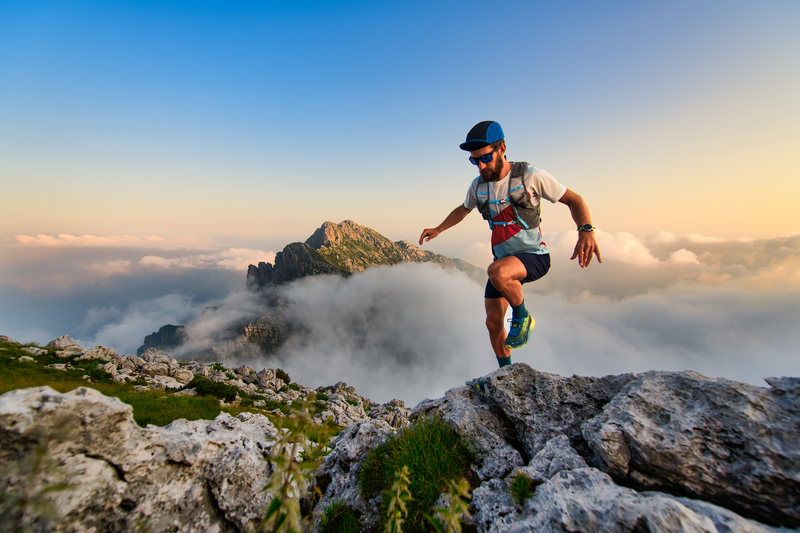
95% of researchers rate our articles as excellent or good
Learn more about the work of our research integrity team to safeguard the quality of each article we publish.
Find out more
ORIGINAL RESEARCH article
Front. Educ. , 19 November 2024
Sec. Digital Learning Innovations
Volume 9 - 2024 | https://doi.org/10.3389/feduc.2024.1465207
This article is part of the Research Topic Designing, implementing and evaluating self-regulated learning experiences in online and innovative learning environments View all 5 articles
Introduction: Students pursuing science, technology, engineering, and math (STEM) majors often struggle with essential skills critical to their academic success and future careers. Traditional self-regulated learning (SRL) training programs, while effective, require significant time investments from both students and instructors, limiting their feasibility in large lecture-based STEM courses.
Methods: This study investigates whether completion of three AI-powered virtual-human training modules—focused on planning, self-monitoring, and reflection—leads to increased use of corresponding MS Planner tools among STEM majors compared to a control group.
Results: Results indicate that students who did not complete the first two training modules were less likely to use MS Planner features for planning and self-monitoring; however, the reflection module did not yield comparable results.
Discussion: These findings highlight the potential of AI-powered virtual-human training as a scalable solution to enhance desirable learning behaviors among STEM majors, particularly in large and diverse classrooms. This research contributes to the understanding of effective interventions for fostering SRL behaviors in STEM education and suggests avenues for future refinement and implementation of digital training tools.
Educational research demonstrates that teaching students learning strategies and developing their self-regulatory skills can significantly improve their learning outcomes (Glick et al., 2023; Zimmerman, 2000, 2011). However, such training opportunities are rarely provided in K–12 curricula, which primarily focus on knowledge and skills dictated by content standards (Bernacki et al., 2020). Consequently, many students graduate high school and enter challenging undergraduate programs with only basic learning skills (ACT, 2008). This lack of emphasis on teaching students how to learn becomes evident when they encounter demanding coursework at the university level, where they are expected to manage their own learning independently.
Students often struggle to adopt effective learning strategies that are appropriate for the complexities of challenging courses in fields such as science, mathematics, and engineering. As a result, they frequently fail to study in ways that foster a deep understanding of the material, leading to poor performance (Bernacki et al., 2020). Many students who have not learned how to learn in high school face significant difficulties in their STEM coursework, which requires the integration of knowledge from various disciplines. In STEM fields, students must possess strong problem-solving and critical-thinking skills, enabling them to plan, analyze, and develop processes and projects that address real-world problems (Gao et al., 2020; Han et al., 2021). Self-regulated learning (SRL) skills such as planning, monitoring, and reflection are essential for breaking down complex tasks into manageable steps, setting goals, developing design processes, evaluating their effectiveness, and making necessary adjustments. These skills are crucial in STEM disciplines, where problems often demand multi-step solutions and an understanding of interconnected concepts (Han et al., 2021; Hsu and Fang, 2019). Additionally, STEM students often experience higher levels of stress compared to their peers in other disciplines. Thus, SRL skills are vital for helping students adapt their behaviors and enhance their performance in STEM courses (Park et al., 2019).
