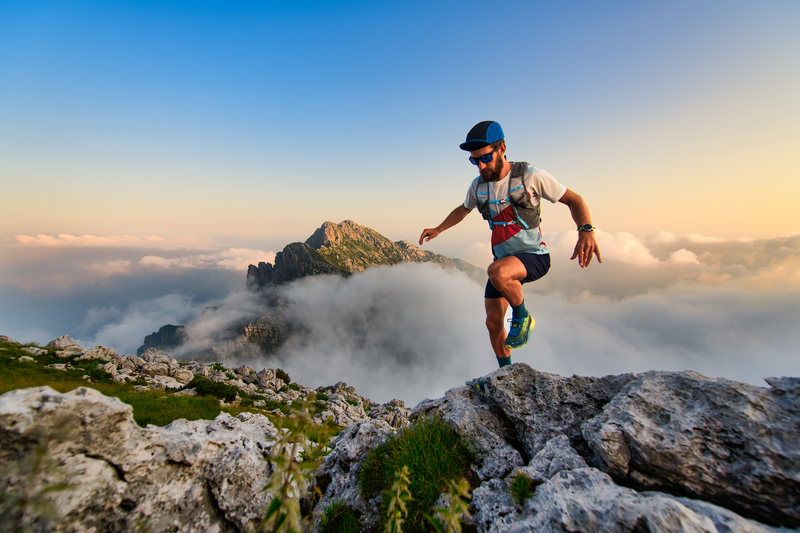
94% of researchers rate our articles as excellent or good
Learn more about the work of our research integrity team to safeguard the quality of each article we publish.
Find out more
ORIGINAL RESEARCH article
Front. Immunol. , 25 September 2024
Sec. Microbial Immunology
Volume 15 - 2024 | https://doi.org/10.3389/fimmu.2024.1463309
This article is part of the Research Topic Stem cell niche, microbes, and cancer View all 9 articles
Background: Despite relevant research, the relationship between skin microbiomes and prostate cancer remains controversial. This study utilizes bidirectional Mendelian randomization (MR) analysis combined with meta-analysis to explore the potential link between the two.
Objective: This study aims to identify the causal relationship between 150 skin microbiomes and prostate cancer (PCa) using bidirectional Mendelian randomization (MR) and meta-analysis.
Methods: This study employed a comprehensive Bidirectional Two-sample MR analysis using publicly available genetic data to ascertain the relationship between 150 skin microbiomes and PCa. We conducted extensive sensitivity analyses, tests for heterogeneity, and assessments of horizontal pleiotropy to ensure the accuracy of our results. Subsequently, we conducted a meta-analysis to strengthen our conclusions’ robustness further. Finally, we performed reverse causal verification on the positive skin microbiomes and PCa.
Results: After conducting a meta-analysis and multiple corrections of the MR analysis results, our findings reveal a correlation between Neisseria in dry skin and PCa risk, identifying it as a risk factor. The IVW result shows an Odds Ratio (OR) of 1.009 (95% Confidence Interval [CI]: 1.004-1.014, P = 0.027). Furthermore, the reverse MR analysis indicates the absence of an inverse causal relationship between the two. Apart from the identified skin microbiome, no significant associations were found between the other microbiomes and PCa.
Conclusions: The study identified a correlation between Neisseria in dry skin, one of the 150 skin microbiomes, and the risk of developing PCa, establishing it as a risk factor for increased susceptibility to PCa.
Prostate cancer (PCa) is one of the most common malignant tumors among men worldwide, with approximately 1.5 million new cases diagnosed each year, accounting for 7.3% of all new cancer cases globally (1). This makes it the second most common cancer type among men, second only to lung cancer (2, 3). The exact etiology of PCa is not fully understood. Current perspectives suggest that genetic factors, racial factors, lifestyle, and dietary habits are all risk factors for the development of PCa (4). Additionally, age is a significant risk factor for PCa (5), with the incidence rate remaining high due to the increasing aging population, making PCa a critical factor affecting men’s physical and mental health globally.
Although early detection and treatment can significantly improve cure rates, with the five-year survival rate for early PCa approaching 100% (6), the prognosis for advanced PCa is poor, with a five-year survival rate of approximately 30% (5). Advanced patients often suffer from severe complications, including urinary incontinence (7), sexual dysfunction (8), bone pain due to bone metastases (9), and pathological fractures (9), severely impacting their physical and mental well-being. Given the high incidence and complication risks, in-depth research on the etiology, prevention, early screening, and treatment of PCa is crucial to optimizing public health strategies, increasing early detection rates, and improving patients’ quality of life, thereby effectively addressing this global health challenge.
The skin is the largest organ of the human body, with a surface area of approximately 1.8 square meters, hosting a rich and complex microbiome (10). These microbiomes mainly include bacteria, fungi, viruses, and parasites, with bacteria being the predominant group (11). The composition and diversity of skin microbiomes are influenced by various factors, including the host’s age, gender, ethnicity, geographic location, lifestyle, immune status, and disease state (12). Skin microbiomes play crucial roles in maintaining skin barrier function, regulating immune responses, and preventing pathogenic infections (13). Recent research has increasingly shown that dysbiosis of the skin microbiome may be closely related to various skin diseases and systemic conditions (11, 14).
