- 1Department of Orthopedics, China-Japan Union Hospital of Jilin University, Changchun, China
- 2Department of Dermatology, The First Hospital of Jilin University, Changchun, China
- 3Department of Neurology, Center for Neuroscience, The First Hospital of Jilin University, Changchun, China
Objectives: There is evidence from observational studies that human microbiota is linked to skin appendage Disorders (SADs). Nevertheless, the causal association between microbiota and SADs is yet to be fully clarified.
Methods: A comprehensive two-sample Mendelian randomization (MR) was first performed to determine the causal effect of skin and gut microbiota on SADs. A total of 294 skin taxa and 211 gut taxa based on phylum, class, order, family, genus, and ASV level information were identified. Summary data of SADs and eight subtypes (acne vulgaris, hidradenitis suppurativa, alopecia areata, rogenic alopecia, rosacea, rhinophyma, seborrhoeic dermatitis, and pilonidal cyst) were obtained from the FinnGen consortium. We performed bidirectional MR to determine whether the skin and gut microbiota are causally associated with multiple SADs. Furthermore, sensitivity analysis was conducted to examine horizontal pleiotropy and heterogeneity.
Results: A total of 65 and 161 causal relationships between genetic liability in the skin and gut microbiota with SADs were identified, respectively. Among these, we separately found 5 and 11 strong causal associations that passed Bonferroni correction in the skin and gut microbiota with SADs. Several skin bacteria, such as Staphylococcus, Streptococcus, and Propionibacterium, were considered associated with multiple SADs. As gut probiotics, Bifidobacteria and Lactobacilli were associated with a protective effect on SAD risk. There was no significant heterogeneity in instrumental variables or horizontal pleiotropy.
Conclusions: Our MR analysis unveiled bidirectional causal relationships between SADs and the gut and skin microbiota, and had the potential to offer novel perspectives on the mechanistic of microbiota-facilitated dermatosis.
Introduction
As the largest organ of the body, the skin functions as our primary barrier against external threats. However, it also serves as a diverse environment for a multitude of microorganisms, whose interactions significantly contribute to the skin’s overall health, immune response, and disease development (1). Skin appendages, including sweat glands, hair follicles, sebaceous glands, and arrector pili muscles, are found in the dermis and adipose tissue. The surface and appendages of the skin are colonized by the skin microbiota, whose composition is contingent upon the microenvironment (2). Disturbances in the balance of the skin microflora, known as dysbiosis, have been recognized as contributing factors in various dermatological conditions, especially skin appendage disorders (SADs) (3, 4).
Meanwhile, the gut, home to the densest microbial population within the human body, exerts effects that extend beyond its confines. Gut microorganisms are primarily recognized for their roles in metabolic processes and immune system development (5). Interestingly, their influence transcends the gut, affecting various physiological systems, including the skin (6). This influential link between the gut and skin, termed the gut-skin axis, is emerging as an integral component in skin health and disease (6, 7). Despite these advances, the concurrent role of gut and skin microbiota in SADs remains an intriguingly uncharted area of research. Additionally, without experimental methods that rely on cultivated isolates, it is challenging to determine causality due to the close relationship between the microbiota and its host (8, 9).
Mendelian randomization (MR) is a powerful epidemiological technique that indicates causal associations by utilizing genetic variations (10). MR is inherently not confounding since environmental and self-adapted variables have no effect on genetic differences, as these are randomly allocated at conception. Moreover, this approach can circumvent the issue of reverse causality, as germline genotypes remain unaltered by physiological disturbances resulting from disease. Our study embarks on an exploration using comprehensive bidirectional MR to unravel potential causal relationships between the skin and gut microbiota and multiple SADs (acne vulgaris, hidradenitis suppurativa, alopecia areata, androgenic alopecia, rosacea, rhinophyma, seborrheic dermatitis, and pilonidal cyst). We aim to offer insights into the possible involvement of these microbial communities in the pathogenesis and progression of SADs, and pave the way for microbiome-oriented therapeutic strategies.
Methods
Data sources
Genetic variations of skin microbiota were derived from the GWAS conducted by Moitinho-Silva et al. (11). A sum of 1656 skin samples was acquired from individuals within two German cohorts, KORA FF4 (n = 635) and PopGen (n=1021). The samples were collected from three skin microenvironments, including moist skin (antecubital fossa in both cohorts), dry skin (dorsal and volar forearm in PopGen), and sebaceous skin (forehead in PopGen and retroauricular fold in KORA FF4). Microbial community patterns were obtained through the 16 S rRNA gene. Amplicon sequence variants (ASVs) and taxonomic groups from genus to phylum level were utilized in the GWAS. In total, 294 taxa in both cohorts were included in the analysis (14 phyla, 22 classes, 24 orders, 30 families, 54 genera, and 150 ASVs).
SNPs associated with the composition of the gut microbiota were selected as instrumental variables (IVs) within a GWAS database belonging to the MiBio-Gen consortium (12). This large-scale multi-ethnic GWAS integrated 16S rRNA gene sequencing data from 18,340 individuals across 24 cohorts to investigate the link between human autosomal genetic variants and the intestinal microbiota. There were 211 taxa in all, including 9 phyla, 16 classes, 20 orders, 35 families, and 131 genera.
GWAS summary data for SADs (377,277 individuals), acne vulgaris (AV) (363,927 individuals), hidradenitis suppurativa (HS) (362,071 individuals), alopecia areata (AA) (361,822 individuals), androgenic alopecia (AGA) (201,214 individuals), rosacea (ROS) (363,350 individuals), rhinophyma (RPH) (361,275 individuals), seborrhoeic dermatitis (SD) (339,277 individuals), and pilonidal cyst (PC) (358,708 individuals) were acquired from the R9 release of FinnGen consortium (13). Comprehensive information regarding the encompassed cohorts, genotypic data, endpoint specifications, and association testing can be accessed through the FinnGen webpage.
Instrumental variable selection
We applied the following criteria to select the instrumental variables (IVs): (1) potential IVs were identified as single nucleotide polymorphisms (SNPs) at the locus-wide significance threshold (P < 1.0×10–5) that was widely utilized in the previous MR studies (14–17); (2) a linkage disequilibrium parameter (R2) of SNP was set at 0.01, with a genetic distance of 10,000 kb; (3) A minor allele frequency (MAF) of less than 0.01 was used to eliminate SNPs; (4) palindromic SNPs were discarded to guarantee that the allelic effects of SNPs during the harmonization process; and (5) IVs with an F statistic <10 were excluded.
Mendelian randomization analysis
The MR study was structured as depicted in Figure 1. We applied five MR methods for features with multiple IVs: inverse-variance weighted (IVW) (18), weighted median (19), MR-Egger regression (20), simple mode (21), and weighted mode (22). The IVW method has been shown to have more power than the others under some conditions (22); hence, we mainly used the IVW method for the results, and the other four methods as supplements. For a more stringent interpretation of the causal relationship, we performed a Bonferroni correction, based on the number of bacteria within each level. For skin microbiota, the threshold significance was set as follows: phylum P = 7.14 × 10−3 (0.05/7), class P = 4.55 × 10−3 (0.05/11), order P = 4.17 × 10−3 (0.05/12), family P = 3.33 × 10−3 (0.05/15), genus P= 1.85 × 10−3 (0.05/27), ASV P= 6.67 × 10−4 (0.05/75). For gut microbiota, the threshold significance was set as follows: phylum P = 5.56 × 10−3 (0.05/9), class P = 3.13 × 10−3 (0.05/16), order P = 2.50 × 10−3 (0.05/20), family P = 1.43 × 10−3 (0.05/35), genus P= 3.82 × 10−3 (0.05/131). P-values falling within the range between 0.05 and the corrected value were regarded as nominal significance with potential causal effects. To investigate whether SADs exerted any causal influence on the identified skin and gut microbiota, we also conducted a reverse MR analysis. The methodologies and settings employed were in line with those of the forward MR. Two-sample MR (version 0.5.6) and MRPRESSO (version 1.0) packages with R software (version 4.2.2) were used. The MR study was conducted in accordance with STROBE-MR guidelines (23, 24).
Sensitivity analysis
To evaluate the heterogeneity of instrumental variables, we employed Cochran’s Q statistics (25). Additionally, a leave-one-out (LOO) analysis was conducted to assess the influence of individual SNPs on the overall causal estimate (26). By systematically excluding each SNP from the analysis, we evaluated the robustness of our results. Consistent results across LOO analyses enhance confidence in the causal inference, indicating that the findings are not driven by specific SNPs (26). We also used MR-Egger intercept tests and MR-PRESSO to verify the existence of horizontal pleiotropy. MR-PRESSO performs a global test to detect the presence of horizontal pleiotropy by comparing the observed distribution of SNP-exposure and SNP-outcome associations with their expected distribution under no pleiotropy (27). A significance (P < 0.05) indicates a substantial influence of pleiotropic SNPs on the original causal estimate. In MR-Egger regression, the intercept term provides an estimate of the average pleiotropic effect across all SNPs (28). A non-zero intercept indicates the presence of directional pleiotropy. To further confirm if the observed causalities were skewed due to reversed causation, the Steiger directionality test was applied (29). If the SNPs explain more variance in the exposure than in the outcome, it supports the correct direction of causality (27). Confirming the direction of effect reduces the risk of reverse causation bias, where the outcome could mistakenly appear to cause the exposure (27). Given the distinctiveness of skin microbiota across different microenvironments (moist, dry, and sebaceous areas) in KORA FF4 and PopGen cohorts, meta-analyses were carried out by combining data sets originating from the same microenvironment. Statistical analyses were applied using the META (version 6.5.0) package.
