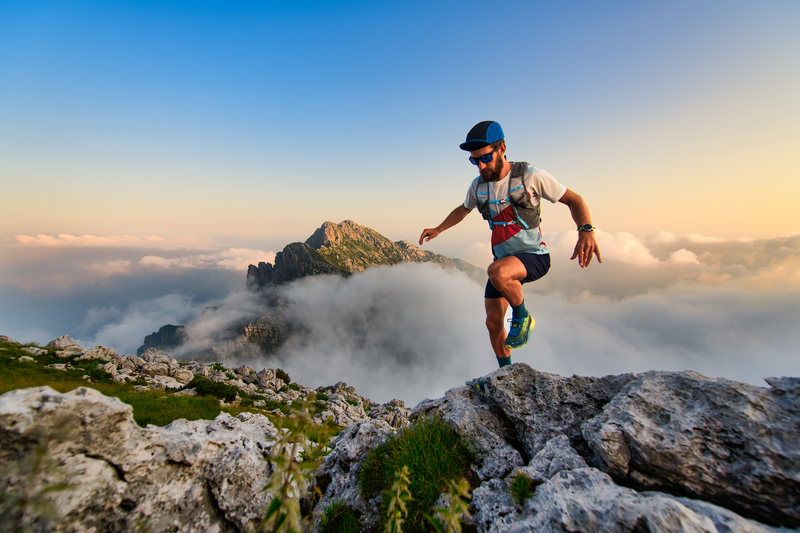
94% of researchers rate our articles as excellent or good
Learn more about the work of our research integrity team to safeguard the quality of each article we publish.
Find out more
ORIGINAL RESEARCH article
Front. Mar. Sci. , 23 January 2023
Sec. Global Change and the Future Ocean
Volume 9 - 2022 | https://doi.org/10.3389/fmars.2022.1070341
This article is part of the Research Topic Ecological Consequences of Climate Change in Boreal Marginal Seas View all 11 articles
Climate change is projected to cause alterations in northern coastal systems, including humification and intensified nutrient loads, which can lead to ecosystem imbalances and establishment of new bacterial species. Several potential pathogens, such as different species of Legionella, hide in the environment between infections, some by living inside protozoan host cells. Knowledge about the occurrence of Legionella in natural waters is missing, which disable risk assessments of exposure. We performed a study of the species diversity of Legionella in the northern Baltic Sea (Gulf of Bothnia) during early summer to map their occurrence and to identify possible environmental drivers. We detected Legionella and potential protozoan hosts along gradients of the Gulf of Bothnia. We also for the first time present third generation full-length 16S rRNA amplicon sequencing (Nanopore) to resolve environmental species classification of Legionella, with a method suitable to study all bacteria. Our data show that full length 16S rRNA sequences is sufficient to resolve Legionella while the standard short Illumina sequences did not capture the entire diversity. For accurate species classification of Legionella, harmonization between the Nanopore classification methods is still needed and the bias toward the well-studied Legionella pneumophila need to be resolved. Different Legionella species occurred both in the Bothnian Sea and in the Bothnian Bay and their abundance were linked to humic substances and low salinity. The relative abundance of Legionella was higher in the humic-rich northern waters of the Bothnian Bay. The link between Legionella species and humic substances may be indirect via promotion of the heterotrophic microbial food web, allowing Legionella species and similar bacteria to establish. Humic substances are rich in iron, which has been shown crucial for growth of Legionella species and other pathogens. Considering climate change projections in this regional area, with increased humification and freshwater inflow, this bacterial niche containing potential pathogens might become more widespread in the future Baltic Sea. This study demonstrates the significance of DNA sequencing to monitor public health relevant bacteria like Legionella species in the environment. Including sequencing of bacteria and protozoa in the environmental monitoring programs could be used to identify ecosystem imbalances, which enable appropriate responses to emerging diseases.
Climate change is projected to cause alterations in the Baltic Sea, such as humification that leads to decreasing salinity and increased water temperatures. This can lead to ecosystem imbalances and the emergence or establishment of new bacterial species. Several bacterial pathogens, such as Legionella pneumophila, has its natural lifecycle in the environment and can live inside protozoan host cells. This ecological niche of predation resistant bacteria are relevant for public health, since some species can cause disease in humans (Thomas et al., 2010).
Bacterial defense strategies has evolved due to the co-existence between bacteria and their predators (protozoa). Some of these strategies contribute to the ability of bacteria to cause human disease. Francisella tularensis, Legionella pneumophila, Vibrio cholerae and Yersinia pestis all are examples of pathogenic bacteria that have anti-predator strategies. The mechanisms bacteria use for both of these traits are often similar (Sun et al., 2018; Amaro and Martín-González, 2021). To understand the emergence of diseases from opportunistic or pathogenic bacteria, it is important to complement studies of bacteria in association to the susceptible host (human cells) with studies of bacteria in the environment of their natural lifecycle. It is likely that bacterial traits that result in pathogenicity to humans originally arose as a response to environmental stress such as predation (Park et al., 2020). For example, Legionella is a predation resistant bacteria that reside in the environment, and sporadically cause problems in water supplies (Hamilton et al., 2018; Leoni et al., 2018; Holsinger et al., 2022). Still, there is a knowledge gap regarding the occurrence and persistence of pathogenic and predation resistant bacteria like Legionella in natural environments including water.
What allows public health relevant species within the genus Legionella to survive in environmental water is unknown, even though more than 20 out of 71 reported species within Legionella have been recognized as human pathogens and the causative agent of Legionnaires’ disease and Pontiac fever (Muder and Yu, 2002; Legionella Species - Special Pathogens Laboratory, 2020; Parte et al., 2020). L. pneumophila is the most studied species in human disease as well as in environmental sampling. Other Legionella non-pneumophila species that cause disease are less studied and undiagnosed (Pascale et al., 2021). Thus, there is a need to investigate non-pneumophila species (Duron et al., 2018).
Bacterial species within Legionella has been isolated from various types of environments such as soils and drinking water (Chambers et al., 2021). Even though freshwater is considered the major reservoir of L. pneumophila (Boamah et al., 2017), the bacterium is mostly studied in man-made water systems such as cooling towers (Brigmon et al., 2020), where the thermophilic bacteria have been shown to dwell (Lesnik et al., 2015). Only a few studies have reported occurrence of DNA sequences specific to the genus Legionella in natural aquatic environments such as in lakes, rivers, brackish and marine waters (Carvalho et al., 2007; Parthuisot et al., 2010; Shimada et al., 2021). Thus, there is a need to increase our understanding of the natural diversity of Legionella spp. in aquatic systems. One approach to understand driving mechanisms of aquatic bacteria is mapping them across dynamic habitats. Freshwater and marine systems are especially dynamic in coastal zones that function as filters for chemical compounds and organisms entering the ocean through rivers (Lamb et al., 2017). Due to this inflow, the coast is generally more nutrient rich compared to the offshore areas. Thus, bacteria that inhabit these interfaces between freshwater and marine systems are exposed to stresses/disturbances such as nutrient rich river inflow, which may lead to fluctuations in selection dynamics.
The Baltic Sea is at risk for large fluctuations, maybe even to the scale of the structure and function of the ecosystem (Blenckner et al., 2015). Projections indicate that the Baltic Sea will suffer alterations due to climate change, both due to increased temperature and due to increased inflow of organic matter caused by precipitation (Andersson et al., 2015; Harvey et al., 2015; Meier et al., 2022). Bacteria can use humic rich organic substances as food resource, giving them an ecological advantage over other plankton organisms. This, in turn, will cause lowered planktonic food quality as for example high C to N ratios and poorer fatty acid composition (Bandara et al., 2022; Guo et al., 2022). Previous research show that in the north of the Gulf of Bothnia phosphorus is the most limiting nutrient, while in the south nitrogen limits organismal growth (Andersson et al., 1996; Tamminen and Andersen, 2007). These habitat variations can select for different bacterial species. The brackish Baltic Sea provides a unique opportunity to study drivers along natural environmental gradients, where the northern parts are more similar to freshwater and the southern parts are more saline.
In the middle of the Gulf of Bothnia, along a 10 km coastal area, a previous study reported widespread occurrence of Legionella spp. in early summer (Eriksson et al., 2022). To map occurrence of Legionella spp. on a larger spatial scale in the Gulf of Bothnia and with the aim to find environmental drivers, we here analyzed samples in the coast-offshore and north-south gradients in early summer. We used two complementary methods for a general bacterial identification to capture the wide ecological niche of predation resistant bacteria. Then, we particularly focused on Legionella, with the aim to also resolve the species. The methods used were sequencing the V4 region of the 16S rRNA gene (using Illumina sequencing) and the V1-9 region (using Nanopore sequencing). To support the species classification, we amplified a L. pneumophila specific macrophage infectivity potentiator (mip) gene that is associated with virulence (Ballard et al., 2000; Nazarian et al., 2008; Collins et al., 2015; Yin et al., 2022). We expected that relatively high concentrations of humic substances in the coast and the open northern basin of the Gulf of Bothnia (Bothnian Bay) would promote bacterial production and their predators (protozoa), which in turn constitute a niche for Legionella spp. In the southern open basin, Bothnian Sea, the humic substance concentration is lower and thus we expected lower bacterial production, as well as lower abundance of protozoa and Legionella spp.