The need for training interventions that support undergraduates in developing these essential learning skills is clear. While substantial efforts have been made to enhance students’ learning and self-regulated learning abilities, many of these programs require significant time investments from both students and instructors, limiting their applicability in large lecture formats commonly used in early undergraduate STEM coursework (Bernacki et al., 2020). To effectively deliver skill training at scale, interventions must minimize instructor involvement, automate support, and ensure that the time spent developing learning skills does not significantly detract from time needed to study course content (Bernacki et al., 2020). With this in mind, we propose an alternative approach to face-to-face skill training and aim to investigate whether a brief AI-powered virtual-human training can help STEM majors effectively apply self-regulated learning strategies in their coursework. In the following section, we review self-regulated learning models and AI-powered skill training tools, and consider how to integrate virtual-human interventions into educational settings to deliver scalable SRL training within STEM environment.
Self-regulation encompasses internally originated thoughts, emotions, and actions guided by individual goals, involving metacognitive, cognitive, and motivational processes (Zimmerman, 2000, 2011). This multifaceted concept includes actions that enable learners to actively select and employ strategies, monitor their learning and behavior, and regulate these processes. Key actions include setting personal goals, adopting strategies to achieve them, monitoring self-performance, self-evaluating, and adapting future methods (Andrade, 2019; Pintrich, 2004). Additionally, self-regulated learners effectively manage their time and proactively seek assistance when necessary (Pintrich et al., 1994; Zimmerman, 2011).
Three main phases are typically used in various SRL models: a preparatory phase, a performance phase, and an appraisal phase (Panadero, 2017). In the preparatory phase, students examine the task and establish objectives. During the performance phase, they monitor their progress toward these goals and make adjustments as needed. In the appraisal phase, students review their progress, reflect on their performance, and modify their learning behaviors if necessary. Thus, SRL involves a cyclical feedback loop and continuous process (Callan et al., 2021; Zimmerman, 2000).
Research (e.g., Winne, 2006) indicates that many students lack awareness of efficient learning methods, may not realize that certain strategies are applicable across various tasks, and often struggle to accurately assess their own understanding, the quality of the information they acquire, and the appropriate timing and duration of study sessions. Despite its recognized value in enhancing learning and significantly impacting student performance and well-being, SRL is not typically acquired spontaneously (Efklides, 2019). Characterized by proactive processes such as goal setting, effort regulation, time management, and reflection, SRL can be developed and enhanced over time through deliberate practice and guidance (Broadbent, 2017; Panadero and Alonso-Tapia, 2014). Consequently, effective academic course design—regarding structure, content, and activities—is crucial. Studies in higher education (e.g., Miedijensky, 2023) suggest that course design and the integration of various digital tools can promote self-regulation and stimulate conscious reflection.
This study focuses on three SRL strategies: planning, self-monitoring, and reflection. Research shows that engaging in planning strategies, such as goal setting and time management, positively influences academic performance (Zimmerman, 2008). Students who actively engage in planning and goal setting outperform those who struggle with self-regulation (Davis et al., 2016; Sitzmann and Ely, 2011). Self-monitoring involves continuously assessing progress toward goals and adapting learning behaviors to enhance performance (Nelson and Narens, 1994). Planning and self-monitoring encourage students to select and execute relevant tasks while stimulating greater effort. Reflection entails making judgments about performance and assessing its quality (Rodgers, 2002). This process enables learners to transition from one experience to another with a deeper understanding of connections to other experiences and concepts (Miedijensky and Tal, 2016). Reflection in SRL typically occurs at the end of the learning process and involves self-assessment, identifying strengths and weaknesses, and adjusting ineffective strategies to achieve goals (Wong et al., 2019).
Rapid advancements in AI have led to the development of talking, human-like robots capable of understanding, conversing, and adapting in real-time. These virtual humans exhibit realistic facial expressions and movements, allowing learners to engage in increasingly human-like conversations (Cui and Liu, 2023; Xia et al., 2022). Literature indicates that non-verbal communication positively impacts human-robot task performance, enhancing efficiency and robustness against errors from miscommunication (Pauw et al., 2022). In educational contexts, virtual humans are digital avatars that simulate human-like interactions, integrating conversational AI technology with 3D animation. These avatars serve as powerful educational tools, moving beyond mere entertainment to enable personalized learning experiences (Zhang and Fang, 2024).
AI tutoring systems can provide tailored guidance, support, or feedback by customizing learning content to match individual students’ learning patterns or knowledge levels. This student-centered approach enhances motivation, facilitates skill development, and provides tailored responses to diverse learners (Bhutoria, 2022; Hwang et al., 2020). Given their strong anthropomorphic characteristics, ability to provide tailored responses (Zawacki-Richter et al., 2019), and capacity to build meaningful connections with students (Pelau et al., 2021), AI-powered virtual humans hold great promise for delivering scalable skill training.
The virtual-human training was developed using AI-powered bot-building technology that utilizes natural language processing (NLP) to understand, analyze, and respond to human speech. This training is designed to teach students three specific self-regulated learning strategies essential for maintaining an effective study environment in undergraduate STEM courses: planning, self-monitoring, and reflection. Each of the three modules that comprise the Virtual-Human training educates students about relevant strategies and identifies corresponding tools available within their learning environment.