Results
SNP selection
Following the quality control procedures, a total of 838 SNPs and 1031 SNPs in the forward MR analysis were selected as IVs from skin and gut microbiota, respectively (Supplementary Tables S1, S2). In the reverse MR analysis, a total of 3316 SNPs and 3259 SNPs were severally selected from SADs as IVs for skin and gut microbiota (Supplementary Tables S3, S4). The F statistics of the remaining IVs were all greater than 10, which suggests that there was less chance of weak instrument bias affecting the estimates. Detailed information on the major IVs in the MR analysis that passed the Bonferroni correction between SADs and skin and gut microbiota was displayed in Supplementary Table S5.
Causal associations of skin and gut microbiota and SADs
In the forward MR analysis, 115 causal associations were found between SADs and skin microbiota of the KORA FF4 and PopGen cohorts (Supplementary Table S6). After the microenvironment-based meta-analysis of two cohorts, 30 causal associations remained significant (P < 0.05) in the moist, dry, or sebaceous skin (Figures 2, 3). As for the gut, 83 causal associations were found between SADs and gut microbiota (Figures 4, 5). Details about the causalities between skin and gut microbiota and multiple SADs are shown below.
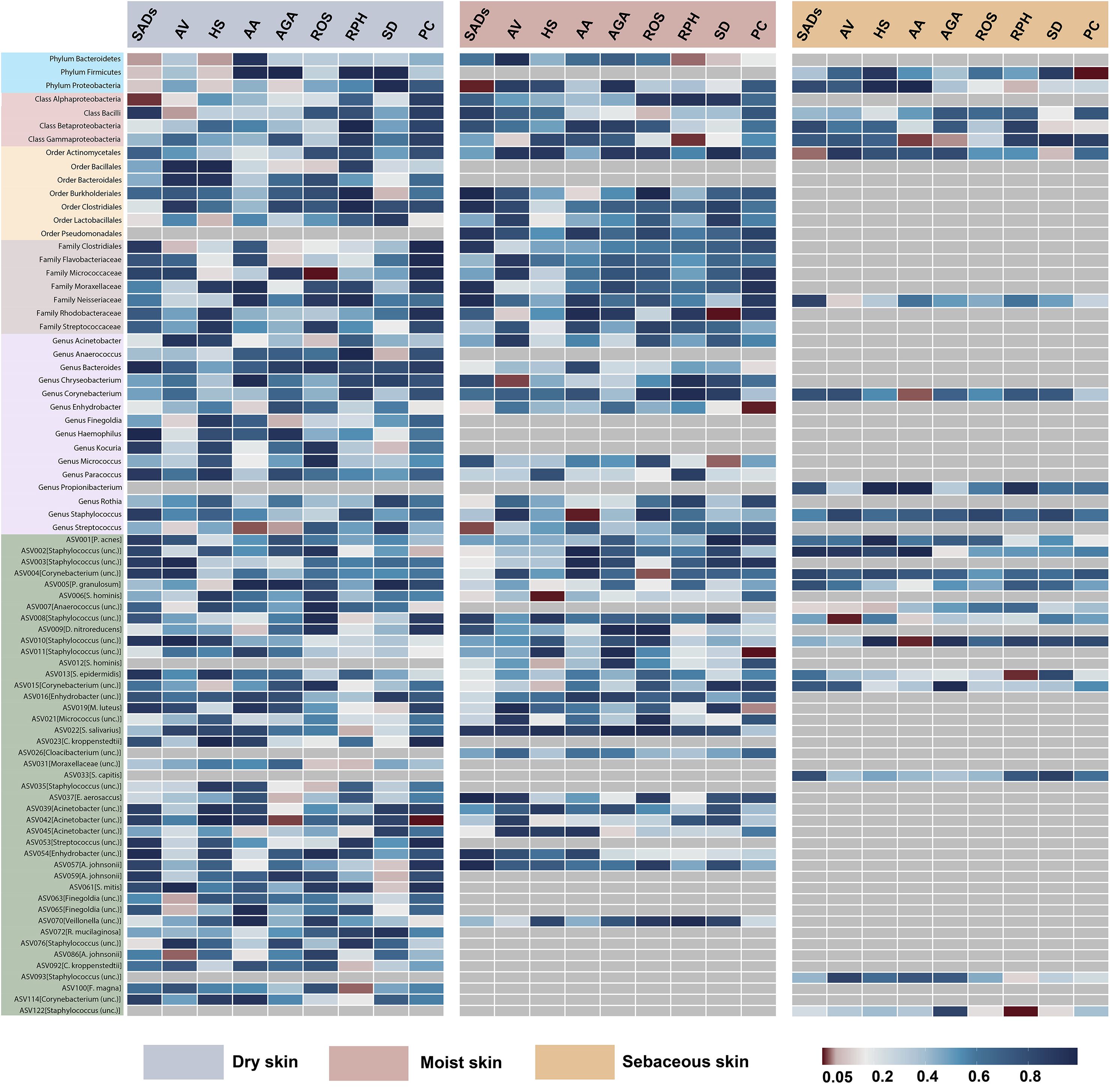
Figure 2. Heatmap showing causal associations between skin microbiota and SADs. SADs, skin appendage Disorders; AV, acne vulgaris; HS, hidradenitis suppurativa; AA, alopecia areata; AGA, androgenic alopecia; ROS, rosacea; RPH, rhinophyma; SD, seborrhoeic dermatitis; and PC, pilonidal cyst.
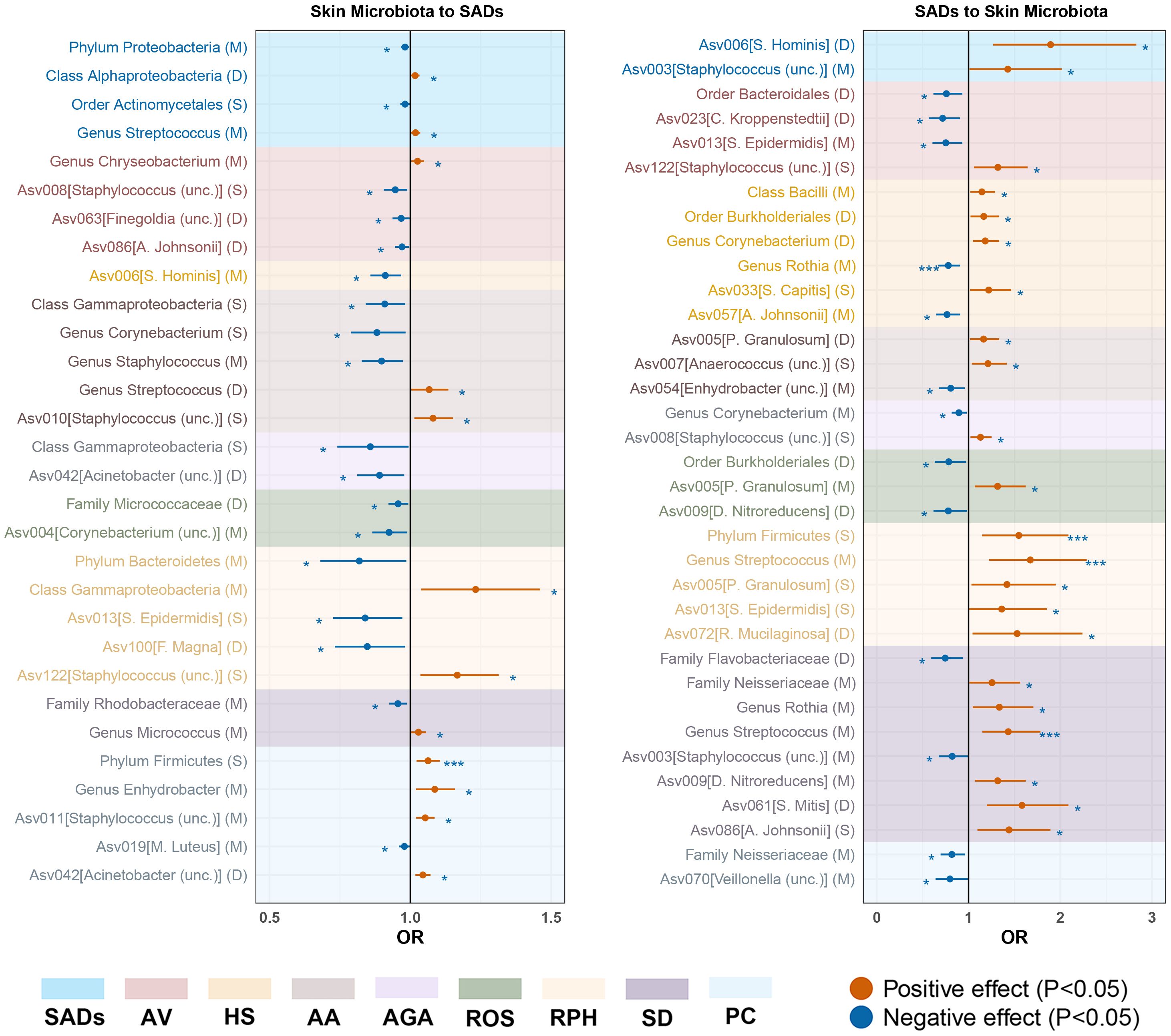
Figure 3. Forest plot of significant bidirectional causalities between skin microbiota and SADs. M, moist; D, dry; S, sebaceous; SADs, skin appendage Disorders; AV, acne vulgaris; HS, hidradenitis suppurativa; AA, alopecia areata; AGA, androgenic alopecia; ROS, rosacea; RPH, rhinophyma; SD, seborrhoeic dermatitis; and PC, pilonidal cyst. * and *** represent nominal causalities and strong causal associations, respectively.
