- 1Department of Microbiology and Immunology, Faculty of Pharmacy, University of Sadat City, Sadat City, Egypt
- 2Department of Microbiology and immunology, Faculty of Pharmacy, Sinai University – Kantara Branch, Ismailia, Egypt
- 3Cell Biology, Histology & Genetics Division, Biology Department, Faculty of Science, Zagazig University, Zagazig, Egypt
- 4Medical Laboratory Department, College of Applied Medical Sciences, Taibah University, Yanbu, Saudi Arabia
- 5Biology Department, Faculty of Science, Taibah University, Yanbu, Saudi Arabia
- 6Department of Pharmaceutical Sciences, Pharmacy Program, Batterjee Medical College, Jeddah, Saudi Arabia
- 7Pharmacology Department, Faculty of Veterinary Medicine, Suez Canal University, Ismailia, Egypt
- 8Pathology Department, College of Medicine, King Khalid University, Abha, Saudi Arabia
- 9Department of Child Health, College of Medicine, King Khalid University, Abha, Saudi Arabia
- 10Anatomy Department, College of Medicine, King Khalid University, Abha, Saudi Arabia
- 11Department of Histology and Cell Biology, College of Medicine, Zagazig University, Zagazig, Egypt
- 12College of Clinical Laboratory Sciences, King Saud bin Abdulaziz University for Health Sciences, Jeddah, Saudi Arabia
- 13Department of Biotechnology, College of Sciences, Taif University, Taif, Saudi Arabia
- 14Department of Medicinal Chemistry, Faculty of Pharmacy, Suez Canal University, Ismailia, Egypt
- 15Department of Natural Products, Faculty of Pharmacy, King Abdulaziz University, Jeddah, Saudi Arabia
Background: A deep understanding of the causes of liability to SARS-CoV-2 is essential to develop new diagnostic tests and therapeutics against this serious virus in order to overcome this pandemic completely. In the light of the discovered role of antimicrobial peptides [such as human b-defensin-2 (hBD-2) and cathelicidin LL-37] in the defense against SARS-CoV-2, it became important to identify the damaging missense mutations in the genes of these molecules and study their role in the pathogenesis of COVID-19.
Methods: We conducted a comprehensive analysis with multiple in silico approaches to identify the damaging missense SNPs for hBD-2 and LL-37; moreover, we applied docking methods and molecular dynamics analysis to study the impact of the filtered mutations.
Results: The comprehensive analysis reveals the presence of three damaging SNPs in hBD-2; these SNPs were predicted to decrease the stability of hBD-2 with a damaging impact on hBD-2 structure as well. G51D and C53G mutations were located in highly conserved positions and were associated with differences in the secondary structures of hBD-2. Docking-coupled molecular dynamics simulation analysis revealed compromised binding affinity for hBD-2 SNPs towards the SARS-CoV-2 spike domain. Different protein–protein binding profiles for hBD-2 SNPs, in relation to their native form, were guided through residue-wise levels and differential adopted conformation/orientation.
Conclusions: The presented model paves the way for identifying patients prone to COVID-19 in a way that would guide the personalization of both the diagnostic and management protocols for this serious disease.
Introduction
The emergence of the novel coronavirus disease 2019 (COVID-19), which impacted global health deleteriously, has attracted worldwide attention in terms of fighting this highly transmissible virus (1). One basic point that is studied continuously with any novel infective agent is the fighting mechanism of the human immune system against this newly emerged pathogen and how this mechanism could affect the transmission and the complications (2). Human antimicrobial peptides (AMPs) have been extensively studied for their roles in fighting against several forms of pathogens such as bacteria and viruses where several trials have been performed to incorporate these peptides with antibiotics for fighting against bacteria (3). Generally, AMPs have demonstrated antiviral activity through different mechanisms where blocking the contact between the virus itself and the human cellular target represented a major mechanism. Human β-defensins (hBDs), a leading class of AMPs, were found in several mucosal sites to perform an innate immune defense mechanism against microbial colonization (4). Recently, this potential mechanism was studied specifically for hBD-2, which was able to bind to SARS-CoV-2 RBD and inhibit the binding of this RBD to hACE2, leading to the inhibition of the virus spreading, which pointed out the role of this AMP in fighting against COVID-19 (5). In addition, a recent study found that patients who developed severe COVID-19 had low serum levels of hBD-2 (6). Human cathelicidin LL‐37 is another example of AMPs that possess antimicrobial activity through the neutralization of the bacterial lipopolysaccharides (7). This AMP was also correlated with COVID-19 where it was found that the deficiency of vitamin D supplementation would negatively affect the level of LL-37 and allow for viral propagation (8). In addition, the high affinity of LL-37 to the RBD of this serious virus and its role in fighting against COVID-19 has been confirmed as well (9, 10).
Single-nucleotide polymorphism (SNP) is a gene-specific site variation that occurs in one base of the DNA nucleotide where it represents the most abundant form of human genetic variation (11). Generally, SNPs can be classified into different forms where missense SNPs, which are characterized by an amino acid substitution, can affect the development of different diseases as well as the human response to that disease progression (12, 13). This amino acid substitution may lead to the generation of a new mutated protein with structural and functional characteristics that largely deviated from the native one. Consequently, the downstream signals and the roles of the newly mutated protein would be significantly different from the original one, an approach that results in a novel way of disease development for cases that experienced that mutation (14, 15). The downstream effect of a specific SNP can range from the modification of a protein solubility or stability to the deregulation of transcription controlling proteins, which, in turn, affects the protein expression machinery in the cell (16).
Examples of the deleterious outcome of missense SNPs include the effects of SNPs in defensin genes on the liability to human immunodeficiency virus (HIV) infection (17), the effect of KRAS SNP on cell division and tumor progression (18), the role of rs4986790 SNP in the toll-like receptor 4 (TLR4) gene in patients’ vulnerability to HIV-1 infection (19), and the impact of AGER SNPs on diabetes complications and heart diseases (20). It is noteworthy that the continuous development in sequencing technology contributed largely to the elevation in the number of reported SNPs for many studied genes. While that development is advantageous, it became essential to study the implications of those reported SNPs and filter out the pathogenic ones from normal variants (21). As mentioned, the large number of reported SNPs makes it difficult to analyze through wet lab experiments and clinical studies, and alternatively, bioinformatics tools, which witnessed a great revolution in the last few years, make it feasible to access and filter a large number of reported SNPs and nominate the most deleterious ones in a timely and cost-effective method (22, 23). These in silico methods were widely used in recent years in various immunological and medical aspects (24–26). Moreover, the development in the structural biology field provides an effective way for altered protein structural prediction where the consequences of these structural modifications in the mutated protein can be assessed through molecular docking and molecular dynamics simulation approaches (27).
Hence, the current work aims to study the correlation between the deleterious SNPs of two AMPs, namely, hBD-2 and LL-37, and the propagation of COVID-19. We planned to retrieve the missense SNPs of these AMPs and nominate the most deleterious ones after a filtration process. Additionally, we investigated the functional and structural consequences of these SNPs and studied the correlation with the spread of our target virus, SARS-CoV-2. Altogether, these data would shed light on the mechanisms of the inter-individual susceptibility to this serious viral disease and contribute significantly to the field of personalized medicine in the fight against this deadly virus.
Materials and methods
General information
The retrieval of general information related to defensin beta 4A gene (DEFB4A) and cathelicidin antimicrobial peptide gene (CAMP) was performed using the National Center for Biotechnology Information (NCBI) database as well as the Ensembl database. Furthermore, Genecards.org’s database was utilized to retrieve information about gene ontology with compartments.jensenlab.org serving as the source for the data on the subcellular localization.
The retrieval of DEFB4A and CAMP gene variants
The retrieval of DEFB4A gene SNPs and CAMP gene SNPs was performed using NCBI databases depending on the variation viewer (https://www.ncbi.nlm.nih.gov/variation/view/) with the selection of dbSNP to be our source database. “DEFB4A” or 1673 [geneid] was the used entry with the DEFB4A gene. “CAMP” or 820 [geneid] was the used entry with the CAMP gene. The filtration of the retrieved variants was performed and the missense SNPs were solely chosen for the additional analysis.
Predicting the SNPs with the most deleterious impacts
Six various in silico tools were utilized to predict which SNPs have the most deleterious impact on protein function for both hBD-2 and LL-37. (1) SIFT (Sorting Intolerant from Tolerant) employs the sequence homology as well as the physical characteristics of amino acids to forecast how variations may affect protein function (https://sift.bii.a-star.edu.sg/) (28). (2) Polymorphism Phenotyping-2 (PolyPhen-2) assesses the effects of substituting amino acids depending on physical approaches as well as comparative techniques (http://genetics.bwh.harvard.edu/pph2) (29). (3) PROVEAN utilizes blast hits and generates the necessary score and prediction (http://provean.jcvi.org/seq_submit.php) (30). (4) SNPs&GO utilizes a functional annotation approach to determine the SNPs with harmful impacts (https://snps.biofold.org/snpsand-go/snps-and-go.html) (31). (5) PHD-SNP utilizes support vector machines (SVMs) to forecast how the novel phenotype resulting from the missense mutations is associated with human illnesses (http://snps.biofold.org/phd-snp/phd-snp.html) (32). (6) SNAP2 was also used with its distinct neural network’s capacity to distinguish between effect SNPs and the other neutral ones (https://rostlab.org/services/snap/) (33). These mutations, which were found to be deleterious by at least five tools, were designated as the most harmful ones. By combining these distinct tools with various methodologies and algorithms, we aimed at improving the precision of our investigation.
The analysis of the variants’ effects on the stability of the protein
I-Mutant 2.0 as well as Mu-Pro tools were utilized to investigate how the selected mutations affected the stability of the protein. I-Mutant 2.0 employs a support vector machine to forecast the direction and the magnitude of the change in the free energy (DDG) (https://folding.biofold.org/i-mutant/i-mutant2.0.html) (34). The ProTherm database, with its extensive experimental data on the change in free energy associated with the stability of proteins affected by the mutations, was utilized for testing I-Mutant 2.0 (35). Mu-Pro employs a robust support vector machine approach showing 84% accuracy when applied to the cross-validation and the verification process (http://mupro.proteomics.ics.uci.edu/) (36).
The recognition of the location of the SNPs on protein domains
InterPro was utilized to find the sites of the deleterious mutations on protein domains (https://www.ebi.ac.uk/interpro/). The functional analysis performed by InterPro could reveal the important domains and the key motifs of the chosen protein (37).
The investigation of the phylogenetic conservation of protein residues
Utilizing ConSurf, the phylogenetic conservation of the protein residues was examined (https://consurf.tau.ac.il). By analyzing the homologous sequences for the existing phylogenetic connections, ConSurf could examine the sequences of the designated protein for phylogenetic conservation (38, 39).
Secondary structure analysis
PSIPRED tool (http://bioinf.cs.ucl.ac.uk/psipred/) was utilized to analyze the secondary structure of the selected protein and determine the specific alignment for the altered amino acids in the examined secondary structure. Furthermore, the secondary structures in case of the damaging mutations were analyzed as well. PSIPRED could analyze the secondary structure related to a certain protein with the use of position-specific matrices produced by PSI-BLAST (40).
Analyzing the effects of our variants on the protein structure
Utilizing the HOPE bioinformatics server, the 3D structure of the designed protein could be examined (https://www3.cmbi.umcn.nl/hope/). HOPE utilizes numerous sources for gathering the relevant information along with the production of homology models with the help of the YASARA program in order to carry out the needed functions (41).
Molecular docking studies
Prior to molecular docking simulations, proteins including the native dimeric hBD-2 (PDB: 1FD3) as well as the constructed SNP-variant dimers were independently prepared through the removal of any bound ligands, crystalized solvent, as well as ionic metals/salts, in addition to subsequent protein protonation (42–45). Residues of hBD-2 were indexed starting from Gly24 to Pro64, since the first 23 amino acids are reported as a signal sequence being removed via the proteolytic advent of signal peptidases permitting the release and secretion of hBD-2 (46). Crystallized SARS-CoV-2 spike (PDB: 6m0j) was also prepared as described above to be only included within the subsequent molecular dynamics simulations serving as a positive control reference. Docking the native or variant hBD-2 dimers on the receptor-binding domain (RBD) of SARS-CoV-2 spike protein was performed using two online docking servers: ClusPro v2.0 (Boston and Brook Universities; https://cluspro.org/) (47–50) and ZDOCK v3.0.2 (Massachusetts University; https://zdock.umassmed.edu/) (51, 52).
Relying on the Fast Fourier Transform correlation protocol, the ClusPro server predicted the hBD-2/RBD complex through a multi-stage process including PIPER-based rigid docking, interaction energy-based conformational filtration, pose ranking based on clustering properties, and finally refinement through energy minimization (48, 53). Interaction energy adopted by ClusPro includes energy terms for van der Waals (Eatt + Erep), electrostatic (Eelec), and pairwise structure-dependent potentials (EDARS) resulting from Decoys as reference state, while it lacks entropic energy terms (48, 53, 54). In this regard, it was suggested to utilize cluster ranking, in terms of cluster populations where hBD-2 inbound with the SARS-CoV-2 RBD site was utilized for hACE2 association, rather than the obtained ClusPro interaction energies in order to rank and identify the best-clustered structure complex (5). Concerning docking with ZDOCK, the protocol was more specific since docking constraints were applied to define the spike RBD binding site with the residue range (Ser436-Tyr508) as it depicted high solvent accessibility and reported enrollment within the RBD/hACE2 complex association (5, 44, 55, 56).
Docking pose assessment and interface analysis
Evaluation of the best docking pose for each bound spike RBD/hBD-2 complex obtained from each docking server was proceeded through macromolecular interface analysis using the online PDBePISA v1.5.2 server tool (European Bioinformatics Institute/EMBL-EBI; https://www.ebi.ac.uk/msd-srv/prot_int/cgi-bin/piserver) (57, 58). This tool provided descriptions for the sole and bound protein interface such as interface residues, total solvent-accessible surface area (Å2), numbers/types of binding interactions, and the gained solvation free energy (ΔiG; kcal/mol), and its p-value (ΔiG p-value) (59). The last two descriptors are indices for higher interface hydrophobicity/protein affinity (high negative ΔiG values) and to how far would the protein–protein interface be interaction-specific (p < 0.5) (57).
Additional protein–protein interface analysis was performed using the Network Analysis of Protein Structures server (NAPS; International Institute of Information Technology, Hyderabad, India; https://bioinf.iiit.ac.in/NAPS/). This server adopts the network global analysis tool for representing the nodes and backbone edges, in addition to residue–residue contact plots between the hBD-2 and spike RBD monomeric units (60). Moreover, an estimation of the spike RBD/hBD-2 binding affinity for the predicted docked complex was performed using the Molecular Mechanics energy-guided Generalized Born and Surface Area (MM/GBSA; kcal/mol) calculations that are implemented at the HawkDock server (Zhejiang University; http://cadd.zju.edu.cn/hawkdock/) (61). The HawkDock MM/GBSA calculation permits an estimation of the energy term components including van der Waal, electrostatic, and polar solvation, in addition to the dissection of these energy terms down to the protein’s per-residue energy contributions (62, 63).