In June 2019, water samples were collected at 9 different stations (5m depth) in the Gulf of Bothnia: Råneå 1 (RA1), Råneå 2 (RA2), A5, A13, Örefjärden (B3), Gavik (GA1), C3, C14 and C24 (Figure 1). Stations RA1, RA2, A5 and A13 are located in the Bothnian Bay basin (northern Gulf of Bothnia). Stations B3, C14, GA1, C3 and C24 are located in Bothnian Sea basin (southern Gulf of Bothnia). Stations RA1, RA2, GA1, B3 and C14 are coastal sampling sites. Coordinates can be found in Supplementary Material 2.
Figure 1 Map of the stations in the Gulf of Bothnia. Northern stations RA1, RA2, A5 and A13 are located in the Bothnian Bay basin (northern Gulf of Bothnia). Southern stations B3, C14, GA1, C3 and C24 are located in the Bothnian Sea basin (southern Gulf of Bothnia). Costal stations: RA1, RA2, B3, C14 and GA1. Offshore stations: A5, A13, C3 and C24.
Umeå Marine Science Centre (UMSC) collected the environmental data via the Swedish National Environmental monitoring program. Data were analyzed according to the HELCOM Manual for Marine Monitoring in the COMBINE report (Cooperative Monitoring in the Baltic Marine Environment).
The included variables for this study were conductivity, temperature and depth (CTD), bacterial production, humic substances, nutrient salts, dissolved organic carbon (DOC), total nitrogen and total phosphorous, collected at 5m depth. For Chlorophyll-a and primary production, a pooled fraction of the surface water (1-10m) was used. Procedures for the measurements are presented at HELCOM (HELCOM, 2017). The data was accessed at SHARKdata (SHARKdata - Datasets, 2022). Differences in environmental concentration levels were estimated using linear models fitted within R, comparing the Bothnian Bay (north) and the Bothnian Sea (south).
At each sampling site, 75-500 ml water was gently filtered (≤20kPa) onto 0.2 µm filters (Pall Corporation sterilized filters, Supor ® 0.2 µm, 47 mm, S-pack white gridded). For samples from RA1 and RA2, <500ml was filtered due to limitations with the filtering of turbid water (100 ml and 250 ml, respectively). A total of 9 water samples were collected. The filters were folded using cleaned tweezers and placed in 2 ml Eppendorf tubes. The samples were stored in -80°C until the DNA extraction. Filters were thawed and placed into Powerwater bead beating tubes. The DNA was extracted using a DNeasy® PowerWater® kit (Qiagen, Hilden, Germany) according to a modified DNeasy PowerWater protocol, where the samples were treated with an additional heating step (horizontal water bath for 30 min, 65°C) and with a bead-beating step in each direction of 20 Hz, 3x3min with a Tissuelyser II (Qiagen, Hilden, Germany). The concentration of the DNA extracts from the stations ranged from 6-46 ng/µl. Two filter blanks were used as negative control during DNA extraction. The final DNA was frozen before subjected to PCR analysis. Sample preparation, thermal cycling and PCR product preparation were performed in separate rooms.
For the 16S rRNA amplicon preparation, DNA was amplified and sequenced as previously described (Hägglund et al., 2018), part from the PCR purification kit used. In short, the DNA was amplified using the No. 5 Hot Mastermix 2.5x kit (5 PRIME, Qiagen, Hilden, Germany) with bacteria/archaeal primers 515F/806R specific for the hypervariable V4 region of the 16S rRNA gene (Caporaso et al., 2012). BSA has been shown to reduce PCR inhibition from humic substances (Opel et al., 2010; Sidstedt et al., 2020). To avoid inhibition, 8 µg of BSA was therefore used for each reaction. The forward and reverse primers were modified to incorporate a 12 bp Golay error-correcting barcode that enables sample multiplexing (Caporaso et al., 2012). All samples were amplified in triplets and pooled after PCR amplification (94°C for 3 min; 35 cycles of 94°C for 45 s, 50°C for 1 min, 72°C for 1.5 min; 10 min rest to finish). For PCR, 3 template blank reactions were included by using nuclease free water instead of template.
For the 18S rRNA amplicon preparation, DNA was amplified using the No. 5 Hot Mastermix 2.5x kit (5 PRIME, Qiagen, Hilden, Germany) with eukaryotic primers V6F/V8R (V6F: 5’- AATTYGAHTCAACRCGGG, V8R: 5’-GACRGGCGGTGTGNACAA) specific for the hypervariable V6-V8 region of the 18S rRNA gene. These primers were designed by Eriksson et al. (2022), using higher degeneracies than earlier publications (Edgcomb et al., 2011; Hadziavdic et al., 2014). The benefits of this region is described in detail by Latz et al. (2022). All samples were amplified in triplets and pooled after PCR amplification (94°C for 3 min; 35 cycles of 94°C for 45 s, 57°C for 1 min, 72°C for 1.5 min; 10 min rest to finish).
The PCR product was run on a 1% agarose gel and the DNA concentration was estimated with a Qubit fluorometer (Invitrogen, Carlsbad, CA, United States). The amplicons were pooled at equimolar concentrations and purified with the QIAquick PCR purification kit (Qiagen, Hilden, Germany) following the supplier’s instructions. The DNA concentration of the pooled amplicon product was measured with a Qubit fluorometer and adjusted to 2 nM. The library was denatured and diluted as described by Illumina (MiSeq System User Guide, Part # 15027617 Rev. C), before it was loaded onto a MiSeq cartridge (Illumina, San Diego, CA, United States) and sequenced using a 2x300 bp paired-end sequencing protocol (Hägglund et al., 2018).
Demultiplexing was done using deML (Renaud et al., 2015). The Quantitative Insights Into Microbial Ecology (QIIME2) pipeline, version 2019.1, was used for processing raw sequence data (Bolyen et al., 2019). Greengenes version 13.8 (McDonald et al., 2012) and PR2 version 4.12.0 (Guillou et al., 2012) databases were used for bacteria and eukaryote taxonomic assignment. Quality filtering was done using dada2 default parameter values. For the 16S rRNA sequence raw data processing, the reads were trimmed to 275 bp for the forward read and to 250 bp for the reverse read. For the 18S rRNA sequence raw data processing, the forward read were trimmed to 290 bp allowing a maximum of 5 expected errors per read. For 18S rRNA, the reverse read were not used due to low quality.
The Illumina 16S rRNA amplicon sequencing yielded 10549128 reads, 5211659 (49.4%) of which remained after the data was processed into amplicon sequence variants (ASVs). The data was filtered, denoised, merged and chimeras were removed, resulting in 4497 ASVs; the number of reads per sample ranged from 242562 to 562960. The Illumina 18S rRNA amplicon sequencing yielded 323690 reads, 63790 of which remained after the data was processed (19.7%, forward read only), resulting in 97 ASVs; the number of reads per sample ranged from 11815 to 2593.
To increase the taxonomic resolution and to compare with Illumina generated data, longer amplicons were also prepared and sequenced using the Oxford Nanopore Technology (ONT). In short for the 16S rRNA amplicons, the DNA was amplified using the Terra PCR Direct Polymerase Mix (Clontech, Mountain View, CA) with bacteria/archaeal primers with ONT overhang (S-D-Bact-0008-c-S-20 (27F): 5’-AGRGTTYGATYMTGGCTCAG-3’, S-D-Bact-1492-a-A-22 (1492-R): 5’-TACGGYTACCTTGTTACGACTT) (Lane, 1991) amplifying the V1-V9 region of the 16S rRNA gene. To avoid inhibition, 8 µg of BSA was used for each reaction. All samples were amplified in duplicates using 30 and 33 cycles (98°C for 2 min; 30 or 33 cycles of 98°C for 10 s, 57°C for 15 s, 68°C for 1.5 min; 68°C for 5 min rest to finish).
Single stranded DNA were removed from the PCR products using 2 µl ExoSAP-IT (Applied Biosystems, USA) to ~150 fmol/sample and each purified sample were then quantified using the Qubit 1×HS Assay Kit (Thermo Fisher Scientific).
The PCR Barcoding Expansion kit (EXP-PBC096) together with Ligation Sequencing Kit (SQK-LSK109) were used to sequence the 16S rRNA amplicons PCR. After the PCR-barcoding step in the protocol a purification of PCR products were performed using 0.5× of AMPure magnetic beads (Beckmann) following the manufacturer’s protocol, thereafter, the purified PCR products were quantified using the Qubit1×HS Assay Kit (Thermo Fisher Scientific), and were pooled in equimolar amounts (200 ng/sample) before proceeding with the protocol. Some modifications were made within the protocol to adjust the volumes for the following sequencing on a MinION Mk1B instrument (ONT) with a Flongle Flow Cell (R9.4.1 chemistry, FLO-FLG001). The MinION instrument was ongoing for approximately 48 hr, or until no further reads could be collected.
Basecalling and demultiplexing were performed using Guppy v6.0.1 (Oxford Nanopore Technologies). The data processing and read classification steps were implemented using the Snakemake workflow management system (Koster and Rahmann, 2012) together with Bioconda (Grüning et al., 2018). For visualization, nanoplot was used (version 1.29.1, https://github.com/wdecoster/NanoPlot/releases). Quality control was done using nanoq (version 0.8.6, https://github.com/esteinig/nanoq). The nanopore data was quality trimmed using Porechop (version 0.2.4, https://github.com/rrwick/porechop). Reads were then filtered by length (0-1 Mb) with Nanofilt (version 2.8.0, https://github.com/wdecoster/nanofilt). We assessed read statistics using NanoStat (version 1.4.0, https://github.com/wdecoster/nanostat) (De Coster et al., 2018). Overall, we obtained 834343 reads, with a mean read quality of 11.7. Otherwise, default settings were used. Taxonomic classification of the consensus sequences was done by kraken2 version 2.1.2 (Wood et al., 2019) and bracken version 2.6.2. (Lu et al., 2017) using a custom database and GTDB v207 (a list of included GCF files and taxonomy can be found in Supplementary material 2). EMU (version 2.0) was used using the original database (Curry et al., 2022).