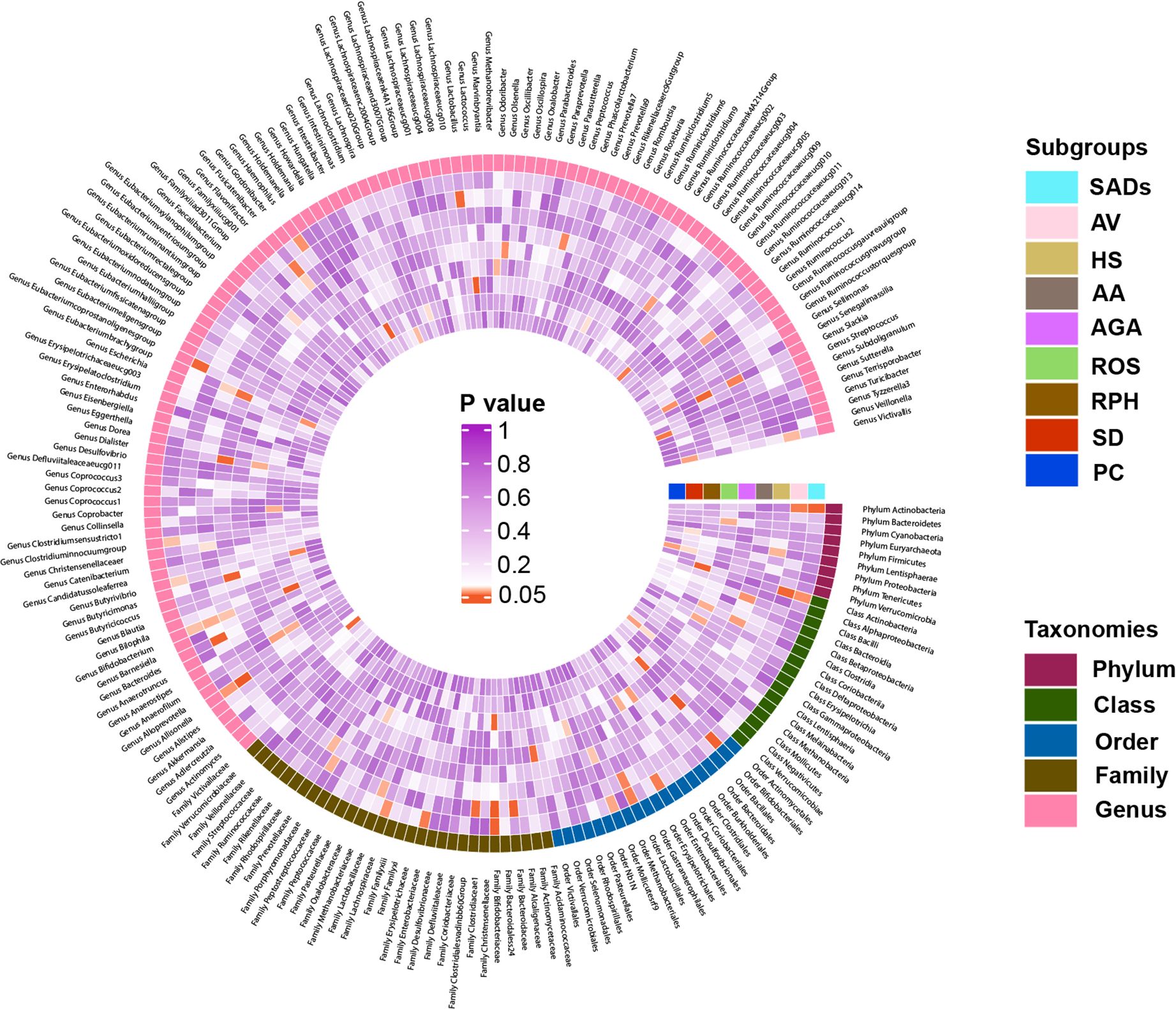
Figure 4. Heatmap showing causal associations between gut microbiota and SADs. SADs, skin appendage Disorders; AV, acne vulgaris; HS, hidradenitis suppurativa; AA, alopecia areata; AGA, androgenic alopecia; ROS, rosacea; RPH, rhinophyma; SD, seborrhoeic dermatitis; and PC, pilonidal cyst.
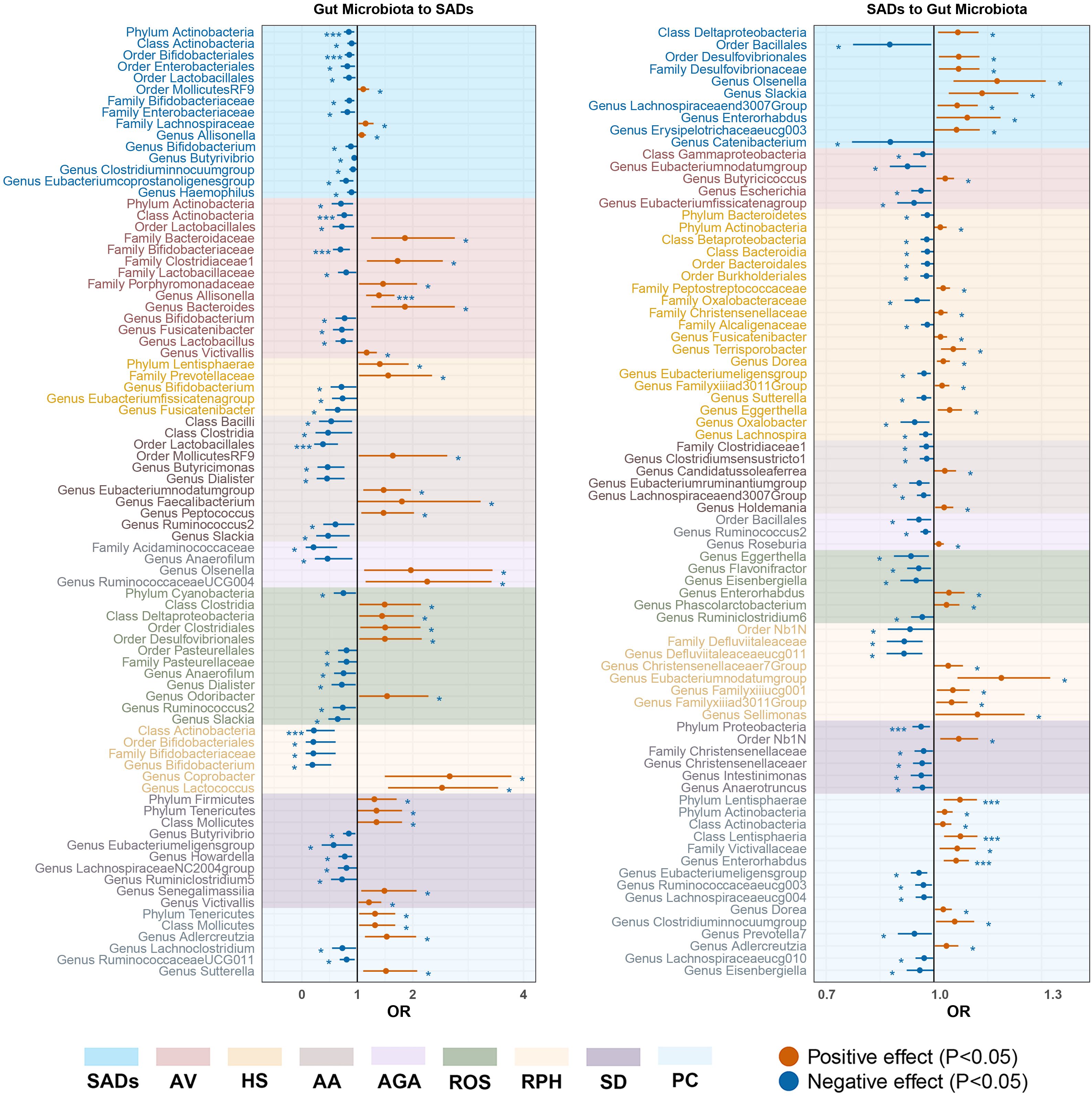
Figure 5. Forest plot of significant bidirectional causalities between gut microbiota and SADs. SADs, skin appendage Disorders; AV, acne vulgaris; HS, hidradenitis suppurativa; AA, alopecia areata; AGA, androgenic alopecia; ROS, rosacea; RPH, rhinophyma; SD, seborrhoeic dermatitis; and PC, pilonidal cyst. * and *** represent nominal causalities and strong causal associations, respectively.
