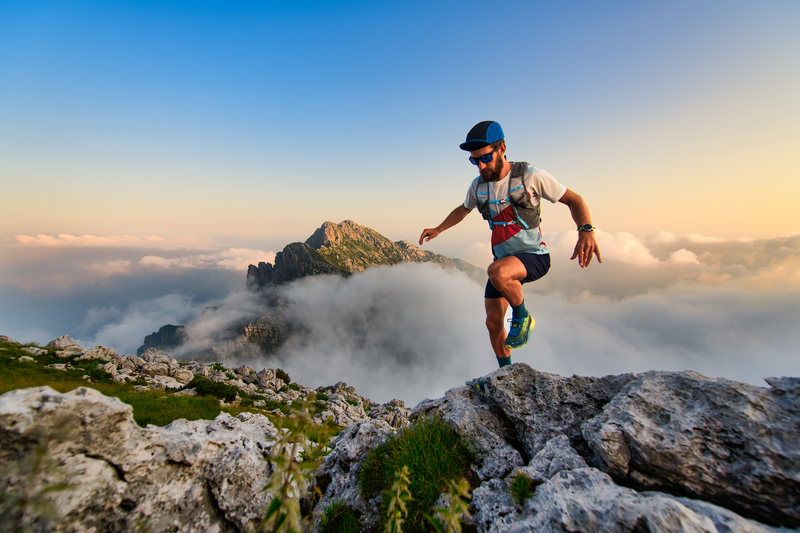
94% of researchers rate our articles as excellent or good
Learn more about the work of our research integrity team to safeguard the quality of each article we publish.
Find out more
ORIGINAL RESEARCH article
Front. Ecol. Evol. , 04 April 2022
Sec. Models in Ecology and Evolution
Volume 10 - 2022 | https://doi.org/10.3389/fevo.2022.841824
This article is part of the Research Topic Modeling the Link Between Microbial Ecology and Biogeochemical Process Dynamics View all 8 articles
The mechanisms underlying diversity-functioning relationships have been a consistent area of inquiry in biogeochemistry since the 1950s. Though these mechanisms remain unresolved in soil microbiomes, many approaches at varying scales have pointed to the same notion—composition matters. Confronting the methodological challenge arising from the complexity of microbiomes, this study used the model DEMENTpy, a trait-based modeling framework, to explore trait-based drivers of microbiome-dependent litter decomposition. We parameterized DEMENTpy for five sites along a climate gradient in Southern California, United States, and conducted reciprocal transplant simulations analogous to a prior empirical study. The simulations demonstrated climate-dependent legacy effects of microbial communities on plant litter decomposition across the gradient. This result is consistent with the previous empirical study across the same gradient. An analysis of community-level traits further suggests that a 3-way tradeoff among resource acquisition, stress tolerance, and yield strategies influences community assembly. Simulated litter decomposition was predictable with two community traits (indicative of two of the three strategies) plus local environment, regardless of the system state (transient vs. equilibrium). Although more empirical confirmation is still needed, community traits plus local environmental factors (e.g., environment and litter chemistry) may robustly predict litter decomposition across spatial-temporal scales. In conclusion, this study offers a potential trait-based explanation for climate-dependent community effects on litter decomposition with implications for improved understanding of whole-ecosystem functioning across scales.
Understanding how ecosystems function across spatial-temporal scales often requires knowledge of biotic community composition. This composition-functioning relationship has been a consistent theme since the 1950s (Harper, 1967). From terrestrial to aquatic to marine systems, species composition has been quantified and related to systems functioning (e.g., Loreau, 2000; Tilman et al., 2014). Given that microbiomes comprise tremendous diversity and complexity in the biosphere (e.g., Bardgett and van der Putten, 2014; Tedersoo et al., 2014; Thompson et al., 2017), understanding how microbiomes drive composition-functioning relationships can therefore inform how entire ecosystems function.
Many efforts have addressed composition-functioning relationships in microbiomes, but there are still unresolved mechanisms. For instance, functioning may saturate with increasing microbial diversity (e.g., CO2 production; Yu et al., 2019). Lab incubations of natural communities showed that composition matters for rates of plant litter decomposition (e.g., Strickland et al., 2009; Cleveland et al., 2014). Similarly, field sampling and subsequent lab incubations under the same conditions also revealed compositional effects (Rivett and Bell, 2018; Pascual-García and Bell, 2020).
In addition to varying community composition, there are studies that also manipulate local environment to study community-environment interactions. For instance, Allison et al. (2013) conducted a reciprocal transplant under varying drought and nitrogen deposition conditions in a grassland ecosystem and found that changes in microbial community composition can indirectly affect litter decomposition. In a gradient of lake sediments, Orland et al. (2019) showed that community structure and environment interacted to influence CO2 production. Notably, overcoming some limitations in these earlier studies, Glassman et al. (2018) conducted a large reciprocal transplant study across a climate gradient in Southern California, United States, and found climate-dependent compositional effects on litter decomposition. Still, even in that study, the mechanistic relationship between microbiome composition and functioning remained elusive.
The challenge of identifying underlying mechanisms may arise from interrelated conceptual and methodological issues in the fields of microbial ecology and biogeochemistry. First, many litter decomposition studies de-emphasize the role of microbial composition in controlling soil carbon dynamics (e.g., Beugnon et al., 2021). This approach reflects the influential conceptual framework of hierarchical control of litter decomposition (e.g., Lavelle et al., 1993; Aerts, 1997). That is, litter decomposition is argued to be hierarchically controlled by climate, substrate, and microorganisms, with microbial community composition occupying the least important position. More recently, this hierarchical theory has been challenged with the argument that decomposers control litter decomposition beyond the local scale and that a more explicit consideration of microbial communities is warranted (Bradford et al., 2017). Second, a high degree of functional redundancy in soil microbiomes introduces methodological issues (Finlay et al., 1997; Allison and Martiny, 2008). Communities with different taxonomic composition can be functionally very similar (Louca et al., 2016), making taxonomy-based approaches less relevant for predicting ecosystem processes. These issues are probably the major contributors to the current underappreciation of composition in modeling litter and soil organic matter decomposition (e.g., Adair et al., 2008; Bradford et al., 2017).