All-atom molecular dynamics simulations
Best docked spike RBD-associated complexes, for the native hBD-2 and each SNP variant, were subjected to 100 ns all-atom molecular dynamics simulations under CHARMM36m forcefield and using the GROMACS program (64). Protein complexes were solvated at the TIP3P cubic box under periodic boundary conditions, while maintaining the 10-Å minimum distance between the protein atoms and box boundaries. The net charge of the system was neutralized via sufficient 0.15 M sodium and chloride ions. Systems were subjected to the steepest-descent minimization for 0.05 ns, followed by two-staged equilibration at standard thermo- and barostats (Berendsen-temp for NVT ensembles, 1 ns at 310 K, followed by the Parrinello–Rahmann barostat for the NPT ensemble, 1 ns at 1 atm and 310 K) (43, 64). Molecular dynamics were run for 100 ns under the NPT ensemble and Particle-Mesh-Ewald algorithms for computing long-range electrostatic interaction (65). For comparison, the prepared spike RBD/ACE2 complex was used in the reference simulation run adopting the same conditions being detailed.
Trajectory analysis was performed using root-mean-square deviations (RMSDs; Å), the radius of gyration (Rg; Å), RMS-Fluctuations (RMSFs; Å), and solvent-accessible surface area (SASA; nm2) via the GROMACS in-house analysis scripts relying on the protein’s backbone alpha-carbon atoms. Visual Molecular Dynamics (VMD) software (Illinois University, v1.9.3, Urbana-Champaign, United States) was used for hydrogen bond analysis defining the hydrogen bond distance/angle cutoffs at 3.0 Å and 20°, respectively, and representing the time of formed particular hydrogen bond as % occupancy. Conformational analysis and visualization of the simulated complexes at specified timeframes were done using PyMOL software (Schrödinger; v2.0.6, United States).
The analysis of gene–gene interactions
By utilizing GeneMANIA, the network describing gene–gene interactions was produced (http://www.genemania.org). GeneMANIA could forecast the genes that have a strong interaction with a selected gene using a variety of resources and types of data (66).
Results
General information
Both DEFB4A and CAMP genes are protein-coding genes with NCBI Gene IDs of 1673 and 820, respectively. The DEFB4A gene is located at 8p23.1; it has two exons and a length of 2,040 nucleotides. There is one transcript for the DEFB4A gene (ensemble.org). The illustration of the subcellular localization of the DEFB4A gene is shown in Figure S1A (Compartments.jensenlab.org/), while the illustration of its gene ontology can be shown in Figure S1B (Genecards.org). In addition, the CAMP gene is located at 3p21.31; it has four exons and a length of 1,991 nucleotides. Moreover, the CAMP gene has two transcripts (ensemble.org). The illustration of the subcellular localization of the CAMP gene is shown in Figure S2A (Compartments.jensenlab.org/), while the illustration of its gene ontology can be shown in Figure S2B (Genecards.org).
Retrieving DEFB4A and CAMP gene variants
A total of 897 single-nucleotide variations could be detected in the DEFB4A gene (accessed 31 May 2022). Out of these SNPs, 52 were missense SNPs, 22 were synonymous SNPs, 611 were intron SNPs, 25 were in the 5′ untranslated region (UTR), and 30 were in the 3′ UTR, besides the other downstream and upstream variants. Meanwhile, for the CAMP gene, 831 single-nucleotide variations could be detected (accessed 27 May 2022). Out of these SNPs, 138 were missense SNPs, 78 were synonymous SNPs, 466 were intron SNPs, 7 were in the 5′ untranslated regions (UTR), and 22 were in the 3′ UTR, besides the other downstream and upstream variants.
Investigating the effect of the SNPs on hBD-2 function
For hBD-2, among the 52 missense SNPs, 24 missense SNPs were found in amino acid sequence from position 24 to position 64 that correspond to hBD-2 as the first 23 amino acids represent a signal peptide (https://www.uniprot.org/uniprot/O15263). These 24 missense SNPs were investigated for their effect on protein function using six in silico tools (SIFT, PolyPhen-2, SNAP, PROVEAN, PHD-SNP, and SNP&GO). Only three SNPs [rs1173728551 (P44L), rs867101800 (G51D), and rs1585735110 (C53G)] were found to be deleterious by at least five tools. Table 1 shows the detailed results for all these 24 SNPs in hBD-2 with the various used tools.
Investigating the effect of the SNPs on LL-37 function
For LL-37, 27 missense SNPs were found in amino acid sequence from position 134 to position 170 that correspond to the mature LL-37 (https://www.uniprot.org/uniprot/P49913). These 27 missense SNPs were investigated for their effect on protein function using six in silico tools (SIFT, PolyPhen-2, SNAP, PROVEAN, PHD-SNP, and SNP&GO). None of these SNP was found to be deleterious by at least five tools. Table S1 shows the detailed results for all these 27 SNPs in LL-37 with the various used tools.
The analysis of the variants’ effects on the stability of hBD-2
The analysis of the variants’ effects on the stability of hBD-2 was performed using the I-Mutant 2.0 tool in addition to the Mu-Pro tool. All the three SNPs were found to decrease the stability of hBD-2 by both I-Mutant 2.0 and Mu-Pro tools. Table 2 shows the detailed results and the values of the analysis.
The recognition of the location of the SNPs on hBD-2 domains
The analysis of hBD-2 by InterPro showed the existence of a domain called Beta/alpha-defensin, C-terminal domain (InterPro entry: IPR006080). The investigation of the locations of our three variants was performed, and the three SNPs were shown to be located in this domain (Table 3).

Table 3 Locations of the selected residues on hBD-2 domains, ConSurf conservation analysis, and predicted secondary structure.
The investigation of the phylogenetic conservation of hBD-2 residues
The residues of the hBD-2 protein were analyzed for their phylogenetic conservation using the ConSurf tool. Two SNPs (G51D and C53G) were found to be positioned in highly conserved locations while the other SNP (P44L) was found to be positioned in a variable location (Table 3).
Secondary structure analysis
The PSIPRED method was used for predicting the secondary structure of hBD-2 as shown in Figure 1A. The secondary structures at residues 44, 51, and 53 were found to be coil, strand, and strand, respectively, as shown in Table 3. Furthermore, the secondary structures of hBD-2 were predicted with our mutations as shown in Figure 1. On one side, P44L was not found to lead to changes in the secondary structure of hBD-2 (Figure 1B). On the other side, G51D and C53G mutations were found to be associated with differences in the secondary structures of HBD-2; both mutations were associated with loss of the strand structure at the end of the protein as shown in Figures 1C, D, respectively.
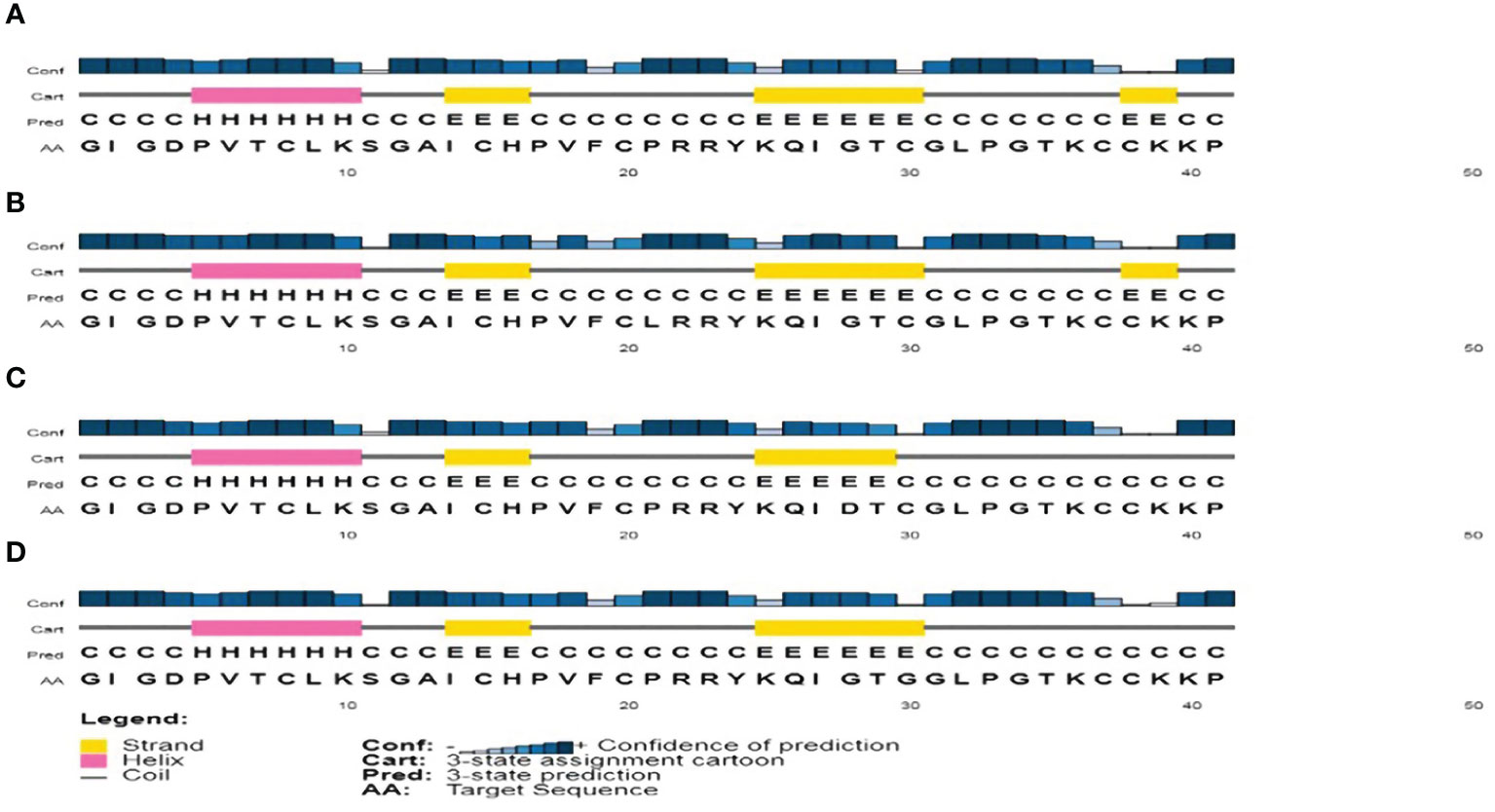
Figure 1 The analysis of secondary structure as produced by the PSIPRED tool. (A) The analysis of the secondary structure of hBD-2 (wild type). (B) The analysis of the secondary structure of hBD-2 with P44L SNP. (C) The analysis of the secondary structure of hBD-2 with G51D SNP. (D) The analysis of the secondary structure of hBD-2 with C53G SNP.
Analyzing the effects of our variants on hBD-2 protein structure
The analysis was extended to study the effects of our variants on hBD-2 protein structure using the HOPE tool. The structural effects in hBD-2 protein with the substitution of the amino acids are described in detail in Table 4. Figures 2A–C illustrate the replacement of the wild-type residue with the mutant one for P44L, G51D, and C53G, respectively.
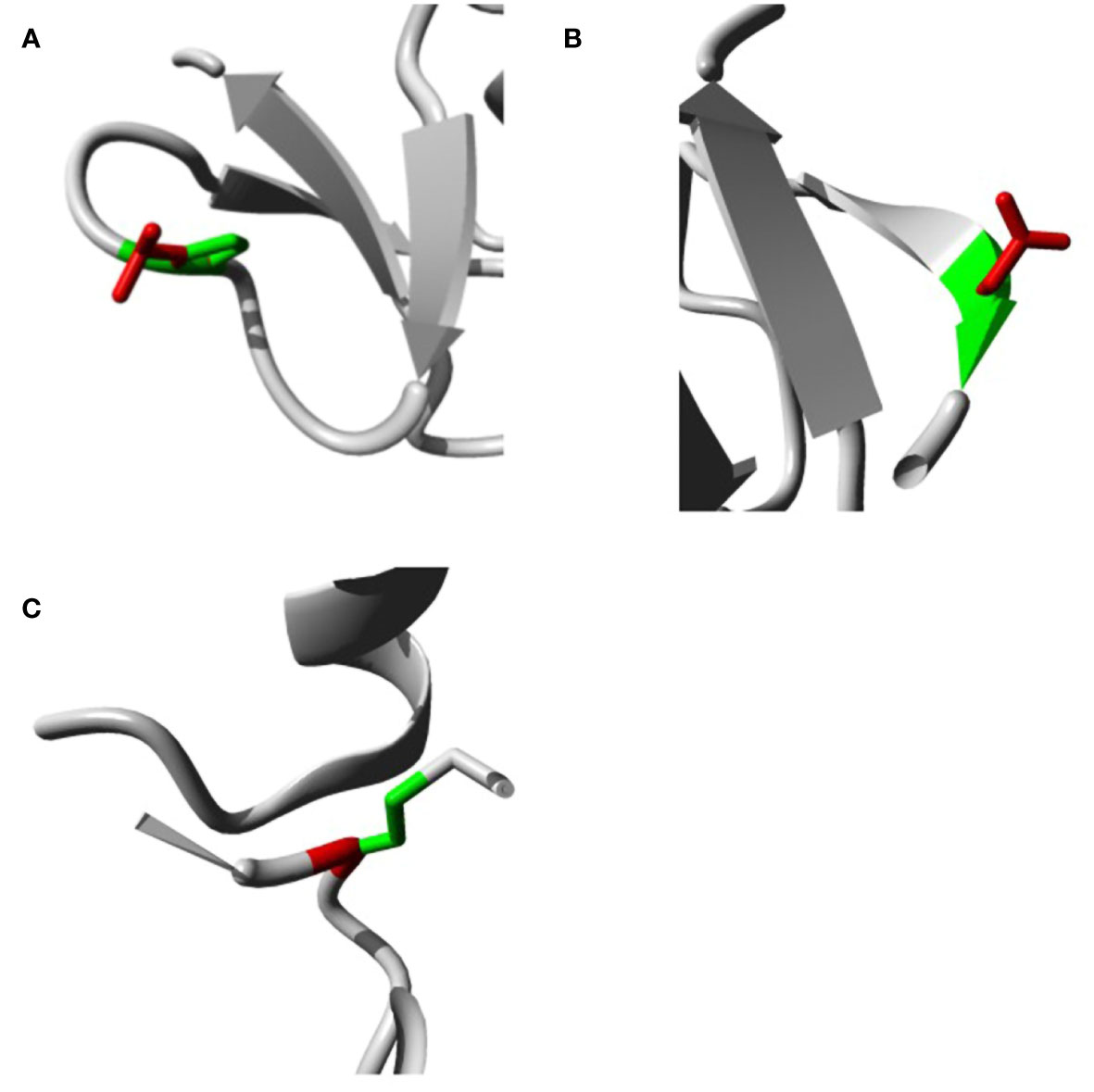
Figure 2 Impacts of SNPs on hBD2 3D structure as produced by the HOPE bioinformatics tool. (A) Impacts of SNPs on hBD-2 3D structure with P44L SNP. (B) Impacts of SNPs on the hBD-2 3D structure with G51D SNP. (C) Impacts of SNPs on the hBD-2 3D structure with C53G SNP.