In recent years, research on the relationship between microbiomes and cancer has gradually increased. The relationship between gut microbiota and colorectal cancer has been extensively studied and recognized (15). Both gut and skin microbiota play important roles in regulating host health, with interactions between them potentially coordinated through the “gut-skin axis,” thereby regulating host health by modulating the immune system and metabolic pathways (16). Previous studies have suggested that the skin microbiome might influence cancer development and progression through various mechanisms (17). Consistent findings indicate a close relationship between the skin microbiome and certain malignancies, such as leukemia (18).
Although studies on the relationship between PCa and the skin microbiome are relatively limited, existing research indicates significant changes in the composition and diversity of the microbiome in PCa patients, suggesting that microbiomes, including those of the skin, may play important roles in the development and progression of PCa (19). This underscores the importance of studying the relationship between the skin microbiome and PCa for the diagnosis and development of new therapeutic targets. Moreover, a study by Davidsson et al. found that Propionibacterium acnes in the skin of patients with prostatitis might be associated with an increased risk of PCa, highlighting the close relationship and significant research value of skin microbiome alterations in PCa (20). However, due to confounding factors and reverse causality, traditional observational studies cannot fully elucidate the relationship between various skin microbiomes and PCa. Additionally, conducting randomized controlled trials to explore this relationship faces significant challenges, including sample size, funding, time constraints, and ethical considerations.
Over the past two decades, MR has gained attention as a reliable research method (21). It uses genetic variants, such as single nucleotide polymorphisms (SNPs), as instrumental variables (IVs) to establish causal relationships between exposure factors and disease outcomes. These variants are associated with the exposure of interest but are not influenced by lifestyle or socioeconomic factors. This method reduces the impact of confounding factors and reverses causality common in traditional observational studies, thereby enhancing the robustness of the results. IVs used in MR studies must meet three key assumptions: they should be strongly associated with the intermediate exposure, independent of confounding factors, and not exert direct pleiotropic effects on the outcome. These assumptions make MR a powerful method for establishing causal relationships in the absence of randomized controlled trials.
Unlike traditional unidirectional MR, bidirectional two-sample MR analysis allows researchers to explore causal relationships in both directions (22). This helps rule out reverse causality and enhances the credibility of the findings by verifying bidirectional causality. Significant causal relationships found in both directions suggest a more likely true association rather than one caused by confounding factors or biases. Additionally, this method leverages genetic and phenotypic data from different datasets, expanding the study scope and enhancing the generalizability of the findings.
Therefore, this study employs bidirectional two-sample MR analysis to explore the causal relationships between 150 skin microbiome phenotypes and PCa, elucidating both forward and reverse associations. We further conducted a meta-analysis on the PCa results from different databases to strengthen the robustness of our findings. After completing the MR analysis, we applied the Bonferroni correction to account for multiple testing. Finally, we performed reverse MR analysis to verify the reverse causal relationship between the skin microbiome phenotypes and PCa phenotypes that showed positive outcomes, thereby excluding the impact of reverse causality and enhancing the robustness of our results.
During the study, we first collected optimal exposure and outcome data and performed data preprocessing. Subsequently, we conducted MR analysis using the preprocessed exposure data (150 skin microbiomes) against outcome data (PCa) from two distinct databases. We then performed a meta-analysis (23) on the IVW results derived from MR analyses using PCa data from different databases and conducted multiple corrections on the meta-analysis results to ensure data accuracy. Finally, we designated the skin microbiomes identified as positive outcomes as the exposure data and PCa as the outcome data, employing the same instrumental variable selection and data analysis methods as in the forward analysis to perform a reverse MR analysis. The advantage of combining MR analysis with meta-analysis lies in its ability to synthesize results from multiple studies, reduce result bias, explore heterogeneity, and enhance the generalizability of the findings. By integrating data from different studies, we can more comprehensively assess the association between exposure and outcome, providing more reliable results and deepening our understanding of the research question. This study utilizes large public datasets, all of which have received approval from relevant institutions and associations, and informed consent was obtained from all participants. Consequently, no additional ethical review is required for this study. To present the research process more clearly, we have drawn a corresponding flowchart (Figure 1). Additionally, our study confirms adherence to the STROBE-MR guidelines (Supplementary Table 1).
The data on the 150 types of skin microbiota used in this study were sourced from the research conducted by Moitinho-Silva et al., published in 2022 in Nature Communications, titled “Host genetic factors related to innate immunity, environmental sensing, and cellular functions are associated with human skin microbiota.” This study performed genome-wide association studies (GWAS) on two German cohorts, including 474 cases and 419 controls, totaling 893 samples. It analyzed the microbiota from different skin microenvironments (dry skin, moist skin, and sebaceous-rich skin), specifically measuring 837,260 SNP markers. These SNP markers were used in GWAS to identify host genetic factors associated with skin microbiota. The study involved 150 different skin microbiota traits and revealed 23 genetic loci significantly associated with skin microbiota characteristics. These genes are mainly involved in innate immune signaling, environmental sensing, cellular differentiation, and proliferation. The data have been included in the public database GWAS Catalog, with the registration numbers GCST90133164-GCST90133313. We downloaded the manually curated complete data from the GWAS Catalog database (download link: https://www.ebi.ac.uk/gwas/publications/36261456, download date: June 1, 2024).
