- Department of Chemical Engineering, Texas A&M University, College Station, TX, USA
The adaptive landscape for an industrially relevant phenotype is determined by the effects of the genetic determinants on the fitness of the microbial system. Identifying the underlying adaptive landscape for a particular phenotype of interest will greatly enhance our abilities to engineer more robust microbial strains. Visualizing evolution in real-time (VERT) is a recently developed method based on in vitro adaptive evolution that facilitates the identification of fitter mutants throughout the course of evolution. Combined with high-throughput genomic tools, VERT can greatly enhance the mapping of adaptive landscapes of industrially relevant phenotypes in microbial systems, thereby expanding our knowledge on the parameters that can be used for strain engineering.
Introduction
The majority of industrially relevant phenotypes in microbial systems involve multiple loci and mechanisms. The identities of these genetic determinants are generally not known, making the rational engineering of strains for these complex phenotypes challenging. Classical strain engineering for these traits generally involve several rounds of random mutagenesis followed by selection. However, with successive rounds of induced mutagenesis, mutations that are deleterious or have negative epistatic effects tend to accumulate by hitchhiking with beneficial alleles. If the desired trait can be coupled with growth, in vitro adaptive evolution can be used to improve the desired phenotype. This process is accomplished by applying a selective pressure so that beneficial mutants (mutants with increased fitness) can be obtained through the process of natural selection. The identities of the mutations residing in adaptive mutants obtained through natural selection or mutagenesis and their subsequent effects on cellular processes must be leveraged for further rational engineering. With advances in genomic tools, the genes and mechanisms involved can now be identified using combinations of whole-genome re-sequencing (Comas et al., 2012; Toprak et al., 2012), transcriptomics (Fitzgerald and Musser, 2001; Paulsen et al., 2001), proteomics (Callister et al., 2008; Boulais et al., 2010), and metabolomics (Ding et al., 2010; Goodarzi et al., 2010) studies.
In vitro adaptive evolution has been used extensively for the engineering of microbial system for both tolerances to inhibitors (Minty et al., 2011) and for enhanced product formation (Hu and Wood, 2010). The adaptive landscape, also known as fitness landscape, is used to describe the collection of relative fitness effects of each genotype under a specific condition. Detailed molecular characterization of adaptive mutants isolated from in vitro adaptive evolution experiments provides insights into the adaptive landscape for the phenotype of interest. Characterization of the adaptive landscape will significantly enhance our knowledge on the important parameters underlying complex phenotypes needed for the rational engineering of strains.
In adaptive evolution, clones are typically isolated from the evolving population after an arbitrarily elapsed time or at the end of the experiment. However, since the evolving population is heterogeneous, interclonal competition (clonal interference; Shaver et al., 2002; Kao and Sherlock, 2008) may lead to the extinction of beneficial mutants. Depending on the population structure during the course of evolution, the random isolation of adaptive mutants may fail to identify some adaptive mutations that arise during the course of the evolution. This review will (1) discuss factors that influence population structure and the impact of complex population dynamics on evolutionary engineering and (2) describe a novel evolutionary engineering method called visualizing evolution in real-time (VERT), that was recently developed to help address some of these limitations in traditional evolutionary engineering approach.
Adaptive Landscape
The idea of an adaptive landscape was first introduced as “surfaces of selective value” by Wright in 1931 (Wright, 1931, 1982, 1988). The adaptive landscape is a multi-dimensional surface representation of the biological fitness of an organism in a particular environment. In an adaptive landscape map for a specific condition, each genotype is correlated with a fitness value (see Figure 1 for an illustration). The resulting landscape can be flat with a single optimum where the evolving population is required to acquire a specific set of mutations, or can be rugged where the accessible optima will depend on the starting point within the landscape. It has been demonstrated that bacteria encounter both types of landscapes in evolution experiments (Orr, 2005; Weinreich et al., 2006; Gresham et al., 2008). Natural selection usually drives a population to the closest local optimum, but not necessarily the global optimum. Evolving populations tend to be trapped in suboptimal solutions (Lenski et al., 1998) in asexual systems. Thus to reach the global optimum, processes that allow for large “jumps” in the adaptive landscape, such as recombination and horizontal DNA transfer, are necessary to reach new regions of the adaptive landscape in a semi-rational manner. Recombination allows the combination of beneficial mutations with positive synergy and the removal of deleterious mutations acquired in the evolutionary process while horizontal gene transfer allows the acquisition of new functions.