Molecular Docking and binding pose prediction
Both employed docking servers illustrated relevant binding of the four different hBD-2 dimers (one native and three SNP variants; SNP1 to SNP3) at the spike RBD interface. For simplicity, the hBD-2 SNP-variant dimers, P44L, G51D, and C53G, would be referred to as SNP1, SNP2, and SNP3, respectively, within the forthcoming context. Examining the top complexes obtained from each server revealed comparable protein arrangement based on the RMSD alignment analysis with values of 0.780 Å, 0.730 Å, 0.934 Å, and 0.816 Å for the native, SNP1, SNP2, and SNP3 docked complexes, respectively (Figure 3). Analyzing the complex interfaces via PDBePISA illustrated a larger interface solvent-accessible area as well as interaction contacts (# hydrogen bonds and # salt bridges) for the complexes obtained from the ZDOCK server over those from ClusPro (Table 5). The depicted preferentiality of the ZDOCK-generated complex was reasonably translated into higher negative Δ1G scores as well as lower Δ1G p-value implying profound interaction specificity for the hBD-2 towards the spike RBD interface surface being of higher hydrophobicity than would-be-average for given structures. Based on the above findings, ZDOCK-obtained complexes were considered more significant for further interface evaluation and to be used for subsequent molecular dynamics simulation studies.
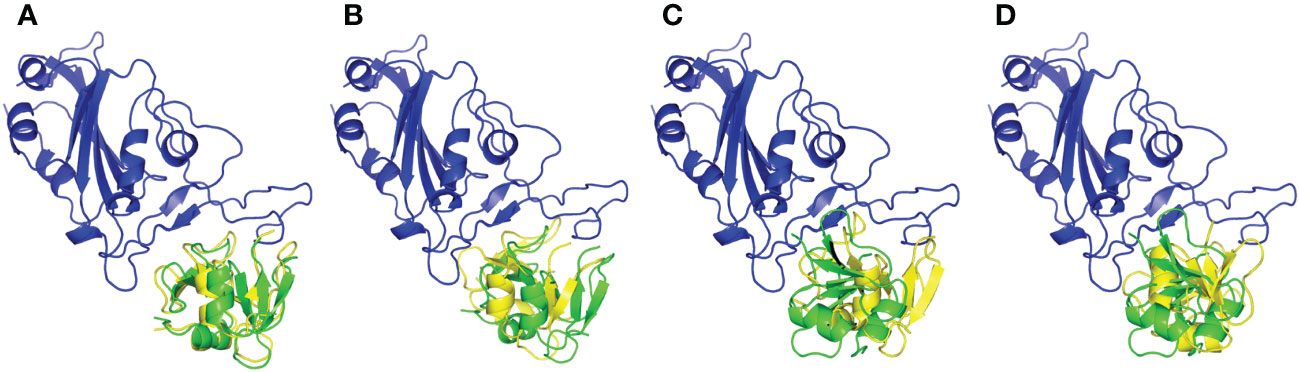
Figure 3 Aligned structures obtained from different docking servers for the docked hBD-2 dimeric forms at the SARS-CoV-2 RBD interface. Docked hBD-2 dimers generated from ClusPro (green cartoon) and ZDOCK (yellow cartoon) were aligned at the binding interface of the SARS-CoV-2 RBD (blue cartoon). (A) native hBD-2 model, (B) P44L hBD-2 mutant model SNP1, (C) G51D hBD-2 mutant model SNP2, and (D) C53G hBD-2 mutant model SNP3.
A general binding affinity trend has been illustrated with the ZDOCK-generated complexes where both ΔiG and # polar interface interactions were more favored for the binding affinity of the native hBD-2 dimer as compared to the SNP variants (Table 5). This trend was quite consistent with complex structural network analysis findings obtained from the NAPS server since # nodes (residues) and edges (bonds) were reduced with the SNP variants as compared to the native hBD-2 form (Figure 4; left panels). Applying 7 Å as the upper threshold and one residue for separation index, the estimated node:edge ratios were 282:1,149, 277:1,030, 276:1,116, and 277:1,120 for the native hBD-2, SNP1, SNP2, and SNP3 bound complexes, respectively. Generally, the reduction within the edge numbers would correlate to compromised protein–protein binding interactions as well as less favored complex stability (60, 67). Generated inter-/intra-molecular contact plots showed lower interaction patterns with the hBD-2 SNP variants in relation to those exhibited by the native congruent form (Figure 4; middle panels). These latter patterns were delineated by circle highlights placed on Figure 4 where these circle highlights show higher native spike RBD/hBD-2 molecular interactions as compared to those of SNP variants.
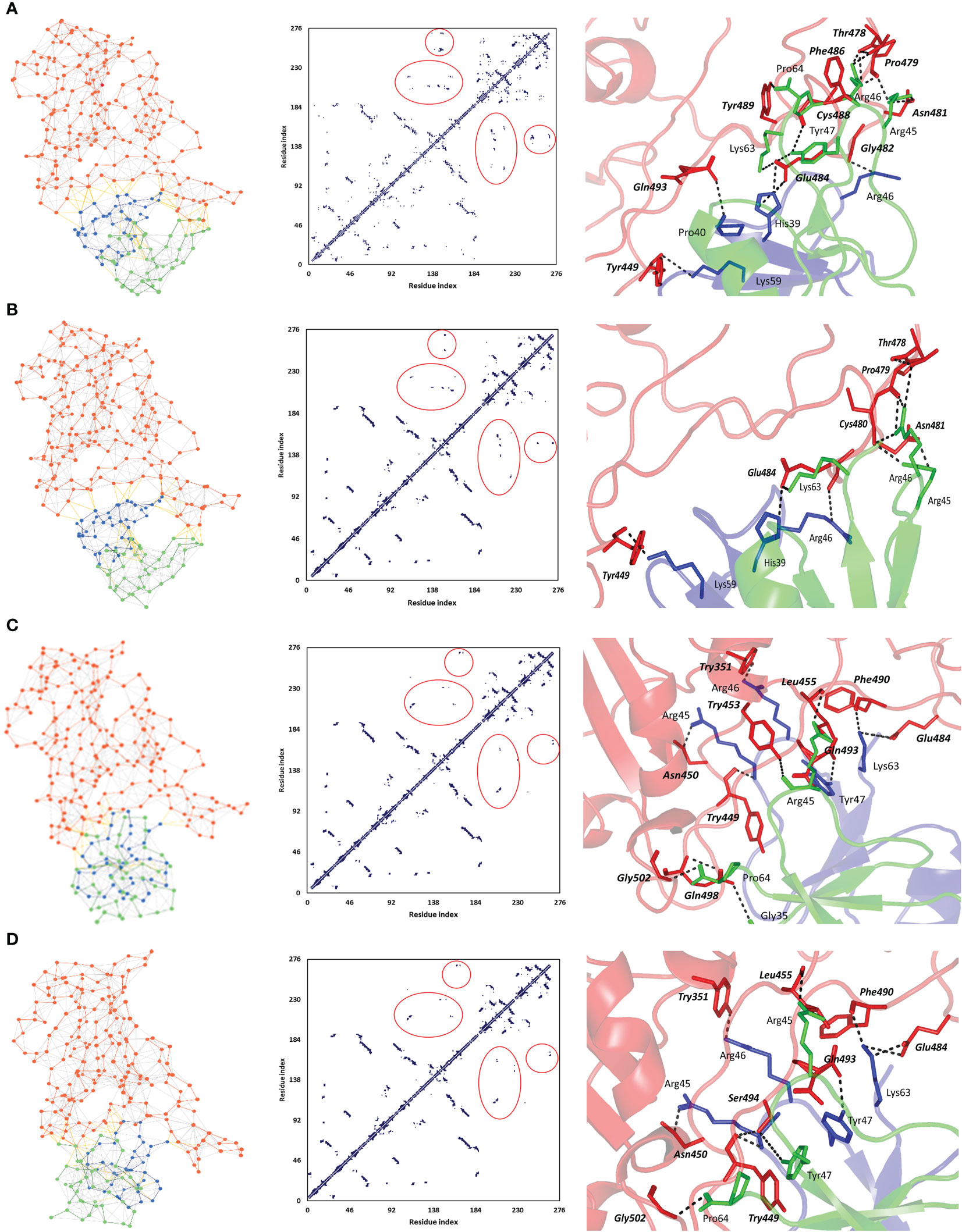
Figure 4 Interface analysis for the ZDOCK-obtained spike RBD/hBD-2 dimer complexes using NASP sever. (A) Native hBD-2 model, (B) SNP1 hBD-2 mutant model, (C) SNP2 hBD-2 mutant model, and (D) SNP3 hBD-2 mutant model. Left panels illustrate the network between edges (inter-/intra-molecular bonds as yellow and gray colors, respectively) and dots/nodes (amino acids; orange for spike RBD and blue/green for the hBD-2 dimers). The middle panels illustrate the contact plots for the residue–residue interactions between the monomeric units of spike RBD (residue index 1–194) and hBD-2 (residue index 195–276). Circle highlights are shown to pinpoint the contact interfacing residues between spike RBD and hBD-2 proteins, as well as hBD-2 protein monomers. Right panels illustrate the interface key polar interactions as predicted by the PDBePISA docking server. Protein backbones are depicted as a transparent cartoon colored red, blue, and green for spike RBD, hBD-2 protomer A, and hBD-2 protomer B, respectively. Key residue pairs are presented as lines, colored depending on their location within the proteins, and numbered according to their respective residue sequence. Spike RBD residues are presented in bold and italic. Bonds of polar interactions are black dashed lines.
Key binding residues at the interface between the spike RBD and investigated hBD-2 dimeric forms are shown in Figure 4 (right panels). Several common polar residues involved in spike RBD binding with native and SNP1 hBD-2 interface include spike Thr478, pro479, Asn481, and Glu484, which mediated hydrogen bond interactions with hBD-2 residues His39, Arg45, Arg46, and/or Tyr47 belonging to either protomer A, protomer B, or both (Table 6; spike residue will be written in italic formate from here forward). On the other hand, amino acids such as Tyr351, Asn450, Tyr453, Phe490, Gln493, and Gly502 were significant for both SNP2 and SNP3 hBD-2 complex stabilities as they furnished hydrogen bonding with Arg45, Arg46, Tyr47, Lys63, and Pro64 of either one or both protomers. Interestingly, binding with spike Tyr449 was common for all four complex interactions where it mediated strong polar hydrogen bond interactions with Lys59 of native and SNP1 hBD-2 proteins. On the other hand, spike Tyr449 was significant for Arg45–hydrogen bond association at SNP2 and SNP3 proteins. The latter spike–Tyr449 direct polar interactions were directed towards the hBD-2 protomer A chain, rather than the protomer B one. Moreover, hBD-2 protomer A Arg46 and protomer B Arg45 and Pro64 amino acids were the most frequent residues involved with spike RBD interface interaction and binding. As these residues were in close proximity to SNP residues (P44L, G51D, and C53G), this could highlight the influence of these mutated residues on the conformation of their neighboring key interacting residues and, thus, in turn, the binding pose, orientation, and affinity of the simulated hBD-2.
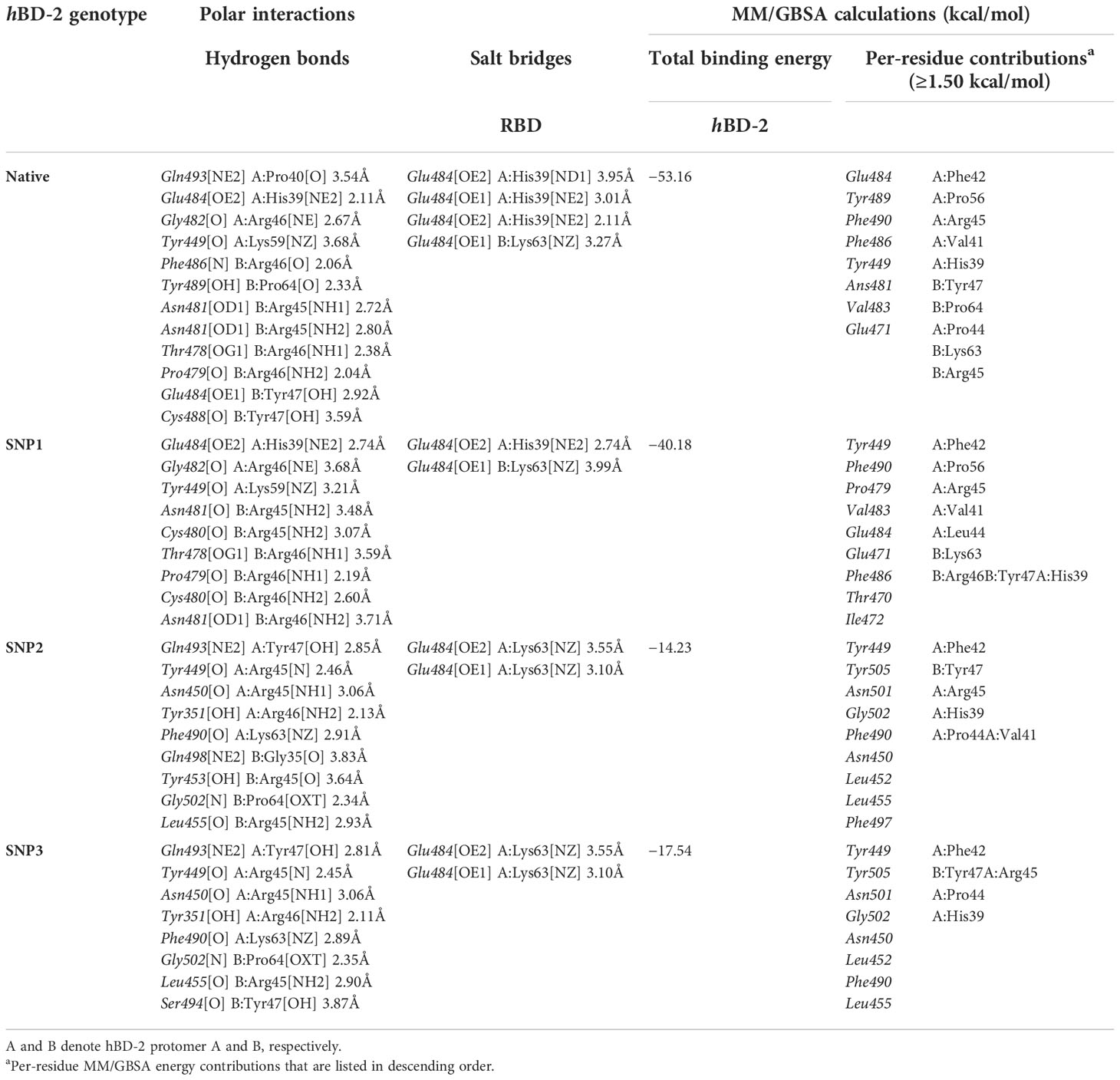
Table 6 Key interface per-residue polar interactions via PDBePISA and predicted MM/GBSA binding energies.