An alternative, emerging framework focuses on community-level functional traits that mediate the composition-functioning relationship in microbial systems under various disturbances. Trait-based investigations have been established in vegetation, showing clear advantages in revealing community composition-function relationships (e.g., McGill et al., 2006). For instance, recent studies demonstrated that traits can predict the long-term functional consequences of biodiversity change, together with data on interacting abiotic factors (e.g., van der Plas et al., 2020; Klimešová et al., 2021; Wolf et al., 2021). Trait-based quantification of microbial community composition, especially considering high functional redundancy (e.g., Allison and Martiny, 2008; Fetzer et al., 2015), holds promise for distinguishing functioning between communities. Malik et al. (2020a) proposed a trait-based Y-A-S framework, arguing microbial communities trade off among three primary strategies—Yield (Y), Acquisition (A), and Stress tolerance (S). Based on this Y-A-S theory, under drought pressure, microbiomes were revealed to trade off resource acquisition for stress tolerance (Wang and Allison, 2021). Therefore, we hypothesize that coordinated changes among traits representative of these three primary strategies may provide a unifying explanation for composition-function relationships under environmental change.
Trait-based modeling offers a flexible framework in which processes influencing microbiomes’ dynamics and functioning can be incorporated and easily manipulated. The modeling approach circumvents some logistic and technical challenges currently facing empirical studies. Following up on a previous reciprocal transplant experiment across a climate gradient in Southern California, United States that spanned nearly 2,000 m of elevation, 15°C in temperature, and multiple vegetation types (Glassman et al., 2018), we explored trait-based mechanisms with DEMENTpy, a trait-based microbial systems modeling framework (Allison, 2012; Wang and Allison, 2021). Here we expand on an earlier modeling study that focused on legacies of drought in a grassland litter microbiome (Wang and Allison, 2021). By simulating Glassman et al.’s (2018) broader reciprocal transplant design, we aimed to disentangle the influence of compositional legacy vs. climate change (temperature and precipitation) and their interactions on litter decomposition while identifying the roles of community traits in mediating microbial decomposition. Climate perturbation may affect microbiome functioning by altering community-level traits through selection on different community-level strategies (e.g., Watt, 1947; Wilson, 1997; Whitham et al., 2006). Guided by the overarching question of how microbial composition affects litter decomposition, this modeling study specifically addressed the following specific questions: (1) What are the relative contributions of microbiome composition (legacy effects) vs. local climate to litter decomposition? (2) Similarly, what are their relative contributions to the community-level traits of enzyme investment and drought tolerance? How are these traits coordinated? And (3) How do community traits relate to litter decomposition?
DEMENTpy (Decomposition Model of Enzymatic Traits in Python) is a spatially explicit, trait-based, microbial systems modeling framework built on top of an individual-based modeling scheme (Figure 1; GitHub Repository1 ; Wang and Allison, 2021). This model simulates microbial systems’ dynamics in composition (in terms of hypothetical taxa) and functioning (in terms of litter decomposition; Figure 1A). This model and its earlier versions (e.g., Allison, 2012; Allison and Goulden, 2017; Wang and Allison, 2019) have been successfully applied to addressing a series of issues in microbial ecology.
Figure 1. Schematic of the DEMENTpy model. DEMENTpy bridges across microbial traits, community-level processes, and system-level functions (A). Major traits include gene richness and production rate for transporters, enzymes, and osmolytes, as well as enzyme kinetic parameters (B). These traits dictate cellular level metabolic processes of constitutive and inducible production of enzymes and osmolytes (C).
Using a trait-based approach, DEMENTpy initiates a microbial community with many hypothetical taxa by randomly drawing values from distributions of physiological traits (Figure 1B) and assigning them to different taxa. These hypothetical taxa with differing combinations of trait values are randomly placed on a grid to form a spatially structured microbial community. Community dynamics are then simulated by explicitly modeling demographic processes of cell metabolism and growth, mortality, and reproduction for each taxon population at a daily time step driven by daily temperature and litter water potential. With explicit intra-cellular metabolism, microbial taxa secrete exoenzymes and produce osmolytes both constitutively and inducibly simultaneously without prescribing an order (Figure 1C). This non-hierarchical approach does not prescribe a cellular level tradeoff between enzyme and osmolyte production. Rather, tradeoffs emerge from community assembly. The exoenzymes degrade different organic compounds at rates that depend on temperature and moisture. The production of inducible osmolytes depends on water potential. The rate of inducible osmolyte production is then normalized to a value from 0 to 1, which is regarded as drought tolerance. This parameterization of drought tolerance is an update to the previous DEMENT version which instead directly introduced a drought tolerance parameter and imposed a penalty on carbon use efficiency (Allison and Goulden, 2017).
Five sites representing five ecosystems (Desert, Scrubland, Grassland, Pine-Oak, and Subalpine) were studied in Southern California, United States, forming a climate gradient spanning nearly 2,000 m of elevation and 15°C in temperature (Glassman et al., 2018). More detailed information about location, mean climate, and soil of these five sites can be found in Supplementary Figure 1 and the section on Gradient Information in Supplementary Appendix. A reciprocal transplant simulation like the field study by Glassman et al. (2018) was conducted with DEMENTpy across this gradient following a “transplant” protocol as follows.
Prior to transplant, we conducted a 3-year spin-up (Wang and Allison, 2021) to equilibrate microbiomes with site-specific litter and climate at each of the five sites. One year of climate data from 2011 (representative of normal meteorological conditions) was recycled three times at each site. At year four, simulated microbiomes (n = 20 per site) were designated as the starting communities for transplantation. These microbiomes were initiated (“inoculated”) on the same amount of grassland litter and reciprocally “transplanted” to the five sites where they were exposed to site-specific climate forcing. Our simulations use only grassland litter to mimic the empirical study, which for tractability reasons focused on a single litter type (grass) that occurred across the gradient (Glassman et al., 2018). These “transplant” simulations lasted 4 years with each transplant corresponding to a spin-up (n = 100 per site, e.g., 5 communities 20 spin-ups). In each new year, a new cohort of grassland litter was initiated.
With this transplant simulation protocol, we ran two different forcing scenarios. One scenario recycled the 2011 climate forcing through 8 years of simulations (hereafter referred to as average forcing; Supplementary Figure 2). Another recycled the 2011 forcing for 3 years to reach equilibrium, then—following the field transplant timeline—used 2015 forcing before the transplant and 2016–2019 forcing after the transplant (hereafter referred to as actual forcing; Supplementary Figure 3). The average forcing scenario was intended to emphasize the effect of site-driven climate variation on microbial legacies and to speed up the system return to equilibrium, whereas the actual forcing overlays a greater range of natural climate variability that slows the system return to equilibrium following transplantation, as in the Glassman et al. (2018) experiment. Daily soil surface temperature (°C) for each site was derived from averaging field soil temperature measurements for the average forcing and approximated using the Daymet (version 4; Thornton et al., 2020) daily temperature product for actual forcing. Estimates of litter water potential (MPa) were derived from analysis of fuel moisture sensors at the grassland site, near Loma Ridge, California (Allison and Goulden, 2017) using two different scaling methods for the average and actual forcing, respectively. See section DEMENTpy forcing in Supplementary Appendix for details.