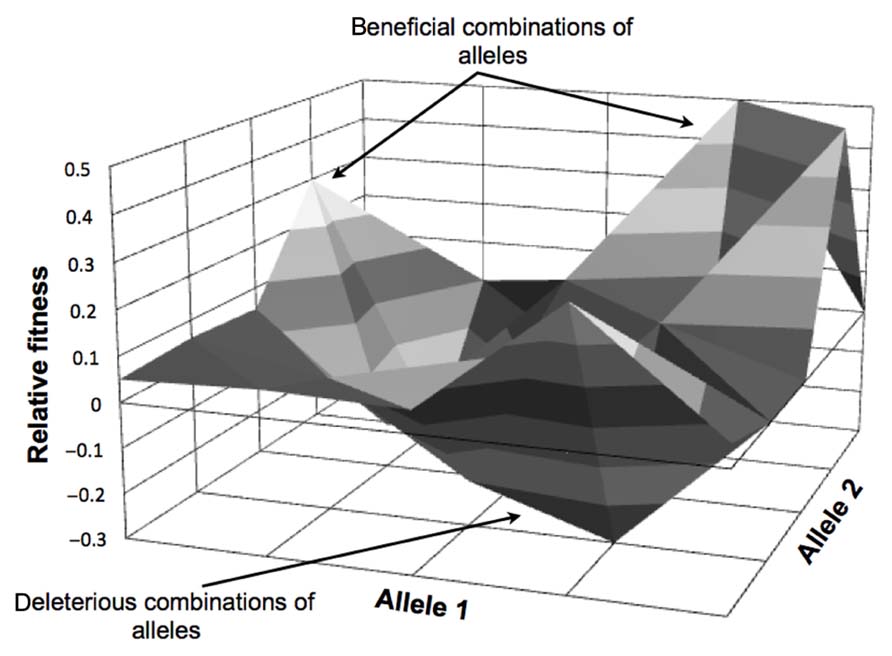
FIGURE 1. Simplified adaptive landscape for two alleles (for one background genotype in one condition). The figure depicts fitness values for beneficial (positive relative fitness values) and deleterious (negative relative fitness values) combinations of alleles.
Theories Governing Population Structure During Asexual Evolution
Numerous theories have been proposed for the population structure in in vitro adaptive evolution experiments. Several factors, including the selective pressure, size of the population, rate of mutations, frequency of beneficial mutations, and relative fitness of beneficial mutants, are involved in determining the population structure during evolution. In the simplest case, a well-adapted mutant rises in the population, and due to its increased fitness compared to background, the genotype will expand and eventually replace the parental population. This population structure is applicable to situations where the evolution is mutation-limited, the population size is small, and the time between the establishments of successive mutations is much larger than the time it takes for a beneficial mutant to fix in the population (strong positive selection). This theory, called clonal replacement (also called succession-fixation regime or strong-selection weak-mutation regime), implies that only one mutation can become fixed at a time, leading to successive complete selective sweeps (depicted in Figure 2A). The resulting population can be assumed to be homogeneous except during the periods when the beneficial mutant is sweeping through (Desai et al., 2007). However, when the mutations are established at a faster rate than the rate of fixation, multiple mutant lineages can coexist and compete for resources until one with the largest fitness advantage outcompetes all the other genotypes and become the next founding genotype for subsequent evolution. This theory, known as clonal interference (or one-by-one clonal interference), assumes that a single mutation can be fixed at a time, producing heterogeneous populations except immediately after the sweeping of the fittest mutant (depicted in Figure 2B); this theory focuses on the competition between different mutations with positive relative fitness (Gerrish and Lenski, 1998; Orr, 2000; Gerrish, 2001; Kim and Stephan, 2003; Campos and de Oliveira, 2004; Wilke, 2004). The two theories described above assume that only one beneficial mutation can be fixed at a time. However, if the population size is large enough or the rate of mutation is high enough, multiple mutations can occur in the same lineage before fixation, leading to the multiple-mutation model (Desai et al., 2007; depicted in Figure 2C). The importance of this third theory on population structure has been demonstrated in several theoretical and experimental studies (Yedid and Bell, 2001; Shaver et al., 2002; Bachtrog and Gordo, 2004). In general, the population size in laboratory conditions is large enough that either one-by-one clonal interference or multiple mutations models shape the population structure.