On similar bases, multiple salt bridges between spike Glu484 at one side and His39 and Lys63 of either hBD-2 protomer were almost consistent across the four ZDOCK-generated docked complexes. Besides polar interacting residues, hydrophobic interface residues at spike RBD showed significant closeness and relevant non-polar contacts with neighboring hBD-2 amino acids. Spike residues such as Val483, Phe490, Leu452, and Ile472 depicted ≤5.0 Å distances from the respective Tyr47, Phe42, and Val41 amino acids at native and SNP1 hBD-2 protomers. Notably, spike Val583 showed hydrophobic contact with native Pro44 and its SNP1 variant residue, Leu44, with a much closer distance toward the earlier native residue (3.9 Å versus 4.5 Å αC distance). Regarding both SNP2 and SNP3 variants, residues such as Pro64, Phe42, His39, Ile37, Tyr47, and Val41 illustrated potential hydrophobic interaction with Ile472, Val483, Tyr489, Tyr449, Tyr505, and/or Val445 of the spike protein. For translating all the above preferential per-residue interactions into respective binding energy terms, the MM/GBSA binding energy calculations were applied for the top four spike RBD/hBD-2 complexes. Higher binding energy was assigned for the native hBD-2 complex as compared to its SNP variants. As expected, higher residue-wise energy contributions were assigned for the key interacting residues of both spike RBD and hBD-2 proteins (Table 6). Focusing on the mutant hBD-2 SNP residues, the residues were of lower negative energy contributions than their native amino acids (Pro44 −2.39 kcal/mol vs. Leu44 −1.35 kcal/mol in SNP1; Gly51 −0.13 kcal/mol vs. Asp51 0.21 kcal/mol in SNP2; Cys53 −0.14 kcal/mol vs. Gly53 0.02 kcal/mol in SNP3). Interestingly, mutant residues at SNP2 and SNP3 depicted positive repulsive energy contributions rather than favored attractive ones.
All-atom molecular dynamics simulation and thermodynamic stability
The RMSD trajectories of both spike RBD and hBD-2 proteins were monitored across the 100-ns all-atom simulation runs in reference to the alpha-carbon atoms (αC) of their respective initial structure. The spike RBD αC-RMSD showed deferential tones based on the ligand-bound protein (Figure 5A). The steadiest RMSD tones were depicted for the RBD in complex with the native hBD-2 ligand as compared to any other simulated RBD proteins. Despite limited fluctuations around 25- to 35-ns timeframes, native-bound RBD was maintained around an average RMSD value (2.49 ± 0.59 Å) for more than half the simulation time. On the other hand, RBD bound with hBD-2 SNP variants were at higher RMSD values (SNP1 3.09 ± 0.65 Å; SNP2 8.27 ± 3.23 Å; SNP3 3.75 ± 1.32 Å) with shown fluctuations across the simulation times. Across different hBD-2/RBD models, the highest fluctuations were assigned to the SNP2-bound RBD reaching up to 11.25 Å at the end of the simulation run.
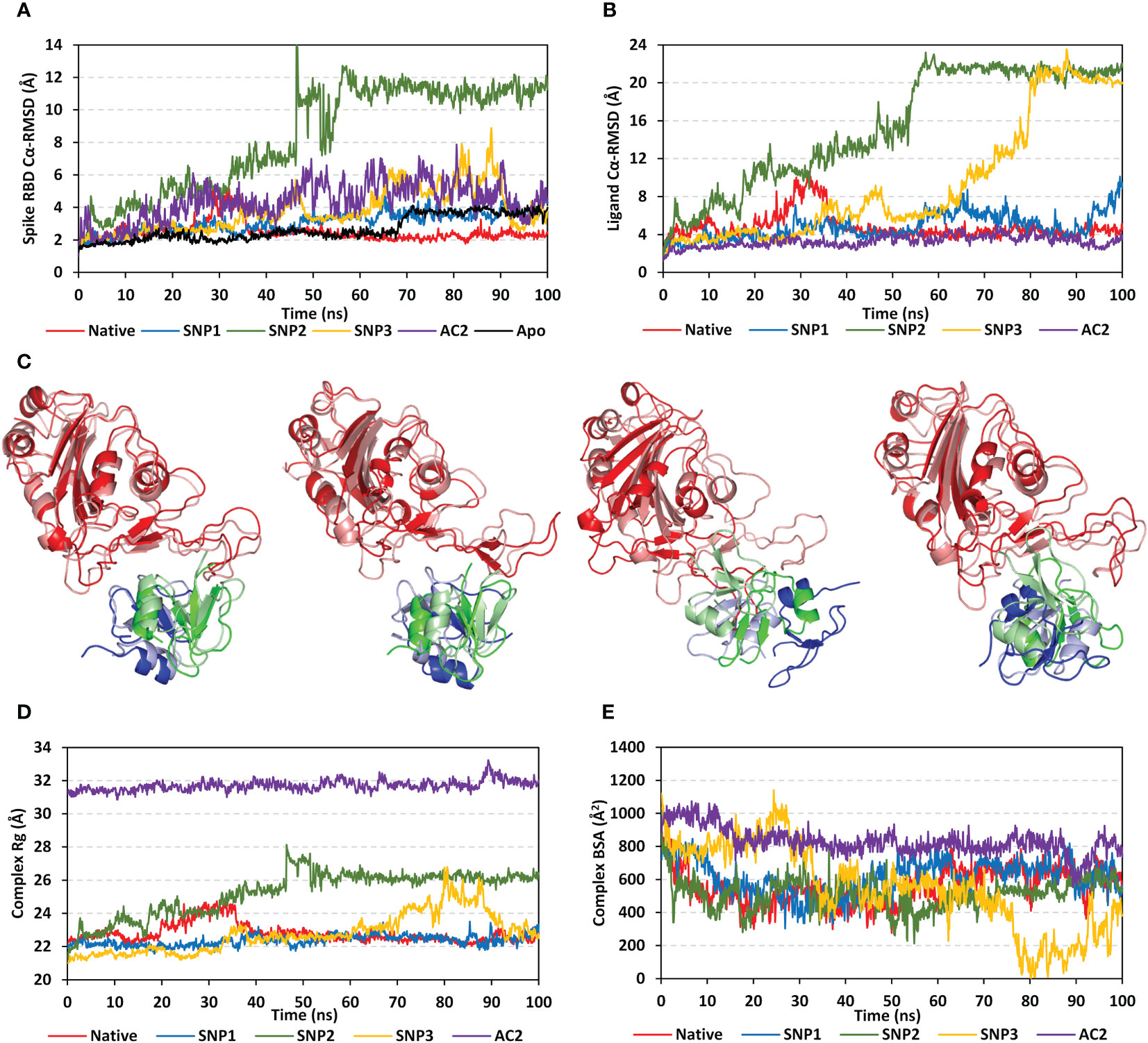
Figure 5 Stability analysis of the simulated spike RBD/ligand complexes across the molecular dynamics simulations. (A) Spike RBD Cα-atom RMSDs; (B) ligand Cα-atom RMSDs, as a function of the simulation times (ns). (C) Overlaid spike RBD/hBD-2 complex snapshots at 0 and 100 ns for native, SNP1, SNP2, and SNP3 ligand proteins from the left to the right side, respectively. Proteins are represented as cartoons and colored red, blue, and green for respective spike RBD, hBD-2 protomer A, and hBD-2 protomer B domains. Initial and final extracted frames were represented in faint or dark colors, respectively. (D) Complex Rgs; (E) Complex BSAs, as a function of the simulated times (ns).
Interestingly, the RMSD trajectories of spike RBD in complex with the crystallized hACE2 protein showed higher and more fluctuating tones (4.34 ± 1.17 Å) as compared to the native hBD-2 model. Moreover, the unliganded/apo spike RBD protein was of steady RMSD trajectories till 70 ns (2.16 ± 0.30 Å), after which they significantly increased and leveled off around 3.74 Å till the end of the simulation run reaching higher values than the native hBD-2/bound RBD. Monitoring the ligand RMSDs of either the bound hBD-2 or hACE2 proteins has shown comparable patterns in regard to their respective bound spike RBD proteins (Figure 5B). Both the native hBD-2 dimer and hACE2 protein units depicted the steadiest tones for more than 50-ns run times (4.24 ± 0.39 Å and 3.59 ± 0.51 Å, respectively). Higher fluctuating RMSD tones were assigned for SNP hBD-2 dimers showing SNP2 with the highest fluctuating values, followed by SNP3 and SNP1 proteins (15.39 ± 6.04 Å, 9.26 ± 6.39 Å, and 4.82 ± 1.35 Å, respectively). Notably, the αC-RMSD tones of bound ligand proteins (hBD-2) were almost 1.5-fold higher than those of their bound spike RBD proteins, except for hACE2 where the latter showed steadier RMSD tones than its bound spike RBD protein.
Conformational analysis through aligning the starting and final spike RBD/hBD-2 complex structures illustrated differential orientations for the bound ligand at the spike RBD interface (Figure 5C). Visually observed as well as correlated to the obtained RMSD trajectories, limited conformational and ordination changes were observed for the spike RBD/native hBD-2 complex in regard to its SNP variant models. A slight conformational shift was depicted for the native hBD-2 location and secondary structure showing minimal rotations of its flexible loops (aligned RMSD 1.44 Å). The SNP1 variant showed significant conformational rotation at almost 90° in regard to its initial structure, yet the whole protein was maintained at its same starting location (aligned RMSD 1.93 Å). The SNP1 conformational changes were associated with significant conformational alteration of the spike interface loop (residue range Ala475–Cys488) adopting a more elongated hairpin-like conformation. On the contrary, a more dramatic conformational and orientational shift was assigned to the SNP2 protein showing a significant drift of ~20 Å far from the spike RBD interface and towards the solvent side (aligned RMSD 8.85 Å). Such dramatic alterations caused the spike RBD interface loop (residues Ser469–Pro491) to adopt a different orientation being directed towards the solvent side in a way that appeared to track the drifted SNP2 protein. Regarding the last hBD-2 variant, SNP3 ligand protein was persistent at the spike interface, yet with inverted orientation (180° rotation) regarding its whole structure. Being dimeric, a moderate aligned RMSD value of 1.65 Å was assigned for the SNP3-bound system. Notably, these conformational changes were with minimal influence on the spike RBD conformation where the interface loop showed limited rotations at the end of the simulation being of a similar fashion to that observed for RBD in complex with native hBD-2 protein.
Tracking the above conformational changes was pursued through estimating the Rgs of spike RBD/hBD-2 complexes in relation to their gathered central masses. Following half of the simulation runs, both native and SNP1-bound complexes were of the lowest steady Rg tones (22.79 ± 0.57 Å and 22.36 ± 0.32 Å) till the end of the timeframes (Figure 5D). This was not consistent with the other simulated hBD-2 SNP variants, where SNP2 depicted a continuous uprising of Rg values from 20 to 50 ns before it leveled off at approximately 26.00 Å till reaching 100 ns. The SNP3-bound complex depicted significant Rg fluctuations up to 26.00 Å across the 70- to 90-ns timeframes. Concerning Rgs of the spike RBD/hACE2 complex, steady but higher tones (31.70 ± 0.31 Å) were depicted across the whole simulation run the thing that would be consistent with the hACE2 large mass (~110 kDa). Further conformational analysis was done by monitoring the amount of solvent-accessible surface areas being buried at the interface between both bound proteins across the simulation times.
Buried surface area (BSA; Å2) for each simulated complex was estimated using the following equation adopting the individual SASA of each bound protein (SASARBD and SASAhBD-2) as well as that of the whole bound complex; BSA = 0.5*(SASARBD + ASAhBD-2 – SASAcomplex) (5). Simulated spike RBD/hACE2 complex showed the highest BSAs fluctuating around an average value of 831.55 ± 81.85 Å2, which was consistent with its larger size as compared to the investigated hBD-2 proteins (Figure 5E). Steady comparable BSA values were depicted for the simulated native and SNP1 hBD-2 complex systems (~600 Å2). Notably, both systems illustrated increased BSA beyond the 50-ns time window where they reached few hundreds just below the BSA of the RBD/hACE2 complex. On the other hand, the SNP2 variant complex was seen with greater fluctuations at initial frames and ended with a low BSA average (502.61 ± 82.27 Å2) across the last 40-ns timeframes, indicating fewer areas being covered. Much higher BSA fluctuations were depicted with the SNP3 system reaching down to the lowest BSA values (~8.00 Å2) around the 80 ns before the BSA values were raised to 738.15 Å2 at the end of the simulation. Findings from RMSDs, Rgs, and BSAs conferred that SNP3 left the spike RBD interface across the 80 to 90 ns and finally returned to its initial location, where it adopted an inverted orientation.
For highlighting the differential contact interfaces for the simulated hBD-2 proteins towards their bound spike RBD target, the per-residue occupancy for each protein across the run frames was estimated, where residues achieving contact distances of ≤5.5 Å were highlighted and visually represented (Figure 6A). Interestingly, higher contact distance occupancies were assigned for the native hBD-2 dimer depicting more red-colored residues as compared to the SNP proteins. Highlighted residues (Val41, Pro44, Arg45, Arg46, Pro56, and Gly57) from native dimeric units depicted high contact distance occupancies towards two main spike RBD interface regions (I: Leu441–Asn450 and II: Gly476–Phe486). The SNP1 variant residues (Val41, Phe42, Arg45, Tyr47, and Pro56) depicted relevant contact distance occupancies with both spike RBD interface residues, yet with lower occupancy values. On other hand, residues of SNP2 protein (Lys33, Val41, Phe42, Pro56, Gly57, Lys59, and Lys63), mostly at its protomer B, depicted preferential contact distance occupancies with the spike RBD interface region II. Such SNP2 preferential contact pattern is consistent with the dramatic conformational/orientation shift across the simulation run being illustrated via the above dynamic analysis parameters. Despite depicting relevant contacts with the two main spike RBD interface regions, residues of the SNP3 protein (Val41, Phe42, Pro44, and Arg45) as well as contacting spike ones illustrated fair contact distance occupancies, which emphasized the ligand drift from the RBD interface throughout the simulation run.