The PCa data were aggregated from two GWAS datasets involving European populations. FinnGen is a large public-private partnership project that utilizes Finland’s unique genetic resources to generate high-quality genetic and health data. This project combines whole-genome data from approximately 500,000 Finnish individuals with extensive health registry information, representing nearly 10% of Finland’s total population. The first dataset (referred to as FinnGen) is derived from the R10 release of the FinnGen database (https://www.finngen.fi/en), encompassing a GWAS on146,465 Europeans (15,199 cases, 131,266 controls) (24). The UK Biobank (UKB) is a significant biomedical research resource, supported by the UK government, the Medical Research Council (MRC), and the Wellcome Trust. This database includes detailed health and genetic information from around 500,000 UK participants aged 40 to 69, covering various traits and diseases. Users can easily access the required data through search and download functionalities, supporting genetic analyses and causal inference studies. The UK Biobank aims to promote open data sharing, advancing genetic epidemiology and public health research, making it a crucial tool for global medical research. The second dataset (referred to as UKB) is sourced from the UK Biobank (Pan-UKB team. https://pan.ukbb.broadinstitute.org.2020.), including a GWAS on 364,233 Europeans (28,010 cases, 336,223 controls). Both datasets have undergone manual review to ensure data accuracy.
In omics MR studies, selecting effective instrumental variables (IVs) is crucial. Initially, this study employed a selection threshold of P < 1x10-5 to ensure that only SNPs strongly associated with various micronutrients were retained. For most micronutrients, the number of associated SNPs exceeded three, ensuring the representativeness and relevance of the data. Next, to further refine the selection of robust instrumental variables, the F-statistic for each SNP was calculated using the formula F = (beta/se) ². Only SNPs with an F value greater than 10 were retained, a step that helps eliminate weak instrumental variables and enhances the reliability of the study’s findings. Additionally, the minor allele frequency (MAF) was calculated using the effect allele frequency (eaf). If the eaf was less than 0.5, the MAF was set to eaf; otherwise, it was set to 1 - eaf. Only SNPs with an MAF greater than 0.01 were retained to exclude rare variants that could affect the study’s results. Finally, the filtered data were formatted for MR analysis, and linkage disequilibrium (LD) was addressed to avoid its impact on the accuracy of the results. Specifically, the distance threshold was set at 10,000 kilobases (kb) and the LD threshold at 0.001. These steps ensured the independence of the instrumental variables and the precision of the results (The results are shown in Supplementary Table 1). Overall, the specific selection criteria were: P < 1x10-5, MAF > 0.01, F > 10, clump_kb = 10000, and clump_r2 = 0.001.
In the first step of the forward analysis, we extracted SNP data from the PCa phenotype outcome that matches the 150 skin microbiome phenotypes. Next, we processed the palindromic SNPs based on the specific criterion of action = 2. Additionally, we excluded data with mr_keep = false.
Before performing MR-PRESSO, we conducted a horizontal pleiotropy test on the processed data. If an SNP had a p-value less than 0.05, it was considered horizontally pleiotropic, indicating an outlier, and subsequently removed using the MR-PRESSO method to ensure data accuracy. The specific parameters for MR-PRESSO were set to NbDistribution = 3000 and SignifThreshold = 0.05. We then excluded SNPs with p-values less than 0.05.
After meticulously processing the data and before proceeding with MR analysis, we also performed a heterogeneity test. Although data heterogeneity has minimal impact on the results, we used the IVW random effects model for MR analysis on heterogeneous SNPs (Q_pval < 0.05) and the IVW fixed effects model for non-heterogeneous SNPs. Additionally, regardless of the presence of heterogeneity, we analyzed the data using the MR-Egger and weighted median methods and calculated their OR values. To enhance the reliability of the results, we conducted a meta-analysis of the MR results for the 150 skin microbiome phenotypes with the two PCa groups and applied multiple corrections to the meta-analysis significance p-values using the Bonferroni correction method to reduce the likelihood of Type I errors.
Horizontal pleiotropy means that different treatments or interventions may have varying effects on different individuals or contexts, which could be mistakenly attributed to differences between the experimental and control groups rather than the actual treatment effect (25). To minimize the impact of horizontal pleiotropy on experimental results, we conducted horizontal pleiotropy testing on the GWAS data. We used MR-PRESSO to exclude SNPs exhibiting horizontal pleiotropy (P < 0.05), with the exclusion criteria set as NbDistribution = 3000 and SignifThreshold = 0.05. Heterogeneity refers to the diversity or variability among study subjects, observations, or experimental conditions (26). In statistics and research methodology, heterogeneity typically denotes differences among samples or individuals, which may stem from individual characteristics and environmental factors, including physiological and psychological conditions or other influences. Common forms of heterogeneity in research may manifest as physiological differences among individuals, variations in socioeconomic status, and the effects of environmental factors. This diversity and variability enhance the generalizability and representativeness of the research findings but also increase the complexity and difficulty of interpretation. During the analysis, we also conducted heterogeneity testing on the data. For SNPs exhibiting heterogeneity (Q-pval < 0.05), we performed MR analysis using a random-effects model within the IVW method. Otherwise, we employed a fixed-effects model to ensure the accuracy and reliability of the results (27).
The research process involved conducting MR analyses to assess the association between exposure and outcomes from two different sources, followed by a meta-analysis of the MR results and multiple corrections. Specifically, the study analyzed the relationship between 150 skin microbiome phenotypes and prostate cancer, utilizing MR analysis results from the UKB and FinnGen databases. The most significant IVW results from the MR analyses were subsequently subjected to meta-analysis using the meta package in R. We then applied the Bonferroni method for multiple corrections to the significant P-values from the meta-analysis to reduce the occurrence of Type I errors (28).