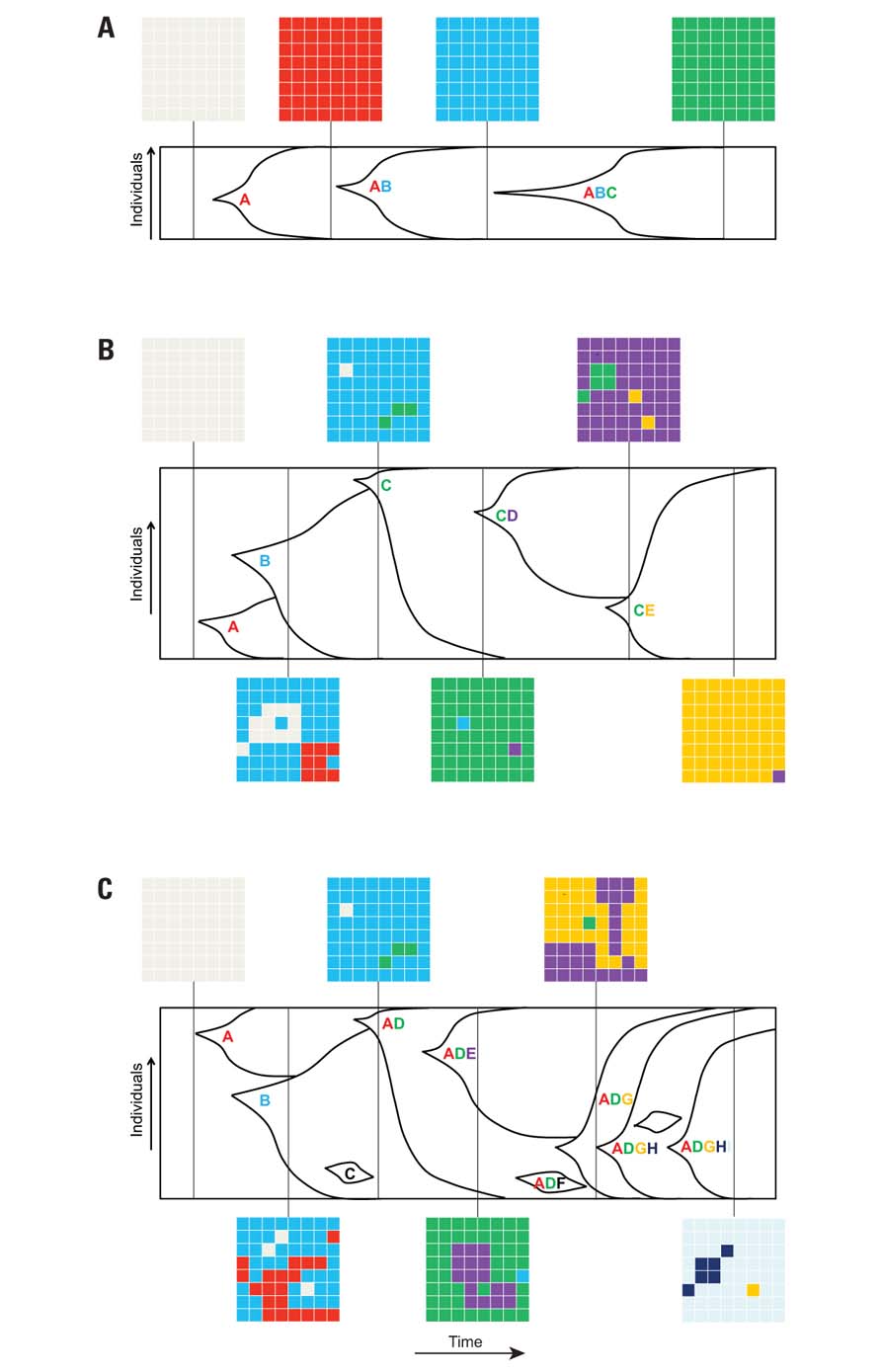
FIGURE 2. Theories governing population structure during asexual evolution. The graphs represent the population structure as a function of time during asexual evolution. The capital letters represent different beneficial mutations in the population. The gridded boxes represent a snapshot of the frequency of different genotypes in the population at that one point in time. (A) Clonal replacement model, where successive sweeps and fixation of different beneficial mutations take place in a small population; snapshots of the genotypes at different elapsed times show that the population is homogeneous except when the beneficial mutant is sweeping through the population. (B) Clonal interference model, where different adaptive mutants compete until one with the largest fitness advantage sweeps through and becomes the founding genotype for subsequent evolution (e.g., mutations A, B, and C compete until C completely takes over the population). (C) Multiple mutations model, where multiple mutations occur in the same lineage before fixation. In the latter two population structures, some adaptive mutations are lost from the population, and depending on when adaptive mutants are isolated, some mutants (and thus the underlying molecular mechanisms for adaptation) may not be identified.