All transplant scenarios were initialized on a 100 by 100 spatial grid with a bacterial community of 100 hypothetical taxa using well-informed parameter values (Supplementary Table 1; Allison and Goulden, 2017; Wang and Allison, 2021). The pool of taxa was the same prior to spin-up at all five sites. Leaf litter concentrations of C-, N-, and P-containing substrates (mg cm–3) were estimated based on near-infrared spectroscopy measurements at each site along the climate gradient (Baker and Allison, 2017). Within each forcing scenario, we ran ensembles of 20 independent simulations (corresponding to 20 independent spin-ups) for each transplant combination (5 sites × 5 communities × 20 spin-ups = 500 simulations in total). Each of the 25 combinations used the same set of 20 seeds for random number generation.
We analyzed simulation outputs for litter mass loss (i.e., total substrate remaining) and community-level traits (enzyme investment and drought tolerance) as well as community-level allocation to enzymes, osmolytes, and yield. Enzyme investment (Ecom) and drought tolerance (Dcom) are biomass-weighted community mean trait values calculated as:
respectively, where Ei and Di refer to the ith taxon’s enzyme production rate and drought tolerance, respectively, and Mi is the relative biomass of the ith taxon in the community. Using site-specific temperature and water potential, a series of statistical analyses were performed on these data. Assumptions of normality and equality of variance were ensured to be met during the analysis.
To validate the model, the simulated percent litter mass loss in the 1st year was compared to empirical measurements at 6 and 12 months after transplant (Glassman et al., 2018). Next, variance partitioning of decomposition (i.e., total substrate remaining) among factors of community (i.e., origin) and site (i.e., local environment), as well as their interactions was conducted with two-way ANOVA. The same analysis was performed twice to further test whether system state (transient: the end of the 1st year; equilibrium: the end of the 4th year under the average forcing) was a factor contributing to the changes in decomposition. These variance partitioning results were compared to the empirical results (Glassman et al., 2018) to further test the performance of DEMENTpy. Similarly, to disentangle factors influencing community enzyme investment and drought tolerance (an advantage of this modeling study), variance partitioning of enzyme investment and drought tolerance with two-way ANOVA was performed. Again, the same analysis was performed at two different time points in different years. Furthermore, Pearson’s correlation was used to examine relationships between the two traits simulated by the model.
To test whether community traits can explain decomposition, a series of four multiple linear regressions of decomposition against covariates of local environment [temperature (temp) and water potential (psi)], enzyme investment (enz), and/or drought tolerance (drt) were performed with the least-squares approach: mode1 1: f(temp, psi), model 2: f(temp, psi, enz), model 3: f(temp, psi, drt), and model 4: f(temp, psi, enz, drt). These multiple linear regression models were fitted separately to the 1st year and the 4th year data (including annual litter decomposition and mean traits and temperature and water potential) pooled together from all five sites. Adjusted R2 and information criteria of AIC (Akaike Information Criterion) and more parsimonious BIC (Bayesian Information Criterion) were used to evaluate model performance.
After the spin-up and before transplant, different microbial communities were realized across the gradient as indicated by differences in community traits of enzyme investment and drought tolerance (Supplementary Figure 4). Overall, from desert to subalpine, the drought tolerance decreased while the enzyme investment increased under both average forcing and actual forcing. However, this overall pattern was less pronounced for enzyme investment than for drought tolerance, especially for enzyme investment under average forcing (Supplementary Figure 4B).
In the 1st year after transplantation, the five communities showed different litter-decomposing capabilities across the gradient (Figure 2). The model-data comparison showed that simulated rates of mass loss were more similar in magnitude to the empirical data at 12 months than at 6 months, although at 12 months the model still tended to overestimate mass loss (Supplementary Figure 5). Even with this temporal difference in model performance, the simulated mass loss patterns were mainly consistent between average forcing and actual forcing (Figure 2 and Supplementary Figure 5). Decomposition was strongly influenced by local environment, with a pronounced increase (i.e., less substrate remaining) from desert to subalpine sites (df = 4, P < 0.001). The microbial community significantly affected decomposition as well (df = 4, P < 0.001), with the desert community overall decomposing the least and the pine-oak and subalpine communities decomposing the most with average forcing. With actual forcing, the subalpine community consistently decomposed the most. However, this community effect varied with local conditions (i.e., significant community-site interaction; df = 16; P < 0.05). The pattern of community differences was comparable across the desert and scrubland sites, but distinct from the other three sites. Particularly for the average forcing, those other three sites differentiated the five communities in terms of substrate remaining more strongly than the desert and scrubland sites.
Figure 2. Decomposition across the gradient during the 1st year and 4th year after transplant under both average forcing (A–J) and actual forcing (K–T). The color band is 90% confidence interval (n = 20).
By the 4th year after transplant, decomposition in the average and actual forcing scenarios became much more similar across the five sites (Year 4 in Figure 2). Though community (df = 4, P < 0.001) and its interaction with local conditions (df = 16, P < 0.05) were still statistically significant, only the desert community at the pine-oak and subalpine sites remained significantly different from the other communities (and only the subalpine site under actual forcing). All the other communities, especially at the desert and scrubland sites, showed similar litter decomposition.
The change in decomposition over time from the 1st through the 4th year was reflected in the changing relative contribution of community, local environment, and their interactions to the variance of litter decomposition. Take the average forcing case for example (Figure 3). After the 1st year, community, local environment, and their interactions accounted for 17.6, 46.4, and 2.0% of the variance in decomposition. By contrast, by the end of the 4th year, the contribution from community sharply declined to only 3.8% (the interaction to 1.5%), and local environment increased to 68.6%.
Figure 3. Variance partitioning of decomposition (A), enzyme trait (B), and drought tolerance (C). For the enzyme trait (B) only community and site are significant in year 1, and only site is significant in year 4. These results are under the average forcing.
Meanwhile, the relative differences in traits between the communities changed from the 1st through the 4th year (Figures 3B,C). After the 1st year, 4.4% of the variance in the enzyme trait was attributed to community (df = 4; P < 0.001) and 3.0% was attributed to local environment (df = 4; P < 0.01). However, after the 4th year, the enzyme trait was only significantly influenced by local environment (df = 4; P < 0.001), which explained only 7.6% of the variance. By contrast, the drought trait was consistently and significantly affected by community (df = 4; P < 0.001), local environment (df = 4; P < 0.001), and their interaction (df = 4; P < 0.001), though the relative contribution from community and local environment decreased (43.6–18.5%) and increased (34.0–59.2%), respectively, from the 1st to the 4th year. The contribution from their interactions increased slightly (6.5–8.4%).