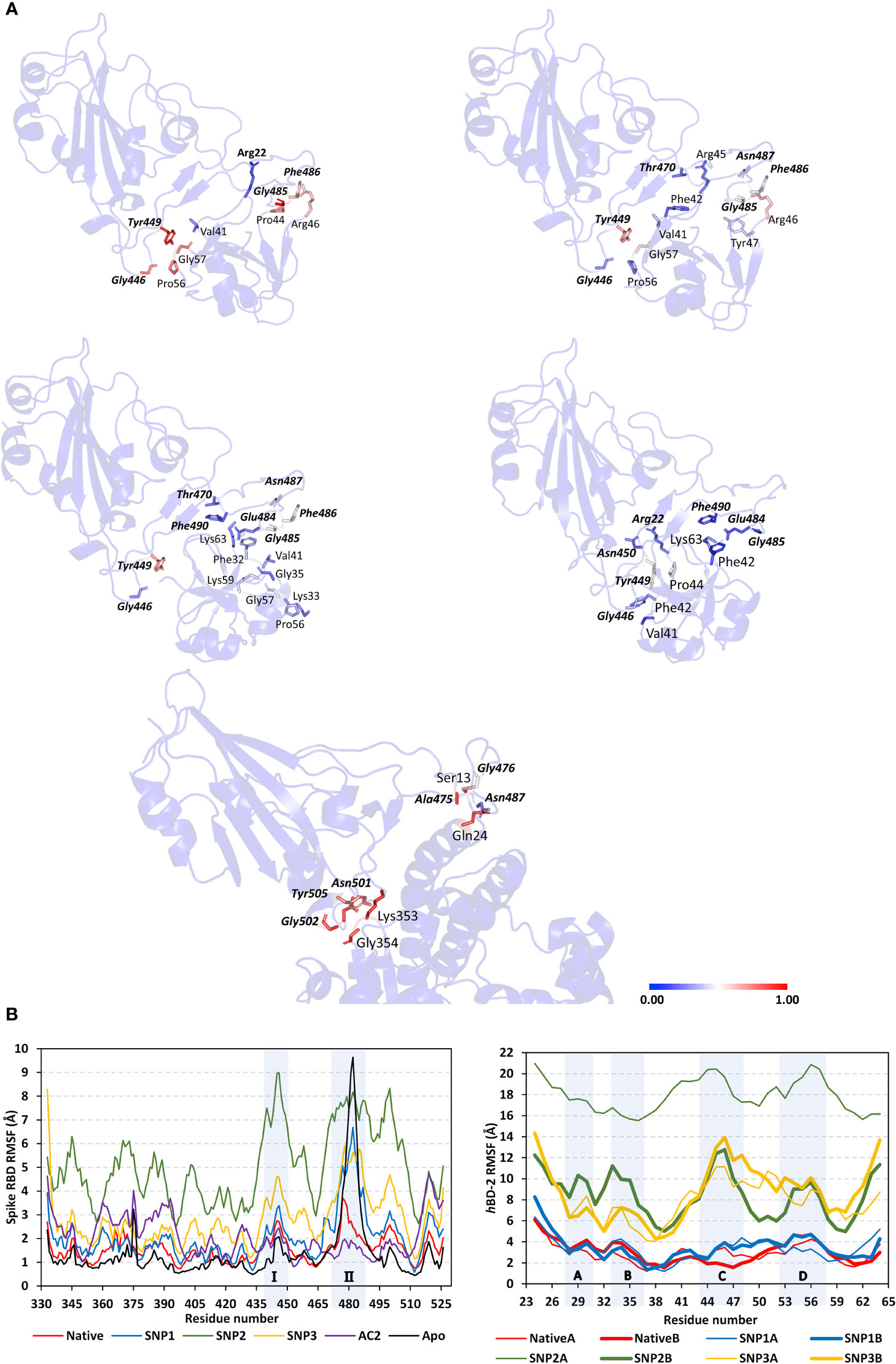
Figure 6 Dynamics of the simulated spike RBD/ligand complexes across the molecular dynamics simulations. (A) Per-residue contact distance occupancy analysis. Complexes of native hBD-2, SNP1, SNP2, SNP3, and hACE2 (upper left, upper right, middle left, middle right, and bottom panels, respectively) are represented as cartoons highlighting residues (sticks) with relevant occupancies exhibiting contact distance at ≤5.50 Å from bound protein. Data are mapped to the residue sticks with a spectrum color bar indicating the range of 0.00 to 1.00, transitioning through 0.50 occupancies (blue, white, and red, respectively). Highlighted residues are numbered according to their respective residue sequence, while spike RBD residues are presented in bold and italic. (B) Spike RBD and hBD-2 RMSF of individual protein residues in respect to their C-alpha, as a function of the simulated times (ns). Shaded regions of spike RBD and hBD-2 residues are common regions for binding the two proteins and are thoroughly described in the above context.
Spike interface residue Tyr449 was shown to be significant for hBD-2 ligand binding as it depicted one of the highest occupancies, being the highest for native hBD-2, throughout each respective simulated complex. Notably, all simulated hBD-2 systems depicted negligible contact distance occupancies towards the anti-parcel β-sheets and the connecting loops (Tyr451–Lys458 and Pro491–Gly496) at the spike RBD interface region being present midway between the spike’s two high occupancy regions I and II. Concerning the contact distance occupancies for the spike RBD/hACE2 complex, preferential binding towards the RBD regional interface residues was also depicted with negligible values for the midway anti-parcel β-sheets. Nevertheless, some subtle contacting residue shifts were shown with hACE2 where the RBD residues Ala475–Thr478 and Asn501–Tyr505, rather than Leu441–Asn450 and Gly476–Phe486, respectively, were associated with hACE2 and not with hBD-2 dimers. Higher occupancies were for the hACE2-associated regions Asn501–Tyr505 of the bound spike RBD, while Leu441–Asn450 residues are more significant for hBD-2 binding.
Thermodynamic behaviors of different hBD-2 and hACE2 in relation to spike RBD protein were further evaluated down to their constituting residue levels via monitoring each respective RMSF fluctuation tones. As expected, the above-described contact residue ranges were of lower RMSFluctuations only for native and hACE2-bound RBD proteins rather than those in complex with the hBD-2 SNP mutants (Figure 6B). The highest RBD RMSF tones were assigned for binding with SNP2, followed by SNP3 and SNP1, while those inbound to native hBD-2 and hACE2 were of almost comparable immobility tones. On the other hand, differential RMSF tones were depicted for the contact interface residue ranges between the most stable holo RBD (i.e., in complex with native hBD-2 or hACE2) and those being unliganded at their apo state. The high-occupancy contact residue range Gly476–Phe486 was of higher flexibility (up to 9.50 Å) at its apo state as compared to those inbound with native hBD-2 or hACE2 (~3.00 Å). On the contrary, the contact residue range Leu441–Asn450 and Asn501–Tyr505 were of more immobility patterns at the apo state as compared to the holo ones (~1.30 Å versus ~2.10 Å, respectively). Nevertheless, the holo/apo RMSF differences within the two latter spike RBD residue ranges were of a lower extent (0.5-fold) as compared to those obtained at the Gly476–Phe486 residue range (>2-fold). Interestingly, only the spike RBD Asn501–Tyr505 residue range was with less flexibility RMSF tones on hACE2 bounding than either the native hBD-2-bound or even apo spike proteins. The latter highlights the significance of the spike RBD Ala475–Thr478 residue for hACE2 rather than hBD-2 binding. Moving towards the RMSF of the simulated hBD-2 proteins, higher values were assigned for the simulated SNP2 and SNP3 hBD-2 mutant proteins (Figure 6B).
Comparable RMSF trajectories were depicted for each protomer of the hBD-2 dimer structure, except for the SNP2 variant where its protomer A was assigned with the highest observed RMSF values (17.95 ± 1.59 Å) among all hBD-2 monomeric units and even those of bound spike RBD proteins. Notably, four hBD-2 core residue regions (A: Asp27–Cys31, B: Lys33–Ile37, C: Cys43–Lys48, D: Gly54–Thr58) showed higher RMSF tones as compared to the remaining core residues of each respective protein. The first two regions (A and B) correspond to the protein’s N-terminal α-helix and β-loop secondary structures where their depicted RMSFs were much higher in SNP2 and SNP3 variants as compared to both SNP1 and native forms. Both site A and site B were observed far from contact with the spike RBD site throughout the simulation time conferring their negligible role in hBD-2/RBD stability and in turn their respective high RMSFs. On the other hand, the last two hBD-2 regions (C and D) showed significant contact with the spike RBD site; however, high contact distance occupancies were only assigned for the native and SNP1 simulations (see Figure 6A). The latter could reasonably explain why sites C and D were of lower RMSFs at native and SNP1 proteins as compared to the other variants.
The MM/PBSA calculations revealed negative average free binding interaction energies for the native hBD-2/spike RBD complex being only second to the hACE2 simulated system (Figure 7). The average free binding energies of SNP mutant proteins were almost half that of the spike RBD/hACE2 system. Dissecting the total free energy into its constituting energy terms revealed a dominant contribution for Coulomb’s electrostatic potentials over those of the hydrophobic ones. Notably, the polar solvation penalty was significantly higher in mutant SNPs as compared to the native protein, the thing that could have compromised the mutant complex stabilities since binding is considered a solvent-displacement process. Among the simulated hBD-2 proteins, the native form was depicted with the highest electrostatic energy contributions, which were further illustrated by monitoring the number of formed hydrogen bonds between the proteins across the simulation runs (Figure 8A).
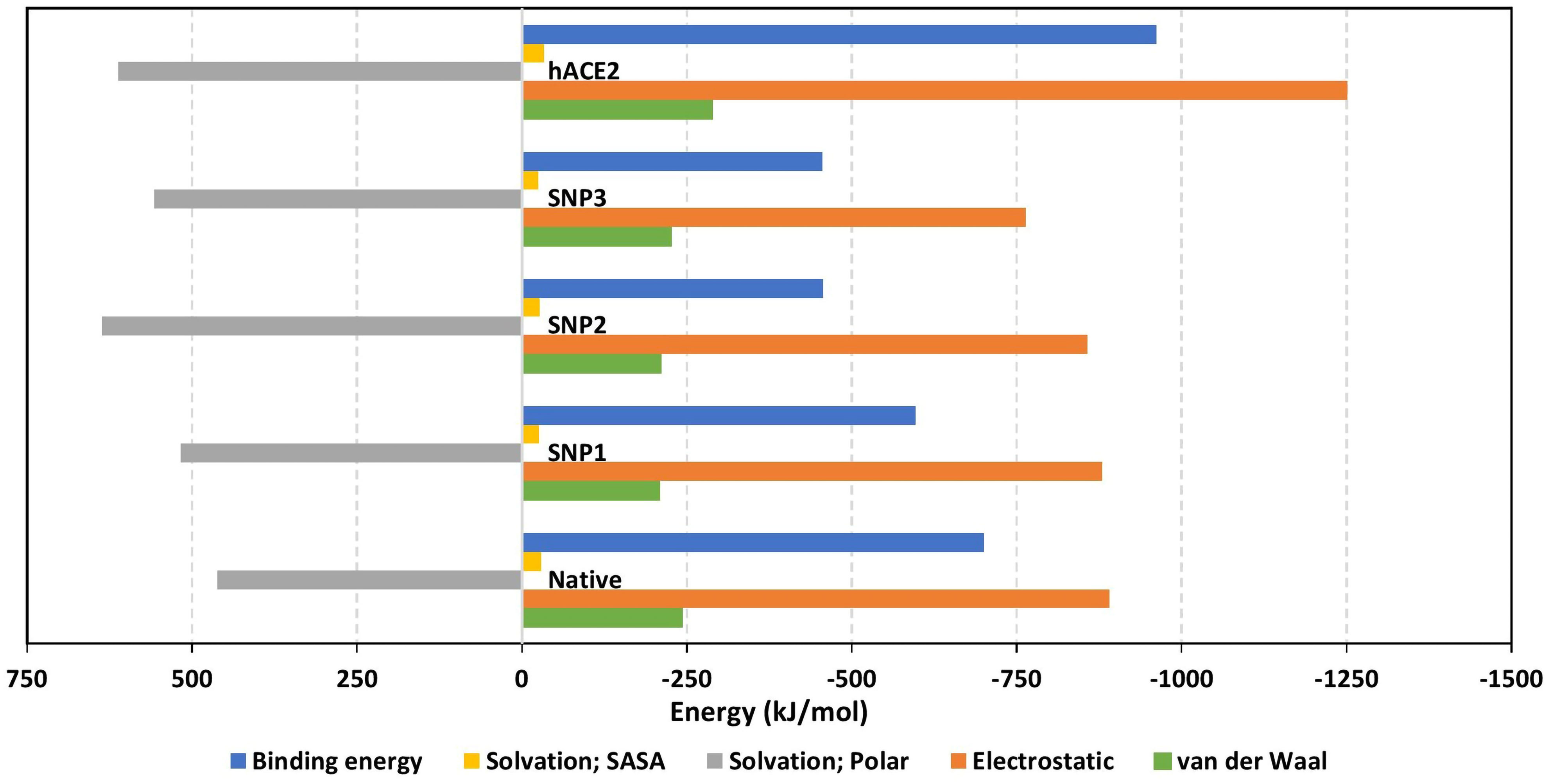
Figure 7 Free binding and individual energy terms for the simulated spike RBD/ligand complexes. Values are estimated in terms of kJ/mol ± SE; error bars are hidden for clarity.
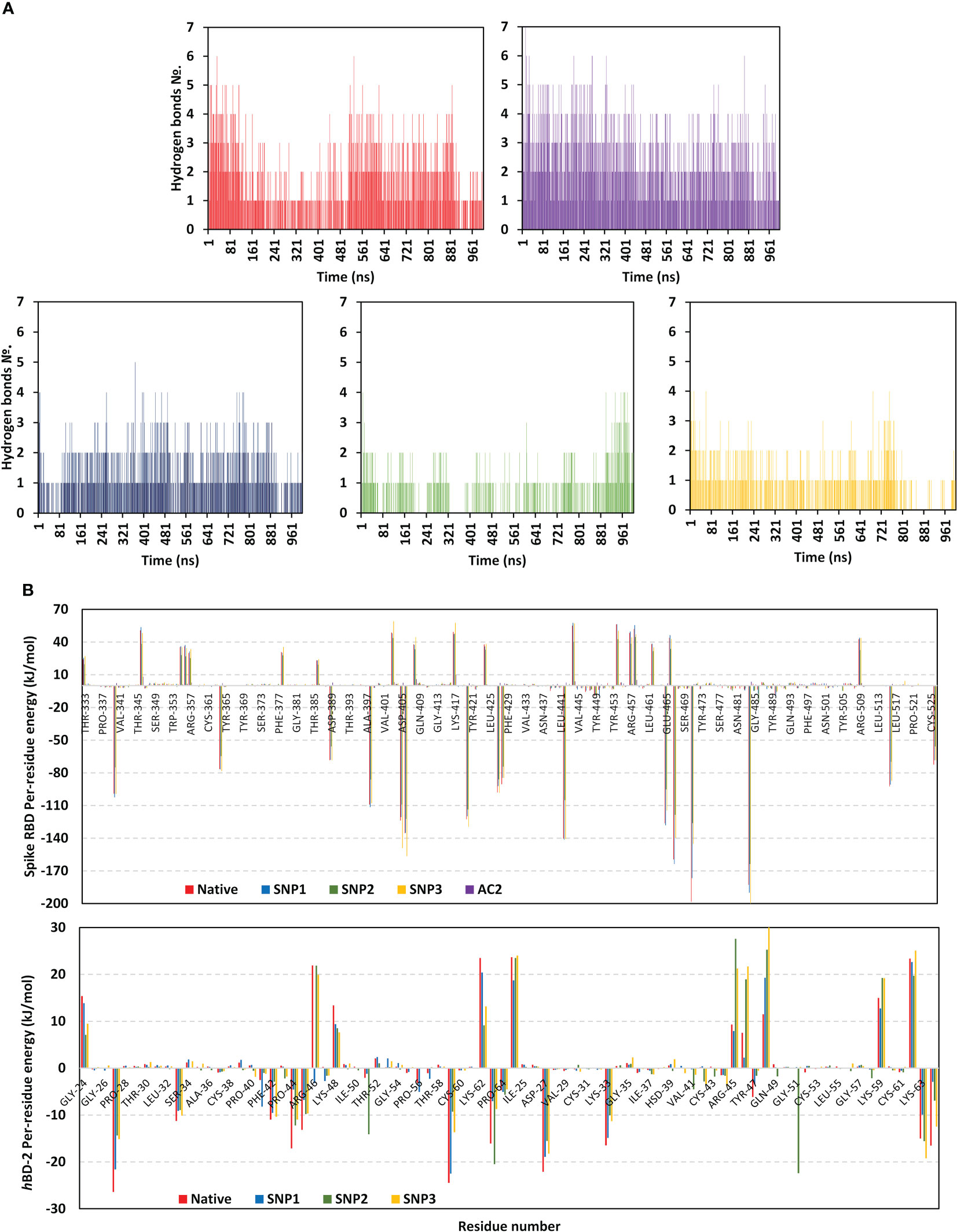
Figure 8 Binding interaction analysis for the simulated spike RBD/ligand complexes across the molecular dynamics simulations. (A) Number of furnished hydrogen bonds by the simulated complexes of native hBD-2, hACE2, SNP1, SNP2, and SNP3 ligands (upper left, upper right, bottom left-to-right panels, respectively), as a function of the simulated times (ns). (B) Per-residue MM-PBSA free binding energy contributions for spike RBD and ligand proteins.