Factors Influencing Population Dynamics
As mentioned above, factors such as mutation rate, relative fitness advantage, population size, and rate of beneficial mutations are important in shaping the population dynamics during evolution. We will briefly discuss each of these factors and how they impact adaptive evolution in different experimental systems. Since the evolution dynamics is dependent on the mutation rate, one would assume higher mutation rate to be advantageous for speeding up evolution by generating mutational diversity. However, an increase in mutation rates does not necessarily accelerate the pace of adaptation (Arjan et al., 1999). While a low mutation rate would result in a slow discovery of beneficial mutations, prolonged exposure to high mutation rate (such as the use of a mutator strain) increases the occurrence and accumulation of deleterious mutations as well as the hitchhiking of apparent “silent” mutations during the course of evolution, increasing the genetic load (Elena and Lenski, 2003; Gresham et al., 2008; Barrick et al., 2009). This is evidenced by the rarity of mutator strains in Lenski’s long-term adaptive evolution experiment with Escherichia coli; where mutators were found only after thousands of generations of evolution (Elena and Lenski, 1997; Sniegowski et al., 1997; Arjan et al., 1999; Vulic et al., 1999; Lenski et al., 2003) and the fitness advantage conferred by the mutator strains is most likely a result of overcoming a mutation-limited bottleneck during the evolution. Mutagens are often used to increase genetic diversity in evolution experiments. However, since it is not convenient to periodically mutagenize the evolving population, a controllable mutator system can be used, where the expression of mutator alleles can be induced only when needed (Selifonova et al., 2001).
The time it takes a beneficial mutation to become the majority in the population is called the fixation time and is an important factor in determining the population dynamics during evolution. This fixation time depends mainly on two factors, genetic drift and the fitness advantage of the beneficial mutation in comparison with the background, and is inversely proportional to the relative fitness advantage of the beneficial mutant (Lenski et al., 1991). A beneficial mutation with a 10% relative fitness advantage will become the majority of the population after approximately 250 generation in serial batch transfer experiments (Elena and Lenski, 2003) and 100 generations in continuous culture experiments (Gresham et al., 2008). Genetic drift is defined as the probability that a beneficial mutation survives extinction (Joanna, 2011). In in vitro adaptive evolution experiments, the main source of drift is genetic bottleneck due to random sampling. This phenomenon takes place when a significant amount of the population suddenly vanishes, as occurs when a fresh batch culture is inoculated from an overnight culture. The survival probability of an allele carrying a beneficial mutation that arose in the culture will depend on its proportion in the culture at the time of transfer and the amount of inoculum transferred; therefore there is a chance that it could be completely lost due to the stochasticity of sampling. In evolution experiments using serial batch transfers, genetic bottlenecks between transfers affect heterogeneity by transferring a small fraction of the population. A reduction of the effect of genetic bottleneck could be achieved by using continuous culture systems such as chemostats or turbidostats (Conrad et al., 2011), where a much smaller genetic bottleneck is present.
Visualizing Evolution in Real-Time
As stated above, the population sizes in most in vitro evolution experiments are large enough to result in heterogeneous populations due to the effects of clonal interference and multiple mutations. Thus, adaptive evolution experiments can significantly benefit from a more systematic isolation of adaptive mutants and ramping-up schedules for selective pressures. The VERT system was developed (Kao and Sherlock, 2008; Huang et al., 2011) to address these limitations in traditional adaptive evolution experiments. The basis for VERT is the use of isogenic, but differentially labeled (typically with fluorescent proteins) strains to seed the initial evolving population. As a beneficial mutant arises and expands in the population, the colored subpopulation that it belongs is expected to increase in proportion. Using fluorescent activated cell sorting (FACS), the relative proportions of each of the colored subpopulations at each point in time can be measured. Each sustained expansion in the proportion of a colored subpopulation is called an “adaptive event.” Thus, the tracking of the different colored subpopulations can serve as a tool for determining when a fitter mutant arises in the population.