The virtual human incorporates four conversation design principles aimed at enhancing user experience. The first principle emphasizes recognizing and responding to user emotions. This involves designing interactions where the bot acknowledges the emotional states of users, such as confusion, frustration, or satisfaction. For example, if the bot detects confusion, it might offer clarification to alleviate concerns (e.g., “Here’s a recap of the third module on Reflection”). The second principle focuses on fallback responses for situations where the virtual human encounters unfamiliar questions or inputs. These responses serve as a safety net, preventing abrupt conversation endings and maintaining user engagement. For instance, the bot could respond with, “I’m sorry, I did not quite catch that. Could you rephrase?” This encourages users to clarify their input and facilitates smoother interactions. The third principle prioritizes delivering clear and concise responses to user queries, ensuring comprehension and minimizing cognitive load. By avoiding unnecessary complexity, the bot enhances communication and reduces the likelihood of misunderstandings (e.g., “You do not have to do everything at once. Instead, break big tasks into smaller blocks”). Finally, the fourth principle emphasizes facilitating contextual understanding. This involves designing interactions that maintain context throughout conversations. By leveraging context-awareness techniques, the bot can anticipate user intent and reduce repetition, leading to more intuitive and efficient exchanges (e.g., “Interesting. Thanks for sharing! On to the next tip”).
In each module of the virtual-human training, the bot educates students about relevant learning strategies and identifies corresponding tools available in MS Planner, a project management app that students use to plan, monitor, and reflect on course assignments. We trace evidence of the transfer of SRL activities by examining student activity on the MS Planner LMS. We hypothesize that students who complete each of the three virtual-human training modules will demonstrate greater utilization of the corresponding MS Planner tools designed to support self-regulated learning processes, including planning, self-monitoring, and reflection, compared to a control group.
This study was conducted at a large public polytechnic in Singapore, which enrolls 13,000 students annually across 36 diploma programs. A total of 146 second-year pre-employment training (PET) students from the School of Informatics and IT participated in the study. These students were enrolled in a four-credit, 60-hour Data Science Essentials course, designed to equip them with knowledge and skills in the emerging field of data science. The course covered topics such as data exploration and analysis techniques, aimed at helping students discover new insights from data to support informed, data-driven decisions. Participants included 105 males and 41 females, with a median age of 18 years, and they represented diverse ethnic backgrounds, including Chinese, Malay, and Indian. Random assignment was conducted at the section level, allowing for either the virtual-human training or control material to be incorporated into each course site. 96 students were randomly assigned to receive access to the virtual human, while the remaining 50 were assigned to the control material, which consisted of a digital training kit presented as an FAQ (Frequently Asked Questions) document. This control material provided training on the same self-regulated learning strategies as those offered to the experimental group.
The final course grade was primarily based on a six-week group project. The objectives of the group project were to: (a) familiarize students with the Cross-Industry Standard Procedures for Data Mining (CRISP-DM) framework; (b) perform data analytics and visualizations; and (c) communicate business insights based on a dataset of their choice. As part of their project, students were required to produce a proposal in PowerPoint and deliver a 15-min in-class presentation detailing how they conducted the “Business Understanding” and “Data Understanding” phases—the first two steps of the Data Science lifecycle as guided by the CRISP-DM framework—within a selected industry. Students had six weeks to complete their group project and were provided access to MS Planner for project planning, self-monitoring, and reflection. Evidence of self-regulated learning (SRL) activity was gathered by examining relevant student activity on the MS Planner LMS. Logs of student activity were extracted from MS Planner and enriched with metadata describing the names and types of tools accessed. The number of clicks on relevant MS Planner tools was tracked, aggregated into weekly counts, and classified based on the specific self-regulated learning strategies each tool was designed to support. All relevant MS Planner tools are defined in Table 1.
Students in the experimental group were given access to the virtual-human training during week 1. They were given the opportunity to complete each of the three virtual-human training modules at a time, place and pace of their choosing. A training module was considered complete only if students completed the entire module. Activity logs of each of the three training modules were extracted from the virtual-human LMS. An overview of the topics addressed in each training module is presented in Table 2.
In the first week of the course, students completed a consent form and an initial survey capturing demographic characteristics. They were then randomly assigned at the section level to either the experimental (virtual-human training) or control (digital training kit) condition. During Weeks 2–7 of the semester, students had the flexibility to complete their assigned training at a time, place, and pace of their choosing. They were also given access to MS Planner to plan, monitor, and reflect on their group project, which was conducted during the same weeks. Specifically, students had one week for planning (Week 2), two weeks for self-monitoring (Weeks 3–4), and three weeks for reflection (Weeks 5–7). A guide to MS Planner, explaining how to create plans, manage tasks, and track task progression, was provided to students in Week 1. Additionally, project assessment criteria, broken down into levels and categories of performance, were shared with the students in Week 2.