Enzyme investment and drought tolerance traits displayed varying correlations in different sites across the gradient (Figure 4 and Supplementary Figure 6). Overall, these two traits were negatively correlated among the five communities both across the gradient and over time. At both time points, the correlation strength displayed an overall descending pattern across the gradient from low to high elevation. In addition, although the strength by the end of the 4th year was overall lower than the 1st year across the sites, it is noteworthy that the desert site did not change (Supplementary Figure 6F), and that the subalpine site became uncorrelated (Supplementary Figure 6J). These changes in traits and their correlations dictated community-level resource allocation among enzymes, osmolytes, and yield (Supplementary Figure 7).
Figure 4. Enzyme investment vs. drought tolerance by the end of year 1 and year 4 after the transplant. (A,B) Are under the average forcing, while (C,D) are under the actual forcing. Data are pooled together across the gradient and color-coded by site.
Community traits were related to annual decomposition with four multiple linear regression models (Table 1). In the 1st year, a model with either enzyme investment (Model 2) or drought tolerance traits (Model 3) explained decomposition better than a model with only local temperature and moisture (Model 1). Although Model 2 with enzyme investment was better than Model 3 with drought tolerance, only Model 4 with both drought tolerance and enzyme investment outperformed all three other models (both the smallest AIC and BIC and the largest adjusted R2-values). The performance of Model 4 was the best as well in the 4th year (both the smallest AIC and BIC and the largest adjusted R2-values), though its margin over Model 2 with enzyme investment was relatively small. In combination, a model with both enzyme and drought tolerance traits had the strongest explanatory power.
Table 1. Regression models predicting annual litter decomposition as a linear function of temperature, water potential, enzyme investment trait, and/or drought tolerance trait.
Identifying the mechanisms underpinning microbiomes’ composition-functioning relationship is a research theme of fundamental importance but with methodological challenges. Our study approached this issue from a trait-based perspective using a theory-driven, trait-based microbiome model—DEMENTpy—complemented by statistical modeling analyses. Overall, our simulations of litter decomposition were consistent with a previous transplant experiment in that both studies found evidence for climate-dependent legacy effects of microbial community composition (Glassman et al., 2018). Our model analysis of community-level traits further suggests that a 3-way tradeoff may mediate these legacies and the effects of local climate on community composition.
This modeling study agrees with the general conclusion of the earlier field transplant experiment by Glassman et al. (2018) that microbial composition matters in climate-dependent litter decomposition, though there are mismatches between the data and model outputs. DEMENTpy captured the pattern of litter mass loss across the gradient better at an annual timescale than at the shorter 6-month timescale (Supplementary Figure 5). In both studies, transplanted communities reflected the legacy of environmental conditions in their ecosystems of origin (including temperature, precipitation, and litter chemistry). Broadly speaking, model-predicted compositional effects and interactions between the community and local environment (Figure 2) were consistent with the field experiment. Moreover, the relative contributions of community, local environment, and their interactions to the variance in decomposition were also similar in both studies. Within 6–18 months after the transplant, Glassman et al. (2018) reported ranges of these contributions of ∼6–10% for the community (vs. 17.6% for the simulations at 1 year after transplant), ∼30–65% (vs. 46.4%) for local environment, and no significance to a maximum of 19% (vs. 2.0%) for their interactions. In accordance with the empirical results that showed an increasing contribution from local environment over time, our model also predicted a decline in legacy effects of composition over time, albeit at a longer timescale of 4 years (Figure 3A).
Although Glassman et al. (2018) found evidence for community-driven difference in litter decomposition, they did not find significant support for Home Field Advantage (HFA; Gholz et al., 2000; Veen et al., 2018) within a measurement time frame of 6–18 months after the transplant. Similarly, in our modeling study, by the end of the 1st year, the average decomposition of the five communities was very close to each other in the desert site (under both the average forcing and actual forcing), and the subalpine community had the most decomposition under actual forcing (though not significantly different) and relatively high decomposition under average forcing (not significantly different as well; Figure 2). Moreover, whether the system reached equilibrium in the 4th year (under the average forcing) or not (under the actual forcing), all HFA disappeared (Figure 2). Therefore, our modeling results and the field investigation were comparable with respect to overall patterns, but the time scale was different.
Though differing from Glassman et al.’s (2018) transplant experiment in some details, our modeling work confirms the empirical result that litter decomposition depends on microbial community composition. This finding adds to an increasing body of evidence along similar lines (e.g., Strickland et al., 2009; Allison et al., 2013; Cleveland et al., 2014; Bradford et al., 2017; Zakem et al., 2021). Moreover, some legacy effects may persist and thus cause different functioning under the same conditions (Wang and Allison, 2021). Such persistence is widely observed across different natural systems (e.g., Herzschuh, 2020; Ortiz et al., 2020; Wilson et al., 2021). Although dispersal in soil microbiomes may counter persistence (Wang and Allison, 2021), these results underscore the non-negligible role of environmental history as a key factor in litter decomposition (Spencer, 2020).
The notion of multidimensional tradeoffs in the biosphere is increasingly being embraced as organisms evolve across organizational and spatial-temporal scales under physical, biological, and ecological constraints (e.g., Kempes et al., 2019). Trait-based quantification of communities provides an approach for identifying those tradeoffs. Most notably, rich data on plant traits have revealed multidimensional tradeoffs first in shoots (e.g., Díaz et al., 2016), then in roots (e.g., Weemstra et al., 2016), and more recently in whole plants (Weigelt et al., 2021). Using these traits, vascular plants can be classified into the CSR (Competitor, Stress-tolerator, Ruderal) strategy scheme (Pierce et al., 2017). The same approach applies to multi-tradeoffs in phytoplankton (Edwards et al., 2011) and animals (de Froment et al., 2014). Our modeling study suggests there are also multi-dimensional tradeoffs in soil microbial communities. These tradeoffs emerge from community assembly of individual taxa with different capabilities in resource acquisition and stress tolerance. In particular, tradeoffs among enzyme investment, drought tolerance, and yield determine the microbial response to climate change (Figure 4 and Supplementary Figure 7).