The meta-analysis approach has been previously developed and applied in MR research. For instance, a Mendelian Randomization study by Noordam et al. on the association between circulating antioxidants and the risk of coronary heart disease provided a detailed account of the MR analysis process using data on coronary heart disease outcomes from three different databases. The IVW results from these three analyses were subsequently meta-analyzed, and the final results did not support the hypothesis that circulating antioxidants have a protective effect against coronary heart disease (23).
Our positive results must meet the following criteria: 1) The meta-analysis results using the IVW method have an adjusted P-value less than 0.05 after multiple corrections; 2) The results of the IVW, weighted median, and MR-Egger methods are consistent in direction (same sign of β value); 3) There is no evidence of horizontal pleiotropy or heterogeneity. Subsequently, we used the skin microbiomes that yielded positive results as the outcomes and performed reverse analysis using PCa phenotypes from different sources as the exposures to test for potential reverse causality between them. By reducing the interference of reverse causality, this approach allows us to more accurately determine the direction of the causal relationship, thereby enhancing the credibility of the forward MR analysis.
After meticulous analysis and processing of the data, we identified 12,251 usable SNPs (results in Supplementary Table 2). Subsequently, we conducted an MR analysis using these SNPs for 150 skin microbiota and two sets of skin cancer data (results in Supplementary Table 3). A meta-analysis of the IVW results from the MR analysis was performed (results in Supplementary Table 4), with multiple corrections applied to the P values of the meta-analysis. Ultimately, we found a significant association between Neisseriaceae_Dry (GWAS ID: GCST90133217) and skin cancer, with Neisseriaceae_Dry acting as a protective factor (The visualized forest plot of the results is shown in Figure 2, and detailed results can be found in Supplementary Table 5).
It is noteworthy that among all the MR analyses and subsequent meta-analyses of skin microbiomes, eight taxa showed significant causal associations. Specifically, the P-value for Acinetobacter_Dry in the combined MR and meta-analysis was 0.045, ASV042_Dry had a P-value of 0.047, Bacteroides_Moist had a P-value of 0.020, Clostridiales_Incertae_Sedis_XI_Dry had a P-value of 0.028, Gammaproteobacteria_Moist had a P-value of 0.025, Neisseriaceae_Dry had a P-value of 0.0002, Rhodobacteraceae_Moist had a P-value of 0.046, and Streptococcus_Moist had a P-value of 0.0085. However, since these results had not been subjected to multiple corrections, the false positive rate could be significantly increased. Therefore, we applied Bonferroni correction to the above results. After correction, only Neisseriaceae_Dry showed a strong significant causal association, with a corrected P-value of 0.016.
The IVW results of the MR analysis for the prostate cancer phenotype in the FinnGen database with Neisseriaceae_Dry show an OR of 1.007 (95% CI: 0.999-1.014, P = 0.086). The scatter plot of the MR results is displayed in Figure 3, with the most strongly significant SNPs being: rs10516313, rs13075225, rs17605241, rs2095502, rs2422596, rs2731840, rs3017687, rs6025143, rs7143789, rs7512417, rs80196090 (detailed information can be found in Table 1). For the MR results between the prostate cancer phenotype in the FinnGen database and Neisseriaceae bacteria, the IVW method, MR-Egger method, and weighted median method all yielded significant positive β values and OR values greater than 1, suggesting that this trait may increase the risk of prostate cancer.
Table 1. Strongly significant SNPs in the MR effect of neisseriaceae_dry bacteria on prostate cancer phenotype in the FinnGen database.
The MR analysis of the prostate cancer phenotype in the UKB database with Neisseriaceae_Dry shows an OR of 1.011 (95% CI: 1.005-1.017, P = 0.001). The scatter plot of the MR results is displayed in Figure 4, with the most strongly significant SNPs being: rs10516313, rs11699213, rs12136471, rs13075225, rs17605241, rs2095502, rs3017687, rs4719814, rs476951, rs7143789, rs74443042, and rs7512417 (detailed information can be found in Table 2). For the MR results between the prostate cancer phenotype in the UKB database and Neisseriaceae bacteria, the IVW method, MR-Egger method, and weighted median method all yielded significant positive β values and OR values greater than 1, similarly indicating that this trait may increase the risk of prostate cancer.
Table 2. Strongly significant SNPs in the MR effect of neisseriaceae_dry bacteria on prostate cancer phenotype in the UKB database.
Subsequently, a meta-analysis of the results from these two outcome databases was conducted, and the P-values from the meta-analysis were corrected for multiple comparisons. The results showed an OR of 1.009 (95% CI: 1.004-1.014, P = 0.027), with the forest plot visualizing the results shown in Figure 2. Moreover, the analysis results of Neisseriaceae bacteria in the FinnGen and UK Biobank databases indicate a positive correlation with prostate cancer.
For the sensitivity analysis results of the positive outcomes, Cochran’s Q test did not reveal a Q_pval < 0.05, indicating no significant heterogeneity in our results (Supplementary Table 6). Additionally, in the above results, for SNPs with horizontal pleiotropy, the MR-PRESSO method removed outliers, and the results are shown in Supplementary Table 7.