The relative subpopulation frequency data collected throughout the course of adaptive evolution represent the history of the population. The observed increase in the relative proportion of a colored subpopulation from consecutive data points is assumed to be the result of the expansion of an adaptive mutant. Therefore, adaptive mutants can be isolated from samples based on the observed expansions and contractions, by sorting out the colored subpopulation that is expected to contain the adaptive mutant of interest. Since experimental data can suffer from noise, the identification of adaptive events may be challenging. Visual inspection of the data to identify adaptive events is a crude, but relatively effective method (Kao and Sherlock, 2008; Huang et al., 2011). However, since small changes in relative frequencies may be difficult to distinguish from noise, computational methods will provide less biased annotation of adaptive events; our group recently developed a supervised learning method for analysis of VERT data (Winkler and Kao, 2012).
The basic feature of the VERT system, the number of labeled subpopulations, is the aspect that can most readily be manipulated directly by the experimentalist, but is somewhat restricted by the available equipment and properties of the labels themselves. The number of fluorescent markers used represents distinct subpopulations that can be visualized during the course of an evolution experiment. VERT labels must have distinguishable emission spectra and preferably have no significant fitness effect in the condition of interest. Widely used fluorescent proteins such as GFP, YFP, and RFP can be detected on most FACS machines and usually have little effect on the physiology of their host strains. At a minimum, two labeled subpopulations are trivially required to observe population dynamics. Three subpopulations, employing RFP, GFP, and YFP labeled strains, have been used successfully (Kao and Sherlock, 2008; Huang et al., 2011) in fungal systems. Additional subpopulations can be included if suitable equipment is available. Simulated evolution may prove a useful tool for unraveling the connection between adaptive event discovery and initial population diversity.
Visualizing evolution in real-time-based in vitro adaptive evolution experiments can be used in either serial batch transfer or continuous culture systems. Provided that the different fluorescently marked strains show no significant fitness bias, then equal proportions of each strain maybe used to seed the population for evolution. Samples are then withdrawn and quantified using FACS every few generations to track the population dynamics. It is typically assumed that the adaptive mutant will expand until a fitter mutant arises in another subpopulation and expands sufficiently to impede its’ expansion. It is further assumed that the generation at which the expanding subpopulation has reached a maximum proportion will contain the largest fraction of the adaptive mutant responsible for the expansion, simplifying the isolation of the mutant considerably.
In traditional adaptive evolution experiments, selective pressure is generally ramped-up at arbitrarily chosen time intervals. An alternative to this approach, based on using a feedback controller to maintain selective pressure so that the overall population growth rate approaches a user-defined set point, was recently introduced by Toprak et al. (2012). Since the use of VERT allows the users to readily identify when adaptive events occur, it can be used to design a more systematic ramp-up schedule. For example, an increase in selective pressure could be applied when a minimum of 2 adaptive events are observed. The optimal frequency of ramp-up as a function of observed number of adaptive events may differ depending on the adaptive landscape for the phenotype of interest and needs to be investigated.
The isolated adaptive mutants can be further characterized to elucidate the molecular mechanisms of resistance in the selective pressure of interest. Whole-genome re-sequencing, transcriptomics, proteomics, and metabolomics analyses can be used to elucidate the evolutionary trajectories during the process of adaptive evolution. The availability and cost of whole-genome re-sequencing has improved significantly, but in most cases is still more expensive than transcriptome analysis using DNA microarrays. VERT tracks the individual subpopulations, making it easier to distinguish whether genome-wide perturbations observed in the transcriptional regulation found in different isolates arose independently or transitively without whole-genome re-sequencing data (if the isolates come from different colored subpopulations). Since not all the observed perturbations are involved in the complex phenotype of interest, common perturbations observed in independent lineages provide a level of confidence for their involvement. The potential adaptive mechanisms identified can serve as targets for further strain engineering.