Dispersal is another key process influencing community assembly, which itself can be shaped by local conditions and stochasticity (e.g., order of taxa arrival; Fukami, 2015; Reijenga et al., 2021). Our earlier study revealed that rapid dispersal could counter legacy effects driven by changes in microbial composition (Wang and Allison, 2021). Potentially many other disturbances (e.g., fire and nitrogen deposition) can combine to shape microbial community strategies. To tease out how each of these factors and their interactions drive 3-way tradeoffs in community traits, additional efforts focused on specific factors of interest are needed (e.g., Coyte et al., 2021).
Our analysis suggests that litter decomposition rates can be predicted with at least two community traits plus local environmental conditions (Table 1). This analysis contrasts with the assumption that community composition makes a negligible contribution to litter decomposition (Lavelle et al., 1993; Aerts, 1997; Adair et al., 2008; Bradford et al., 2017). Moreover, our model provides a useful approach for predicting decomposition across spatial-temporal scales that can integrate effects of past disturbances. Such an approach is important as empirical studies of litter decomposition and soil carbon stocks move beyond snapshots of large-scale spatial data to include information on disturbance and recovery (Bradford et al., 2021). However, this approach points to a challenge of measuring and deriving traits empirically.
Our modeling framework is promising but could benefit from more empirical data to parameterize microbial traits and reduce uncertainties. Forcing uncertainty does not appear to be a major issue because of the similarity in simulations between the two forcing scenarios (Figure 2 and Supplementary Figure 5). However, uncertainty remains in the simulated community traits due to missing processes such as fungi-bacteria interactions (e.g., Wright and Vetsigian, 2016), realistic dispersal (e.g., Cunillera-Montcusí et al., 2021), and evolution. Notably, a recent study across the Glassman et al. (2018) climate gradient found fast bacterial evolution in addition to ecological adaptation (Chase et al., 2021). This fast evolution, plus ecological drift resulting from fluctuating population sizes due to chance events (e.g., Travisano et al., 1995), can cause differences in community trait composition. Representing these processes in DEMENTpy may help make the predicted shifts in trait-based strategies more accurate, thereby improving model predictions of process rates at appropriate time scales (Supplementary Figure 5).
Making these model improvements will require additional trait measurements. Our simulations assume that enzymes and osmolytes are the main metabolites driving Y-A-S tradeoffs. However, microbes have complex metabolic networks, suggesting that many more traits are involved in Y-A-S strategies (Malik et al., 2020a). These additional traits (e.g., Weisskopf et al., 2021) may still fit in the 3-way tradeoff framework, but new techniques are needed to translate trait measurements into the parameters used by DEMENTpy to predict litter decomposition. A promising, yet challenging, approach would be to apply machine learning techniques (e.g., Chen et al., 2021) to derive indices of stress tolerance and resource allocation from rich -omics data along environmental gradients (e.g., Malik et al., 2020b; Heinken et al., 2021; Monson et al., 2022).
Our findings are applicable to improved prediction of whole ecosystem functioning. For example, incorporating plant-microbiome interactions into such predictions has been challenging (e.g., Van Voris et al., 1980; Ramirez et al., 2019), but our modeling approach provides a starting point. Litter chemistry, which can be associated with above- and below-ground plant traits (Cornwell et al., 2008), clearly influences litter decomposition. Therefore, it could be fruitful to predict litter decomposition at large scales by linking plant and microbial traits. Such an approach could even allow simultaneous consideration of legacy effects on vegetation and microbial communities, as well as their interaction (e.g., Schmid et al., 2021).
More generally, our study, together with recent vegetation modeling (e.g., Wang et al., 2018; Rüger et al., 2020), suggests model predictions could be improved by considering the multi-dimensional nature of trait tradeoffs in microbiomes and the biosphere in general. Vegetation modeling is already moving in this direction (e.g., Kraft et al., 2015; Bruelheide et al., 2018; Weigelt et al., 2021), and microbiome studies are catching up (Westoby et al., 2021). Still, there remains the challenge of incorporating these multidimensional tradeoffs into ecosystem and Earth system models (e.g., Fiedler et al., 2021; Terrer et al., 2021) while avoiding the computational expense of simulating microbial and vegetation composition locally. Informing larger-scale models with outputs from trait-based community models, either through direct or offline coupling, may be a potential way forward.
Our theory-driven modeling study suggests that climate-dependent changes in litter decomposition depend on shifts in microbial functional strategies within a 3-way tradeoff space. These shifts integrate legacies of past disturbance as a key driver of decomposition. Emerging from these findings is a framework for predicting microbial litter decomposition as a function of at least two community-level traits interacting with local climate and litter substrate. This framework implies that a data-driven statistical model could predict litter decomposition and soil organic matter dynamics if high-quality empirical measurements of community traits are available at sufficient temporal resolution. Our work also suggests that trait-based modeling, together with progress made in trait-based vegetation studies, is an effective tool for exploring the mechanisms underlying ecosystem functioning in the context of disturbance. Although uncertainty remains in model performance, especially at a higher temporal resolution, these tools, together with more complementary trait measurements, should be applied to understand the roles of microbiomes in the functioning of the Earth system. Overall, our study sheds light on the mechanisms underpinning the diversity-functioning relationship in complex microbiomes.
The datasets presented in this study can be found in online repositories. The names of the repository/repositories and accession number(s) can be found below: https://github.com/bioatmosphere/microbiome-climate-gradient.
BW and SA conceived the design. BW performed the simulation and analysis and wrote the first draft. SA contributed largely to the analysis and editing, and secured the funding. Both authors contributed to the article and approved the submitted version.
This research used resources of the Compute and Data Environment for Science (CADES) at the Oak Ridge National Laboratory, which was supported by the Office of Science of the US Department of Energy (DOE) under Contract No. DE-AC05-00OR22725. Funding was provided by the US DOE, Office of Science, Biological and Environmental Research award DE-SC0020382, and the US National Science Foundation award DEB-1457160.
The authors declare that the research was conducted in the absence of any commercial or financial relationships that could be construed as a potential conflict of interest.
All claims expressed in this article are solely those of the authors and do not necessarily represent those of their affiliated organizations, or those of the publisher, the editors and the reviewers. Any product that may be evaluated in this article, or claim that may be made by its manufacturer, is not guaranteed or endorsed by the publisher.
The version of DEMENTpy used in this study is available at https://github.com/bioatmosphere/DEMENTpy. Data and code underlying the analyses of this manuscript are publicly available in this GitHub Repository: https://github.com/bioatmosphere/microbiome-climate-gradient.