This study is specifically designed to examine the effect of the AI-powered virtual-human intervention on students’ self-regulated learning (SRL) behavior through the analysis of clicks on relevant MS Planner features. This approach aligns with established research methodologies, as evidenced by studies that have utilized clickstream data to assess the impact of digital training interventions on SRL (e.g., Akpinar et al., 2020; Azevedo et al., 2016; Cogliano et al., 2022; Liu et al., 2023). For instance, Azevedo et al. (2016) investigated differences in cognitive and metacognitive SRL processes between experimental and control groups by analyzing student clicks on an SRL palette. Similarly, Cogliano et al. (2022) employed self-regulated learning analytics using student log data to predict and support students performing poorly in an undergraduate STEM course. These studies demonstrate the efficacy of such data in gaging student engagement and behavioral changes in digital learning environments.
To explore our research hypothesis regarding the relationship between completion of the virtual-human training and greater utilization of SRL tools within MS Planner, we conducted logistic regression. Completion of each of the three virtual-human training modules served as a categorical predictor variable, dummy coded as 1 (incomplete) or 2 (complete). Students in the experimental group were compared to those in the control condition, which was dummy coded as 0. The use of relevant MS Planner features designed to support specific learning strategies was treated as a binary outcome variable, dummy coded as 0 (no use) or 1 (use). We used logistic regression due to the binary nature of the outcome variable and the need to model the probability of an event occurring (i.e., use of relevant MS Planner tools). This method relies on several key assumptions, including independence of observations and the absence of multicollinearity among predictors. The first assumption—independence of observations—requires that each observation is independent of others. Our dataset consists of individual observations that are not derived from repeated measures or matched pairs, fulfilling this assumption. The second assumption—absence of multicollinearity—assumes that the predictor variables are not perfectly correlated. We assessed multicollinearity among our categorical predictor variables using the Generalized Variance Inflation Factor (GVIF). The obtained GVIF value was 1.002, indicating minimal or no multicollinearity among the predictors. A GVIF value close to 1 suggests that the predictors in our model are not highly correlated, reducing concerns about multicollinearity affecting the robustness of our regression results. Thus, our logistic regression analysis is unlikely to be biased by high correlations among predictor variables.
We then conducted linear regression to examine the effect of completion of each training module on the number of clicks on relevant MS Planner buttons. Completion of each module was treated as a binary predictor variable, dummy coded as 0 (incomplete) or 1 (complete). The number of clicks on relevant MS Planner buttons, aggregated to weekly counts, served as a continuous outcome variable. Evidence of MS Planner tool usage that supports planning was traced only in the first week of the group project (Week 2 of the course), as planning should occur prior to task engagement according to self-regulated learning theory. Use of tools that support self-monitoring and reflection was traced throughout the project duration. Effects with p < 0.05 were considered statistically significant.
Our hypothesis posited that students completing AI-powered virtual-human training which focuses on self-regulation, would demonstrate greater use of MS Planner tools designed to support these learning processes compared to a control group. We examined students’ engagement with each of the three training modules—Planning, Self-monitoring and Reflection—constituting the virtual-human training. Logs of student activity in these modules were extracted from the virtual-human LMS. We then examined the use of relevant MS Planner tools recommended during the training. Relevant MS Planner tools for Planning included listing weekly tasks on the MS Planner board, setting task start and due dates, prioritizing tasks, and assigning task owners. Tools for Self-monitoring included checking task status and marking tasks as complete, while use of the Comments box to reflect on progress toward goals was noted as a trace of Reflection. We evaluated the effect of the virtual-human training on these self-regulated learning behaviors using logistic regression. Of the 96 students in the experimental group, 19.8% (n = 19) completed the entire course (dummy coded as 2), while 80.2% (n = 77) completed it partially (dummy coded as 1). Control-group students (dummy coded as 0) were given access to a digital training kit. The results of the logistic regression model are detailed below and reported in Table 3.
The logistic regression model was statistically significant (p < 0.05). Students in the experimental group who did not complete the entire module were less likely to use MS Planner tools for planning compared to students in the control group (β = −2.55, SE = 1.43). Specifically, those who did not complete the first training module were 0.079 times less likely to use relevant MS Planner tools compared to control-group students (95% CI = [0.07, 0.54]). The confidence interval for the odds ratio does not include 1, indicating a statistically significant association between the predictor and outcome variables. This suggests that failing to complete the first module of the virtual-human training significantly decreases the likelihood of using relevant MS Planner tools designed to support Planning.