SADs
For the skin microbiota, class Alphaproteobacteria and genus Streptococcus were associated with an inducing effect on total SAD risk. Order Actinomycetales and phylum Proteobacteria were associated with a protective effect on total SAD risk (Supplementary Table S8).
For the gut microbiota, order Bifidobacteriales (OR = 0.86, 95% CI = 0.77 - 0.95, P = 2.55 × 10−3, IVW) and phylum Actinobacteria (OR = 0.85, 95% CI = 0.76 - 0.94, P = 2.82 × 10−3, IVW) displayed strong causal associations with a decreased risk of SADs. Order MollicutesRF9, family Lachnospiraceae, and genus Allisonella were associated with an inducing effect on SADs. Ten bacterial taxa, namely, order Enterobacteriales and Lactobacillales, class Actinobacteria, family Bifidobacteriaceae and Enterobacteriaceae, and genus Bifidobacterium, Butyrivibrio, Clostridiuminnocuumgroup, Eubacteriumcoprostanoligenesgroup, and Haemophilus, were associated with a protective effect on SADs risk (Supplementary Table S10).
Acne vulgaris
For the skin microbiota, ASV008 [Staphylococcus (unc.)], ASV063 [Finegoldia (unc.)] and ASV086 [A. johnsonii] were associated with an inducing effect on AV risk. Genus Chryseobacterium was associated with a protective effect on AV risk (Supplementary Table S8).
For the gut microbiota, class Actinobacteria (OR = 0.76, 95% CI = 0.64 - 0.92, P = 4.21 × 10−3, IVW), and family Bifidobacteriaceae (OR = 0.70, 95% CI = 0.56 - 0.87, P = 1.08 × 10−3, IVW) displayed strong causal associations with a decreased risk of AV. Genus Allisonella (OR = 1.39, 95% CI = 1.16 - 1.67, P = 3.81 × 10−3, IVW) showed a strong causal association with an increased risk of AV. Five bacterial taxa, namely, family Bacteroidaceae, Clostridiaceae1, Porphyromonadaceae, and genus Bacteroides and Victivallis, were associated with an inducing effect on AV risk. Six bacterial taxa, namely, phylum Actinobacteria, order Lactobacillales, family Lactobacillaceae, and genus Bifidobacterium, Fusicatenibacter, and Lactobacillus were associated with a protective effect on AV risk (Supplementary Table S10).
Hidradenitis suppurativa
For the skin microbiota, ASV006 [S. hominis] was associated with a protective effect on the risk of HS (Supplementary Table S8).
For the gut microbiota, phylum Lentisphaerae and family Prevotellaceae were associated with an inducing effect on HS risk. Genus Bifidobacterium, Eubacteriumfissicatenagroup, and Fusicatenibacter were associated with a protective effect on HS risk (Supplementary Table S10).
Alopecia areata
For the skin microbiota, ASV010 [Staphylococcus (unc.)] and genus streptococcus were associated with an inducing effect on AA risk. Class Gammaproteobacteria, genus Corynebacterium, and Staphylococcus were associated with a protective effect on AA risk (Supplementary Table S8).
For the gut microbiota, order Lactobacillales (OR = 0.38, 95% CI = 0.22 - 0.65, P = 4.07 × 10−4, IVW) showed a strong causal association with a decreased risk of AA. Six bacterial taxa, namely, order MollicutesRF9, genus Olsenella, RuminococcaceaeUCG004, Eubacteriumnodatumgroup, Faecalibacterium, and Peptococcus, were associated with an inducing effect on AA risk. Eight bacterial taxa, namely, class Bacilli and Clostridia, family Acidaminococcaceae, and genus Anaerofilum, Butyricimonas, Dialister, Ruminococcus2, and Slackia, were associated with a protective effect on AA risk (Supplementary Table S10).
Androgenic alopecia
For the skin microbiota, ASV042 [Acinetobacter (unc.)] and class Gammaproteobacteria were associated with a protective effect on AGA risk (Supplementary Table S8).
For the gut microbiota, genus Olsenella and RuminococcaceaeUCG004 were associated with an inducing effect on AGA risk. Family Acidaminococcaceae and genus Anaerofilum were associated with a protective effect on AGA risk (Supplementary Table S10).
Rosacea
For the skin microbiota, ASV004 [Corynebacterium (unc.)] and family Micrococcaceae were associated with an inducing effect on ROS risk (Supplementary Table S8).
For the gut microbiota, five bacterial taxa, namely, class Clostridia and Deltaproteobacteria, order Clostridiales and Desulfovibrionales, and genus Odoribacter, were associated with an inducing effect on ROS risk. Phylum Cyanobacteria, order Pasteurellales, Family Pasteurellaceae, and genus Anaerofilum, Dialister, Ruminococcus2, and Slackia, were associated with a protective effect on ROS risk (Supplementary Table S10).
Rhinophyma
For the skin microbiota, ASV122 [Staphylococcus (unc.)] and class gammaproteobacteria were associated with an inducing effect on RPH risk. Phylum Bacteroidetes, ASV013 [S. epidermidis] and ASV100 [F. magna] were associated with a protective effect on RPH risk (Supplementary Table S8).
For the gut microbiota, class Actinobacteria (OR = 0.22, 95% CI = 0.08 - 0.59, P = 2.93 × 10−3, IVW) exhibited a strong causal association with a decreased risk of RPH. Genus Coprobacter and Lactococcus were associated with an inducing effect on RPH risk. Order Bifidobacteriales, family Bifidobacteriaceae, and genus Bifidobacterium were associated with a protective effect on RPH risk (Supplementary Table S10).
Seborrhoeic dermatitis
For the skin microbiota, the genus Micrococcus was associated with an inducing effect on SD risk. Family Rhodobacteraceae was associated with a protective effect on SD risk (Supplementary Table S8).
For the gut microbiota, five bacterial taxa, namely, phylum Tenericutes and Firmicutes, class Mollicutes, and genus Senegalimassilia and Victivallis, were associated with an inducing effect on SD risk. Genus Butyrivibrio, Eubacteriumeligensgroup, Howardella, LachnospiraceaeNC2004group, and Ruminiclostridium5 were associated with a protective effect on SD risk (Supplementary Table S10).
Pilonidal cyst
For the skin microbiota, phylum Firmicutes (OR = 0.22, 95% CI = 0.08 - 0.59, P = 2.93 × 10−3, IVW) displayed a strong causal association with an increased risk of PC. ASV011[Staphylococcus (unc.)], ASV042[Acinetobacter (unc.)] and genus Enhydrobacter were associated with an inducing effect on PC risk. ASV019[M. luteus] was associated with a protective effect on PC risk (Supplementary Table S8).
For the gut microbiota, phylum Tenericutes, class Mollicutes, genus Adlercreutzia, and Sutterella were associated with an inducing effect on PC risk. Genus Lachnoclostridium and RuminococcaceaeUCG011 were associated with a protective effect on PC risk (Supplementary Table S10).
Reverse MR analysis
In the reverse MR analysis, 119 causal associations were found between SADs and skin microbiota of the KORA FF4 and PopGen cohorts (Supplementary Table S7). After the microenvironment-based meta-analysis of two cohorts, 35 causal associations remained significant (P < 0.05) in the moist, dry, or sebaceous skin (Supplementary Table S9). Among the causalities, HS displayed a strong causal association with a decreased abundance of genus Rothia (OR = 0.78, 95% CI = 0.67 - 0.91, P = 1.10 × 10−3, IVW). RPA showed strong causal associations with an increased abundance of phylum Firmicutes (OR = 1.55, 95% CI = 1.14 - 2.08, P = 4.20 × 10−3, IVW) and genus Streptococcus (OR = 1.67, 95% CI = 1.22 - 2.29, P = 1.20 × 10−3, IVW). SD exhibited a strong causal association with an increased abundance of genus Streptococcus (OR = 1.43, 95% CI = 1.15 - 1.78, P = 1.30 × 10−3, IVW).