The original development of VERT used the yeast Saccharomyces cerevisiae evolving under glucose-limited conditions; a three-colored VERT system was used to seed eight parallel populations (Kao and Sherlock, 2008). The VERT data from one of the populations is shown in Figure 3; generations and subpopulations from which adaptive mutants were isolated from are indicated. Detailed genotypic and transcriptomics analyses of the isolated adaptive mutants showed convergence in the perturbation of the protein kinase A regulatory network in independent lineages (Kao and Sherlock, 2008). Subsequent development and application of a two-colored VERT system in E. coli for n-butanol tolerance revealed previously undiscovered resistance mechanisms (Reyes et al., 2012).
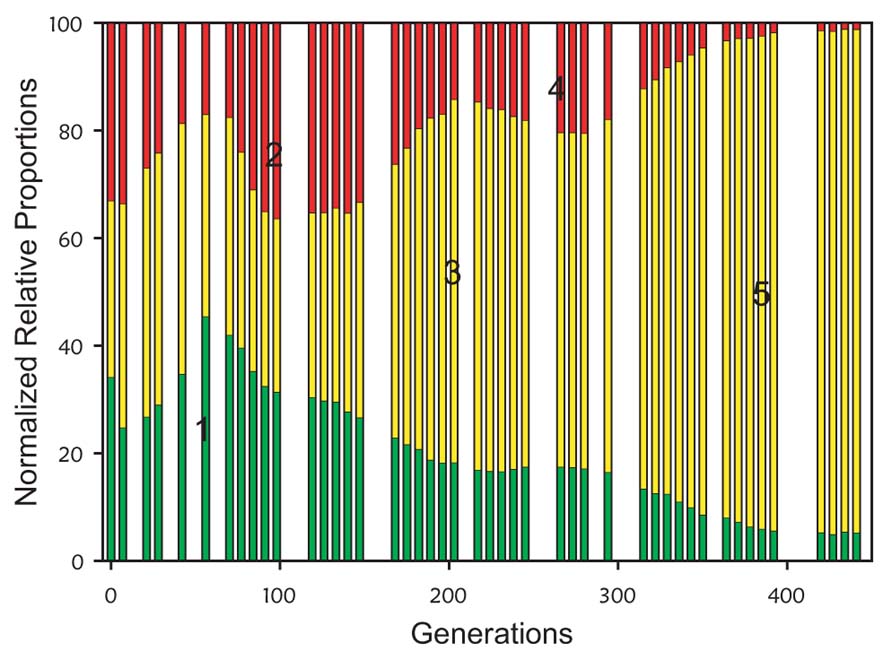
FIGURE 3. Example population dynamics from a three-colored VERT system (adapted from Kao and Sherlock, 2008). The colored bars represent the relative proportions of each colored subpopulation. An increase in the relative proportion of a colored subpopulation is indicative of the occurrence and expansion of an adaptive mutation in that subpopulation, and is defined as an adaptive event. Under the assumption that the adaptive mutant responsible for the specific adaptive event is at its’ highest proportion at the end of the sustained expansion, the adaptive mutants are isolated from the expanding subpopulation from the generation at the end of each expansion. The generation and colored subpopulation from which adaptive mutants were isolated are numbered 1–5.
Conclusion
Understanding the adaptive landscape for the phenotypes of interest is important for the rational engineering of strains. The use of evolutionary engineering has been used extensively to improve strains for complex phenotypes where there is limited knowledge on the associated genetic determinants. Advances in molecular biology tools in recent years have significantly improved our ability to obtain insights into the molecular mechanisms involved in the desire phenotypes in isolated adaptive mutants from in vitro evolution experiments. VERT was a recently developed tool for evolutionary engineering that can helps to provide a rough population structure for the evolving population, allowing the systematic isolation of adaptive mutants and ramp-up of selective pressure. Combined with advanced genomic tools, use of VERT in evolutionary engineering can help to gain additional insight regarding the adaptive landscape for complex phenotypes.
Conflict of Interest Statement
The authors declare that the research was conducted in the absence of any commercial or financial relationships that could be construed as a potential conflict of interest.
Acknowledgments
The authors would like to thank NSF MCB-1054276 (Katy C. Kao), and the Graduate Research Fellowship program (James Winkler) for partial financial support.
References
Arjan, J. A., Visser, M., Zeyl, C. W., Gerrish, P. J., Blanchard, J. L., and Lenski, R. E. (1999). Diminishing returns from mutation supply rate in asexual populations. Science 283, 404–406.