The Supplementary Material for this article can be found online at: https://www.frontiersin.org/articles/10.3389/fevo.2022.841824/full#supplementary-material
Adair, E. C., Parton, W. J., Del Grosso, S. J., Silver, W. L., Harmon, M. E., Hall, S. A., et al. (2008). Simple three-pool model accurately describes patterns of long-term litter decomposition in diverse climates. Glob. Change Biol. 14, 2636–2660.
Aerts, R. (1997). Climate, leaf litter chemistry and leaf litter decomposition in terrestrial ecosystems: a triangular relationship. OIKOS 79, 439–449. doi: 10.2307/3546886
Allison, S. D. (2012). A trait-based approach for modelling microbial litter decomposition. Ecol. Lett. 15, 1058–1070. doi: 10.1111/j.1461-0248.2012.01807.x
Allison, S. D., and Goulden, M. L. (2017). Consequences of drought tolerance traits for microbial decomposition in the DEMENT model. Soil Biol. Biochem. 107, 104–113. doi: 10.1016/j.soilbio.2017.01.001
Allison, S. D., and Martiny, J. B. (2008). Resistance, resilience, and redundancy in microbial communities. Proc. Natl. Acad. Sci. U.S.A. 105, 11512–11519. doi: 10.1073/pnas.0801925105
Allison, S. D., Lu, Y., Weihe, C., Goulden, M. L., Martiny, A. C., Treseder, K. K., et al. (2013). Microbial abundance and composition influence litter decomposition response to environmental change. Ecology 94, 714–725. doi: 10.1890/12-1243.1
Baker, N. R., and Allison, S. D. (2017). Extracellular enzyme kinetics and thermodynamics along a climate gradient in southern California. Soil Biol. Biochem. 114, 82–92.
Bardgett, R., and van der Putten, W. (2014). Belowground biodiversity and ecosystem functioning. Nature 515, 505–511. doi: 10.1038/nature13855
Beugnon, R., Du, J., Cesarz, S., Jurburg, S. D., Pang, Z., Singavarapu, B., et al. (2021). Tree diversity and soil chemical properties drive the linkages between soil microbial community and ecosystem functioning. ISME Commun. 1, 1–11.
Bradford, M. A., Veen, G. F., Bonis, A., Bradford, E. M., Classen, A. T., Cornelissen, J. H. C., et al. (2017). A test of the hierarchical model of litter decomposition. Nat. Ecol. Evol. 1, 1836–1845. doi: 10.1038/s41559-017-0367-4
Bradford, M. A., Wood, S. A., Addicott, E. T., Fenichel, E. P., Fields, N., González-Rivero, J., et al. (2021). Quantifying microbial control of soil organic matter dynamics at macrosystem scales. Biogeochemistry 156, 19–40. doi: 10.1007/s10533-021-00789-5
Bruelheide, H., Dengler, J., Purschke, O., Lenoir, J., Jiménez-Alfaro, B., Hennekens, S. M., et al. (2018). Global trait–environment relationships of plant communities. Nat. Ecol. Evol. 2, 1906–1917. doi: 10.1038/s41559-018-0699-8
Chase, A. B., Weihe, C., and Martiny, J. B. (2021). Adaptive differentiation and rapid evolution of a soil bacterium along a climate gradient. Proc. Natl. Acad. Sci. U.S.A. 118, e2101254118. doi: 10.1073/pnas.2101254118
Chen, L., Lu, W., Wang, L., Xing, X., Chen, Z., Teng, X., et al. (2021). Metabolite discovery through global annotation of untargeted metabolomics data. Nat. Methods 18, 1377–1385. doi: 10.1038/s41592-021-01303-3
Cleveland, C. C., Reed, S. C., Keller, A. B., Nemergut, D. R., O’Neill, S. P., Ostertag, R., et al. (2014). Litter quality versus soil microbial community controls over decomposition: a quantitative analysis. Oecologia 174, 283–294. doi: 10.1007/s00442-013-2758-9
Cornwell, W. K., Cornelissen, J. H., Amatangelo, K., Dorrepaal, E., Eviner, V. T., Godoy, O., et al. (2008). Plant species traits are the predominant control on litter decomposition rates within biomes worldwide. Ecol. Lett. 11, 1065–1071. doi: 10.1111/j.1461-0248.2008.01219.x
Coyte, K. Z., Rao, C., Rakoff-Nahoum, S., and Foster, K. R. (2021). Ecological rules for the assembly of microbiome communities. PLoS Biol. 19:e3001116. doi: 10.1371/journal.pbio.3001116
Cunillera-Montcusí, D., Borthagaray, A. I, Boix, D., Gascón, S., Sala, J., Tornero, I., et al. (2021). Metacommunity resilience against simulated gradients of wildfire: disturbance intensity and species dispersal ability determine landscape recover capacity. Ecography 44, 1022–1034. doi: 10.1111/ecog.05347
de Froment, A. J., Rubenstein, D. I., and Levin, S. A. (2014). An extra dimension to decision-making in animals: the three-way trade-off between speed, effort per-unit-time and accuracy. PLoS Comput. Biol. 10:e1003937. doi: 10.1371/journal.pcbi.1003937
Díaz, S., Kattge, J., Cornelissen, J. H., Wright, I. J., Lavorel, S., Dray, S., et al. (2016). The global spectrum of plant form and function. Nature 529, 167–171.
Edwards, K. F., Klausmeier, C. A., and Litchman, E. (2011). Evidence for a three-way trade-off between nitrogen and phosphorus competitive abilities and cell size in phytoplankton. Ecology 92, 2085–2095. doi: 10.1890/11-0395.1
Fetzer, I., Johst, K., Schäwe, R., Banitz, T., Harms, H., and Chatzinotas, A. (2015). The extent of functional redundancy changes as species’ roles shift in different environments. Proc. Natl. Acad. Sci. U.S.A. 112, 14888–14893. doi: 10.1073/pnas.1505587112
Fiedler, S., Monteiro, J. A., Hulvey, K. B., Standish, R. J., Perring, M. P., and Tietjen, B. (2021). Global change shifts trade-offs among ecosystem functions in woodlands restored for multifunctionality. J. Appl. Ecol. 58, 1705–1717. doi: 10.1111/1365-2664.13900
Finlay, B. J., Maberly, S. C., and Cooper, J. I. (1997). Microbial diversity and ecosystem function. OIKOS 80, 209–213. doi: 10.2307/3546587
Fukami, T. (2015). Historical contingency in community assembly: integrating niches, species pools, and priority effects. Annu. Rev. Ecol. Evol. Syst. 46, 1–23. doi: 10.1108/JKM-05-2013-0195
Gholz, H. L., Wedin, D. A., Smitherman, S. M., Harmon, M. E., and Parton, W. J. (2000). Long-term dynamics of pine and hardwood litter in contrasting environments: toward a global model of decomposition. Glob. Change Biol. 6, 751–765. doi: 10.1046/j.1365-2486.2000.00349.x
Glassman, S. I., Weihe, C., Li, J., Albright, M. B., Looby, C. I., Martiny, A. C., et al. (2018). Decomposition responses to climate depend on microbial community composition. Proc. Natl. Acad. Sci. U.S.A. 115, 11994–11999. doi: 10.1073/pnas.1811269115
Harper, J. L. (1967). A Darwinian approach to plant ecology. J. Ecol. 55, 247–270. doi: 10.2307/2257876
Heinken, A., Basile, A., and Thiele, I. (2021). Advances in constraint-based modelling of microbial communities. Curr. Opin. Syst. Biol. 27:100346.