To include as many of the possible species of Legionella, the ASVs were selected at a higher taxonomic level for the Illumina dataset (at family level Legionellaceae). Phylogenies were estimated based on FastTree 2 (Price et al., 2010). For the Nanopore dataset, the genus Legionella was selected.
In order to compare the co-occurrence of Legionella and include heterotrophic and mixotrophic protists, other phylogenetic groups were excluded from the eukaryotic data set based on knowledge of feeding style (Olenina et al., 2006). The supergroup Archaeplastida were excluded. For the supergroup Opisthokonta, Choanoflagellates were kept as they are filter feeders (Richter and Nitsche, 2017), while the Divison of Metazoa and Fungi were excluded. For the supergroup Stramenopiles, heterotrophic and mixotrophic Chrysophyceae was included since they have phagotrophic feeding style. From the Division Ochrophyta, class Chrysophyceae, Dictyochophyceae and unknown assignment (NA) were kept while non-relevant classes were excluded (Bolidophyceae, Bacillariophyta, MOCH-2, Picozoa, Phaeothamniophyceae, MOCH-5 Raphidophyceae, Synurophyceae, Eustigmatophyceae, Phaeophyceae, and Xanthophyceae). The sequence data was visualized using R package phyloseq (McMurdie and Holmes, 2013) and ggplot2 (Wickham, 2016).
To obtain the protist biomass, microscopic counting and analysis of phytoplankton from the environmental monitoring program was included in this study. Phytoplankton were analyzed microscopically using the Utermöhl method according to the Helcom Combine manual (Utermöhl, 1958; Olenina et al., 2006; HELCOM, 2017). Autotrophic and mixotrophic phytoplankton were identified to the highest possible taxonomic level. Most heterotrophic protists were however not counted. The size of the cells was measured and their biovolume calculated (Olenina et al., 2006). Carbon biomass was calculated using biovolume to carbon biomass conversion factors (Menden-Deuer and Lessard, 2000). This microscopic method is constrained to nano- (2-20 µm) and micro scale (20-200 µm), and thus picoeukaryotes (<2 µm) are not included in the analyses.
To investigate the validity of the classification of the ASVs assigned to Legionella pneumophila by kraken, a multiple sequence alignment and phylogentic tree was created for the environmental ASVs and Legionella spp. The similarity of Legionella spp. 16S rRNA sequences was determined using the Basic Local Alignment Search Tool (BLASTN) at the National Centre for Biotechnological Information (NCBI) and EzTaxon-e server (Kim et al., 2012). The 16S rRNA of the closest hit was retrieved from the IMG-JGI database (Chen et al., 2021). The SBDI Sativa curated 16S GTDB database (version 5) (Lundin and Andersson, 2021) was downloaded and sequences annotated as Legionella were extracted to construct the phylogeny. The extracted sequences were concatenated with sequences selected in this study and the sequence of Francisella tularensis subsp. tularensis SCHU S4 was selected as the outgroup. All concatenated sequences were aligned using MAFFT (Katoh and Standley, 2013) and alignment was trimmed using ClipKIT with the smart-gap option (Steenwyk et al., 2020). The evolutionary relationship was inferred by the Maximum Likelihood method using RAxML-NG (Kozlov et al., 2019) with model GTR+G with Transfer Bootstrap Expectation (TBE) calculation (Lemoine et al., 2018) and visualized using Figtree (version 1.4.4, https://github.com/rambaut/figtree/).
A generalized linear latent variable model (GLLVM) was used to estimate the importance of the environmental factors on the occurrence on Legionella spp. To include as many of the possible species, the ASVs were selected at a higher taxonomic level (at family level Legionellaceae). For accurate frequency distribution of ASVs, the Illumina dataset was used for the model (a more error prone nanopore dataset lead to many unique ASVs). The GLLVM was fitted to the data using the R-package gllvm (Niku et al., 2019). Gaussian distribution function (i.e. identity link) for the responses was selected: environmental variables was included in the model as continuous predictors: humic substances, TDP and temperature. To avoid including highly correlated variables as predictors in the model, a pre-selection step was performed based on the PCA scoreplot (Figure 2), of the environmental variables (i.e. only one of a group of tightly clustered variables was selected, Figure 2). This resulted in a model with fewer parameters that, according to the theory of Occam’s razor, is preferred since they are easier to fit and less prone to convergence issues compared to complex models. For example, temperature and bacterial production were highly correlated (correlation: 0.90), thus bacterial production was excluded from the statistical analyses. In the analysis, it is therefore difficult to disentangle the effect of these variables on the abundance of Legionellaceae. To identify how much of the ASV variation each variable could explain, a separate model was first made for each variable (Table 1). For the selection of the final model, a sensitivity analysis was performed, where the model with the best model of fit and lowest AIC and BIC scores was chosen, i.e. the most optimal trade-off between model fit and complexity (Table 1). Finally, based on the model selection results, humic substances, TDP and temperature were included in the model along with a factor for coastal habitat and a factor for filter volumes (to compensate for when less than 500 ml of water could be filtered).
Figure 2 PCA of the spatial distribution of environmental variables at the stations. The prcomp function was used in the R package stats with default settings, using normalized and scaled data.
Table 1 Variation explained in the Amplicon Sequence Variant (ASV) communities for each environmental variable (according to the ratio of traces).
The continuous variables were normalized so that the mean value and standard deviation were set to zero and one, respectively, for each included predictor. To improve convergence, jitter variance for starting values of latent variables were set to 0.5, and the number of initial runs were set to five. Since additional latent variables did not improve the model, one latent variable was selected to capture the residual variation. Otherwise, default values of the GLLVMs function were used. The identified Legionella occurred at relatively low abundances in the sequence data, constituting of <1% of the entire bacterial community. To manage rare sequence variants and avoiding bias, and to reduce noise in the models, rare sequence variants that only occurred in one sample were excluded in the model.
Primer and probe sequences for the L. pneumophila-specific mip gene target were used (Nazarian et al., 2008). The L. pneumophila specific TaqMan® probe had a 6-carboxy-fluorescein (FAM) as the fluorescent reporter on the 5′ end and a 6-carboxytetramethylrhodamine (TAMRA) as the quencher dye on the 3′ end of the probe (Nazarian et al., 2008). SsoAdvanced Universal Probes Supermix was used (Bio-Rad). To avoid inhibition, 8 µg of BSA was used for each reaction. For each reaction, 1 μL of template DNA was used for a total volume of 20 μL. Thermal cycling, fluorescent data collection, and data analysis were carried out on an iCycler (Bio-Rad Laboratories, Hercules, CA). The thermal cycling profile consisted of an initial incubation at 95°C for 3 min, followed by 45 cycles of 95°C for 5 s and 60°C for 30 s.
Primer targeting a 16S rRNA gene region specific for Legionella were used. For each reaction 1 µl of template DNA was used in a mix of SsoAdvanced Universal Probes Supermix (Bio-Rad), BSA and primer iQLeg1F 5’-CACTGTATGTCAAGGGTAGGTAAG-3’ and iQLeg1R5’-TTAGTGGCGCAGCAAACGCGAT-3’ (this study) for a total volume of 20 µL. The thermal cycling profile consisted of an initial incubation at 95°C for 3 min, followed by 40 cycles of 95°C for 5 s and 60°C for 5 s. Water samples spiked with L. pneumophila concentrations ranging from 10 to 105 colony forming units per mL were analyzed in duplicates to test the limit of detection and generate a standard curve for assessing target DNA concentrations. The standard curve was evaluated with the iQLeg1 primer pair. Positive control mixtures, using DNA from L. pneumophila and negative control mixtures without a template, were included in each PCR run.
Principal component analysis (PCA) of the environmental variables show that the northern parts of the Gulf of Bothnia was characterized by high DOC and humic substances while the southern parts was clearer, had relatively higher salinity and higher pH (Figure 2). In addition, the spatial distribution of environmental variables was larger in the northern parts of the Gulf of Bothnia compared to the south. There were several significant environmental differences between the north and the south: for humic substances, pH, TDN, chlorophyll-a, and salinity (Tables S1, S2 and Supplementary Figure S1).
The concentration of humic substances were highest at station RA1, RA2, and B3 (Figure 3), where the bacterial production were also high compared to the rest of the stations (>8 compared to <6 µgC/(dm3*day)). Nitrogen concentrations were higher in the north (mean north 19.21 +/- 2.65, mean south 15.22 +/- 1.00, p<0.05, Supplementary Figure S1; Tables S1, S2), especially at the coastal stations (RA1: 23.42 µM and RA2: 19.42 µM), followed by the offshore stations in the north (A13: 17.21 µM and A5: 19.28 µM). Phosphorus concentrations were especially low at station A13 (0.18 µM), while station RA1 and B3 had the highest concentrations of phosphorous (>0.4 µM). The temperature were the highest in the coastal northern RA1 and RA2 stations, followed by the southern C24, B3, C14 and C3 stations.