The average number of protein–protein intermolecular hydrogen bonds was higher at native hBD-2 (1.52 ± 1.22) in relation to its mutant variants (SNP1; 1.18 ± 0.93, SNP2; 0.62 ± 0.82, SNP3; 0.70 ± 0.82), but the earlier was only second to the hACE2-associated complex (2.19 ± 1.29). Within the native hBD-2:RBD complex, high hydrogen bond occupancies were assigned for Lys63-Side : Glu484-Side (38%), Arg45-Side : Glu484-Side (34%), and Arg46-Side : Glu484-Main (22%). For SNP1, hydrogen bond pairs such as Arg46-Side : Glu484-Main (18%), Arg45-Side : Glu484-Side (17%), Arg46-Main : Asn487-Main (13%), and Lys48-Main : Asn487-Side (20%) were of lower occupancies as compared to the native protein. For the less stable SNP mutant complexes, only Arg45-Side : Glu484-Side (11%) and Lys63-Side : Glu484-Side (31%) hydrogen bond pairs at SNP2 and SNP3 complexes, respectively, were assigned with occupancies just ≥10%.
Notably, the above-described native and SNP1 hydrogen bond pairs were of reduced persistency at SNP2 and SNP3 complexes; the thing that was proposed correlated to the dramatic conformational dynamic behaviors of these latter variants. Regarding the reference complex, preferentiality of hACE2 towards the spike Ala475–Thr478 and Asn501–Tyr505 residue ranges were further highlighted since several high-frequency hydrogen bond pairings were depicted across these regions and vicinal residues: Tyr505-Side : Glu37-Side (48%), Gly502-Main : Lys353-Main (60%), Ser19-Side : Ala475-Main (29%), Lys417-Side : Asp30-Side (20%), Tyr83-Side : Asn487-Side (35%), Tyr453-Side : His34-Side (19%), and Lys31-Side : Gln493-Side (13%).
Exploring the residue-wise free binding energy contribution for the simulated complex was highlighted in Figure 8B. Higher negative-value energy contribution was assigned for native hBD-2 over its mutant proteins. Preferentiality for hBD-2 to bind to spike regional I and II residues was illustrated since several constituting and vicinal residues depicted relevant negative-value energy contributions: Asp442 (−104.91 to −141.30 kJ/mol), Glu465 (−95.13 to −128.11 kJ/mol), Asp467 (−118.48 to −163.76 kJ/mol), Glu471 (−126.07 to −198.34 kJ/mol), and Glu484 (−163.67 to −200.00 kJ/mol). In contrast, residues of the spike’s anti-parcel β-sheets and midway loops (Tyr451–Lys458 and Pro491–Gly496) were assigned with positive repulsive energy contributions; Arg454 (42.61 to 56.26 kJ/mol), Arg457 (33.41 to 49.60 kJ/mol), and Lys458 (44.60 to 55.64 kJ/mol) highlighted their unfavored binding with hBD-2 ligands. Per-residue energy contribution at hBD-2 proteins was significant for Lys33 (−8.96 to −11.25 kJ/mol), Phe42 (−2.88 to −10.97 kJ/mol), Pro44 (−4.57 to −17.11 kJ/mol), Arg45 (−6.78 to −13.16 kJ/mol), Lys59 (−9.29 to −24.48 kJ/mol), and Lys63 (−7.05 to −16.08 kJ/mol).
Focusing on comparative mutant residues with their native form, replacing Pro44 at native hBD-2 with Leu44 in SNP1 lowered the residue-wise energy contribution from −17.11 to −4.57 kJ/mol as well as the vicinal residue Arg45 from −13.16 to −6.78 kJ/mol. Regarding SNP2, replacing native hydrophobic Gly51 with polar Asp51 increased energy contribution (−2.03 to −14.10 kJ/mol), contributing to the dominant electrostatic potential of the ligand binding. Nevertheless, Asp28 possesses increased residue-wise size that might have contributed to steric clashes with spike residue for unfavored binding and SNP2’s depicted conformational shift. Finally, C53G SNP3 has associated more positive energy contribution in regard to its native form (1.47 versus 0.19 kJ/mol, respectively). All the above electrostatic preferentiality and comparative hydrogen bond occupancies were consistent with the obtained contact distance occupancy analysis as well as the preliminary docking results where polar residue pairs were much assigned to the native hBD-2 complex.
The analysis of gene–gene interactions
The analysis of the gene–gene interaction of the DEFB4A gene was performed using the GeneMania tool, leading to the identification of the 20 genes with the closest connection to the DEFB4A gene (Figure S3). Among these genes, the defensin beta 103A gene (DEFB103A) occupied the first rank. After that, the C–C motif chemokine receptor 6 gene (CCR6) occupied the second rank.
Discussion
In addition to the well-known role of hBD-2 and LL-37 in combatting different invading microbes and viruses (68, 69), recent studies have found an important role for hBD-2 and LL-37 in defending the human body against COVID-19 infection (70). Biophysical experiments and biochemical studies performed by Zhang and colleagues confirmed the ability of hBD-2 to bind the RBD of SARS-CoV-2 and inhibit the binding of this RBD to hACE2 (5). These results confirmed the in silico findings regarding the ability of hBD-2 to bind to the RBD of SARS-CoV-2 (5). Similar results were found with LL-37 and confirmed the high affinity of its binding to the RBD of this serious virus (9, 10). The damaging missense SNPs could have serious effects on protein structure and function (71), raising questions about the fate of this preventive role against COVID infection in these cases. Therefore, we aimed to find the damaging missense SNPs for hBD-2 and LL-37 and study the impact of these damaging mutations on the susceptibility to this serious disease.
Beginning from 897 SNPs in the DEFB4A gene, 24 missense SNPs were found in the sequence corresponding to hBD-2. These mutations were applied to analysis with six bioinformatics tools with various approaches and algorithms to ensure the robustness of the analysis. As a result, three SNPs were predicted to be disease-causing and deleterious. On the other side, beginning with 831 SNPs in the CAMP gene, 27 missense SNPs were found in the sequence corresponding to LL-37. However, by applying the same six bioinformatics tools, none of these SNP was found to be deleterious by at least five tools. Consequently, none of the LL-37 SNPs was selected for further analysis and only the damaging mutations with the DEFB4A gene were selected for further investigation.
Due to the importance of protein stability for maintaining the functions and the structure of the protein (72), the consequences of the selected mutations on the stability of hBD-2 were analyzed by I-mutant 2 and MUpro tools. All three mutations were predicted to decrease the stability of the protein by both tools. Moreover, the functional analysis of the protein was performed to determine its important domains depending on the InterPro tool. The analysis revealed the presence of a domain called (Beta/alpha-defensin, C-terminal domain), on which all the three mutations were located. After that, the phylogenetic conservation was analyzed using the ConSurf server showing that G51D and C53G SNPs were positioned on highly conserved residues while P44L SNP was positioned on variable residue. As the functionally and structurally important amino acids usually display high phylogenetic conservancy (38), the occurrence of SNPs in these conserved residues is expected to have an impact on the structure or function of the protein. In addition, using PSIPRED to analyze the secondary structure revealed the presence of changes in the secondary structures of hBD-2 with G51D and C53G mutations. The importance of the secondary structure of a protein is manifested in its essential roles in the structure and the folding of the protein (73). The effects of the three SNPs on hBD-2 structure were analyzed by the HOPE server showing the damaging effects of the three mutations on hBD-2 structure, in particular with G51D and C53G SNPs.
Molecular docking-coupled dynamic simulations demonstrated significant binding for native hBD-2 being just lower than the reference hACE2/spike RBD complex. The simulated native hBD-2 ligand showed overlapped binding at the spike RBD interface surface being similar to that for the reference hACE2 complex. Binding to spike RBD is proposed to be residue-wise dependent since differential stability, fluctuation patterns, and binding energy contributions were assigned for each protein down to its amino acid level. Herein, we adopted the spike Ser436–Tyr508 external loop domain for hBD-2 ligand anchoring as being significant for hACE2 recognition and binding (5, 44, 55, 56). Reported studies showed preferential recognition of the spike RBD towards the N-terminal subdomain-1 of hACE2 through predominant polar interactions (concentrated hydrogen bonding and salt bridges) between several hydrophilic residues along distinctive annealing regions (sites I and II) (74). Wang et al. showed that a hydrophobic stacking patch coexists for Tyr489 and Phe486 of RBD against Phe28, Leu79, Met82, and Tyr83 of hACE2, yet does not greatly contribute to virus-receptor engagement since a single L353A mutation was sufficient to abolish such interactions (56). Therefore, polar anchoring of ligands at RBD/hACE2 connective interface, with any of the interface polar residues, would probably impact both subdomain binding affinities or even alter the RBD conformation to be uncleavable via the host protease, TMPRSS2 (75, 76). Both suggested scenarios would halt the crucial stage of COVID-19 infection which is the virus–host membrane fusion and subsequent release of viral payload RNA into the host cytoplasm.
To our delight, both MM/GBSA and MM/PBSA binding energy calculations for respective docked and molecular dynamics complexes illustrated the predominance of electrostatic potentials and polar residue energy contributions for hBD-2 towards the spike RBD site. Greater negative values, suggesting stronger binding affinities, were consistent with the reported results of other research groups (5, 77). We decided to study the dimeric form of hBD-2, rather than their monomeric ones since these ligands exist at non-covalent hydrophobically bonded dimers within high concentrations (46, 78). Despite that, the reported hBD-2 dimerization could be quite modest and binding to spike RBD could stabilize the hBD-2 proteins at their oligomeric levels (5, 46). This was the case with the study by Zhang et al., where long ns all-atom simulation of dimeric hBD-2 showed higher free binding energies, greater hydrogen bond frequencies, and larger contact distances being maintained via both protomers as compared to the monomeric form (5).
Preferential binding of native hBD-2 over its SNP variants, being only second to the reference hACE2/spike RBD complex, was demonstrated through a multi-level stability analysis. The RMSD analysis was significant for showing limited conformational changes and superior relative stability of the native hBD-2 complex depicting steady tones across the simulation times. On general bases, RMSD trajectories provide accurate measurement regarding a molecular deviation from its reference structure at the beginning of the molecular dynamics simulations (79). High-protein RMSDs usually correlate to significant conformation alterations and instability, while for ligands, they confer compromised ligand-target affinity and ligand-pocket accommodation (80). Both Rgs and BSAs were translated well for the RMSD findings, since these parameters depicted inherited stability, compactness, and larger contact distances for the native hBD-2 complex in relation to its SNP proteins. Generally, lower Rg values with limited fluctuations suggested optimum structural compactness in terms of favored inter- or intra-molecular interactions (81). In contrast, larger BSA across the simulation timeline is correlated with a bigger protein–protein contact interface denoted as the buried surface area between both molecules (82).
It is worth noting that the here-simulated hACE2 and hBD-2 complexes were in dynamic motion at the spike RBD interface, which was consistent with the reported thermodynamic behavior of various protein–protein complexes (83–85). However, hBD-2 was more dynamic than hACE2, which was suggested to furnish less unfavored entropy on binding than those obtained with complexes where both or either one partner is significantly rigid (5, 86). This was consistent with our MM/PBSA energy term contribution findings where hBD-2 complexes were of lower polar solvation entropy, which would favor ligand binding since the latter is considered a solvent-displacement process (87). Moreover, flexible peptide-based blocking strategies were suggested as beneficial to circumvent mutations that could compromise hBD-2 blocking affinities (88). Nevertheless, thermodynamic flexibility could be double-bladed since higher conformational changes seen with SNP proteins compared to the native form were suggested with ligand drifting. Additionally, depicted SNP dynamic behaviors were likely accompanied by indirect hydrogen bonding with water molecules at or even near the interface bridging such polar interactions, which would underestimate the electrostatic binding potential and further increase polar solvation entropic penalties resulting from displacing highly ordered water molecules at interacting protein surfaces. The latter was seen with several protein–protein complexes where one partner had more solvent exposure (22, 24, 89).
Notably, SNP mutant residues at hBD-2 were proposed to influence ligand binding and conformation, which was confirmed here through lower per-residue free binding energy contributions, reduced contact distance occupancies, and/or significant steric clashes with congruent protein interface being in concordance with current literature data (90–92). Computational analysis through molecular docking and dynamics studies revealed the detrimental impact of SNP at hBD-1 gene on the stability and protein function to bind with the bacterial phosphatidylinositol-4,5-bisphosphate (PIP-2) protein target. Some of the depicted SNPs were associated with a PIP-2 binding interface and compromised binding energies (93). Another study by Teng et al. evaluated the binding free energies of more than 260 protein–protein complexes with known SNPs via CHARMM forcefield/continuum electrostatic calculations where they revealed that these SNPs tend to destabilize the binding energy electrostatic components (94). Additionally, a study by Ranjith-Kumar et al. investigating the impact of SNP on Toll-like receptor-3 structure, expression, and function showed through molecular modeling that two relevant SNPs would alter the receptor conformation, particularly its leucine-rich repeated motifs, which was also correlated with defective receptor activation activity (95).
The engagement with gene–gene interactions became very important when investigating the disease–gene relationship as it was confirmed that various genetic loci show interactions between them (96). Using GeneMANIA showed that the DEFB103A gene and CCR6 gene showed the closest connection with the DEFB4A gene. These genes with a close connection to DEFB4A may be affected by DEFB4A damaging SNPs as well.