Bachtrog, D., and Gordo, I. (2004). Adaptive evolution of asexual populations under Muller’s ratchet. Evolution 58, 1403–1413.
Barrick, J. E., Yu, D. S., Yoon, S. H., Jeong, H., Oh, T. K., Schneider, D., Lenski, R. E., and Kim, J. F. (2009). Genome evolution and adaptation in a long-term experiment with Escherichia coli. Nature 461, 1243–1274.
Boulais, J., Trost, M., Landry, C. R., Dieckmann, R., Levy, E. D., Soldati, T., Michnick, S. W., Thibault, P., and Desjardins, M. (2010). Molecular characterization of the evolution of phagosomes. Mol. Syst. Biol. 6, 423.
Callister, S. J., McCue, L. A., Turse, J. E., Monroe, M. E., Auberry, K. J., Smith, R. D., Adkins, J. N., and Lipton, M. S. (2008). Comparative bacterial proteomics: analysis of the core genome concept. PLoS ONE 3, e1542. doi: 10.1371/journal.pone.0001542
Campos, P. R., and de Oliveira, V. M. (2004). Mutational effects on the clonal interference phenomenon. Evolution 58, 932–937.
Comas, I., Borrell, S., Roetzer, A., Rose, G., Malla, B., Kato-Maeda, M., Galagan, J., Niemann, S., and Gagneux, S. (2012). Whole-genome sequencing of rifampicin-resistant Mycobacterium tuberculosis strains identifies compensatory mutations in RNA polymerase genes. Nat. Genet. 44, 106–110.
Conrad, T. M., Lewis, N. E., and Palsson, B. O. (2011). Microbial laboratory evolution in the era of genome-scale science. Mol. Syst. Biol. 7, 509.
Desai, M. M., Fisher, D. S., and Murray, A. W. (2007). The speed of evolution and maintenance of variation in asexual populations. Curr. Biol. 17, 385–394.
Ding, M. Z., Zhou, X., and Yuan, Y. J. (2010). Metabolome profiling reveals adaptive evolution of Saccharomyces cerevisiae during repeated vacuum fermentations. Metabolomics 6, 42–55.
Elena, S. F., and Lenski, R. E. (1997). Long-term experimental evolution in Escherichia coli. VII. Mechanisms maintaining genetic variability within populations. Evolution 51, 1058–1067.
Elena, S. F., and Lenski, R. E. (2003). Evolution experiments with microorganisms: the dynamics and genetic bases of adaptation. Nat. Rev. Genet. 4, 457–469.
Fitzgerald, J. R., and Musser, J. M. (2001). Evolutionary genomics of pathogenic bacteria. Trends Microbiol. 9, 547–553.
Gerrish, P. J., and Lenski, R. E. (1998). The fate of competing beneficial mutations in an asexual population. Genetica 102–103, 127–144.
Goodarzi, H., Bennett, B. D., Amini, S., Reaves, M. L., Hottes, A. K., Rabinowitz, J. D., and Tavazoie, S. (2010). Regulatory and metabolic rewiring during laboratory evolution of ethanol tolerance in E. coli. Mol. Syst. Biol. 6, 378.
Gresham, D., Desai, M. M., Tucker, C. M., Jenq, H. T., Pai, D. A., Ward, A., DeSevo, C. G., Botstein, D., and Dunham, M. J. (2008). The repertoire and dynamics of evolutionary adaptations to controlled nutrient-limited environments in yeast. PLoS Genet. 4, e1000303. doi: 10.1371/journal.pgen.1000303
Hu, H., and Wood, T. K. (2010). An evolved Escherichia coli strain for producing hydrogen and ethanol from glycerol. Biochem. Biophys. Res. Commun. 391, 1033–1038.
Huang, M., McClellan, M., Berman, J., and Kao, K. C. (2011). Evolutionary dynamics of Candida albicans during in vitro evolution. Eukaryot. Cell 10, 1413–1421.
Kao, K. C., and Sherlock, G. (2008). Molecular characterization of clonal interference during adaptive evolution in asexual populations of Saccharomyces cerevisiae. Nat. Genet. 40, 1499–1504.
Kim, Y., and Stephan, W. (2003). Selective sweeps in the presence of interference among partially linked loci. Genetics 164, 389–398.