As for the gut, 78 causal associations were found between SADs and gut microbiota (Supplementary Table S11). Among the causalities, PC showed strong causal associations with an increased abundance of phylum Lentisphaerae (OR = 1.07, 95% CI = 1.03 - 1.12, P = 1.40 × 10−3, IVW), class Lentisphaeria (OR = 1.07, 95% CI = 1.03 - 1.12, P = 1.10 × 10−3, IVW) and genus Enterorhabdus (OR = 1.06, 95% CI = 1.03 - 1.10, P = 3.50 × 10−4, IVW). SD demonstrated a strong causal relationship with a drop abundance in the phylum Proteobacteria (OR = 0.96, 95% CI = 0.94 - 0.99, P = 4.60 × 10−3, IVW).
Sensitivity analysis
The causal estimates for magnitude and direction remained consistent across the weighted median, MR-Egger, weighted mode, and simple mode methods (Figure 6). The results of the LOO analysis indicated that no single SNP disproportionately influenced the overall causal estimate. No horizontal pleiotropy of the IVs was detected, as evidenced by the MR-PRESSO global test (P > 0.05) and MR-Egger regression (P > 0.05). Moreover, the Cochrane Q statistics indicated no significant heterogeneity (P > 0.05). The Steiger directionality test implied that the causalities identified were free of reverse causality bias (P < 0.05) (Supplementary Table S12-15).
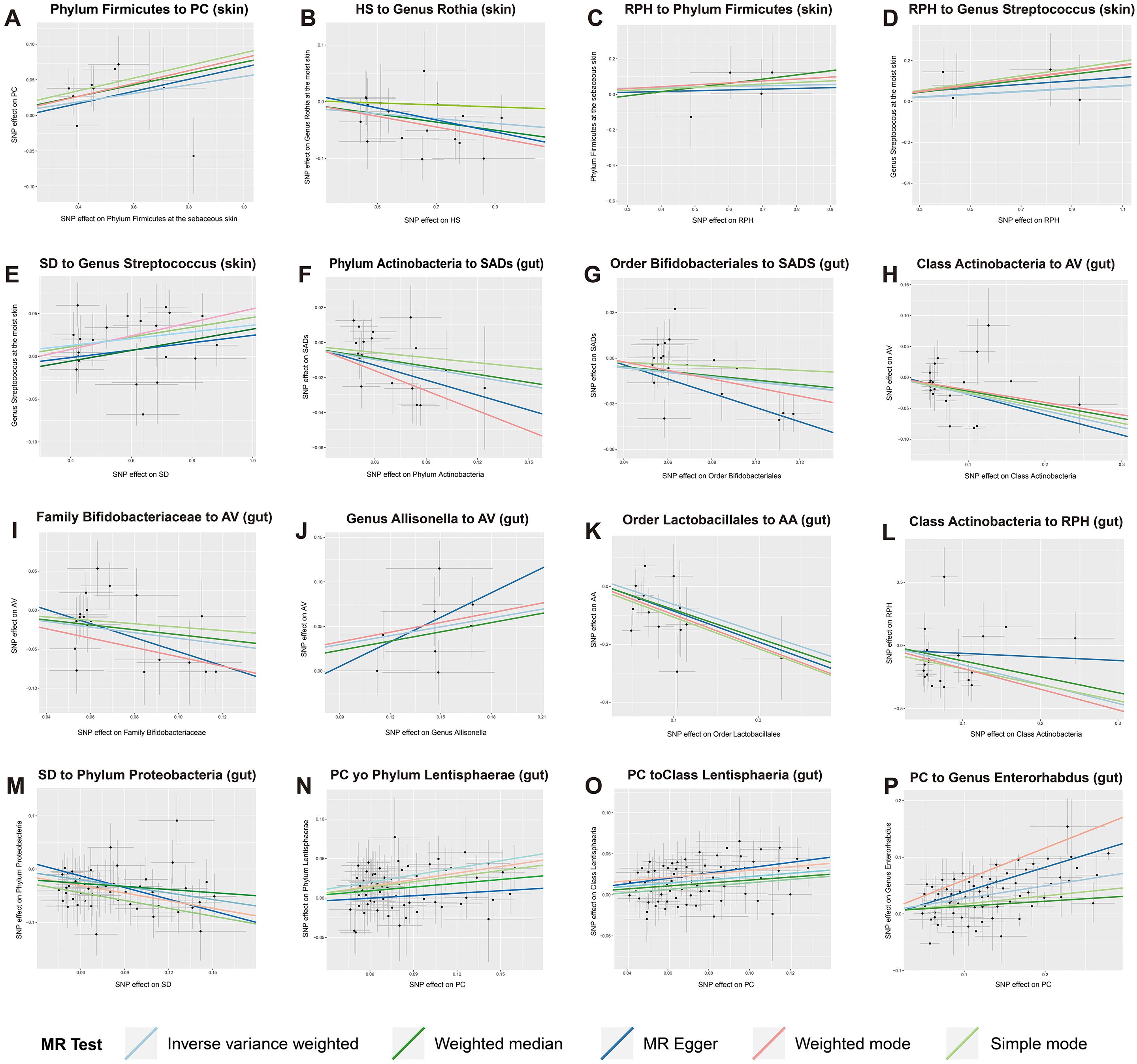
Figure 6. Scatter plots of strong causal associations between skin and gut microbiota and SADs. (A–E) the causal associations between skin microbiota and multiple SADs; (F–P) the causal associations between gut microbiota and multiple SADs. SADs, skin appendage Disorders; AV, acne vulgaris; SD, seborrhoeic dermatitis; HS, hidradenitis suppurativa; AA, alopecia areata; RPH, rhinophyma; and PC, pilonidal cyst.
Discussion
The results of this study provide a robust foundation for understanding the intricate relationships between the skin and gut microbiota and SADs. A total of 65 and 161 causal relationships between genetic liability in the skin and gut microbiota with SADs were identified, respectively. Among these, we separately found 5 and 11 strong causal associations that passed Bonferroni correction in the skin and gut microbiota with SADs. Several skin bacteria, such as Staphylococcus, Streptococcus, and Propionibacterium, were considered associated with multiple SADs. As gut probiotics, Bifidobacteria and Lactobacilli were associated with a protective effect on SAD risk. Our findings indicated the crucial role that gut and skin microbiota play in the host-microbiota interaction of skin diseases, supporting the notion that adjusting the host-microbe balance is crucial for the prevention and therapy of SADs.
Commensal bacteria are crucial for maintaining the immune system. Numerous studies have investigated the gut microbiome concerning various illnesses, including its effects on the skin (5). Skin bacteria, although less numerous than those found in the gastrointestinal tract, have comparable roles in immunological modulation and disease development (9). It is increasingly evident that both the cutaneous and gut microbiomes profoundly impact human health, particularly in the context of SADs.
P. acnes is recognized as the main disease-associated bacterium in the case of AV (30, 31). Research comparing the skin of acne sufferers and healthy individuals found that while the relative abundances of P. acnes species were similar, significant differences were observed at the strain level between the two cohorts (32). Certain strains had a strong connection to acne, whereas other strains were more prevalent in healthy skin. Interestingly, microbiome research has shown that AV is more closely associated with the virulence of specific P. acnes strains rather than the total quantity of P. acnes (31). Dysbiosis in AV is indicated by a reduction in the percentage of P. acnes strains RT6 and a notable increase in the percentages of P. acnes strains RT4, RT5, RT7, RT8, RT9, and RT10 (33). Moreover, a competitive relationship has been observed between S. epidermidis and C. acnes (34, 35). S. epidermidis promotes glycerol fermentation and releases succinic acid, which prevents C. acnes from proliferating (34). Conversely, P. acnes maintains the acidic environment of the pilosebaceous follicle, hydrolyzes sebaceous triglycerides, and produces propionic acid to inhibit S. epidermidis proliferation (35). Our research demonstrated that ASV001 [P. acnes] at sebaceous skin sites was positively correlated with AV in the PopGen cohort, whereas AV was negatively correlated with ASV013 [S. epidermidis] at moist skin sites in the KORA FF4 cohort. Although these associations lost significance in the meta-analysis, they potentially illustrate the role of P. acnes in promoting acne and inhibiting the colonization of S. epidermidis in acne patients.
Bifidobacteria and Lactobacilli, the commensal microorganisms inhabiting the gastrointestinal tract, have garnered interest for their therapeutic potential as probiotics in the amelioration of inflammatory dermatological conditions, including AV (34, 36). Their therapeutic actions are purportedly mediated through the modulation of systemic oxidative stress levels, the regulation of cytokine production, and the attenuation of inflammatory biomarkers (34). Clinical investigations have revealed that the administration of a composite probiotic formulation containing Lactobacillus acidophilus, Lactobacillus delbrueckii bulgaricus, and Bifidobacterium bifidum may rival the efficacy of conventional antibiotic therapy, such as minocycline, in acne management (37). An observed lesion reduction of 67% after a 12-week therapeutic regimen, coupled with a reduced incidence of adverse effects, substantiates this claim (37). The oral administration of various Lactobacillus species has been shown to reduce the total lesion count by 56%-67%, decrease sebum content by 81%, and improve the Investigators Global Assessment in 80% of patients (38). The MR outcomes of our research are consistent with the findings of previous studies. Bifidobacteria exhibited a significant causal association with inhibiting AV, and Lactobacilli were also regarded as potential protective factors for AV. Notably, the genus Allisonella showed a significant causal effect on facilitating AV, which may be due to its unique biochemical capability to produce histamine, a compound associated with gut inflammation, immune response, and allergic reactions (39).