Overall, our analysis showed the existence of three damaging missense mutations in hBD-2. These SNPs were predicted to affect the stability and the structure of hBD-2. Moreover, G51D and C53G mutations were located in highly conserved positions and had effects on the secondary structures of hBD-2. Furthermore, all-atom molecular dynamics simulations and free binding energy calculations assured that the native form has a preferential hBD-2 binding with the SARS-CoV-2 spike interface over the SNP mutant forms. Our results could increase our understanding of the genetic factors associated with COVID-19, which could improve the prevention as well as the management guidelines of such a serious disease (97, 98).
Conclusion
The revealed role of hBD-2 in fighting against SARS-CoV-2 raised questions about the effects of the damaging missense mutations on this protective role against COVID-19. Our comprehensive investigation showed that three mutations in hBD-2 have the most damaging impact: P44L, G51D, and C53G SNPs. These SNPs showed decreasing effects on the stability of hBD-2 and damaging effects on the structure of hBD-2. G51D and C53G SNPs also had high conserved positions and showed alterations in hBD-2 secondary structures. Furthermore, our computational model showed preferential hBD-2 binding, for the native over the SNPs mutant forms, at site I and site II of the SARS-CoV-2 spike interface guided by predominant electrostatic binding potentials. This was confirmed by all-atom molecular dynamics simulations and free binding energy calculations. The further implementation of experimental procedures could pave the way for the identification of patients prone to COVID-19 and the development of new diagnostics and procedures for the management of this serious infectious disease.
Data availability statement
The original contributions presented in the study are included in the article/Supplementary Material. Further inquiries can be directed to the corresponding authors.
Author contributions
MYB, MAS, MAE, and KMD: conceptualization, methodology, and original draft preparation. HO, MSAZ, SA, GA, IJ, EF, MaA and SA: writing—review and editing. SSE, MA-D, JA and RAE: supervision and project administration. All authors contributed to the article and approved the submitted version.
Funding
The Deanship of Scientific Research of King Khalid University in Abha, Saudi Arabia, entirely supported this research (Grant No. G.R.P.1-27-43).
Acknowledgments
This work is dedicated to the memory of Dr. Yehya Behairy, the consultant of internal medicine at the Ismailia General Hospital, whose fruitful advice and consultations helped a lot in the conceptualization of this research and who was always doing all he could to help whoever seeks help. The authors extend their gratitude to the King Khalid University Deanship of Scientific Research for funding this project through the General Research Project under the grant number (G.R.P.1-27-43). In addition, the simulations in this work were performed at King Abdulaziz University’s High-Performance Computing Center (Aziz Supercomputer) (https://hpc.kau.edu.sa). The authors, therefore, acknowledge with thanks the center for technical support.
Conflict of interest
The authors declare that the research was conducted in the absence of any commercial or financial relationships that could be construed as a potential conflict of interest.
Publisher’s note
All claims expressed in this article are solely those of the authors and do not necessarily represent those of their affiliated organizations, or those of the publisher, the editors and the reviewers. Any product that may be evaluated in this article, or claim that may be made by its manufacturer, is not guaranteed or endorsed by the publisher.
Supplementary material
The Supplementary Material for this article can be found online at: https://www.frontiersin.org/articles/10.3389/fimmu.2022.1008463/full#supplementary-material
References
1. Shereen MA, Khan S, Kazmi A, Bashir N, Siddique R. COVID-19 infection: Origin, transmission, and characteristics of human coronaviruses. J Adv Res (2020) 24:91–8. doi: 10.1016/j.jare.2020.03.005
2. Krausgruber T, Fortelny N, Fife-Gernedl V, Senekowitsch M, Schuster LC, Lercher A, et al. Structural cells are key regulators of organ-specific immune responses. Nature (2020) 583:296–302. doi: 10.1038/s41586-020-2424-4
3. Mulder KCL, Lima LA, Miranda VJ, Dias SC, Franco OL. Current scenario of peptide-based drugs: The key roles of cationic antitumor and antiviral peptides. Front Microbiol (2013) 4. doi: 10.3389/fmicb.2013.00321
4. Laneri S, Brancaccio M, Mennitti C, De Biasi MG, Pero ME, Pisanelli G, et al. Antimicrobial peptides and physical activity: A great hope against covid 19. Microorganisms (2021) 9:1415. doi: 10.3390/microorganisms9071415
5. Zhang L, Ghosh SK, Basavarajappa SC, Chen Y, Shrestha P, Penfield J, et al. HBD-2 binds SARS-CoV-2 RBD and blocks viral entry: Strategy to combat COVID-19. iScience (2022) 25:103856. doi: 10.1016/j.isci.2022.103856
6. Al-Bayatee NT, Ad’hiah AH. Human beta-defensins 2 and 4 are dysregulated in patients with coronavirus disease 19. Microb Pathog (2021) 160:105205. doi: 10.1016/j.micpath.2021.105205
7. Sigurdardottir T, Andersson P, Davoudi M, Malmsten M, Schmidtchen A, Bodelsson M. In silico identification and biological evaluation of antimicrobial peptides based on human cathelicidin LL-37. Antimicrob Agents Chemother (2006) 50:2983–9. doi: 10.1128/AAC.01583-05
8. Crane-Godreau MA, Clem KJ, Payne P, Fiering S. Vitamin d deficiency and air pollution exacerbate COVID-19 through suppression of antiviral peptide LL37. Front Public Heal (2020) 8. doi: 10.3389/fpubh.2020.00232
9. Wang C, Wang S, Li D, Chen P, Han S, Zhao G, et al. Human cathelicidin inhibits SARS-CoV-2 infection: Killing two birds with one stone. ACS Infect Dis (2021) 7:1545–54. doi: 10.1021/acsinfecdis.1c00096
10. Lokhande KB, Banerjee T, Swamy KV, Ghosh P, Deshpande M. An in silico scientific basis for LL-37 as a therapeutic for covid-19. Proteins (2022) 90:1029–43. doi: 10.1002/prot.26198
11. Liao PY, Lee KH. From SNPs to functional polymorphism: The insight into biotechnology applications. Biochem Eng J (2010) 49:149–58. doi: 10.1016/j.bej.2009.12.021
12. Emadi E, Akhoundi F, Kalantar SM, Emadi-Baygi M. Predicting the most deleterious missense nsSNPs of the protein isoforms of the human HLA-G gene and in silico evaluation of their structural and functional consequences. BMC Genet (2020) 17:1–13. doi: 10.1186/s12863-020-00890-y
13. Behairy MY, Abdelrahman AA, Abdallah HY, Ibrahim EE-DA, Say ed AA, Azab MM. In silico analysis of missense variants of the C1qA gene related to infection and autoimmune diseases. J Taibah Univ Med Sci (2022) 17:1074–82. doi: 10.1016/j.jtumed.2022.04.014
14. Chandramohan V, Nagaraju N, Rathod S, Kaphle A, Muddapur U. Identification of deleterious SNPs and their effects on structural level in CHRNA3 gene. Biochem Genet (2015) 53:159–68. doi: 10.1007/s10528-015-9676-y
15. Robert F, Pelletier J. Exploring the impact of single-nucleotide polymorphisms on translation. Front Genet (2018) 9:507. doi: 10.3389/fgene.2018.00507
16. Chasman D, Adams RM. Predicting the functional consequences of non-synonymous single nucleotide polymorphisms: Structure-based assessment of amino acid variation. J Mol Biol (2001) 307:683–706. doi: 10.1006/jmbi.2001.4510
17. Mehlotra RK, Zimmerman PA, Weinberg A. Defensin gene variation and HIV/AIDS: A comprehensive perspective needed. J Leukoc Biol (2016) 99:687–92. doi: 10.1189/jlb.6RU1215-560R
18. Chin LJ, Ratner E, Leng S, Zhai R, Nallur S, Babar I, et al. A SNP in a let-7 microRNA complementary site in the KRAS 3′ untranslated region increases non-small cell lung cancer risk. Cancer Res (2008) 68:8535–40. doi: 10.1158/0008-5472.CAN-08-2129
19. Kim YC, Jeong BH. Strong association of the Rs4986790 single nucleotide polymorphism (SNP) of the toll-like receptor 4 (TLR4) gene with human immunodeficiency virus (HIV) infection: A meta-analysis. Genes (Basel) (2021) 12:36. doi: 10.3390/genes12010036
20. Serveaux-Dancer M, Jabaudon M, Creveaux I, Belville C, Blondonnet R, Gross C, et al. Pathological implications of receptor for advanced glycation end-product (AGER) gene polymorphism. Dis Markers (2019) 2019:17. doi: 10.1155/2019/2067353
21. Zhang J, Yang J, Zhang L, Luo J, Zhao H, Zhang J, et al. A new SNP genotyping technology target SNP-seq and its application in genetic analysis of cucumber varieties. Sci Rep (2020) 10:1–11. doi: 10.1038/s41598-020-62518-6
22. Soltan MA, Behairy MY, Abdelkader MS, Albogami S, Fayad E, Eid RA, et al. In silico designing of an epitope-based vaccine against common e. coli pathotypes. Front Med (2022) 9:829467. doi: 10.3389/fmed.2022.829467
23. Jia M, Yang B, Li Z, Shen H, Song X, Gu W. Computational analysis of functional single nucleotide polymorphisms associated with the CYP11B2 gene. PloS One (2014) 9:e104311. doi: 10.1371/journal.pone.0104311
24. Soltan MA, Eldeen MA, Elbassiouny N, Kamel HL, Abdelraheem KM, El-Gayyed HA, et al. In silico designing of a multitope vaccine against rhizopus microsporus with potential activity against other mucormycosis causing fungi. Cells (2021) 10:3014. doi: 10.3390/cells10113014
25. Behairy MY, Abdelrahman AA, Abdallah HY, Ibrahim EE-DA, Hashem HR, Sayed AA, et al. Role of MBL2 polymorphisms in sepsis and survival: A pilot study and in silico analysis. Diagnos (Basel Switzerland) (2022) 12:460. doi: 10.3390/diagnostics12020460
26. Hossain MS, Roy AS, Islam MS. In silico analysis predicting effects of deleterious SNPs of human RASSF5 gene on its structure and functions. Sci Rep (2020) 10:14542. doi: 10.1038/s41598-020-71457-1
27. Navapour L, Mogharrab N. In silico screening and analysis of nonsynonymous SNPs in human CYP1A2 to assess possible associations with pathogenicity and cancer susceptibility. Sci Rep (2021) 11:4977. doi: 10.1038/s41598-021-83696-x
28. Sim NL, Kumar P, Hu J, Henikoff S, Schneider G, Ng PC. SIFT web server: Predicting effects of amino acid substitutions on proteins. Nucleic Acids Res (2012) 40:W452–7. doi: 10.1093/nar/gks539
29. Adzhubei IA, Schmidt S, Peshkin L, Ramensky VE, Gerasimova A, Bork P, et al. A method and server for predicting damaging missense mutations. Nat Methods (2010) 7:248–9. doi: 10.1038/nmeth0410-248
30. Choi Y, Chan AP. PROVEAN web server: A tool to predict the functional effect of amino acid substitutions and indels. Bioinformatics (2015) 31:2745–7. doi: 10.1093/bioinformatics/btv195
31. Capriotti E, Calabrese R, Fariselli P, Martelli PL, Altman RB, Casadio R. WS-SNPs&GO: a web server for predicting the deleterious effect of human protein variants using functional annotation. BMC Genomics (2013) 14(Suppl 3):S6. doi: 10.1186/1471-2164-14-s3-s6
32. Capriotti E, Calabrese R, Casadio R. Predicting the insurgence of human genetic diseases associated to single point protein mutations with support vector machines and evolutionary information. Bioinformatics (2006) 22:2729–34. doi: 10.1093/bioinformatics/btl423
33. Hecht M, Bromberg Y, Rost B. Better prediction of functional effects for sequence variants. BMC Genomics (2015) 16(Suppl 8):S1. doi: 10.1186/1471-2164-16-s8-s1
34. Capriotti E, Fariselli P, Casadio R. I-Mutant2.0: predicting stability changes upon mutation from the protein sequence or structure. Nucleic Acids Res (2005) 33:W306–10. doi: 10.1093/nar/gki375
35. Bava KA, Gromiha MM, Uedaira H, Kitajima K, Sarai A. ProTherm, version 4.0: thermodynamic database for proteins and mutants. Nucleic Acids Res (2004) 32:D120–1. doi: 10.1093/nar/gkh082
36. Cheng J, Randall A, Baldi P. Prediction of protein stability changes for single-site mutations using support vector machines. Proteins (2006) 62:1125–32. doi: 10.1002/prot.20810
37. Blum M, Chang HY, Chuguransky S, Grego T, Kandasaamy S, Mitchell A, et al. The InterPro protein families and domains database: 20 years on. Nucleic Acids Res (2021) 49:D344–d354. doi: 10.1093/nar/gkaa977
38. Berezin C, Glaser F, Rosenberg J, Paz I, Pupko T, Fariselli P, et al. ConSeq: The identification of functionally and structurally important residues in protein sequences. Bioinformatics (2004) 20:1322–4. doi: 10.1093/bioinformatics/bth070
39. Ashkenazy H, Abadi S, Martz E, Chay O, Mayrose I, Pupko T, et al. ConSurf 2016: An improved methodology to estimate and visualize evolutionary conservation in macromolecules. Nucleic Acids Res (2016) 44:W344–50. doi: 10.1093/nar/gkw408
40. Jones DT. Protein secondary structure prediction based on position-specific scoring matrices. J Mol Biol (1999) 292:195–202. doi: 10.1006/jmbi.1999.3091
41. Venselaar H, Beek TAT, Kuipers RKP, Hekkelman ML, Vriend G. Protein structure analysis of mutations causing inheritable diseases. an e-science approach with life scientist friendly interfaces. BMC Bioinf (2010) 11:548. doi: 10.1186/1471-2105-11-548
42. Elmaaty AA, Darwish KM, Chrouda A, Boseila AA, Tantawy MA, Elhady SS, et al. In silico and In vitro studies for benzimidazole anthelmintics repurposing as VEGFR-2 antagonists: Novel mebendazole-loaded mixed micelles with enhanced dissolution and anticancer activity. ACS omega (2022) 7:875–99. doi: 10.1021/acsomega.1c05519