Kvitek, D. J., and Sherlock, G. (2011). Reciprocal sign epistasis between frequently experimentally evolved adaptive mutations causes a rugged fitness landscape. PLoS Genet. 7, e1002056. doi: 10.1371/journal.pgen.1002056
Lenski, R. E., Mongold, J. A., Sniegowski, P. D., Travisano, M., Vasi, F., Gerrish, P. J., and Schmidt, T. M. (1998). Evolution of competitive fitness in experimental populations of E. coli: what makes one genotype a better competitor than another? Antonie van Leeuwenhoek 73, 35–47.
Lenski, R. E., Rose, M. R., Simpson, S. C., and Tadler, S. C. (1991). Long-term experimental evolution in Escherichia coli. 1. Adaptation and divergence during 2,000 generations. Am. Nat. 138, 1315–1341.
Lenski, R. E., Winkworth, C. L., and Riley, M. A. (2003). Rates of DNA sequence evolution in experimental populations of Escherichia coli during 20,000 generations. J. Mol. Evol. 56, 498–508.
Minty, J., Lesnefsky, A., Lin, F., Chen, Y., Zaroff, T., Veloso, A., Xie, B., McConnell, C., Ward, R., Schwartz, D., Rouillard, J.-M., Gao, Y., Gulari, E., and Lin, X. (2011). Evolution combined with genomic study elucidates genetic bases of isobutanol tolerance in Escherichia coli. Microb. Cell Fact. 10, 18.
Paulsen, I. T., Chen, J., Nelson, K. E., and Saier, M. H. (2001). Comparative genomics of microbial drug efflux systems. J. Mol. Microbiol. Biotechnol. 3, 145–150.
Reyes, L. H., Almario, M. P., Winkler, J., Orozco, M. M., and Kao, K. C. (2012). Visualizing evolution in real time to determine the molecular mechanisms of n-butanol tolerance in Escherichia coli. Metab. Eng. (in press).
Selifonova, O., Valle, F., and Schellenberger, V. (2001). Rapid evolution of novel traits in microorganisms. Appl. Environ. Microbiol. 67, 3645–3649.
Shaver, A. C., Dombrowski, P. G., Sweeney, J. Y., Treis, T., Zappala, R. M., and Sniegowski, P. D. (2002). Fitness evolution and the rise of mutator alleles in experimental Escherichia coli populations. Genetics 162, 557–566.
Sniegowski, P. D., Gerrish, P. J., and Lenski, R. E. (1997). Evolution of high mutation rates in experimental populations of E. coli. Nature 387, 703–705.
Toprak, E., Veres, A., Michel, J.-B., Chait, R., Hartl, D. L., and Kishony, R. (2012). Evolutionary paths to antibiotic resistance under dynamically sustained drug selection. Nat. Genet. 44, 101–105.
Vulic, M., Lenski, R. E., and Radman, M. (1999). Mutation, recombination, and incipient speciation of bacteria in the laboratory. Proc. Natl. Acad. Sci. U.S.A. 96, 7348–7351.
Weinreich, D. M., Delaney, N. F., DePristo, M. A., and Hartl, D. L. (2006). Darwinian evolution can follow only very few mutational paths to fitter proteins. Science 312, 111–114.
Winkler, J., and Kao, K. C. (2012). Computational identification of adaptive mutants using the VERT system. J. Biol. Eng. 6, 3.
Keywords: adaptive evolution, strain development, population dynamics, evolutionary engineering
Citation: Reyes LH, Winkler J and Kao KC (2012) Visualizing evolution in real-time method for strain engineering. Front. Microbio. 3:198. doi:10.3389/fmicb.2012.00198
Received: 31 March 2012; Accepted: 14 May 2012;
Published online: 29 May 2012.
Edited by:
David Nielsen, Arizona State University, USAReviewed by:
Giuseppe Spano, University of Foggia, ItalyWensheng Lan, Shenzhen Entry-Exit Inspection and Quarantine Bureau, China
Keith Tyo, Northwestern University, USA
Copyright: © 2012 Reyes, Winkler and Kao. This is an open-access article distributed under the terms of the Creative Commons Attribution Non Commercial License, which permits non-commercial use, distribution, and reproduction in other forums, provided the original authors and source are credited.
*Correspondence: Katy C. Kao, Department of Chemical Engineering, Texas A&M University, 3122 TAMU, College Station, TX 77843-3122, USA. e-mail: kao.katy@gmail.com