Microbial changes in patients with AA revealed a lower abundance of S. epidermidis and over-colonization with P. acnes; however, it remains unclear if these alterations are the cause or effect of the illness (35). In this study, AA was positively correlated with ASV005 [P. granulosum] at moist skin sites, while the genus Staphylococcus at moist skin sites displayed a potential inhibitory effect on AA. Recent research has also connected AA to gut dysbiosis in addition to skin microbiota alterations. The role of the gut microbiome in the pathogenesis of AA is supported by cases of long-term hair growth following fecal microbiota transplants (40). However, some detected variations in gut flora in AA patients were not statistically significant (41). Following the Bonferroni adjustment, no apparent causal relationships between AA and the gut microbiota were found according to the reverse MR analysis, which aligns with previous studies. However, this MR study indicated that the order Lactobacillales displayed a significant causal association with inhibiting AA. Mechanistic studies have shown that Lactobacillales, as an important probiotic group, may influence AA through the modulation of immune function (36). Lactobacillales can regulate the body’s immune response via various mechanisms, such as promoting the production of regulatory T cells and reducing the release of pro-inflammatory cytokines (42). This immunomodulatory action may contribute to the suppression of the autoimmune response underlying AA.
There is growing evidence that a dysbiotic skin microbiome is associated with HS (43); however, the exact causal link between changes in the skin microbiome and the onset of the disease is still unknown. It was found that the bacterial community on the skin surface of HS patients was significantly altered, primarily characterized by a significant decrease in Staphylococcus epidermidis and Staphylococcus hominis in the axilla, gluteal cleft, and groin areas of HS patients (44). Propionibacterium was also observed to be more abundant in controls than in HS patients (44). The MR outcomes indicated that ASV006 [S. hominis] at moist skin sites was regarded as a potential protective factor for HS, and HS could induce the growth of ASV033 [S. capitis] at sebaceous skin sites. However, no causal association was found between Propionibacterium and HS, suggesting that this genus may not have a direct impact on the etiology of the illness. This dysbiosis of the skin microbiome may be related to chronic inflammation and a hypoxic environment in the HS focal areas. There are several comorbid conditions linked to HS. Notably, patients with HS have up to eight times higher rates of inflammatory bowel disease compared to the general population, with Crohn’s disease outpacing ulcerative colitis in frequency (45). An altered gut microbiota may be a factor in the development of HS since it has been linked to several pathophysiologies, including immune dysregulation (46). In this study, the genera Bifidobacterium, Eubacterium fissicatenagroup, and Fusicatenibacter exhibited potential causal effects on inhibiting HS, while the family Prevotellaceae and phylum Lentisphaerae showed potential causal effects on promoting HS. However, the specific mechanisms and modes of intervention for these causalities need to be further explored.
ROS, RPH, and SD are skin conditions associated with microbiological and immunological dysbiosis of the skin environment, as well as with Demodex mites and Malassezia fungus (47–49). Microbiota-associated alterations in the skin and small intestine have been concurrently noted in these diseases. Since ROS is exacerbated or triggered by emotional stress, the brain may play a role in the gut-brain-skin axis (47, 48). Unfortunately, conflicting findings have been reported in microbial research conducted on ROS patients at both the skin and gut levels (50). Some bacteria thought to be connected to ROS include S. epidermidis, Helicobacter pylori, Chlamydophila pneumoniae, and Bacillus oleronius (50). Our MR results indicated that ASV013 [S. epidermidis] at sebaceous skin sites could reduce the incidence of RPH, which is often considered a late complication of ROS. It has been suggested that ROS may be improved by taking oral probiotics such as Lactobacillus salivarius and Bifidobacterium, which was also observed in the MR results (36). The family Bifidobacteriaceae and the genus Bifidobacterium exhibited potential causal associations with inhibiting RPH. Additionally, it was reported that Acinetobacter, Staphylococcus, and Streptococcus predominated in lesional skin when examining the bacterial microbiota in 24 individuals with SD (3). This phenomenon was also reflected in the reverse MR analysis, which showed an increase in the genus Streptococcus and ASV086 [A. johnsonii]. Furthermore, it was shown that the phylum Firmicutes significantly contributed to the development of PC. Nevertheless, the connection between PC and the skin and gut microbiota has not been well studied, and further research is still required.
The intricate relationships between the skin and gut microbiota and SADs can be explained through several biological mechanisms. Firstly, microbes can significantly influence the host immune system. For instance, Staphylococcus aureus is known to produce superantigens and other virulence factors that hyperactivate the immune system, leading to chronic inflammation and tissue damage observed in conditions such as atopic dermatitis and hidradenitis suppurativa (51). These virulence factors can trigger the release of pro-inflammatory cytokines such as IL-1β, IL-6, and TNF-α, contributing to the inflammatory milieu characteristic of these disorders (52). Conversely, beneficial gut microbes like Bifidobacteria and Lactobacilli play a protective role by modulating systemic immune responses. These probiotics can enhance the production of anti-inflammatory cytokines, such as IL-10, and promote the differentiation of regulatory T cells, which help maintain immune homeostasis and prevent excessive inflammation (53). Secondly, microbial metabolites, such as short-chain fatty acids (SCFAs), play a crucial role in maintaining skin health. SCFAs like butyrate, acetate, and propionate, produced by gut microbiota during the fermentation of dietary fibers, have potent anti-inflammatory properties and can strengthen gut barrier function (54). These metabolites can enter the bloodstream and exert systemic effects, including on the skin. For example, SCFAs have been shown to enhance the differentiation of keratinocytes and promote wound healing, which could be beneficial for conditions like eczema and psoriasis (55). Moreover, the integrity of epithelial barriers in both the gut and skin is vital for preventing pathogen invasion and maintaining overall health. In the gut, harmful bacteria can disrupt tight junction proteins, leading to increased intestinal permeability, also known as “leaky gut,” which allows endotoxins to enter the bloodstream and trigger systemic inflammation (56). This systemic inflammation can adversely affect skin health, exacerbating conditions like acne and psoriasis (57).
Although the conservativeness of the Bonferroni correction method may lead to false negatives (58), the stringent control provided by the Bonferroni correction is essential for the integrity of our findings, thereby enhancing the reliability of causal inferences in genetic epidemiology (27, 28, 59). Many causal correlations in this study were regarded as nominally significant due to failing the Bonferroni corrected test. We speculate that the contribution of a single microbiome to illness may not be as substantial as initially estimated. Rather, the illnesses may be caused and coordinated by multiple bacteria. These bacteria with nominal causalities may also be involved in skin and intestinal-related SADs. The investigation of the human microbiota in SADs is presently ongoing, and the precise role of the microbiome in the pathophysiology of SADs remains to be thoroughly studied. Understanding the causality of the interplay between various microbiotas and SADs can aid in comprehending the intricate crosstalk between the skin and gut, and offer guidance for future targeted multi-flora medication development.
To the best of our knowledge, this is the first MR study to comprehensively examine the causal effect between skin and gut microbiota and SADs. A bidirectional, two-sample MR design following STROBE-MR guidelines was employed to eliminate the potential for reverse causation and confounding factors. Exposure and outcome summary data were separately acquired from German and Finnish populations to ensure nonoverlapping data sets and avoid bias. A microenvironment-based meta-analysis of skin microbiota was conducted to enhance the statistical power of the results. However, several limitations of our study should be noted. First, we included SNPs that met the locus-wide significance level (1 × 10−5), as the SNPs identified using the genome-wide significance threshold (5 × 10−8) were insufficient for sensitivity analysis and horizontal pleiotropy detection. Second, some taxa of skin microbiota at the ASV level lacked species-level annotations, possibly due to uncertain matches to the Ribosomal Database Project (RDP) database. Additionally, most 16S rRNA sequencing of human microbiota has focused on species composition. Recent research has indicated that distinct strains of microorganisms can exert significantly different effects on the host, even within the same species (60). Variations at the strain level have not been well studied and remain an area of interest for microbiota research. Third, there is limited knowledge of the causal link between SADs and non-bacterial components of the microbiota, such as fungi, archaea, and viruses. Therefore, future research using more advanced sequencing technology should be conducted to further elucidate the effects of skin and gut microbiota on SADs.
In summary, our study provided comprehensive evidence for the causal roles of skin and gut microbiota in SADs through the application of bidirectional MR analysis. This novel approach allowed us to establish strong causal links between specific microbial taxa and various SADs, underscoring the intricate interplay between microbial communities and skin health. The identification of specific skin and gut microbiota that influence the risk of SADs offers promising avenues for the development of microbiome-based diagnostic and therapeutic strategies. Future studies involving genomic, transcriptomic, and metabolomic analyses should focus on elucidating the underlying mechanisms of how specific microbes influence SADs and modulate skin health.