Herzschuh, U. (2020). Legacy of the Last Glacial on the present-day distribution of deciduous 521 versus evergreen boreal forests. Glob. Ecol. Biogeogr. 29, 198–206. doi: 10.1111/geb.13018
Kempes, C. P., Koehl, M. A. R., and West, G. B. (2019). The scales that limit: the physical boundaries of evolution. Front. Ecol. Evol. 7:242. doi: 10.3389/fevo.2019.00242
Klimešová, J., Mudrák, O., Martínková, J., Lisner, A., Lepš, J., Filartiga, A. L., et al. (2021). Are belowground clonal traits good predictors of ecosystem functioning in temperate grasslands? Funct. Ecol. 35, 787–795. doi: 10.1111/1365-2435.13755
Kraft, N. J., Godoy, O., and Levine, J. M. (2015). Plant functional traits and the multidimensional nature of species coexistence. Proc. Natl. Acad. Sci. U.S.A. 112, 797–802. doi: 10.1073/pnas.1413650112
Lavelle, P., Blanchart, E., Martin, A., Martin, S., and Spain, A. (1993). A hierarchical model for decomposition in terrestrial ecosystems: application to soils of the humid tropics. Biotropica 25, 130–150. doi: 10.2307/2389178
Loreau, M. (2000). Biodiversity and ecosystem functioning: recent theoretical advances. OIKOS 91, 3–17.
Louca, S., Parfrey, L. W., and Doebeli, M. (2016). Decoupling function and taxonomy in the global ocean microbiome. Science 353, 1272–1277. doi: 10.1126/science.aaf4507
Malik, A. A., Martiny, J. B., Brodie, E. L., Martiny, A. C., Treseder, K. K., and Allison, S. D. (2020a). Defining trait-based microbial strategies with consequences for soil carbon cycling under climate change. ISME J. 14, 1–9. doi: 10.1038/s41396-019-0510-0
Malik, A. A., Swenson, T., Weihe, C., Morrison, E. W., Martiny, J. B., Brodie, E. L., et al. (2020b). Drought and plant litter chemistry alter microbial gene expression and metabolite production. ISME J. 14, 2236–2247. doi: 10.1038/s41396-020-0683-6
McGill, B. J., Enquist, B. J., Weiher, E., and Westoby, M. (2006). Rebuilding community ecology from functional traits. Trends Ecol. Evol. 21, 178–185.
Monson, R. K., Trowbridge, A. M., Lindroth, R. L., and ad Lerdau, M. T. (2022). Coordinated resource allocation to plant growth-defense tradeoffs. New Phytologist. 233, 1051–1066. doi: 10.1111/nph.17773
Orland, C., Emilson, E. J. S., Basiliko, N., Mykytczuk, N. C., Gunn, J. M., and Tanentzap, A. J. (2019). Microbiome functioning depends on individual and interactive effects of the environment and community structure. ISME J. 13, 1–11. doi: 10.1038/s41396-018-0230-x
Ortiz, Y., Restrepo, C., Vilanova-Cuevas, B., Santiago-Valentin, E., Tringe, S. G., and Godoy-Vitorino, F. (2020). Geology and climate influence rhizobiome composition of the phenotypically diverse tropical tree Tabebuia heterophylla. PLoS One 15:e0231083. doi: 10.1371/journal.pone.0231083
Pascual-García, A., and Bell, T. (2020). Community-level signatures of ecological succession in natural bacterial communities. Nat. Commun. 11:2386. doi: 10.1038/s41467-020-16011-3
Pierce, S., Negreiros, D., Cerabolini, B. E., Kattge, J., Díaz, S., Kleyer, M., et al. (2017). A global method for calculating plant CSR ecological strategies applied across biomes world-wide. Funct. Ecol. 31, 444–457.
Ramirez, K. S., Snoek, L. B., Koorem, K., Geisen, S., Bloem, L., ten Hooven, F. C., et al. (2019). Range-expansion effects on the belowground plant microbiome. Nat. Ecol. Evol. 3, 604–611. doi: 10.1038/s41559-019-0828-z
Reijenga, B. R., Murrell, D. J., and Pigot, A. L. (2021). Priority effects and the macroevolutionary dynamics of biodiversity. Ecol. Lett. 24, 1455–1466. doi: 10.1111/ele.13766
Rivett, D. W., and Bell, T. (2018). Abundance determines the functional role of bacterial phylotypes in complex communities. Nat. Microbiol. 3, 767–772. doi: 10.1038/s41564-018-0180-0
Rüger, N., Condit, R., Dent, D. H., DeWalt, S. J., Hubbell, S. P., Lichstein, J. W., et al. (2020). Demographic trade-offs predict tropical forest dynamics. Science 368, 165–168. doi: 10.1126/science.aaz4797
Schmid, M. W., van Moorsel, S. J., Hahl, T., De Luca, E., Deyn, G. B., Wagg, C., et al. (2021). Effects of plant community history, soil legacy and plant diversity on soil microbial communities. J. Ecol. 109, 3007–3023.
Spencer, H. G. (2020). Beyond Equilibria: the neglected role of history in ecology and evolution. Q. Rev. Biol. 95, 311–321.