We used reverse MR analysis to assess the causal effect of PCa on Neisseriaceae_Dry. The primary analytical methods included IVW, MR Egger, and weighted median methods to ensure the robustness and reliability of the results. Using Finngen R10 data, the IVW method showed no significant causal relationship between PCa and the abundance of Neisseriaceae (β = -0.096, SE = 0.305, P = 0.752, OR = 0.91, 95% CI = 0.50 - 1.65). The MR Egger analysis also showed no significant causal relationship between PCa and Neisseriaceae abundance (β = 0.007, SE = 1.170, P = 0.995, OR = 1.01, 95% CI = 0.10 - 9.99). The weighted median method indicated no significant causal relationship of PCa on Neisseriaceae abundance (β = -0.435, SE = 0.280, P = 0.121, OR = 0.65, 95% CI = 0.37 - 1.12). Using UKB data, the IVW method again showed no significant causal relationship between PCa and the abundance of Neisseriaceae (β = -0.072, SE = 0.504, P = 0.886, OR = 0.93, 95% CI = 0.35 - 2.50). The MR Egger analysis showed no significant causal relationship between PCa and Neisseriaceae abundance (β = 0.156, SE = 2.284, P = 0.948, OR = 1.17, 95% CI = 0.01 - 102.80). These results suggest that across the different statistical methods and datasets used, PCa does not have a significant causal effect on Neisseriaceae_Dry levels. (Supplementary Table 8).
In this study, we found a significant positive correlation between Neisseriaceae bacteria in dry skin and prostate cancer (PCa) through bidirectional MR analysis combined with meta-analysis. Specifically, the analysis results from the FinnGen and UKB databases showed that exposure to this bacterium was associated with an increased risk of prostate cancer. This association remained statistically significant after meta-analysis and Bonferroni correction.
Notably, only Neisseriaceae bacteria in dry skin environments showed a positive correlation with prostate cancer, whereas in moist and sebaceous gland environments, Neisseriaceae bacteria exhibited negative results. We hypothesize that the microbial composition of dry skin environments differs from that of moist or sebaceous gland-rich environments. The dry environment may promote the growth and proliferation of certain Neisseriaceae bacteria, making them more likely to interact with host cells and influence disease risk (12, 13). In moist or sebaceous gland-rich environments, other microorganisms may dominate, inhibiting the growth of Neisseriaceae bacteria (11). The diverse microbiome in these environments may limit the proliferation of Neisseriaceae bacteria and their potential pathogenic effects through competitive mechanisms (29).
Neisseriaceae bacteria in dry skin may influence the development of PCa through several potential biological mechanisms. Firstly, the regulation of the immune system may play a crucial role (29). Neisseriaceae bacteria in dry skin may affect local and systemic immune responses, stimulating immune cells in the skin and inducing the production of various cytokines and inflammatory mediators (30). These immune responses may further impact the overall immune status, including the prostate. Chronic low-grade inflammation over time may promote malignant transformation in prostate tissue (31, 32). Neisseriaceae bacteria may induce chronic inflammation in the skin and systemically through their metabolites or direct bacteria-cell interactions (17).
Secondly, the interaction between the microbiome and the endocrine system is also an important consideration. Skin microbiota may influence the endocrine system through complex pathways (33). Bacteria may be involved in regulating hormone levels in the skin and throughout the body (16). It is known that prostate cancer is closely related to androgen levels (e.g., testosterone) (34), and skin bacteria may indirectly affect the hormonal environment of the prostate. Additionally, the skin’s bacterial community may influence the metabolism of corticosteroids, which help regulate immune and inflammatory responses, thereby indirectly affecting the prostate’s health (17).
Bacterial metabolites may also play a role in this process. Neisseriaceae bacteria may produce certain metabolites that can reach the prostate through the bloodstream and have direct pro-cancer or anti-cancer effects on its cells (35, 36). For example, certain lipids or short-chain fatty acids may have pro-cancer effects (37). Furthermore, microorganisms may compete with host cells for certain nutrients or metabolites, and the deficiency or excess of these substances may affect the growth and proliferation of prostate cells (38).
Finally, gene-environment interactions also need to be considered. Host genes may determine individual susceptibility to Neisseriaceae bacteria and the composition of the microbiome (39, 40). These genetic factors may also simultaneously influence the risk of prostate cancer. Gene-environment interactions establish a link between these two factors.
Our research process involved multi-center database validation, confirming the robustness of the findings with consistent results across different databases (FinnGen and UKB). Meta-analysis and Bonferroni correction were used to ensure statistical significance, reducing the likelihood of false positives and obtaining reliable research results. However, further experimental and biological studies are necessary to validate the conclusions, including in vitro cell experiments, animal models, and clinical studies. Our study provides a foundation and direction for understanding and further exploring the relationship between Neisseriaceae bacteria in dry skin and prostate cancer. Through bidirectional MR analysis combined with meta-analysis, we found a significant positive correlation between Neisseriaceae bacteria in dry skin and PCa.
The study reveals a potential mechanism linking Neisseriaceae bacteria in dry skin to a significant positive association with prostate cancer, offering new perspectives for the prevention, early intervention, and treatment of prostate cancer. Based on these findings, future research should further investigate the specific biological mechanisms underlying the association between the skin microbiome and cancer, particularly how it may promote the development and progression of prostate cancer through immune and metabolic pathways. Additionally, in light of this study’s findings, future clinical research could assess the feasibility of modifying the skin microbiome to prevent or treat prostate cancer. Moreover, research should also expand to other diseases potentially influenced by the skin microbiome to explore whether similar associations exist. This could not only enhance our understanding of the skin microbiome’s crucial role in overall health but also lead to the discovery of novel biomarkers and the development of innovative therapeutic strategies.