43. Elhady SS, Abdelhameed RFA, Malatani RT, Alahdal AM, Bogari HA, Almalki AJ, et al. Molecular docking and dynamics simulation study of hyrtios erectus isolated scalarane sesterterpenes as potential SARS-CoV-2 dual target inhibitors. Biol (Basel) (2021) 10:389. doi: 10.3390/biology10050389
44. Helal MA, Shouman S, Abdelwaly A, Elmehrath AO, Essawy M, Sayed SM, et al. Molecular basis of the potential interaction of SARS-CoV-2 spike protein to CD147 in COVID-19 associated-lymphopenia. J Biomol Struct Dyn (2022) 40:1109–19. doi: 10.1080/07391102.2020.1822208
45. Almalki AJ, Ibrahim TS, Elhady SS, Hegazy WAH, Darwish KM. Computational and biological evaluation of β-adrenoreceptor blockers as promising bacterial anti-virulence agents. Pharm (Basel) (2022) 15:110. doi: 10.3390/ph15020110
46. Hoover DM, Rajashankar KR, Blumenthal R, Puri A, Oppenheim JJ, Chertov O, et al. The structure of human β-Defensin-2 shows evidence of higher order oligomerization. J Biol Chem (2000) 275:32911–8. doi: 10.1074/jbc.M006098200
47. Porter KA, Xia B, Beglov D, Bohnuud T, Alam N, Schueler-Furman O, et al. ClusPro PeptiDock: Efficient global docking of peptide recognition motifs using FFT. Bioinformatics (2017) 33:3299–301. doi: 10.1093/bioinformatics/btx216
48. Kozakov D, Hall DR, Xia B, Porter KA, Padhorny D, Yueh C, et al. The ClusPro web server for protein-protein docking. Nat Protoc (2017) 12:255–78. doi: 10.1038/nprot.2016.169
49. Kozakov D, Beglov D, Bohnuud T, Mottarella SE, Xia B, Hall DR, et al. How good is automated protein docking? Proteins (2013) 81:2159–66. doi: 10.1002/prot.24403
50. Desta IT, Porter KA, Xia B, Kozakov D, Vajda S. Performance and its limits in rigid body protein-protein docking. Structure (2020) 28:1071–1081.e3. doi: 10.1016/j.str.2020.06.006
51. Pierce BG, Wiehe K, Hwang H, Kim B-H, Vreven T, Weng Z. ZDOCK server: Interactive docking prediction of protein-protein complexes and symmetric multimers. Bioinformatics (2014) 30:1771–3. doi: 10.1093/bioinformatics/btu097
52. Chen R, Li L, Weng Z. ZDOCK: An initial-stage protein-docking algorithm. Proteins (2003) 52:80–7. doi: 10.1002/prot.10389
53. Kozakov D, Brenke R, Comeau SR, Vajda S. PIPER: An FFT-based protein docking program with pairwise potentials. Proteins (2006) 65:392–406. doi: 10.1002/prot.21117
54. Chuang G-Y, Kozakov D, Brenke R, Comeau SR, Vajda S. DARS (Decoys as the reference state) potentials for protein-protein docking. Biophys J (2008) 95:4217–27. doi: 10.1529/biophysj.108.135814
55. Lan J, Ge J, Yu J, Shan S, Zhou H, Fan S, et al. Structure of the SARS-CoV-2 spike receptor-binding domain bound to the ACE2 receptor. Nature (2020) 581:215–20. doi: 10.1038/s41586-020-2180-5
56. Wang Q, Zhang Y, Wu L, Niu S, Song C, Zhang Z, et al. Structural and functional basis of SARS-CoV-2 entry by using human ACE2. Cell (2020) 181:894–904.e9. doi: 10.1016/j.cell.2020.03.045
57. Schlee S, Straub K, Schwab T, Kinateder T, Merkl R, Sterner R. Prediction of quaternary structure by analysis of hot spot residues in protein-protein interfaces: The case of anthranilate phosphoribosyltransferases. Proteins (2019) 87:815–25. doi: 10.1002/prot.25744
58. Krissinel E. Crystal contacts as nature’s docking solutions. J Comput Chem (2010) 31:133–43. doi: 10.1002/jcc.21303
59. Krissinel E, Henrick K. Inference of macromolecular assemblies from crystalline state. J Mol Biol (2007) 372:774–97. doi: 10.1016/j.jmb.2007.05.022
60. Chakrabarty B, Parekh N. NAPS: Network analysis of protein structures. Nucleic Acids Res (2016) 44:W375–82. doi: 10.1093/nar/gkw383
61. Weng G, Wang E, Wang Z, Liu H, Zhu F, Li D, et al. HawkDock: A web server to predict and analyze the protein-protein complex based on computational docking and MM/GBSA. Nucleic Acids Res (2019) 47:W322–30. doi: 10.1093/nar/gkz397
62. Hou T, Wang J, Li Y, Wang W. Assessing the performance of the MM/PBSA and MM/GBSA methods. 1. the accuracy of binding free energy calculations based on molecular dynamics simulations. J Chem Inf Model (2011) 51:69–82. doi: 10.1021/ci100275a
63. Zhong S, Huang K, Luo S, Dong S, Duan L. Improving the performance of the MM/PBSA and MM/GBSA methods in recognizing the native structure of the bcl-2 family using the interaction entropy method. Phys Chem Chem Phys (2020) 22:4240–51. doi: 10.1039/c9cp06459a
64. Páll S, Abraham MJ, Kutzner C, Hess B, Lindahl E. “Tackling exascale software challenges in molecular dynamics simulations with GROMACS.,”. In: Solving software challenges for exascale. (Cham: Springer International Publishing) (2015). 3–27. doi: 10.1007/978-3-319-15976-8_1
65. Darden T, York D, Pedersen L. Particle mesh ewald: An n ·log( n ) method for ewald sums in large systems. J Chem Phys (1993) 98:10089–92. doi: 10.1063/1.464397
66. Warde-Farley D, Donaldson SL, Comes O, Zuberi K, Badrawi R, Chao P, et al. The GeneMANIA prediction server: Biological network integration for gene prioritization and predicting gene function. Nucleic Acids Res (2010) 38:W214–20. doi: 10.1093/nar/gkq537
67. Chaudhari AM, Singh I, Joshi M, Patel A, Joshi C. Defective ORF8 dimerization in SARS-CoV-2 delta variant leads to a better adaptive immune response due to abrogation of ORF8-MHC1 interaction. Mol Divers (2022) 3:1–3. doi: 10.1007/s11030-022-10405-9
68. Brice DC, Diamond G. Antiviral activities of human host defense peptides. Curr Med Chem (2020) 27:1420–43. doi: 10.2174/0929867326666190805151654
69. Solanki SS, Singh P, Kashyap P, Sansi MS, Ali SA. Promising role of defensins peptides as therapeutics to combat against viral infection. Microb Pathog (2021) 155:104930. doi: 10.1016/j.micpath.2021.104930
70. Ghosh SK, Weinberg A. Ramping up antimicrobial peptides against severe acute respiratory syndrome coronavirus-2. Front Mol Biosci (2021) 8:620806. doi: 10.3389/fmolb.2021.620806
71. Alshatwi AA, Hasan TN, Syed NA, Shafi G, Grace BL. Identification of functional SNPs in BARD1 gene and in silico analysis of damaging SNPs: Based on data procured from dbSNP database. PloS One (2012) 7:e43939. doi: 10.1371/journal.pone.0043939
72. Deller MC, Kong L, Rupp B. Protein stability: A crystallographer’s perspective. Acta Crystallogr Sect F Struct Biol Commun (2016) 72:72–95. doi: 10.1107/S2053230X15024619
73. Ji Y-Y, Li Y-Q. The role of secondary structure in protein structure selection. Eur Phys J E Soft Matter (2010) 32:103–7. doi: 10.1140/epje/i2010-10591-5
74. Shang J, Ye G, Shi K, Wan Y, Luo C, Aihara H, et al. Structural basis of receptor recognition by SARS-CoV-2. Nature (2020) 581:221–4. doi: 10.1038/s41586-020-2179-y
75. Hoffmann M, Kleine-Weber H, Schroeder S, Krüger N, Herrler T, Erichsen S, et al. SARS-CoV-2 cell entry depends on ACE2 and TMPRSS2 and is blocked by a clinically proven protease inhibitor. Cell (2020) 181:271–280.e8. doi: 10.1016/j.cell.2020.02.052
76. Gkogkou E, Barnasas G, Vougas K, Trougakos IP. Expression profiling meta-analysis of ACE2 and TMPRSS2, the putative anti-inflammatory receptor and priming protease of SARS-CoV-2 in human cells, and identification of putative modulators. Redox Biol (2020) 36:101615. doi: 10.1016/j.redox.2020.101615
77. He J, Tao H, Yan Y, Huang S-Y, Xiao Y. Molecular mechanism of evolution and human infection with SARS-CoV-2. Viruses (2020) 12:428. doi: 10.3390/v12040428
78. Seo ES, Blaum BS, Vargues T, De Cecco M, Deakin JA, Lyon M, et al. Interaction of human β-defensin 2 (HBD2) with glycosaminoglycans. Biochemistry (2010) 49:10486–95. doi: 10.1021/bi1011749
79. Arnittali M, Rissanou AN, Harmandaris V. Structure of biomolecules through molecular dynamics simulations. Proc Comput Sci (2019) 156:69–78. doi: 10.1016/j.procs.2019.08.181
80. Liu K, Watanabe E, Kokubo H. Exploring the stability of ligand binding modes to proteins by molecular dynamics simulations. J Comput Aided Mol Des (2017) 31:201–11. doi: 10.1007/s10822-016-0005-2
81. Likić VA, Gooley PR, Speed TP, Strehler EE. A statistical approach to the interpretation of molecular dynamics simulations of calmodulin equilibrium dynamics. Protein Sci (2005) 14:2955–63. doi: 10.1110/ps.051681605
82. Lee B, Richards FM. The interpretation of protein structures: Estimation of static accessibility. J Mol Biol (1971) 55:379–IN4. doi: 10.1016/0022-2836(71)90324-X
83. Zhang L, Borthakur S, Buck M. Dissociation of a dynamic protein complex studied by all-atom molecular simulations. Biophys J (2016) 110:877–86. doi: 10.1016/j.bpj.2015.12.036
84. Zhang L, Buck M. Molecular dynamics simulations reveal isoform specific contact dynamics between the plexin rho GTPase binding domain (RBD) and small rho GTPases Rac1 and Rnd1. J Phys Chem B (2017) 121:1485–98. doi: 10.1021/acs.jpcb.6b11022
85. Sami SA, Marma KKS, Mahmud S, Khan MAN, Albogami S, El-Shehawi AM, et al. Designing of a multi-epitope vaccine against the structural proteins of marburg virus exploiting the immunoinformatics approach. ACS Omega (2021) 6:32043–71. doi: 10.1021/acsomega.1c04817
86. Peccati F, Jiménez-Osés G. Enthalpy–entropy compensation in biomolecular recognition: A computational perspective. ACS Omega (2021) 6:11122–30. doi: 10.1021/acsomega.1c00485
87. Barillari C, Taylor J, Viner R, Essex JW. Classification of water molecules in protein binding sites. J Am Chem Soc (2007) 129:2577–87. doi: 10.1021/ja066980q
88. Agarwal G, Gabrani R. Antiviral peptides: Identification and validation. Int J Pept Res Ther (2021) 27:149–68. doi: 10.1007/s10989-020-10072-0
89. Soltan MA, Abdulsahib WK, Amer M, Refaat AM, Bagalagel AA, Diri RM, et al. Mining of marburg virus proteome for designing an epitope-based vaccine. Front Immunol (2022) 13:907481. doi: 10.3389/fimmu.2022.907481
90. Solem AC, Halvorsen M, Ramos SBV, Laederach A. The potential of the riboSNitch in personalized medicine. Wiley Interdiscip Rev RNA (2015) 6:517–32. doi: 10.1002/wrna.1291
91. Fragoza R, Das J, Wierbowski SD, Liang J, Tran TN, Liang S, et al. Extensive disruption of protein interactions by genetic variants across the allele frequency spectrum in human populations. Nat Commun (2019) 10:4141. doi: 10.1038/s41467-019-11959-3
92. Zhao N, Han JG, Shyu C-R, Korkin D. Determining effects of non-synonymous SNPs on protein-protein interactions using supervised and semi-supervised learning. PloS Comput Biol (2014) 10:e1003592. doi: 10.1371/journal.pcbi.1003592
93. Fareed MM, Ullah S, Aziz S, Johnsen TA, Shityakov S. In-silico analysis of non-synonymous single nucleotide polymorphisms in human β-defensin type 1 gene reveals their impact on protein-ligand binding sites. Comput Biol Chem (2022) 98:107669. doi: 10.1016/j.compbiolchem.2022.107669
94. Teng S, Madej T, Panchenko A, Alexov E. Modeling effects of human single nucleotide polymorphisms on protein-protein interactions. Biophys J (2009) 96:2178–88. doi: 10.1016/j.bpj.2008.12.3904
95. Ranjith-Kumar CT, Miller W, Sun J, Xiong J, Santos J, Yarbrough I, et al. Effects of single nucleotide polymorphisms on toll-like receptor 3 activity and expression in cultured cells. J Biol Chem (2007) 282:17696–705. doi: 10.1074/jbc.M700209200
96. Cordell HJ. Detecting gene-gene interactions that underlie human diseases. Nat Rev Genet (2009) 10:392–404. doi: 10.1038/nrg2579
97. Ramos-Lopez O, Daimiel L, Ramírez de Molina A, Martínez-Urbistondo D, Vargas JA, Martínez JA. Exploring host genetic polymorphisms involved in SARS-CoV infection outcomes: Implications for personalized medicine in COVID-19. Int J Genomics (2020) 2020:6901217. doi: 10.1155/2020/6901217
98. Ramos-Lopez O, Milagro FI, Allayee H, Chmurzynska A, Choi MS, Curi R, et al. Guide for current nutrigenetic, nutrigenomic, and nutriepigenetic approaches for precision nutrition involving the prevention and management of chronic diseases associated with obesity. J Nutrigenet Nutrigenomics (2017) 10:43–62. doi: 10.1159/000477729
Keywords: COVID-19, SNPs, molecular dynamics, antimicrobial peptides, hBD-2
Citation: Behairy MY, Soltan MA, Eldeen MA, Abdulhakim JA, Alnoman MM, Abdel-Daim MM, Otifi H, Al-Qahtani SM, Zaki MSA, Alsharif G, Albogami S, Jafri I, Fayad E, Darwish KM, Elhady SS and Eid RA (2022) HBD-2 variants and SARS-CoV-2: New insights into inter-individual susceptibility. Front. Immunol. 13:1008463. doi: 10.3389/fimmu.2022.1008463
Received: 31 July 2022; Accepted: 17 November 2022;
Published: 09 December 2022.
Edited by:
Lynn Morris, National Institute of Communicable Diseases (NICD), South AfricaReviewed by:
Caroline T Tiemessen, National Institute of Communicable Diseases (NICD), South AfricaSantosh K Ghosh, Case Western Reserve University, United States
Copyright © 2022 Behairy, Soltan, Eldeen, Abdulhakim, Alnoman, Abdel-Daim, Otifi, Al-Qahtani, Zaki, Alsharif, Albogami, Jafri, Fayad, Darwish, Elhady and Eid. This is an open-access article distributed under the terms of the Creative Commons Attribution License (CC BY). The use, distribution or reproduction in other forums is permitted, provided the original author(s) and the copyright owner(s) are credited and that the original publication in this journal is cited, in accordance with accepted academic practice. No use, distribution or reproduction is permitted which does not comply with these terms.
*Correspondence: Mohamed A Soltan, mohamed.mohamed@su.edu.eg; Mohammed Y. Behairy, mohamedyehya950@gmail.com
†These authors have contributed equally to this work