Strickland, M. S., Lauber, C., Fierer, N., and Bradford, M. A. (2009). Testing the functional significance of microbial community composition. Ecology 90, 441–451. doi: 10.1890/08-0296.1
Tedersoo, L., Bahram, M., Põlme, S., Kõljalg, U., Yorou, N. S., Wijesundera, R., et al. (2014). Global diversity and geography of soil fungi. Science 346:1256688. doi: 10.1126/science.1256688
Terrer, C., Phillips, R. P., Hungate, B. A., Rosende, J., Pett-Ridge, J., Craig, M. E., et al. (2021). A trade-off between plant and soil carbon storage under elevated CO2. Nature 591, 599–603. doi: 10.1038/s41586-021-03306-8
Thompson, L., Sanders, J., McDonald, D., Amir, A., Ladau, J., Locey, K. J., et al. (2017). A communal catalogue reveals Earth’s multiscale microbial diversity. Nature 551, 457–463. doi: 10.1038/nature24621
Thornton, M. M., Shrestha, R., Wei, Y., Thornton, P. E., Kao, S., and Wilson, B. E. (2020). Daymet: Daily Surface Weather Data on a 1-km Grid for North America, Version 4. Oak Ridge, TN: ORNL DAAC. doi: 10.3334/ORNLDAAC/1840
Tilman, D., Isbell, F., and Cowles, J. M. (2014). Biodiversity and ecosystem functioning. Annu. Rev. Ecol. Evol. Syst. 45, 471–493.
Travisano, M., Mongold, J. A., Bennett, A. F., and Lenski, R. E. (1995). Experimental tests of the roles of adaptation, chance, and history in evolution. Science 267, 87–90. doi: 10.1126/science.7809610
van der Plas, F., Schröder-Georgi, T., Weigelt, A., Barry, K., Meyer, S., Alzate, A., et al. (2020). Plant traits alone are poor predictors of ecosystem properties and long-term ecosystem functioning. Nat. Ecol. Evol. 4, 1602–1611. doi: 10.1038/s41559-020-01316-9
Van Voris, P., O’Neill, R. V., Emanuel, W. R., and Shugart, H. H. Jr. (1980). Functional complexity and ecosystem stability. Ecology 61, 1352–1360.
Veen, G. F., Keiser, A. D., van der Putten, W. H., and Wardle, D. A. (2018). Variation in home-field advantage and ability in leaf litter decomposition across successional gradients. Funct. Ecol. 32, 1563–1574. doi: 10.1111/1365-2435.13107
Wang, B., and Allison, S. D. (2019). Emergent properties of organic matter decomposition by soil enzymes. Soil Biol. Biochem. 136:107522. doi: 10.1016/j.envpol.2016.09.094
Wang, B., and Allison, S. D. (2021). Drought legacies mediated by trait trade-offs in soil microbiomes. Ecosphere 12:e03562.
Wang, B., Shuman, J., Shugart, H. H., and Lerdau, M. T. (2018). Biodiversity matters in feedbacks between climate change and air quality: a study using an individual-based model. Ecol. Appl. 28, 1223–1231. doi: 10.1002/eap.1721
Weemstra, M., Mommer, L., Visser, E. J., van Ruijven, J., Kuyper, T. W., Mohren, G. M., et al. (2016). Towards a multidimensional root trait framework: a tree root review. New Phytol. 211, 1159–1169. doi: 10.1111/nph.14003
Weigelt, A., Mommer, L., Andraczek, K., Iversen, C. M., Bergmann, J., Bruelheide, H., et al. (2021). An integrated framework of plant form and function: the belowground perspective. New Phytol. 232, 42–59. doi: 10.1111/nph.17590
Weisskopf, L., Schulz, S., and Garbeva, P. (2021). Microbial volatile organic compounds in intra-kingdom and inter-kingdom interactions. Nat. Rev. Microbiol. 19, 391–404. doi: 10.1038/s41579-020-00508-1
Westoby, M., Gillings, M. R., Madin, J. S., Nielsen, D. A., Paulsen, I. T., and Tetu, S. G. (2021). Trait dimensions in bacteria and Archaea compared to vascular plants. Ecol. Lett. 24, 1487–1504. doi: 10.1111/ele.13742
Whitham, T. G., Bailey, J. K., Schweitzer, J. A., Shuster, S. M., Bangert, R. K., LeRoy, C. J., et al. (2006). A framework for community and ecosystem genetics: from genes to ecosystems. Nat. Rev. Genet. 7, 510–523. doi: 10.1038/nrg1877
Wilson, D. S. (1997). Biological communities as functionally organized units. Ecology 78, 2018–2024.
Wilson, R. M., Zayed, A. A., Crossen, K. B., Woodcroft, B., Tfaily, M. M., Emerson, J., et al. (2021). Functional capacities of microbial communities to carry out large scale geochemical processes are maintained during ex situ anaerobic incubation. PLoS One 16:e0245857. doi: 10.1371/journal.pone.0245857
Wolf, A. A., Funk, J. L., Selmants, P. C., Morozumi, C. N., Hernández, D. L., Pasari, J. R., et al. (2021). Trait-based filtering mediates the effects of realistic biodiversity losses on ecosystem functioning. Proc. Natl. Acad. Sci. U.S.A. 118, e2022757118. doi: 10.1073/pnas.2022757118
Wright, E., and Vetsigian, K. (2016). Inhibitory interactions promote frequent bistability among competing bacteria. Nat. Commun. 7:11274. doi: 10.1038/ncomms11274
Yu, X., Polz, M. F., and Alm, E. J. (2019). Interactions in self-assembled microbial communities saturate with diversity. ISME J 13, 1602–1617. doi: 10.1038/s41396-019-0356-5
Keywords: microbiome, composition, decomposition, trait, tradeoff, climate, litter, legacy
Citation: Wang B and Allison SD (2022) Climate-Driven Legacies in Simulated Microbial Communities Alter Litter Decomposition Rates. Front. Ecol. Evol. 10:841824. doi: 10.3389/fevo.2022.841824
Received: 22 December 2021; Accepted: 24 February 2022;
Published: 04 April 2022.
Edited by:
Holger Pagel, University of Hohenheim, GermanyReviewed by:
Ember Morrissey, West Virginia University, United StatesCopyright © 2022 Wang and Allison. This is an open-access article distributed under the terms of the Creative Commons Attribution License (CC BY). The use, distribution or reproduction in other forums is permitted, provided the original author(s) and the copyright owner(s) are credited and that the original publication in this journal is cited, in accordance with accepted academic practice. No use, distribution or reproduction is permitted which does not comply with these terms.
*Correspondence: Bin Wang, d2J3ZW53dUBnbWFpbC5jb20=
Disclaimer: All claims expressed in this article are solely those of the authors and do not necessarily represent those of their affiliated organizations, or those of the publisher, the editors and the reviewers. Any product that may be evaluated in this article or claim that may be made by its manufacturer is not guaranteed or endorsed by the publisher.
From Frontiers
Learn more about the work of our research integrity team to safeguard the quality of each article we publish.