Our study employed rigorously selected IVs to conduct a bidirectional two-sample MR analysis, investigating the relationships between 150 skin microbiota and PCa. We performed a meta-analysis of the results derived from PCa data obtained from two different sources to enhance the robustness of our findings. Additionally, we applied the Bonferroni method for multiple corrections. These methods help reduce confounding factors and reverse causality, thereby increasing the credibility of the results. However, due to the stringent experimental protocols, this approach has certain limitations and may miss some associations. It is particularly important to note that MR analysis reflects lifelong genetic susceptibility rather than short-term effects, so caution should be exercised in clinical inference. Furthermore, our genetic data are all derived from European ancestry, which may limit the applicability of our conclusions to other ancestries. Therefore, further research is needed to confirm whether these findings apply to other populations. We plan to conduct future studies involving multi-ethnic cohorts to supplement our research and further validate our conclusions.
The study identified a correlation between Neisseria in dry skin, one of the 150 skin microbiomes, and the risk of developing PCa, establishing it as a risk factor for increased susceptibility to PCa. However, it should be noted that this association requires further clinical validation.
The original contributions presented in the study are included in the article/Supplementary Material. Further inquiries can be directed to the corresponding author.
Participants in FinnGen provided informed consent for biobank research on basis of the Finnish Biobank Act. Alternatively, separate research cohorts, collected before the Finnish Biobank Act came into effect (in September 2013) and the start of FinnGen (August 2017) were collected on the basis of study-specific consent and later transferred to the Finnish biobanks after approval by Fimea, the National Supervisory Authority for Welfare and Health. Recruitment protocols followed the biobank protocols approved by Fimea. The Coordinating Ethics Committee of the Hospital District of Helsinki and Uusimaa (HUS) approved the FinnGen study protocol (number HUS/990/2017). The FinnGen study is approved by the THL (approval number THL/2031/6.02.00/2017, amendments THL/1101/5.05.00/2017, THL/341/6.02.00/2018, THL/2222/6.02.00/2018, THL/283/6.02.00/2019 and THL/1721/5.05.00/2019), the Digital and Population Data Service Agency (VRK43431/2017-3, VRK/6909/2018-3 and VRK/4415/2019-3), the Social Insurance Institution (KELA) (KELA 58/522/2017, KELA 131/522/2018, KELA 70/522/2019 and KELA 98/522/2019) and Statistics Finland (TK-53-1041-17).The Biobank Access Decisions for FinnGen samples and data utilized in FinnGen Data Freeze 5 include the following datasets: THL Biobank BB2017_55, BB2017_111, BB2018_19, BB_2018_34, BB_2018_67, BB2018_71, BB2019_7, BB2019_8 and BB2019_26; Finnish Red Cross Blood Service Biobank 7.12.2017; Helsinki Biobank HUS/359/2017; Auria Biobank AB17-5154; Biobank Borealis of Northern Finland_2017_1013; Biobank of Eastern Finland 1186/2018; Finnish Clinical Biobank Tampere MH0004; Central Finland Biobank 1-2017; and Terveystalo Biobank STB 2018001. The UK Biobank Resource under Application Number 24983, “Generating effective therapeutic hypotheses from genomic and hospital linkage data” (http://www.ukbiobank.ac.uk/wp-content/uploads/2017/06/24983-Dr-Manuel-Rivas.pdf). Based on the information provided in Protocol 44532 the Stanford IRB has determined that the research does not involve human subjects as defined in 45 CFR 46.102(f) or 21 CFR 50.3(g). All participants of UK Biobank provided written informed consent (more information is available at https://www.ukbiobank.ac.uk/2018/02/gdpr/). For FinnGen, all patients and control subjects provided informed consent, a biobank research consent, based on the Finnish Biobank Act. Recruitment protocols followed the biobank protocols approved by Valvira, the National Supervisory Authority for Welfare and Health. The Ethical Review Board of the Hospital District of Helsinki and Uusimaa approved the FinnGen study protocol Nr HUS/990/2017. All participants signed informed consent to study protocols approved by the Sardinian Regional Ethics Committee (protocol no. 2171/CE). All studies were approved by local ethics committees, and all participants provided written, informed consent. Informed consent was obtained from all participants and/or their LAR. This study was conducted in accordance to relevant guidelines and regulations.
DC: Writing – original draft, Visualization, Validation, Supervision, Software, Resources, Project administration, Methodology, Investigation, Funding acquisition, Formal analysis, Data curation, Conceptualization. SH: Writing – original draft, Visualization, Validation, Software, Resources, Project administration, Investigation, Funding acquisition, Formal analysis, Conceptualization. XW: Writing – original draft, Validation, Supervision, Software, Methodology, Funding acquisition, Formal analysis, Conceptualization. ZC: Writing – original draft, Validation, Resources, Project administration, Funding acquisition, Data curation, Conceptualization. WX: Writing – review & editing, Writing – original draft, Visualization, Software, Resources, Methodology, Investigation, Funding acquisition, Formal analysis, Data curation, Conceptualization.
The author(s) declare that no financial support was received for the research, authorship, and/or publication of this article.
We thank FinnGen, GWAS Catalog, and UKB for providing the data used in this study and acknowledge the contributions of all the authors of this paper.
The authors declare that the research was conducted in the absence of any commercial or financial relationships that could be construed as a potential conflict of interest.
All claims expressed in this article are solely those of the authors and do not necessarily represent those of their affiliated organizations, or those of the publisher, the editors and the reviewers. Any product that may be evaluated in this article, or claim that may be made by its manufacturer, is not guaranteed or endorsed by the publisher.