Short amplicon (Illumina) sequence variants assigned to genus Legionella were only detected in the northern samples (RA1, RA2, A13, A5 and B3), (Supplementary Figure S2, top). A total of 2 amplicon sequence variants (ASVs) were identified that were assigned to genus Legionella (Supplementary Figure S3, top). Legionellaceae was however detected in all samples from north to south. In total, 24 ASVs were detected (Supplementary Figure S2, bottom), 14 occurred in more than one sample (Figure 4). Station A5 had the highest relative abundance of ~0.3%, followed by station A13 and B3 with relative abundances of about 0.25% (Supplementary Figure S2, top). The relative abundance in the remaining samples was <0.15%. The relative abundance at A5 was about four times higher compared to the stations with the lowest relative abundance (RA1, C24 and C3).
Figure 4 Filtered Illumina Legionellaceae ASVs, detected in more than one sample (ASVs used in GLLVM).
For the long amplicon sequencing (the nanopore dataset), species were assigned by classification algorithm emu, kraken and bracken. Some differences between the classifiers were observed. For the genus Legionella, 5 species were suggested by EMU (Figure 5, top), where L. pneumophila were the most frequently suggested species (sample A13, A5, B3, GA1 and RA2). According to kraken, 16 species were suggested (Figure 5, middle), where L. waltersii were the most frequently suggested species (sample A13, A5, B3 and RA2). Bracken assigned 20 species (Figure 5, bottom), where L. pneumophila were one of the most frequently suggested species (sample A5, B3, C14 and GA1). Correlations between the two 16S rRNA datasets indicated that an assigned species often correlated strongly with several Illumina ASVs (Figure 6). For the turbid samples RA1, Legionellaceae was not detected by the classifier EMU. Also, the classifier did not detect Legionella in sample C3 and C24. The relative abundance of ASVs assigned to Legionella species was highest at B3 (EMU: ~0.35% of total reads, kraken: ~100 reads out of 48468 and bracken: 0.45% of total reads), about 4-9 times higher relative abundance, according to the different classifiers, compared to the stations with the lowest relative abundance (RA1, C24 and C3). This is supported by qPCR analysis performed targeting a Legionella specific region of the 16S rRNA. Occurrence of Legionella specific DNA was confirmed for all samples and ranged from 2 to 40 bacteria/mL sea water (low versus high abundance). The A5, B3, RA1 and RA2 samples all had Legionella corresponding to >20 bacteria/mL sea water, while samples from C14, C24, C3 and GA1 all had Legionella corresponding to 2 bacteria/mL sea water (Supplementary Figure S4).
Figure 5 Relative abundance of nanopore Amplicon Sequence Variant reads (ASV) of genera Legionella at each station, assigned by classification algorithm EMU (top), kraken (middle) and bracken (bottom). Each sample was sequenced twice, after 30 and 33 PCR cycles.
Figure 6 Correlations (≥0.5) between ASVs in the family Legionellaceae (Illumina) and species classification in the Legionella genus (Nanopore, bracken classification).
The ASVs that were assigned to L. pneumophila by kraken was aligned along with other 16S rRNA sequences for other species of Legionella (Figure 7). Phylogenetic analysis reveals that most sequences are most similar to L. sp011764505 (deposited in NCBI as L. antarctica). Legionella sp. GA1-1 is closest related to Legionella A oakridgensis while Legionella sp. A5-1 and Legionella sp. A5-2 forms a novel branch in the tree without previous characterized species representative. None of the sequences generated in this study were most similar to L. pneumophila. Also, the Legionella specific amplification of the mip gene were negative for all samples (data not shown), indicating that the Legionella species detected in the Gulf of Bothnia samples does not have this exact mip variant that is associated with virulence, and suggest that the detected species aren’t the virulent L. pneumophila.
Figure 7 Phylogenetic relationship of 16S rRNA gene sequences of species of Legionella and ASVs assigned to L. pneumophila by kraken.
The estimated biomass of phagotrophic protozoa using microscopy are presented in Supplementary Figure S5. Station B3 had the largest phytoplankton biomass, over 70 µgC/L (70000 µgC/m3), while station A13, GA1, C3 and C24 had a biomass around 40 µgC/L. Station RA1 and RA2 had the lowest biomass, around 5 µgC/L (station A5 and C14 were not counted). Diatoms were abundant at the A13, B3 and RA1 stations. For the phagotrophic protists, the biomass were higher in the south, where the ciliate Mesodinium rubrum was abundant.
For the genetic method, amplification of the 18S rRNA gene (region V6-V8) indicate that the ciliate Mesodinium rubrum was most relatively abundant compared to other phagotrophic protozoans, except in the RA-stations. At the A-stations and at station B3, Woloszynskia halophila was detected, an organism that had positive co-occurrence with several Legionella ASVs (Supplementary Figures S6, S7). Unlike the 18S V9 region, this longer 18S V6-V8 region were able to assign the majority of the taxa to species level (>70%), which enabled investigation of specific species co-occurrence (Supplementary Figure S7). In general, the co-occurrence varied between Legionella ASVs and phagotrophic protozoa. Legionella ASVs number 5, 6 and 14 had positive co-occurrence with Mesodinium, Protaspa lineage, Botuliforma, and Telonema.
To identify drivers for the occurrence of Legionella spp. we corrected for sampling location while taking dependencies among taxa into account (Figures 2, 6). Included in the model were ASVs that occurred in more than one sample, 14 in total (Figure 4). Significant parameter estimates for each organismal group show that humic substances had a positive effect on the ASV abundance for 6 of the Legionellaceae ASVs (Figure 8). Humic substances were the predictor with the most significant positive associations for Legionella ASVs.
Figure 8 Coefficient estimates by GLLVM analysis showing average effect and uncertainty (as 95% CI) for each organismal group.
The contribution of each independent variable in the model is found in Table 1, where temperature explain 9% of the total variation of the Legionellaceae ASVs. TDP explains 15%, coastal habitat explain 19%, bacterial production 10%, chlorophyll-a explains 11% of the total variation, salinity explain 6% and humic substances explain 3%. The full model explains 48%, including the predictors Humic substances, TDP, Coast, Temperature and Filtered volume. Salinity were not included in the model since it had a strong negative correlation to humic substances (correlation: -0.94). One ASVs abundance were negatively influenced by TDP and one were positively influenced. Four ASVs abundances were negatively influenced by temperature. Five ASV abundances are significant for a coastal habitat, some ASVs were negatively influenced by reduced filtered volumes (when < 500 ml water were filtered, Supplementary Table S3), only one ASV were significantly negatively impacted. Overall, three potential niches were identified. Legionella ASV number 4 and 13 co-occurred. Legionella ASV number 5, 6, 8 and 10 were not strongly co-occurring with other taxa. The remaining Legionella ASVs were strongly positively correlated (Supplementary Figure S8). This remaining subset of Legionella ASVs were positively correlated with humic substances (Figure 8, Supplementary Table S4).
Data on occurrence and persistence of potentially pathogenic bacteria like Legionella spp. in natural waters are missing, which hinders assessment of the risk of exposure. In this study we identified humic substances to be a potential environmental driver for occurrence of Legionella species ASVs (6 out of 14) in the northern Baltic Sea, where the ASVs were more relatively abundant in the humic northern waters. Here, we for the first time present data of the species biodiversity of Legionella from long 16S rRNA sequencing using the Nanopore technologies. This rising technology unravelled opportunities and challenges for describing the occurrence of this public health relevant genus in natural waters.
A previous study identified Legionella in a 10 km coastal area in the Bothnian Sea during June 2018 using short 16S rRNA Illumina sequencing (Eriksson et al., 2022). Here, we identify Legionella both using standard short 16S rRNA Illumina sequencing and by using longer Nanopore sequencing. With Illumina, Legionella was mainly detected in the Bothnian Bay where Legionella was confirmed at all stations. In addition to detection in the Bothnian Bay, Legionella was detected at station B3, confirming the occurrence in this region. Interestingly, at B3 the relative abundance of Legionella ASVs was the highest of all stations, constituting ~0.15% of the reads (Supplementary Figure S2, top). Regarding the ASVs of the family Legionellaceae, these were detected in all samples (Supplementary Figure S2, bottom). Some specific ASVs were mainly detected in the offshore in the north (e.g. ASV no. 14), while others were mainly detected in the southern samples (e.g. ASV no. 6), indicating a spatial variation of Legionella spp. in the Gulf of Bothnia.
Previous studies have suggested temperature as a driver for Legionellales in freshwater (Graells et al., 2018). Generally, for the Gulf of Bothnia, the south-north differences in temperature and the salinity gradient makes the Bothnian Bay more similar to freshwater. A study of bacteria (16S rRNA amplicon sequences) along the salinity gradient of the Baltic Sea argued that the Bothnian Bay is an easier habitat to exploit by species adapted to freshwater compared to more saline water (Herlemann et al., 2011), such as Legionella. This is consistent with our finding of the highest relative abundance of Legionella spp. at the northern stations where the salinity was lower. However, in our model, the relative abundance of only one ASV (no. 5) were significantly correlated to higher temperature. Instead, the occurrence of several ASVs were significantly correlated to lower temperatures (Figure 8). Temperature was thus not found to be a driver for Legionella spp. in this study.