The logistic regression model was statistically significant (p < 0.05). Experimental-group students who did not complete the entire module were less likely to use MS Planner tools for self-monitoring compared to students in the control group (β = −1.12, SE = 0.55). Specifically, those who did not complete the second training module were 0.323 times less likely to use relevant MS Planner tools than control-group students (95% CI = [0.109, 0.965]). The confidence interval for the odds ratio does not include 1, indicating a statistically significant association between the predictor and outcome variables. This suggests that failing to complete the second module of the virtual-human training significantly decreases the likelihood of using relevant MS Planner tools designed to support Self-monitoring.
Completion of the third training module—whether entirely or partially—was not associated with an increased likelihood of using relevant MS Planner tools compared to control-group students (p > 0.05). The confidence interval for the odds ratio includes 1 (95% CI = [0.014, 3.771]), indicating that we cannot conclude a positive relationship between the predictor and outcome variables in the model. These results suggest that the third training module does not significantly affect the likelihood of using MS Planner tools designed to support Reflection (β = −1.48, SE = 1.43).
Linear regression was conducted to examine the effect of completing each of the three training modules on the number of clicks on relevant MS Planner buttons designed to support self-regulated learning processes. Completion of each virtual-human training module was treated as a binary predictor variable, dummy coded as 0 (incomplete) or 1 (complete). The number of clicks on relevant MS Planner buttons, aggregated into weekly counts, served as the continuous outcome variable. Relevant buttons for Planning included listing weekly tasks on the MS Planner board, setting task start and due dates, prioritizing tasks, and assigning task owners. Relevant buttons for Self-monitoring included checking task status and marking tasks as complete. For Reflection, we tracked the number of clicks on the Submit button during an open-ended reflective activity. The results of the linear regression are described below and reported in Table 4.
The results of the linear regression indicate that students who completed the first training module tended to click on relevant MS Planner buttons more often (β = 1.07); however, this difference was not statistically significant at the 5% level (β = 1.07, SE = 0.66, p > 0.05). Thus, completion of the first training module does not have a statistically significant effect on the number of clicks on MS Planner buttons that support Planning.
The results indicate that completion of the second training module significantly predicts the number of clicks on relevant buttons (β = 0.59, SE = 0.35, p < 0.05). This suggests that students who completed the second training module clicked on buttons supporting Self-monitoring, on average, 0.59 times more than students who did not complete the module.
The results indicate that completion of the third training module does not have a statistically significant effect on the number of clicks on MS Planner buttons that support Reflection (β = 0.11, SE = 0.18, p > 0.05). Although students who completed the third training module tended to click on the Submit button more often (β = 0.11), this difference was not statistically significant at the 5% level.
This study aimed to examine whether AI-powered virtual-human training could help students effectively apply self-regulated learning (SRL) strategies—such as planning, self-monitoring, and reflection—in their STEM courses. MS Planner was integrated into the study as the tool for measuring and supporting the targeted SRL behaviors. We tracked specific MS Planner tools to determine if students who completed the virtual-human training demonstrated greater utilization of tools designed to support self-regulated learning processes.
Students who completed the first virtual-human training module were more likely to engage in planning activities using MS Planner tools, highlighting the training’s effectiveness in enhancing essential project management skills. The finding that experimental-group students who did not complete the first module were significantly less likely to use MS Planner for planning suggests that failing to complete this module decreases the likelihood of utilizing these features. This aligns with previous research indicating that goal-setting interventions can significantly enhance students’ planning and organizational skills (Davis et al., 2016; Sitzmann and Ely, 2011). While Winne (2006) and Efklides (2019) noted that digital interventions alone may not suffice for sustained planning behaviors without additional support, the blended learning format of this course provided opportunities for tutors to offer necessary guidance. However, completion of the training module did not significantly affect the number of clicks on MS Planner buttons that support Planning. As outlined in the Procedure section, students were advised to spend 1 week planning and using MS Planner to log and visualize tasks and workflows required to meet project objectives. By the time they set up tasks in MS Planner, discussions and agreements on those tasks had already been established, making frequent task changes unnecessary during the planning phase.
The results show that experimental-group students who did not complete the second training module were less likely to use MS Planner tools for self-monitoring compared to the control group. This finding is supported by analyses of user reviews of MS Planner on Capterra, which indicate that many users find the tool “…unintuitive, cumbersome, and not suitable for beginners.” (Capterra, 2024). These reviews may explain why first-time MS Planner users who did not complete the training modules were less inclined to utilize the relevant tools. It suggests that students who failed to familiarize themselves with MS Planner and did not complete the virtual-human training were less likely to engage with its features for planning and self-monitoring.
Interestingly, completion of the third training module did not significantly affect the use of reflective features in MS Planner. This suggests that reflection requires more than digital prompts and might benefit from more interactive or in-depth reflective practices. A systematic review by Chan and Lee (2021) highlights that time and student availability significantly influence motivation and engagement in reflective activities. In addition to their group project, participants had other assignments, limiting their ability to engage in reflective practices. The review indicates that engaging in reflection demands commitment and can be perceived as tedious by students, which may explain why participants in our Data Science Essentials course chose not to utilize MS Planner tools for reflection and only briefly reflected on their project during an end-of-course presentation.