The Supplementary Material for this article can be found online at: https://www.frontiersin.org/articles/10.3389/fimmu.2024.1463309/full#supplementary-material
Supplementary Table 1 | STROBE-MR checklist.
Supplementary Table 2 | Instrumental Variable Screening Results.
Supplementary Table 3 | Results of the MR Analysis.
Supplementary Table 4 | Results of Meta-Analysis of all Skin Microbiomes Traits.
Supplementary Table 5 | Results of the Meta-Analysis.
Supplementary Table 6 | Results of Cochran’s Q test.
Supplementary Table 7 | Results of Horizontal pleiotropy.
Supplementary Table 8 | Results of the reverse MR analysis.
MR, Mendelian randomization; CI, confidence interval; OR, odds ratio; IVW, Inverse variance weighted; GWAS, genome-wide association study; SNPs, single nucleotide polymorphisms; IVs, Instrumental variables; UKB, UK Biobank; RCTs, randomized controlled trials; PCa, prostatic cancer; PSA, prostate-specific antigen.
1. Siegel DA, O'Neil ME, Richards TB, Dowling NF, Weir HK. Prostate cancer incidence and survival, by stage and race/ethnicity - United States, 2001-2017. MMWR Morb Mortal Wkly Rep. (2020) 69:1473–80. doi: 10.15585/mmwr.mm6941a1
2. Ferlay J, Colombet M, Soerjomataram I, Dyba T, Randi G, Bettio M, et al. Cancer incidence and mortality patterns in Europe: Estimates for 40 countries and 25 major cancers in 2018. Eur J Cancer. (2018) 103:356–87. doi: 10.1016/j.ejca.2018.07.005
3. Siegel RL, Miller KD, Fuchs HE, Jemal A. Cancer statistics, 2022. CA Cancer J Clin. (2022) 72:7–33. doi: 10.3322/caac.21708
4. Chan JM, Stampfer MJ, Giovannucci EL. What causes prostate cancer? A brief summary of the epidemiology. Semin Cancer Biol. (1998) 8:263–73. doi: 10.1006/scbi.1998.0075
6. Lavallée LT, Manuel DC, van Walraven C. Survival of men with prostate cancer undergoing radical prostatectomy in Ontario. J Urol. (2014) 192:1385–9. doi: 10.1016/j.juro.2014.04.087
7. Colombel M, Mallame W, Abbou CC. Influence of urological complications on the prognosis of prostate cancer. Eur Urol. (1997) 31 Suppl 3:21–4. doi: 10.1159/000474556
8. Cormie P, Newton RU, Taaffe DR, Spry N, Galvão DA. Exercise therapy for sexual dysfunction after prostate cancer. Nat Rev Urol. (2013) 10:731–6. doi: 10.1038/nrurol.2013.206
9. Jimenez-Andrade JM, Mantyh WG, Bloom AP, Ferng AS, Geffre CP, Mantyh PW. Bone cancer pain. Ann N Y Acad Sci. (2010) 1198:173–81. doi: 10.1111/j.1749-6632.2009.05429.x
10. Proksch E, Brandner JM, Jensen JM. The skin: an indispensable barrier. Exp Dermatol. (2008) 17:1063–72. doi: 10.1111/j.1600-0625.2008.00786.x
11. Byrd AL, Belkaid Y, Segre JA. The human skin microbiome. Nat Rev Microbiol. (2018) 16:143–55. doi: 10.1038/nrmicro.2017.157
12. Oh J, Byrd AL, Park M, Kong HH, Segre JA. Temporal stability of the human skin microbiome. Cell. (2016) 165:854–66. doi: 10.1016/j.cell.2016.04.008
13. Grice EA, Segre JA. The skin microbiome. Nat Rev Microbiol. (2011) 9:244–53. doi: 10.1038/nrmicro2537
14. Schommer NN, Gallo RL. Structure and function of the human skin microbiome. Trends Microbiol. (2013) 21:660–8. doi: 10.1016/j.tim.2013.10.001
16. Sanford JA, Gallo RL. Functions of the skin microbiota in health and disease. Semin Immunol. (2013) 25:370–7. doi: 10.1016/j.smim.2013.09.005
17. Belkaid Y, Hand TW. Role of the microbiota in immunity and inflammation. Cell. (2014) 157:121–41. doi: 10.1016/j.cell.2014.03.011
18. Zitvogel L, Daillère R, Roberti MP, Routy B, Kroemer G. Anticancer effects of the microbiome and its products. Nat Rev Microbiol. (2017) 15:465–78. doi: 10.1038/nrmicro.2017.44
19. Cavarretta I, Ferrarese R, Cazzaniga W, Saita D, Lucianò R, Ceresola ER, et al. The microbiome of the prostate tumor microenvironment. Eur Urol. (2017) 72:625–31. doi: 10.1016/j.eururo.2017.03.029
20. Davidsson S, Mölling P, Rider JR, Unemo M, Karlsson MG, Carlsson J, et al. Frequency and typing of Propionibacterium acnes in prostate tissue obtained from men with and without prostate cancer. Infect Agent Cancer. (2016) 11:26. doi: 10.1186/s13027-016-0074-9
21. Bowden J, Davey Smith G, Burgess S. Mendelian randomization with invalid instruments: effect estimation and bias detection through Egger regression. Int J Epidemiol. (2015) 44:512–25. doi: 10.1093/ije/dyv080
22. Chalitsios CV, Tsilidis KK, Tzoulaki I. Psoriasis and COVID-19: A bidirectional Mendelian randomization study. J Am Acad Dermatol. (2023) 88:893–5. doi: 10.1016/j.jaad.2022.10.019