In contrast to our expectation, predators (protozoa) were more abundant in the south of the Gulf of Bothnia (Supplementary Figure S5), where there were less humic substances. It is, however, notable that the total phytoplankton biomass was highest at station B3, where the relative abundance of Legionella spp. were also the highest among the samples (Figure 5, Supplementary Figure S4). Most mixotrophic algae can consume bacteria through phagocytosis (Stoecker et al., 2017). Non-phagotrophic algae such as diatoms have other endocytic mechanisms for ingesting nutrients like iron (Sutak et al., 2020). Interestingly, by taking advantage of the process of endocytosis, it has been shown that bacteria can enter eukaryotic cells (Bonazzi and Cossart, 2006). Since Legionella mainly is an intracellular organism, this observation opens for the hypothesis that Legionella also can use phytoplankton as host. However, the standard monitoring of the Baltic Sea does not include counting of strictly heterotrophic protists, which is limiting the assessment of potential hosts. DOC and predation pressure from unicellular eukaryotes, such as amoebas, together drive the evolution of bacteria. If the amounts of DOC and predators increase, this bacterial resilience to stress may also rise (Fang et al., 2022). Therefore, it can be argued that bacteria that already are resistant to predation might have an ecological advantage.
Another factor that may impact the distribution of Legionella is availability of iron, which has shown to play an important role for the growth, survival and virulence of L. pneumophila. Several studies showed that the growth of this bacterium depends on the presence of iron (Cianciotto, 2015; Portier et al., 2016). Several intracellular bacteria has mechanisms to carefully take up iron without killing their host (Ratledge and Dover, 2000; Wandersman and Delepelaire, 2004; Leon-Sicairos et al., 2015; Yan et al., 2021). Iron has been described as the most valuable metal from a metabolic perspective, since a multitude of enzymes and co-factor require this vital compound (Klebba et al., 2021). Interestingly, there is a correlation between iron sequestration and pathogenicity, and many gram-negative bacterial pathogens have elaborate pathways to acquire iron (Klebba et al., 2021).
Importantly, iron is bound to humic substances and is transported into the ocean together along with the humic substances, providing iron beyond estuaries, especially during spring when the water flow is higher (Herzog et al., 2020). When the pH is lower, iron can be released. In soils, iron has shown to bind humic acids at pH 7 twice as much compared to at pH 5 (Boguta et al., 2019). Humic substances can thus control the biological availability of iron, also in seawater (Laglera and Van Den Berg, 2009). Several studies have investigated the role of humic substances complexed with iron in seawater (Yang et al., 2017; Whitby et al., 2020), but humic substances potential role in the uptake of iron by Legionella in marginal seas is unknown. However, a new iron-regulated gene (IroT/MavN) involved in ferrous iron transport has been identified in Legionella (Cianciotto, 2015; Portier et al., 2015). In a climate change perspective, a further understanding of the microbial degradation of humic substances may be of great importance, especially in humic-rich waters like boreal marginal seas.
Along the gradients of the Gulf of Bothnia, the pH ranged from 7.25 (RA1) to 8.31 (C3). Station RA1 is located near a river mouth (Råne river), and is characterized as an estuarine area (Soerensen et al., 2017). Station B3 is also situated 5 km from a river mouth (Öre river). Of the Legionellaceae, several ASV abundances (6 out of 14) were positively linked to humic substances, perhaps as an indirect effect of iron availability. Since climate change most likely will cause increased precipitation in this region of northern Europe (Meier et al., 2022), freshwater inflows of iron-containing terrestrial humic substances can be expected to rise in the northern Baltic Sea. Potentially, this may lead to promotion of iron-demanding Legionella spp.
The study of particular bacterial species in their natural habitat is challenging due to the limitations with the standard methods used to study these organisms. Therefore, bacteria can most often only be resolved to genus level by using the standard short Illumina amplicon sequencing. For Legionella, only two ASVs were assigned to the genus, all detected in the northern stations (RA1, RA2, A13, A5 and B3, Supplementary Figure S2). These two ASVs are not representative of the whole family tree of Legionellaceae (Supplementary Figure S3). Thus, potential misleading conclusions could easily be drawn when studying this genus based solely on short amplicon Illumina data, since the majority of the diversity is not considered.
To complement the standard Illumina sequencing, we used Nanopore sequencing. The advancement of the Nanopore sequencing technologies are providing new possibilities to sequence longer amplicons (Benítez-Páez et al., 2016; Heikema et al., 2020; Rodríguez-Pérez et al., 2021), which has the potential to classify the bacteria to species level (Johnson et al., 2019; Urban et al., 2021). We performed full-length nanopore sequencing with the aim to resolve the genus of Legionella to species level. To verify the robustness of the technique, we sequenced each sample twice, with 30 and 33 PCR cycles. We used the software EMU that is specifically developed for erogenous ONT sequencing data, since it has been suggested to outcompete other software considering specificity (Curry et al., 2022). In addition, we used classifier kraken and bracken for comparison. Nanopore sequencing resulted in comparable relative abundances to the Illumina dataset for the family Legionellaceae. It therefore seems that the Nanopore sequencing results suggested species for all of the Legionellaceae reads detected using Illumina sequencing (Figure 5, Supplementary Figure S2).
Nanopore sequencing is a promising technique for bacterial species classification. However, current limitations on base accuracy together with limitations in database completeness, limits classification specificity, especially when working on environmental samples with many uncharacterized environmental species (Latorre-Pérez et al., 2021). Therefore, caution is needed when interpreting read classification data based on ONT sequencing. Comparisons between short and long read 16S rRNA classification has shown that there are different biases in the two methods which complicates direct comparison of the results. A question that arise is how to determine if a particular classified ASV from a Nanopore sequencing run belong to an already sequenced species, or if it comes from a non-sequenced relative? For example, Legionellaceae is a diverse family and the majority of environmental variants remain uncultured (Graells et al., 2018). EMU classified all ASVs to species; the level of uncertainty allowed for classification of taxa in environmental samples could therefore be a target for future optimization.
In addition to the more error prone sequences, the pathogenic strain L. pneumophila is well studied and hence overrepresented in databases. Even though L. pneumophila is the most virulent among the Legionellaceae, more than 20 other species of Legionella have shown to cause disease. The remaining strains are considered to be non-pathogens until the opposite has been proven (Legionella Species - Special Pathogens Laboratory, 2020). Thus, future efforts in the sequencing of isolates from the field and patients could aid in reducing this bias.
For non-L. pneumophila strains, cases of disease are estimated to be underrepresented due to the standard testing methods to confirm Legionnaires’ disease and Pontiac fever (Chambers et al., 2021), and alternative methods for detection is debated, often including specific gene amplification to facilitate time consuming culture based methods (Vittal et al., 2022).
By combining short and long 16S rRNA amplicon sequencing to map the distribution of Legionella spp. in the Gulf of Bothnia, this study highlights that there is an environmental niche for several species of Legionella in the Gulf of Bothnia. This study also suggest that these public health relevant bacteria that previously has shown the capability to cause disease such as L. oakridgensis, L. parisiensis, L. longbeachae, L. cherrii and L. anisa (Bibb et al., 1981; Tang et al., 1985; Bornstein et al., 1989; Fang et al., 1989; Lo Presti et al., 1997) can be part of the natural diversity. It is known that pathogenic strains live in natural aquatic environments, and that they are being selected in manmade water systems such as drinking water pipes and cooling towers (Lesnik et al., 2015; Tsao et al., 2019). Therefore, these bacteria could be of importance considering climate change and future water management. Future field studies should thus focus on how species of Legionella are influenced by natural gradients such as humic substances, iron, temperature and salinity over time. Genus specific targets such as the non-Legionella pneumophila target rpoB gene (Pascale et al., 2021) may also be useful to further resolve the pathogenic and non-pathogenic species of Legionella spp. In addition, experimental studies could provide direct evidence of environmental drivers for the occurrence of Legionella, preferably separating the effect of humic substances and salinity.
In conclusion, this study highlights that there is an environmental niche for the occurrence of several species of Legionella in the Gulf of Bothnia, and that pathogenic bacteria can be part of the natural diversity. Partial 16S rRNA sequencing did not capture the diversity within Legionella while the third generation long Nanopore 16S rRNA sequencing was shown promising for bacterial species classification, even though harmonization between classification methods is still needed. Importantly, the Nanopore classification methods had a bias toward the well-studied L. pneumophila, limiting classification of environmental strains. Thus, sequencing environmental isolates could improve the classification using this powerful and rising sequencing method. These bacteria are facultative intracellular, can be pathogenic and have anti-predator strategies. Therefore, knowledge regarding potential hosts for these bacteria such as phagotrophic protozoa could be crucial, since they might provide a niche for emerging pathogens. Currently, taxonomic information of bacteria and heterotrophic protozoa is not included in the environmental monitoring. Here, we demonstrate the significance of sequencing techniques to map public health relevant bacteria such as Legionella spp. in the environment, using a method that can be used to study all bacteria. By applying generalized linear latent variable models (GLLVMs) we show that the occurrence of opportunistic Legionella spp. were linked to high concentrations of humic substances and low salinity. Considering climate change projections in this area, with increased humification and freshwater inflow, this bacterial niche might become more widespread in the future Baltic Sea. Environmental monitoring including sequencing of bacteria and protozoa could thus help identify climate change induced ecosystem imbalances and to appropriately respond to emerging diseases.
The original contributions presented in the study are publicly available. This data can be found here: NCBI, PRJNA901258.
KE and AA conceived the study. AA organized the data collection and KE processed the samples for sequencing. KE, JA and DS processed the data after sequencing. KE and JA conducted the statistical data analyses. KR and AS performed the phylogenetic analysis. AS and LK developed the Nanopore 16S rRNA amplicon workflow. JT organized the qPCR. KE wrote the manuscript together with all co-authors. All authors contributed to the article and approved the submitted version.
This project was financed by the Industrial doctoral school at Umeå University, the marine research environment EcoChange (FORMAS) and the Swedish Ministry of Defence (grant A4001).