The findings underscore the potential of AI-powered virtual-human training as a scalable solution for enhancing self-regulated learning among STEM majors. Traditional SRL training often falls short in K-12 education, leaving students ill-prepared for rigorous undergraduate coursework. Many enter university with only basic learning strategies, which can hinder academic performance in complex subjects. This study highlights the importance of integrating innovative digital solutions to address this gap. The significant impact of virtual-human training on the use of MS Planner tools for planning and self-monitoring suggests that technology-enhanced interventions can effectively foster essential SRL skills. For educators and curriculum designers, this emphasizes the need to incorporate such digital training methods within existing course frameworks. By embedding virtual-human modules into early STEM courses, institutions can equip students with the tools necessary to develop effective study habits and learning strategies without overwhelming them with additional coursework.
The results indicate that while the initial modules on planning and self-monitoring were beneficial, the third module on reflection did not yield the same outcomes. This finding encourages designers to critically evaluate and refine training content to ensure that each component is engaging and impactful. Further research is needed to understand how to enhance reflective practices within digital training formats. Incorporating interactive elements or peer feedback mechanisms could increase engagement and effectiveness during the reflection phase. Additionally, the study’s design, which minimizes instructor involvement, highlights a key advantage of AI-powered training: scalability. Traditional SRL programs often require significant time investments from both students and instructors, but this virtual-human training approach could be implemented in large lecture formats without sacrificing educational quality. This shift could democratize access to essential learning strategies for a broader range of students, particularly in large and diverse classrooms where personalized attention is challenging.
Despite promising results indicating a positive association between completion of virtual-human training and increased utilization of self-regulated learning strategies, this study has several limitations. First, the low completion rate of the training module (20%) raises concerns about the representativeness of the sample and the generalizability of the findings to the broader student population. Second, the absence of baseline assessments to measure and adjust for demographic variables such as age, gender, ethnicity, and first-generation college student status may have resulted in uneven distribution across these categories between experimental and control groups, potentially impacting the internal validity of the results. Finally, this study relies solely on quantitative measures derived from clickstream data. Finally, this study relies solely on quantitative measures derived from clickstream data. While the quantitative approach aligns with prior research in the field (e.g., Azevedo et al., 2016; Cogliano et al., 2022), the use of qualitative methods, such as real-time student feedback or interviews, could provide additional insights into students’ self-regulated learning behaviors.
Future studies should explore strategies to improve completion rates of virtual-human training modules among students. Implementing incentives or gamification elements within the training could enhance engagement and motivation, thereby increasing participation rates and ensuring a more representative sample for analysis. Additionally, future research should conduct thorough baseline assessments across demographic variables such as age, gender, ethnicity, and first-generation college student status. This approach would allow for identifying and adjusting any initial differences between experimental and control groups, ensuring more equitable comparisons and strengthening the internal validity of the findings. Finally, future studies should replicate this research in diverse educational settings and with a broader spectrum of student populations to provide insights into how virtual-human training impacts self-regulated learning behaviors across different institutional contexts and demographics.
This study advances our understanding of effective training interventions needed to help STEM majors develop essential self-regulated learning skills. There is a pressing need for scalable and efficient training programs that do not demand substantial investments of time and effort from both students and instructors, particularly in large undergraduate courses. Our findings suggest that brief, fully automated virtual-human training can help STEM majors effectively apply SRL strategies in their coursework. This training approach is promising because it is low-cost, requires minimal time investment from both students and instructors, and is easily scalable to new courses.
The virtual-human training effectively enhanced students’ planning and self-monitoring behaviors, as evidenced by their increased use of relevant MS Planner features. Specifically, students who completed the training were more likely to engage in planning and self-monitoring activities, demonstrating its effectiveness in promoting these critical SRL behaviors. However, the training did not significantly impact the use of reflective features, suggesting that deeper or more interactive reflective practices might be necessary to fully develop this aspect of SRL. These findings imply that virtual-human interventions can be integrated into large-scale educational settings to enhance learning outcomes without requiring extensive resources. However, co-facilitation with human tutors could help develop more interactive and deeper reflective activities, encouraging meaningful reflection. Leveraging advanced AI techniques and expanding the virtual-human LMS could also tap into the richness of data to provide personalized feedback and support tailored to individual learner needs at different stages of SRL.
Overall, this study provides compelling evidence that AI-powered virtual-human interventions can support SRL in STEM education, offering a scalable, cost-effective solution that maintains educational quality while reducing the resource burden on both students and instructors.
The raw data supporting the conclusions of this article will be made available by the authors, without undue reservation.