Data availability statement
The datasets presented in this study can be found in online repositories. The names of the repository/repositories and accession number(s) can be found in the article/Supplementary Material.
Author contributions
YZ: Writing – original draft, Methodology, Investigation, Formal analysis, Data curation. WL: Writing – original draft, Methodology, Formal analysis, Data curation. MW: Writing – original draft, Visualization, Software, Resources. XW: Writing – original draft, Visualization, Software, Resources. SW: Writing – review & editing, Validation, Conceptualization.
Funding
The author(s) declare financial support was received for the research, authorship, and/or publication of this article. This work was supported by the Jilin Provincial Natural Science Foundation (YDZJ202401246ZYTS).
Acknowledgments
We are grateful to all study participants and researchers for their participation and dedication to this research, as well as to the consortium studies for releasing comprehensive association information for public consumption. Figure 1 was partly created using BioRender.com.
Conflict of interest
The authors declare that the research was conducted in the absence of any commercial or financial relationships that could be construed as a potential conflict of interest.
Publisher’s note
All claims expressed in this article are solely those of the authors and do not necessarily represent those of their affiliated organizations, or those of the publisher, the editors and the reviewers. Any product that may be evaluated in this article, or claim that may be made by its manufacturer, is not guaranteed or endorsed by the publisher.
Supplementary material
The Supplementary Material for this article can be found online at: https://www.frontiersin.org/articles/10.3389/fimmu.2024.1427276/full#supplementary-material
Additional File 2 | STROBE-MR checklist of suggested items for Mendelian randomization studies.
References
1. Liu Q, Ranallo R, Rios C, Grice EA, Moon K, Gallo RL. Crosstalk between skin microbiota and immune system in health and disease. Nat Immunol. (2023) 24:895–8. doi: 10.1038/s41590-023-01500-6
2. Ito Y, Amagai M. Dissecting skin microbiota and microenvironment for the development of therapeutic strategies. Curr Opin Microbiol. (2023) 74:102311. doi: 10.1016/j.mib.2023.102311
3. Zhang XE, Zheng P, Ye SZ, Ma X, Liu E, Pang YB, et al. Microbiome: role in inflammatory skin diseases. J Inflammation Res. (2024) 17:1057–82. doi: 10.2147/JIR.S441100
4. Ito Y, Amagai M. Controlling skin microbiome as a new bacteriotherapy for inflammatory skin diseases. Inflammation Regen. (2022) 42:26. doi: 10.1186/s41232-022-00212-y
5. Perdijk O, Azzoni R, Marsland BJ. The microbiome: an integral player in immune homeostasis and inflammation in the respiratory tract. Physiol Rev. (2024) 104:835–79. doi: 10.1152/physrev.00020.2023
6. Ryguła I, Pikiewicz W, Grabarek BO, Wójcik M, Kaminiów K. The role of the gut microbiome and microbial dysbiosis in common skin diseases. Int J Mol Sci. (2024) 25:1984. doi: 10.3390/ijms25041984
7. Sinha S, Lin G, Ferenczi K. The skin microbiome and the gut-skin axis. Clin Dermatol. (2021) 39:829–39. doi: 10.1016/j.clindermatol.2021.08.021
9. Harris-Tryon TA, Grice EA. Microbiota and maintenance of skin barrier function. Science. (2022) 376:940–5. doi: 10.1126/science.abo0693
10. Zhang Y, Wang M, Li Z, Yang X, Li K, Xie A, et al. An overview of detecting gene-trait associations by integrating GWAS summary statistics and eQTLs. Sci China Life Sci. (2024) 67:1133–54. doi: 10.1007/s11427-023-2522-8
11. Moitinho-Silva L, Degenhardt F, Rodriguez E, Emmert H, Juzenas S, Möbus L, et al. Host genetic factors related to innate immunity, environmental sensing and cellular functions are associated with human skin microbiota. Nat Commun. (2022) 13:6204. doi: 10.1038/s41467-022-33906-5
12. Kurilshikov A, Medina-Gomez C, Bacigalupe R, Radjabzadeh D, Wang J, Demirkan A, et al. Large-scale association analyses identify host factors influencing human gut microbiome composition. Nat Genet. (2021) 53:156–65. doi: 10.1038/s41588-020-00763-1
13. Kurki MI, Karjalainen J, Palta P, Sipilä TP, Kristiansson K, Donner KM, et al. FinnGen provides genetic insights from a well-phenotyped isolated population. Nature. (2023) 613:508–18. doi: 10.1038/s41586-022-05473-8
14. Chen H, Peng L, Wang Z, He Y, Zhang X. Exploring the causal relationship between periodontitis and gut microbiome: Unveiling the oral-gut and gut-oral axes through bidirectional Mendelian randomization. J Clin Periodontol. (2024) 51:417–30. doi: 10.1111/jcpe.13906
15. Hou T, Dai H, Wang Q, Hou Y, Zhang X, Lin H, et al. Dissecting the causal effect between gut microbiota, DHA, and urate metabolism: A large-scale bidirectional Mendelian randomization. Front Immunol. (2023) 14:1148591. doi: 10.3389/fimmu.2023.1148591
16. Jin Q, Ren F, Dai D, Sun N, Qian Y, Song P. The causality between intestinal flora and allergic diseases: Insights from a bi-directional two-sample Mendelian randomization analysis. Front Immunol. (2023) 14:1121273. doi: 10.3389/fimmu.2023.1121273
17. Lou J, Cui S, Li J, Jin G, Fan Y, Huang N. Causal relationship between the gut microbiome and basal cell carcinoma, melanoma skin cancer, ease of skin tanning: evidence from three two-sample mendelian randomisation studies. Front Immunol. (2024) 15:1279680. doi: 10.3389/fimmu.2024.1279680
18. Burgess S, Butterworth A, Thompson SG. Mendelian randomization analysis with multiple genetic variants using summarized data. Genet Epidemiol. (2013) 37:658–65. doi: 10.1002/gepi.21758
19. Bowden J, Davey Smith G, Haycock PC, Burgess S. Consistent estimation in Mendelian randomization with some invalid instruments using a weighted median estimator. Genet Epidemiol. (2016) 40:304–14. doi: 10.1002/gepi.21965
20. Bowden J, Davey Smith G, Burgess S. Mendelian randomization with invalid instruments: effect estimation and bias detection through Egger regression. Int J Epidemiol. (2015) 44:512–25. doi: 10.1093/ije/dyv080
21. Hemani G, Bowden J, Davey Smith G. Evaluating the potential role of pleiotropy in Mendelian randomization studies. Hum Mol Genet. (2018) 27:R195–r208. doi: 10.1093/hmg/ddy163
22. Hartwig FP, Davey Smith G, Bowden J. Robust inference in summary data Mendelian randomization via the zero modal pleiotropy assumption. Int J Epidemiol. (2017) 46:1985–98. doi: 10.1093/ije/dyx102
23. Skrivankova VW, Richmond RC, Woolf BAR, Davies NM, Swanson SA, VanderWeele TJ, et al. Strengthening the reporting of observational studies in epidemiology using mendelian randomisation (STROBE-MR): explanation and elaboration. Bmj. (2021) 375:n2233. doi: 10.1136/bmj.n2233
24. Skrivankova VW, Richmond RC, Woolf BAR, Yarmolinsky J, Davies NM, Swanson SA, et al. Strengthening the reporting of observational studies in epidemiology using Mendelian randomization: the STROBE-MR statement. Jama. (2021) 326:1614–21. doi: 10.1001/jama.2021.18236
25. Gkatzionis A, Burgess S, Newcombe PJ. Statistical methods for cis-Mendelian randomization with two-sample summary-level data. Genet Epidemiol. (2023) 47:3–25. doi: 10.1002/gepi.22506
26. Xiong H, Zhang H, Bai J, Li Y, Li L, Zhang L. Associations of the circulating levels of cytokines with the risk of myeloproliferative neoplasms: a bidirectional mendelian-randomization study. BMC Cancer. (2024) 24:531. doi: 10.1186/s12885-024-12301-x
27. Xie S, Zhang R, Tang Y, Dai Q. Exploring causal correlations between inflammatory cytokines and Ménière's disease: a Mendelian randomization. Front Immunol. (2024) 15:1373723. doi: 10.3389/fimmu.2024.1373723
28. Chen Y, Xie Y, Ci H, Cheng Z, Kuang Y, Li S, et al. Plasma metabolites and risk of seven cancers: a two-sample Mendelian randomization study among European descendants. BMC Med. (2024) 22:90. doi: 10.1186/s12916-024-03272-8