We thank Umeå Marine Sciences Centre for chemical analyses, Ingrid Dacklin and Emelie Näslund Salomonsson for help with the sequencing. We thank Stina Bäckman and Mattias Jonsson for the help with the qPCR. Umeå Marine Science Centre is acknowledged for providing data on physicochemical variables and phytoplankton microscopy, via the Swedish National Marine Monitoring Program.
The authors declare that the research was conducted in the absence of any commercial or financial relationships that could be construed as a potential conflict of interest.
All claims expressed in this article are solely those of the authors and do not necessarily represent those of their affiliated organizations, or those of the publisher, the editors and the reviewers. Any product that may be evaluated in this article, or claim that may be made by its manufacturer, is not guaranteed or endorsed by the publisher.
The Supplementary Material for this article can be found online at: https://www.frontiersin.org/articles/10.3389/fmars.2022.1070341/full#supplementary-material
Supplementary data related to this article can be accessed at the Short Read Archive (https://www.ncbi.nlm.nih.gov/sra), project accession: PRJNA, ID: 901258. Supplementary Material 1 contains all associations between ASVs and predictors included in the GLLVM. ASV tables are provided in Supplementary Material 2 and 3.
Amaro F., Martín-González A. (2021). Microbial warfare in the wild–the impact of protists on the evolution and virulence of bacterial pathogens. Int. Microbiol. 24, 559–571. doi: 10.1007/s10123-021-00192-y
Andersson A., Hajdu S., Haecky P., Kuparinen J., Wikner J. (1996). Succession and growth limitation of phytoplankton in the gulf of bothnia (Baltic Sea). Mar. Biol. 126, 791–801. doi: 10.1007/BF00351346
Andersson A., Meier H. E. M., Ripszam M., Rowe O., Wikner J., Haglund P., et al. (2015). Projected future climate change and Baltic Sea ecosystem management. Ambio 44, 345–356. doi: 10.1007/s13280-015-0654-8
Ballard A. L., Fry N. K., Chan L., Surman S. B., Lee J. V., Harrison T. G., et al. (2000). Detection of legionella pneumophila using a real-time PCR hybridization assay. J. Clin. Microbiol. 38, 4215–4218. doi: 10.1128/JCM.38.11.4215-4218.2000
Bandara T., Brugel S., Andersson A., Chun D., Lau P. (2022). Seawater browning alters community composition and reduces nutritional quality of plankton in a subarctic marine ecosystem. Canadian Journal of Fisheries and Aquatic Sciences 79 1–11. doi: 10.1139/CJFAS-2021-0118
Benítez-Páez A., Portune K. J., Sanz Y. (2016). Species-level resolution of 16S rRNA gene amplicons sequenced through the MinIONTM portable nanopore sequencer. Gigascience 5, 4. doi: 10.1186/S13742-016-0111-Z/2720968
Bibb W. F., Sorg R. J., Thomason B. M., Hicklin M. D., Steigerwalt A. G., Brenner D. J., et al. (1981). Recognition of a second serogroup of legionella longbeachae. J. Clin. Microbiol. 14, 674–677. doi: 10.1128/JCM.14.6.674-677.1981
Blenckner T., Österblom H., Larsson P., Andersson A., Elmgren R. (2015). Baltic Sea Ecosystem-based management under climate change: Synthesis and future challenges. Ambio 44, 507–515. doi: 10.1007/s13280-015-0661-9
Boamah D. K., Zhou G., Ensminger A. W., O’Connor T. J. (2017). From many hosts, one accidental pathogen: The diverse protozoan hosts of legionella. Front. Cell. Infect. Microbiol. 7. doi: 10.3389/fcimb.2017.00477
Boguta P., D’Orazio V., Senesi N., Sokołowska Z., Szewczuk-Karpisz K. (2019). Insight into the interaction mechanism of iron ions with soil humic acids. the effect of the pH and chemical properties of humic acids. J. Environ. Manage. 245, 367–374. doi: 10.1016/j.jenvman.2019.05.098
Bolyen E., Rideout J. R., Dillon M. R., Bokulich N. A., Abnet C. C., Al-Ghalith G. A., et al. (2019). Reproducible, interactive, scalable and extensible microbiome data science using QIIME 2. Nat. Biotechnol. 37, 852–857. doi: 10.1038/s41587-019-0209-9
Bonazzi M., Cossart P. (2006). Bacterial entry into cells: A role for the endocytic machinery. FEBS Lett. 580, 2962–2967. doi: 10.1016/j.febslet.2006.04.010
Bornstein N., Mercatello A., Marmet D., Surgot M., Deveaux Y., Fleurette J. (1989). Pleural infection caused by legionella anisa. J. Clin. Microbiol. 27, 2100–2101. doi: 10.1128/JCM.27.9.2100-2101.1989
Brigmon R. L., Turick C. E., Knox A. S., Burckhalter C. E. (2020). The impact of storms on legionella pneumophila in cooling tower water, implications for human health. Front. Microbiol. 11. doi: 10.3389/fmicb.2020.543589
Caporaso J. G., Lauber C. L., Walters W. A., Berg-Lyons D., Huntley J., Fierer N., et al. (2012). Ultra-high-throughput microbial community analysis on the illumina HiSeq and MiSeq platforms. ISME. J. 6, 1621–1624. doi: 10.1038/ismej.2012.8
Carvalho F. R. S., Vazoller R. F., Foronda A. S., Pellizari V. H. (2007). Phylogenetic study of legionella species in pristine and polluted aquatic samples from a tropical Atlantic forest ecosystem. Curr. Microbiol. 55, 288–293. doi: 10.1007/s00284-006-0589-1
Chambers S. T., Slow S., Scott-Thomas A., Murdoch D. R. (2021). Legionellosis caused by non-legionella pneumophila species, with a focus on legionella longbeachae. Microorganisms 9, 291. doi: 10.3390/microorganisms9020291
Chen I.-M. A., Chu K., Palaniappan K., Ratner A., Huang J., Huntemann M., et al. (2021). The IMG/M data management and analysis system v.6.0: new tools and advanced capabilities. Nucleic Acids Res. 49, D751–D763. doi: 10.1093/nar/gkaa939
Cianciotto N. P. (2015). An update on iron acquisition by legionella pneumophila: new pathways for siderophore uptake and ferric iron reduction. Future Microbiol. 10, 841–851. doi: 10.2217/FMB.15.21
Collins S., Jorgensen F., Willis C., Walker J. (2015). Real-time PCR to supplement gold-standard culture-based detection of legionella in environmental samples. J. Appl. Microbiol. 119, 1158–1169. doi: 10.1111/JAM.12911
Curry K. D., Wang Q., Nute M. G., Tyshaieva A., Reeves E., Soriano S., et al. (2022). Emu: species-level microbial community profiling of full-length 16S rRNA Oxford nanopore sequencing data. Nat. Methods 19, 845–853. doi: 10.1038/s41592-022-01520-4
De Coster W., D’Hert S., Schultz D. T., Cruts M., Van Broeckhoven C. (2018). NanoPack: visualizing and processing long-read sequencing data. Bioinformatics 34, 2666–2669. doi: 10.1093/bioinformatics/bty149
Duron O., Doublet P., Vavre F., Bouchon D. (2018). The importance of revisiting legionellales diversity. Trends Parasitol. 34, 1027–1037. doi: 10.1016/J.PT.2018.09.008
Edgcomb V., Orsi W., Bunge J., Jeon S., Christen R., Leslin C., et al. (2011). Protistan microbial observatory in the cariaco basin, caribbean. i. pyrosequencing vs Sanger insights into species richness. ISME. J. 5, 1344–1356. doi: 10.1038/ismej.2011.6
Eriksson K. I. A., Thelaus J., Andersson A., Ahlinder J. (2022). Microbial interactions — underexplored links between public health relevant bacteria and Protozoa in coastal environments. Front. Microbiol. 13. doi: 10.3389/fmicb.2022.877483
Fang W., Lin M., Shi J., Liang Z., Tu X., He Z., et al. (2022). Organic carbon and eukaryotic predation synergistically change resistance and resilience of aquatic microbial communities. Sci. Total. Environ. 830, 154386. doi: 10.1016/j.scitotenv.2022.154386
Fang G. D., Yu V. L., Vickers R. M. (1989). Disease due to the legionellaceae (other than legionella pneumophila): Historical, microbiological, clinical, and epidemiological review. Med. 68, 116–132. doi: 10.1097/00005792-198903000-00005
Graells T., Ishak H., Larsson M., Guy L. (2018). The all-intracellular order legionellales is unexpectedly diverse, globally distributed and lowly abundant. FEMS Microbiol. Ecol. 94, 185. doi: 10.1093/femsec/fiy185
Grüning B., Dale R., Sjödin A., Chapman B. A., Rowe J., Tomkins-Tinch C. H., et al. (2018). Bioconda: sustainable and comprehensive software distribution for the life sciences. Nat. Methods 15, 475–476. doi: 10.1038/s41592-018-0046-7