The studies involving humans were approved by Temasek Polytechnic Ethics Committee. The studies were conducted in accordance with the local legislation and institutional requirements. The participants provided their written informed consent to participate in this study. Written informed consent was obtained from the individual(s) for the publication of any potentially identifiable images or data included in this article.
DG: Conceptualization, Methodology, Writing – original draft, Writing – review & editing. SM: Conceptualization, Writing – original draft, Writing – review & editing. HZ: Project administration, Writing – original draft.
The author(s) declare that no financial support was received for the research, authorship, and/or publication of this article.
The authors declare that the research was conducted in the absence of any commercial or financial relationships that could be construed as a potential conflict of interest.
All claims expressed in this article are solely those of the authors and do not necessarily represent those of their affiliated organizations, or those of the publisher, the editors and the reviewers. Any product that may be evaluated in this article, or claim that may be made by its manufacturer, is not guaranteed or endorsed by the publisher.
ACT (2008). The forgotten middle: ensuring that all students are on target for college and career readiness before high school. Iowa City, IA: ACT.
Akpinar, N.-J., Ramdas, A., and Acar, U. (2020). Analyzing student strategies in blended courses using clickstream data. In Proceedings of the 13th international conference on educational data mining. Georgia: EDM. (6–17).
Andrade, H. L. (2019). A critical review of research on student self-assessment. Front. Educ. 4:87. doi: 10.3389/feduc.2019.00087
Azevedo, R., Martin, S. A., Taub, M., Mudrick, N. V., Millar, G. C., and Grafsgaard, J. (2016). “Are pedagogical agents’ external regulation effective in fostering learning with intelligent tutoring systems?” in Proceedings of the 13th international conference on intelligent tutoring systems—Lecture notes in computer science 9684. eds. A. Micarelli, J. Stamper, and K. Panourgia (Amsterdam: Springer), 197–207.
Bernacki, M. L., Vosicka, L., and Utz, J. C. (2020). Can a brief, digital skill training intervention help undergraduates “learn to learn” and improve their STEM achievement? J. Educ. Psychol. 112, 765–781. doi: 10.1037/edu0000405
Bhutoria, A. (2022). Personalized education and artificial intelligence in the United States, China, and India: a systematic review using a human-in-the-loop mode. Comput. Educ. Artif. Intell. 3:100068. doi: 10.1016/j.caeai.2022.100068
Broadbent, J. (2017). Comparing online and blended learners' self-regulated learning strategies and academic performance. Internet High. Educ. 33, 24–32. doi: 10.1016/j.iheduc.2017.01.004
Callan, G. L., Rubenstein, L. D., Ridgley, L. M., Neumeister, K. S., and Hernández Finch, M. E. (2021). Self-regulated learning as a cyclical process and predictor of creative problem-solving. Educ. Psychol. 41, 1139–1159. doi: 10.1080/01443410.2021.1913575
Capterra. (2024). Microsoft planner reviews. Available at:. (https://www.capterra.com.sg/reviews/1010402/microsoft-planner?time_used=LessThan6Months#facets).
Chan, C. K. Y., and Lee, K. K. W. (2021). Reflection literacy: a multilevel perspective on the challenges of using reflections in higher education through a comprehensive literature review. Educ. Res. Rev. 32:100376. doi: 10.1016/j.edurev.2020.100376
Cogliano, M., Bernacki, M. L., Hilpert, J. C., and Strong, C. L. (2022). A self-regulated learning analytics prediction-and-intervention design: detecting and supporting struggling biology students. J. Educ. Psychol. 114, 1801–1816. doi: 10.1037/edu0000745
Cui, L., and Liu, J. (2023). Virtual human: a comprehensive survey on academic and applications. IEEE Access 11, 123830–123845. doi: 10.1109/ACCESS.2023.3329573
Davis, D., Chen, G., van der Zee, T., Hauff, C., and Houben, G. J. (2016). “Retrieval practice and study planning in MOOCs: exploring classroom-based self-regulated learning strategies at scale” in Adaptive and adaptable learning, 9891. eds. K. Verbert, M. Sharples, and T. Klobučar (Cham: Springer).
Efklides, A. (2019). Gifted students and self-regulated learning: the MASRL model and its implications for SRL. High Abil. Stud. 30, 79–102. doi: 10.1080/13598139.2018.1556069
Gao, X., Li, P., Shen, J., and Sun, H. (2020). Reviewing assessment of student learning in interdisciplinary STEM education. Int. J. STEM Educ. 7:24. doi: 10.1186/s40594-020-00225-4
Glick, D., Bergin, J., and Chang, C. (2023). Supporting self-regulated learning and student success in online courses. Hershey, PA: IGI-Global.
Han, J., Kelley, T., and Knowles, J. G. (2021). Factors influencing student STEM learning: self-efficacy and outcome expectancy, 21st century skills, and career awareness. J. STEM Educ. Res. 4, 117–137. doi: 10.1007/s41979-021-00053-3
Hsu, Y. S., and Fang, S. C. (2019). “Opportunities and challenges of STEM education” in Asia-Pacific STEM teaching practices. eds. Y. S. Hsu and Y. F. Yeh (Cham: Springer), 1–16.