29. Xue Y, Wang X, Liu H, Kang J, Liang X, Yao A, et al. Assessment of the relationship between gut microbiota and bone mineral density: a two-sample Mendelian randomization study. Front Microbiol. (2024) 15:1298838. doi: 10.3389/fmicb.2024.1298838
30. Ramasamy S, Barnard E, Dawson TL Jr., Li H. The role of the skin microbiota in acne pathophysiology. Br J Dermatol. (2019) 181:691–9. doi: 10.1111/bjd.18230
31. Xu H, Li H. Acne, the skin microbiome, and antibiotic treatment. Am J Clin Dermatol. (2019) 20:335–44. doi: 10.1007/s40257-018-00417-3
32. Dessinioti C, Katsambas A. The microbiome and acne: perspectives for treatment. Dermatol Ther (Heidelb). (2024) 14:31–44. doi: 10.1007/s13555-023-01079-8
33. Zhou H, Shi L, Ren Y, Tan X, Liu W, Liu Z. Applications of human skin microbiota in the cutaneous disorders for ecology-based therapy. Front Cell Infect Microbiol. (2020) 10:570261. doi: 10.3389/fcimb.2020.570261
34. Dréno B, Dagnelie MA, Khammari A, Corvec S. The skin microbiome: A new actor in inflammatory acne. Am J Clin Dermatol. (2020) 21:18–24. doi: 10.1007/s40257-020-00531-1
35. Carmona-Cruz S, Orozco-Covarrubias L, Sáez-de-Ocariz M. The human skin microbiome in selected cutaneous diseases. Front Cell Infect Microbiol. (2022) 12:834135. doi: 10.3389/fcimb.2022.834135
36. Gao T, Wang X, Li Y, Ren F. The role of probiotics in skin health and related gut-skin axis: A review. Nutrients. (2023) 15:3132. doi: 10.3390/nu15143123
37. Kim HJ, Kim YH. Exploring acne treatments: from pathophysiological mechanisms to emerging therapies. Int J Mol Sci. (2024) 25:5302. doi: 10.3390/ijms25105302
38. Shields A, Ly S, Wafae B, Chang YF, Manjaly P, Archila M, et al. Safety and effectiveness of oral nutraceuticals for treating acne: A systematic review. JAMA Dermatol. (2023) 159:1373–82. doi: 10.1001/jamadermatol.2023.3949
39. Sarasola MP, Táquez Delgado MA, Nicoud MB, Medina VA. Histamine in cancer immunology and immunotherapy. Current status and new perspectives. Pharmacol Res Perspect. (2021) 9:e00778. doi: 10.1002/prp2.778
40. Passeron T, King B, Seneschal J, Steinhoff M, Jabbari A, Ohyama M, et al. Inhibition of T-cell activity in alopecia areata: recent developments and new directions. Front Immunol. (2023) 14:1243556. doi: 10.3389/fimmu.2023.1243556
41. De Pessemier B, Grine L, Debaere M, Maes A, Paetzold B, Callewaert C. Gut-skin axis: current knowledge of the interrelationship between microbial dysbiosis and skin conditions. Microorganisms. (2021) 9:353. doi: 10.3390/microorganisms9020353
42. Zhang J, Yao Z. Immune cell trafficking: a novel perspective on the gut-skin axis. Inflammation Regen. (2024) 44:21. doi: 10.1186/s41232-024-00334-5
43. Luck ME, Tao J, Lake EP. The skin and gut microbiome in Hidradenitis suppurativa: current understanding and future considerations for research and treatment. Am J Clin Dermatol. (2022) 23:841–52. doi: 10.1007/s40257-022-00724-w
44. Molinelli E, Gioacchini H, Marani A, Rizzetto G, Gambini D, De Simoni E, et al. Topical and systemic retinoids in the management of Hidradenitis suppurativa: A comprehensive literature review. Dermatol Ther (Heidelb). (2024) 14:1079–91. doi: 10.1007/s13555-024-01169-1
45. Brydges HT, Onuh OC, Friedman R, Barrett J, Betensky RA, Lu CP, et al. Autoimmune, autoinflammatory disease and cutaneous Malignancy associations with hidradenitis suppurativa: A cross-sectional study. Am J Clin Dermatol. (2024). 25:473–84. doi: 10.1007/s40257-024-00844-5
46. McCarthy S, Barrett M, Kirthi S, Pellanda P, Vlckova K, Tobin AM, et al. Altered skin and gut microbiome in Hidradenitis suppurativa. J Invest Dermatol. (2022) 142:459–68.e15. doi: 10.1016/j.jid.2021.05.036
47. Zhu W, Hamblin MR, Wen X. Role of the skin microbiota and intestinal microbiome in rosacea. Front Microbiol. (2023) 14:1108661. doi: 10.3389/fmicb.2023.1108661
48. Sánchez-Pellicer P, Eguren-Michelena C, García-Gavín J, Llamas-Velasco M, Navarro-Moratalla L, Núñez-Delegido E, et al. Rosacea, microbiome and probiotics: the gut-skin axis. Front Microbiol. (2023) 14:1323644. doi: 10.3389/fmicb.2023.1323644
49. Tao R, Li R, Wang R. Skin microbiome alterations in seborrheic dermatitis and dandruff: A systematic review. Exp Dermatol. (2021) 30:1546–53. doi: 10.1111/exd.14450
50. Kim HS. Microbiota in rosacea. Am J Clin Dermatol. (2020) 21:25–35. doi: 10.1007/s40257-020-00546-8
51. Piewngam P, Otto M. Staphylococcus aureus colonisation and strategies for decolonisation. Lancet Microbe. (2024) 5:e606–e18. doi: 10.1016/S2666-5247(24)00040-5
52. Ganal-Vonarburg SC, Duerr CU. The interaction of intestinal microbiota and innate lymphoid cells in health and disease throughout life. Immunology. (2020) 159:39–51. doi: 10.1111/imm.13138
53. Facchin S, Bertin L, Bonazzi E, Lorenzon G, De Barba C, Barberio B, et al. Short-chain fatty acids and human health: from metabolic pathways to current therapeutic implications. Life (Basel). (2024) 14:559. doi: 10.3390/life14050559
54. Abdelhalim KA. Short-chain fatty acids (SCFAs) from gastrointestinal disorders, metabolism, epigenetics, central nervous system to cancer - A mini-review. Chem Biol Interact. (2024) 388:110851. doi: 10.1016/j.cbi.2023.110851
55. Trompette A, Pernot J, Perdijk O, Alqahtani RAA, Domingo JS, Camacho-Muñoz D, et al. Gut-derived short-chain fatty acids modulate skin barrier integrity by promoting keratinocyte metabolism and differentiation. Mucosal Immunol. (2022) 15:908–26. doi: 10.1038/s41385-022-00524-9
56. Busch CBE, Bergman J, Nieuwdorp M, van Baar ACG. Role of the intestine and its gut microbiota in metabolic syndrome and obesity. Am J Gastroenterol. (2024) 119:1038–46. doi: 10.14309/ajg.0000000000002730
57. Mahmud MR, Akter S, Tamanna SK, Mazumder L, Esti IZ, Banerjee S, et al. Impact of gut microbiome on skin health: gut-skin axis observed through the lenses of therapeutics and skin diseases. Gut Microbes. (2022) 14:2096995. doi: 10.1080/19490976.2022.2096995
58. Li N, Wang Y, Wei P, Min Y, Yu M, Zhou G, et al. Causal effects of specific gut microbiota on chronic kidney diseases and renal function-A two-sample Mendelian randomization study. Nutrients. (2023) 15:360. doi: 10.3390/nu15020360
59. Wang K, Shi X, Zhu Z, Hao X, Chen L, Cheng S, et al. Mendelian randomization analysis of 37 clinical factors and coronary artery disease in East Asian and European populations. Genome Med. (2022) 14:63. doi: 10.1186/s13073-022-01067-1
Keywords: skin microbiota, gut microbiota, skin appendage disorders, Mendelian randomization, causal inference
Citation: Zhu Y, Liu W, Wang M, Wang X and Wang S (2024) Causal roles of skin and gut microbiota in skin appendage disorders suggested by genetic study. Front. Immunol. 15:1427276. doi: 10.3389/fimmu.2024.1427276
Received: 03 May 2024; Accepted: 22 August 2024;
Published: 10 September 2024.
Edited by:
Omar Ramos-Lopez, Universidad Autónoma de Baja California, Tijuana, MexicoReviewed by:
Danielle Oliveira Nascimento, Federal Rural University of Rio de Janeiro, BrazilMangala Hegde, Indian Institute of Technology Guwahati, India
Xiaonan Zhang, Chongqing Medical University, China
Copyright © 2024 Zhu, Liu, Wang, Wang and Wang. This is an open-access article distributed under the terms of the Creative Commons Attribution License (CC BY). The use, distribution or reproduction in other forums is permitted, provided the original author(s) and the copyright owner(s) are credited and that the original publication in this journal is cited, in accordance with accepted academic practice. No use, distribution or reproduction is permitted which does not comply with these terms.
*Correspondence: Sibo Wang, wangsibo@jlu.edu.cn