Guillou L., Bachar D., Audic S., Bass D., Berney C., Bittner L., et al. (2012). The protist ribosomal reference database (PR2): a catalog of unicellular eukaryote small Sub-unit rRNA sequences with curated taxonomy. Nucleic Acids Res. 41, D597–D604. doi: 10.1093/nar/gks1160
Guo J., Brugel S., Andersson A., Lau D. C. P. (2022). Spatiotemporal carbon, nitrogen and phosphorus stoichiometry in planktonic food web in a northern coastal area. Estuar. Coast. Shelf. Sci. 272, 107903. doi: 10.1016/J.ECSS.2022.107903
Hadziavdic K., Lekang K., Lanzen A., Jonassen I., Thompson E. M., Troedsson C. (2014). Characterization of the 18S rRNA gene for designing universal eukaryote specific primers. PloS One 9, e87624. doi: 10.1371/journal.pone.0087624
Hägglund M., Bäckman S., Macellaro A., Lindgren P., Borgmästars E., Jacobsson K., et al. (2018). Accounting for bacterial overlap between raw water communities and contaminating sources improves the accuracy of signature-based microbial source tracking. Front. Microbiol. 9. doi: 10.3389/fmicb.2018.02364
Hamilton K. A., Prussin A. J., Ahmed W., Haas C. N. (2018). Outbreaks of legionnaires’ disease and pontiac fever 2006–2017. Curr. Environ. Heal. Rep. 5, 263–271. doi: 10.1007/s40572-018-0201-4
Harvey E. T., Kratzer S., Andersson A. (2015). Relationships between colored dissolved organic matter and dissolved organic carbon in different coastal gradients of the Baltic Sea. Ambio 44, 392–401. doi: 10.1007/s13280-015-0658-4
Heikema A. P., Horst-Kreft D., Boers S. A., Jansen R., Hiltemann S. D., de Koning W., et al. (2020). Comparison of illumina versus nanopore 16S rRNA gene sequencing of the human nasal microbiota. Genes 11, 1105. doi: 10.3390/GENES11091105
Herlemann D. P. R., Labrenz M., Jürgens K., Bertilsson S., Waniek J. J., Andersson A. F. (2011). Transitions in bacterial communities along the 2000 km salinity gradient of the Baltic Sea. ISME. J. 5, 1571–1579. doi: 10.1038/ismej.2011.41
Herzog S. D., Persson P., Kvashnina K., Kritzberg E. S. (2020). Organic iron complexes enhance iron transport capacity along estuarine salinity gradients of Baltic estuaries. Biogeosciences 17, 331–344. doi: 10.5194/bg-17-331-2020
Holsinger H., Tucker N., Regli S., Studer K., Roberts V. A., Collier S., et al. (2022). Characterization of reported legionellosis outbreaks associated with buildings served by public drinking water systems: United states 2001–2017. J. Water Health 20, 702–711. doi: 10.2166/WH.2022.002
Johnson J. S., Spakowicz D. J., Hong B.-Y., Petersen L. M., Demkowicz P., Chen L., et al. (2019). Evaluation of 16S rRNA gene sequencing for species and strain-level microbiome analysis. Nat. Commun. 10, 5029. doi: 10.1038/s41467-019-13036-1
Katoh K., Standley D. M. (2013). MAFFT multiple sequence alignment software version 7: Improvements in performance and usability. Mol. Biol. Evol. 30, 772–780. doi: 10.1093/molbev/mst010
Kim O.-S., Cho Y.-J., Lee K., Yoon S.-H., Kim M., Na H., et al. (2012). Introducing EzTaxon-e: a prokaryotic 16S rRNA gene sequence database with phylotypes that represent uncultured species. Int. J. Syst. Evol. Microbiol. 62, 716–721. doi: 10.1099/ijs.0.038075-0
Klebba P. E., Newton S. M. C., Six D. A., Kumar A., Yang T., Nairn B. L., et al. (2021). Iron acquisition systems of gram-negative bacterial pathogens define TonB-dependent pathways to novel antibiotics. Chem. Rev. 121, 5193–5239. doi: 10.1021/acs.chemrev.0c01005
Koster J., Rahmann S. (2012). Snakemake–a scalable bioinformatics workflow engine. Bioinformatics 28, 2520–2522. doi: 10.1093/bioinformatics/bts480
Kozlov A. M., Darriba D., Flouri T., Morel B., Stamatakis A. (2019). RAxML-NG: a fast, scalable and user-friendly tool for maximum likelihood phylogenetic inference. Bioinformatics 35, 4453–4455. doi: 10.1093/bioinformatics/btz305
Laglera L. M., Van Den Berg C. M. G. (2009). Evidence for geochemical control of iron by humic substances in seawater. Limnol. Oceanogr. 54, 610–619. doi: 10.4319/LO.2009.54.2.0610
Lamb J. B., van de Water J. A. J. M., Bourne D. G., Altier C., Hein M. Y., Fiorenza E. A., et al. (2017). Seagrass ecosystems reduce exposure to bacterial pathogens of humans, fishes, and invertebrates. Science 355, 731–733. doi: 10.1126/science.aal1956
Latorre-Pérez A., Gimeno-Valero H., Tanner K., Pascual J., Vilanova C., Porcar M. (2021). A Round Trip to the Desert: In situ Nanopore Sequencing Informs Targeted Bioprospecting. Front. Microbiol. 12, 3777. doi: 10.3389/fmicb.2021.768240
Latz M. A. C., Grujcic V., Brugel S., Lycken J., John U., Karlson B., et al. (2022). Short- and long-read metabarcoding of the eukaryotic rRNA operon: evaluation of primers and comparison to shotgun metagenomics sequencing. Mol. Ecol. Resour 22 (6):2304–2318. doi: 10.1111/1755-0998.13623
Legionella Species - Special Pathogens Laboratory (2020). Available at: https://specialpathogenslab.com/legionella-species/ (Accessed September 2, 2022).
Lemoine F., Domelevo Entfellner J.-B., Wilkinson E., Correia D., Dávila Felipe M., De Oliveira T., et al. (2018). Renewing felsenstein’s phylogenetic bootstrap in the era of big data. Nature 556, 452–456. doi: 10.1038/s41586-018-0043-0
Leoni E., Catalani F., Marini S., Dallolio L. (2018). Legionellosis associated with recreational waters: A systematic review of cases and outbreaks in swimming pools, spa pools, and similar environments. Int. J. Environ. Res. Public Heal. 15, 1612. doi: 10.3390/IJERPH15081612
Leon-Sicairos N., Reyes-Cortes R., Guadrón-Llanos A. M., Madueña-Molina J., Leon-Sicairos C., Canizalez-Román A. (2015). Strategies of intracellular pathogens for obtaining iron from the environment. BioMed. Res. Int. 2015, 1–17. doi: 10.1155/2015/476534
Lesnik R., Brettar I., Höfle M. G. (2015). Legionella species diversity and dynamics from surface reservoir to tap water: from cold adaptation to thermophily. ISME. J. 10, 1064–1080. doi: 10.1038/ismej.2015.199
Lo Presti F., Riffard S., Vandenesch F., Reyrolle M., Ronco E., Ichai P., et al. (1997). The first clinical isolate of legionella parisiensis, from a liver transplant patient with pneumonia. J. Clin. Microbiol. 35, 1706–1709. doi: 10.1128/JCM.35.7.1706-1709.1997
Lu J., Breitwieser F. P., Thielen P., Salzberg S. L. (2017). Bracken: estimating species abundance in metagenomics data. PeerJ. Comput. Sci. 3, e104. doi: 10.7717/peerj-cs.104
Lundin D., Andersson A. (2021). SBDI sativa curated 16S GTDB database. SciLifeLab Dataset doi: 10.17044/scilifelab.14869077.v5
McDonald D., Price M. N., Goodrich J., Nawrocki E. P., DeSantis T. Z., Probst A., et al. (2012). An improved greengenes taxonomy with explicit ranks for ecological and evolutionary analyses of bacteria and archaea. ISME. J. 6, 610–618. doi: 10.1038/ismej.2011.139
McMurdie P. J., Holmes S. (2013). Phyloseq: An r package for reproducible interactive analysis and graphics of microbiome census data. PloS One 8, e61217. doi: 10.1371/journal.pone.0061217
Meier H. E. M., Kniebusch M., Dieterich C., Gröger M., Zorita E., Elmgren R., et al. (2022). Climate change in the Baltic Sea region: a summary. Earth Syst. Dyn. 13, 457–593. doi: 10.5194/esd-13-457-2022
Menden-Deuer S., Lessard E. J. (2000). Carbon to volume relationships for dinoflagellates, diatoms, and other protist plankton. Limnol. Oceanogr. 45, 569–579. doi: 10.4319/lo.2000.45.3.0569
Muder R. R., Yu V. L. (2002). Infection due to Legionella species other than L. pneumophila. Clin. Infect. Dis. 35, 990–998. doi: 10.1086/342884
Nazarian E. J., Bopp D. J., Saylors A., Limberger R. J., Musser K. A. (2008). Design and implementation of a protocol for the detection of legionella in clinical and environmental samples. Diagn. Microbiol. Infect. Dis. 62, 125–132. doi: 10.1016/j.diagmicrobio.2008.05.004
Niku J., Hui F. K. C., Taskinen S., Warton D. I. (2019). Gllvm: Fast analysis of multivariate abundance data with generalized linear latent variable models in r. Methods Ecol. Evol. 10, 2173–2182. doi: 10.1111/2041-210X.13303
Olenina I., Hajdu S., Edler L., Andersson A., Wasmund N., Busch S., et al. (2006). Biovolumes and size-classes of phytoplankton in the Baltic Sea HELCOM. Balt. Sea Env. Proc. 106, 1–44.