23. Luo J, le Cessie S, van Heemst D, Noordam R. Diet-derived circulating antioxidants and risk of coronary heart disease: A mendelian randomization study. J Am Coll Cardiol. (2021) 77:45–54. doi: 10.1016/j.jacc.2020.10.048
24. Kurki MI, Karjalainen J, Palta P, Sipilä TP, Kristiansson K, Donner KM, et al. FinnGen provides genetic insights from a well-phenotyped isolated population. Nature. (2023) 613:508–18. doi: 10.1038/s41586-022-05473-8
25. Verbanck M, Chen CY, Neale B, Do R. Detection of widespread horizontal pleiotropy in causal relationships inferred from Mendelian randomization between complex traits and diseases. Nat Genet. (2018) 50:693–8. doi: 10.1038/s41588-018-0099-7
26. Bowden J, Holmes MV. Meta-analysis and Mendelian randomization: A review. Res Synth Methods. (2019) 10:486–96. doi: 10.1002/jrsm.v10.4
27. Li P, Wang H, Guo L, Gou X, Chen G, Lin D, et al. Association between gut microbiota and preeclampsia-eclampsia: a two-sample Mendelian randomization study. BMC Med. (2022) 20:443. doi: 10.1186/s12916-022-02657-x
28. Curtin F, Schulz P. Multiple correlations and Bonferroni's correction. Biol Psychiatry. (1998) 44:775–7. doi: 10.1016/S0006-3223(98)00043-2
29. Costello EK, Lauber CL, Hamady M, Fierer N, Gordon JI, Knight R. Bacterial community variation in human body habitats across space and time. Science. (2009) 326:1694–7. doi: 10.1126/science.1177486
30. Naik S, Bouladoux N, Wilhelm C, Molloy MJ, Salcedo R, Kastenmuller W, et al. Compartmentalized control of skin immunity by resident commensals. Science. (2012) 337:1115–9. doi: 10.1126/science.1225152
31. De Marzo AM, Platz EA, Sutcliffe S, Xu J, Grönberg H, Drake CG, et al. Inflammation in prostate carcinogenesis. Nat Rev Cancer. (2007) 7:256–69. doi: 10.1038/nrc2090
32. Sfanos KS, De Marzo AM. Prostate cancer and inflammation: the evidence. Histopathology. (2012) 60:199–215. doi: 10.1111/j.1365-2559.2011.04033.x
33. Chen YE, Fischbach MA, Belkaid Y. Skin microbiota-host interactions. Nature. (2018) 553:427–36. doi: 10.1038/nature25177
34. Huggins C, Hodges CV. Studies on prostatic cancer. I. The effect of castration, of estrogen and androgen injection on serum phosphatases in metastatic carcinoma of the prostate. CA Cancer J Clin. (1972) 22:232–40. doi: 10.3322/canjclin.22.4.232
35. Rooks MG, Garrett WS. Gut microbiota, metabolites and host immunity. Nat Rev Immunol. (2016) 16:341–52. doi: 10.1038/nri.2016.42
36. Jobin C. Precision medicine using microbiota. Science. (2018) 359:32–4. doi: 10.1126/science.aar2946
37. Schwabe RF, Jobin C. The microbiome and cancer. Nat Rev Cancer. (2013) 13:800–12. doi: 10.1038/nrc3610
38. Devkota S, Chang EB. Nutrition, microbiomes, and intestinal inflammation. Curr Opin Gastroenterol. (2013) 29:603–7. doi: 10.1097/MOG.0b013e328365d38f
39. Goodrich JK, Davenport ER, Waters JL, Clark AG, Ley RE. Cross-species comparisons of host genetic associations with the microbiome. Science. (2016) 352:532–5. doi: 10.1126/science.aad9379
Keywords: skin microbiomes, prostate cancer, bidirectional Mendelian randomization, meta-analysis, genome-wide association study
Citation: Chen D, Hu S, Wang X, Chen Z and Xu W (2024) Causal relationship between 150 skin microbiomes and prostate cancer: insights from bidirectional mendelian randomization and meta-analysis. Front. Immunol. 15:1463309. doi: 10.3389/fimmu.2024.1463309
Received: 11 July 2024; Accepted: 30 August 2024;
Published: 25 September 2024.
Edited by:
Bikul Das, KaviKrishna Laboratory, IndiaReviewed by:
Mangala Hegde, Indian Institute of Technology Guwahati, IndiaCopyright © 2024 Chen, Hu, Wang, Chen and Xu. This is an open-access article distributed under the terms of the Creative Commons Attribution License (CC BY). The use, distribution or reproduction in other forums is permitted, provided the original author(s) and the copyright owner(s) are credited and that the original publication in this journal is cited, in accordance with accepted academic practice. No use, distribution or reproduction is permitted which does not comply with these terms.
*Correspondence: Wanxian Xu, MjAyMjE1NzJAa21tdS5lZHUuY29t
Disclaimer: All claims expressed in this article are solely those of the authors and do not necessarily represent those of their affiliated organizations, or those of the publisher, the editors and the reviewers. Any product that may be evaluated in this article or claim that may be made by its manufacturer is not guaranteed or endorsed by the publisher.
Research integrity at Frontiers
Learn more about the work of our research integrity team to safeguard the quality of each article we publish.