Opel K. L., Chung D., McCord B. R. (2010). A study of PCR inhibition mechanisms using real time PCR. J. Forensic. Sci. 55, 25–33. doi: 10.1111/j.1556-4029.2009.01245.x
Park J. M., Ghosh S., O’Connor T. J. (2020). Combinatorial selection in amoebal hosts drives the evolution of the human pathogen legionella pneumophila. Nat. Microbiol. 5, 599–609. doi: 10.1038/s41564-019-0663-7
Parte A. C., Sardà Carbasse J., Meier-Kolthoff J. P., Reimer L. C., Göker M. (2020). List of prokaryotic names with standing in nomenclature (LPSN) moves to the DSMZ. Int. J. Syst. Evol. Microbiol. 70, 5607–5612. doi: 10.1099/ijsem.0.004332
Parthuisot N., West N. J., Lebaron P., Baudart J. (2010). High diversity and abundance of legionella spp. in a pristine river and impact of seasonal and anthropogenic effects. Appl. Environ. Microbiol. 76, 8201–8210. doi: 10.1128/AEM.00188-10
Pascale M. R., Salaris S., Mazzotta M., Girolamini L., Fregni Serpini G., Manni L., et al. (2021). New insight regarding legionella non- pneumophila species identification: Comparison between the traditional mip gene classification scheme and a newly proposed scheme targeting the rpoB gene. Microbiol. Spectr. 9, e01161-21. doi: 10.1128/Spectrum.01161-21
Portier E., Bertaux J., Labanowski J., Hechard Y. (2016). Iron availability modulates the persistence of Legionella pneumophila in complex biofilms. Microbes Environ. 31, 387–394. doi: 10.1264/jsme2.ME16010
Portier E., Zheng H., Sahr T., Burnside D. M., Mallama C., Buchrieser C., et al. (2015). IroT/mavN , a new iron-regulated gene involved in l egionella pneumophila virulence against amoebae and macrophages. Environ. Microbiol. 17, 1338–1350. doi: 10.1111/1462-2920.12604
Price M. N., Dehal P. S., Arkin A. P. (2010). FastTree 2 - approximately maximum-likelihood trees for large alignments. PloS One 5, e9490. doi: 10.1371/journal.pone.0009490
Ratledge C., Dover L. G. (2000). Iron metabolism in pathogenic bacteria. Annu. Rev. Microbiol. 54, 881–941. doi: 10.1146/annurev.micro.54.1.881
Renaud G., Stenzel U., Maricic T., Wiebe V., Kelso J. (2015). deML: robust demultiplexing of illumina sequences using a likelihood-based approach. Bioinformatics 31, 770–772. doi: 10.1093/bioinformatics/btu719
Richter D. J., Nitsche F. (2017). “Choanoflagellatea,” in Handbook of the protists: Second edition (Cham: Springer International Publishing), 1479–1496. doi: 10.1007/978-3-319-28149-0_5
Rodríguez-Pérez H., Ciuffreda L., Flores C. (2021). NanoCLUST: a species-level analysis of 16S rRNA nanopore sequencing data. Bioinformatics 37, 1600–1601. doi: 10.1093/bioinformatics/btaa900
SHARKdata - Datasets (2022). Available at: https://sharkdata.smhi.se/ (Accessed October 11, 2022).
Shimada S., Nakai R., Aoki K., Shimoeda N., Ohno G., Kudoh S., et al. (2021). Chasing waterborne pathogens in Antarctic human-made and natural environments, with special reference to legionella spp. Appl. Environ. Microbiol. 87, 1–15. doi: 10.1128/AEM.02247-20
Sidstedt M., Rådström P., Hedman J. (2020). PCR inhibition in qPCR, dPCR and MPS–mechanisms and solutions. Anal. Bioanal. Chem. 412, 2009–2023. doi: 10.1007/s00216-020-02490-2
Soerensen A. L., Schartup A. T., Skrobonja A., Björn E. (2017). Organic matter drives high interannual variability in methylmercury concentrations in a subarctic coastal sea. Environ. pollut. 229, 531–538. doi: 10.1016/j.envpol.2017.06.008
Steenwyk J. L., Buida T. J., Li Y., Shen X.-X., Rokas A. (2020). ClipKIT: A multiple sequence alignment trimming software for accurate phylogenomic inference. PloS Biol. 18, e3001007. doi: 10.1371/journal.pbio.3001007
Stoecker D. K., Hansen P. J., Caron D. A., Mitra A. (2017). Mixotrophy in the marine plankton. Ann. Rev. Mar. Sci. 9, 311–335. doi: 10.1146/annurev-marine-010816-060617
Sun S., Noorian P., McDougald D. (2018). Dual role of mechanisms involved in resistance to predation by Protozoa and virulence to humans. Front. Microbiol. 9 1017. doi: 10.3389/fmicb.2018.01017
Sutak R., Camadro J.-M., Lesuisse E. (2020). Iron uptake mechanisms in marine phytoplankton. Front. Microbiol. 11. doi: 10.3389/fmicb.2020.566691
Tamminen T., Andersen T. (2007). Seasonal phytoplankton nutrient limitation patterns as revealed by bioassays over Baltic Sea gradients of salinity and eutrophication. Mar. Ecol. Prog. Ser. 340, 121–138. doi: 10.3354/MEPS340121
Tang P. W., Toma S., MacMillan L. G. (1985). Legionella oakridgensis: laboratory diagnosis of a human infection. J. Clin. Microbiol. 21, 462–463. doi: 10.1128/JCM.21.3.462-463.1985
Thomas V., McDonnell G., Denyer S. P., Maillard J.-Y. (2010). Free-living amoebae and their intracellular pathogenic microorganisms: risks for water quality. FEMS Microbiol. Rev. 34, 231–259. doi: 10.1111/j.1574-6976.2009.00190.x
Tsao H. F., Scheikl U., Herbold C., Indra A., Walochnik J., Horn M. (2019). The cooling tower water microbiota: Seasonal dynamics and co-occurrence of bacterial and protist phylotypes. Water Res. 159, 464–479. doi: 10.1016/j.watres.2019.04.028
Urban L., Holzer A., Baronas J. J., Hall M. B., Braeuninger-Weimer P., Scherm M. J., et al. (2021). Freshwater monitoring by nanopore sequencing. Elife 10, 1–27. doi: 10.7554/eLife.61504
Utermöhl H. (1958). Zur vervollkommnung der quantitativen phytoplankton-methodik. SIL. Commun. 9, 1–38. doi: 10.1080/05384680.1958.11904091
Vittal R., Roshini J., Raj M., Kumar B. K., Karunasagar I. (2022). Advances in environmental detection and clinical diagnostic tests for legionella species. J. Heal. Allied Sci. NU. 12, 168–174. doi: 10.1055/S-0041-1731863
Wandersman C., Delepelaire P. (2004). Bacterial iron sources: From siderophores to hemophores. Annu. Rev. Microbiol. 58, 611–647. doi: 10.1146/annurev.micro.58.030603.123811
Whitby H., Planquette H., Cassar N., Bucciarelli E., Osburn C. L., Janssen D. J., et al. (2020). A call for refining the role of humic-like substances in the oceanic iron cycle. Sci. Rep. 2020. 101. 10, 1–12. doi: 10.1038/s41598-020-62266-7
Wickham H. (2016). ggplot2: Elegant graphics for data analysis (Cham: Springer International Publishing). doi: 10.1007/978-3-319-24277-4
Wood D. E., Lu J., Langmead B. (2019). Improved metagenomic analysis with kraken 2. Genome Biol. 20, 257. doi: 10.1186/s13059-019-1891-0
Yang R., Su H., Qu S., Wang X. (2017). Capacity of humic substances to complex with iron at different salinities in the Yangtze river estuary and East China Sea. Sci. Rep. 7, 1–9. doi: 10.1038/s41598-017-01533-6
Yan Q., Zhang W., Lin M., Teymournejad O., Budachetri K., Lakritz J., et al. (2021). Iron robbery by intracellular pathogen via bacterial effector–induced ferritinophagy. Proc. Natl. Acad. Sci. 118, e2026598118. doi: 10.1073/pnas.2026598118
Keywords: Legionella, protozoa, predation resistance, aquatic microbiology, climate change, ecology change, marginal seas, humification
Citation: Eriksson KIA, Ahlinder J, Ramasamy KP, Andersson A, Sundell D, Karlsson L, Sjödin A and Thelaus J (2023) Association between Legionella species and humic substances during early summer in the northern Baltic Sea. Front. Mar. Sci. 9:1070341. doi: 10.3389/fmars.2022.1070341
Received: 14 October 2022; Accepted: 27 December 2022;
Published: 23 January 2023.
Edited by:
Xianghui Guo, Xiamen University, ChinaReviewed by:
Antonio Busquets Bisbal, University of the Balearic Islands, SpainCopyright © 2023 Eriksson, Ahlinder, Ramasamy, Andersson, Sundell, Karlsson, Sjödin and Thelaus. This is an open-access article distributed under the terms of the Creative Commons Attribution License (CC BY). The use, distribution or reproduction in other forums is permitted, provided the original author(s) and the copyright owner(s) are credited and that the original publication in this journal is cited, in accordance with accepted academic practice. No use, distribution or reproduction is permitted which does not comply with these terms.
*Correspondence: Karolina Ida Anna Eriksson, a2Fyb2xpbmEuZXJpa3Nzb25AdW11LnNl
Disclaimer: All claims expressed in this article are solely those of the authors and do not necessarily represent those of their affiliated organizations, or those of the publisher, the editors and the reviewers. Any product that may be evaluated in this article or claim that may be made by its manufacturer is not guaranteed or endorsed by the publisher.
Research integrity at Frontiers
Learn more about the work of our research integrity team to safeguard the quality of each article we publish.