Hwang, G. J., Xie, H., Wah, B. W., and Gašević, D. (2020). Vision, challenges, roles and research issues of artificial intelligence in education. Comput. Educ. Artif. Intell. 1:100001. doi: 10.1016/j.caeai.2020.100001
Liu, Y., Fan, S., Xu, S., Sajjanhar, A., Yeom, S., and Wei, Y. (2023). Predicting student performance using clickstream data and machine learning. Educ. Sci. 13:17. doi: 10.3390/educsci13010017
Miedijensky, S. (2023). “Metacognitive knowledge and self-regulation of in-service teachers in an online learning environment” in Supporting self-regulated learning and students success in online courses. eds. D. Glick, J. Bergin, and C. Chang (Pennsylvania: IGI global).
Miedijensky, S., and Tal, T. (2016). Reflection and assessment for learning in enrichment science courses for the gifted. Stud. Educ. Eval. 50, 1–13. doi: 10.1016/j.stueduc.2016.05.001
Nelson, T. O., and Narens, L. (1994). “Why investigate metacognition?” in Metacognition: Knowing about knowing. eds. J. Metcalfe and A. P. Shimamura (Cambridge, MA: MIT Press), 1–25.
Panadero, E. (2017). A review of self-regulated learning: six models and four directions for research. Front. Psychol. 8:28. doi: 10.3389/fpsyg.2017.00422
Panadero, E., and Alonso-Tapia, J. (2014). How do students self-regulate? Review of Zimmerman's cyclical model of self-regulated learning. Ann. Psychol. 30, 450–462. doi: 10.6018/analesps.30.2.167221
Park, C. L., Williams, M. K., Hernandez, P. R., Agocha, V. B., Carney, L. M., DePetris, A. E., et al. (2019). Self-regulation and STEM persistence in minority and non-minority students across the first year of college. Soc. Psychol. Educ. 22, 91–112. doi: 10.1007/s11218-018-9465-7
Pauw, L. S., Sauter, D. A., van Kleef, G. A., Lucas, G. M., Gratch, J., and Fischer, A. H. (2022). The avatar will see you now: support from a virtual human provides socio-emotional benefits. Comput. Hum. Behav. 136:107368. doi: 10.1016/j.chb.2022.107368
Pelau, C., Dabija, D.-C., and Ene, I. (2021). What makes an AI device human-like? The role of interaction quality, empathy and perceived psychological anthropomorphic characteristics in the acceptance of artificial intelligence in the service industry. Comput. Hum. Behav. 122:106855. doi: 10.1016/j.chb.2021.106855
Pintrich, P. R. (2004). A conceptual framework for assessing motivation and self-regulation learning in college students. Educ. Psychol. Rev. 16, 385–407. doi: 10.1007/s10648-004-0006-x
Pintrich, P. R., Roeser, R. W., and De Groot, E. A. M. (1994). Classroom and individual differences in early adolescents' motivation and self-regulated learning. J. Early Adolesc. 14, 139–161. doi: 10.1177/027243169401400204
Rodgers, C. (2002). Defining reflection: another look a John Dewey and reflective thinking. Teach. Coll. Rec. 104, 842–866. doi: 10.1177/016146810210400402
Sitzmann, K., and Ely, K. (2011). A meta-analysis of self-regulated learning in work-related training and educational attainment: what we know and where we need to go. Psychol. Bull. 137, 421–442. doi: 10.1037/a0022777
Winne, P. H. (2006). How software technologies can improve research on learning and bolster school reform. Educ. Psychol. 41, 5–17. doi: 10.1207/s15326985ep4101_3
Wong, J., Baars, M., Davis, D., Van Der Zee, T., Houben, G.-J., and Paas, F. (2019). Supporting self-regulated learning in online learning environments and MOOCs: a systematic review. Int. J. Hum. Comput. Int. 35, 356–373. doi: 10.1080/10447318.2018.1543084
Xia, Q., Chiu, T. K. F., Lee, M., Temitayo, I., Dai, Y., and Chai, C. S. (2022). A self-determination theory design approach for inclusive and diverse artificial intelligence (AI) K-12 education. Comput. Educ. 189:104582. doi: 10.1016/j.compedu.2022.104582
Zawacki-Richter, O., Marín, V. I., Bond, M., and Gouverneur, F. (2019). Systematic review of research on artificial intelligence applications in higher education – where are the educators? Int. J. Educ. Technol. High. Educ. 16:39. doi: 10.1186/s41239-019-0171-0
Zhang, H., and Fang, L. (2024). Successful chatbot design for polytechnic students in Singapore. Educ. Media Int. 60, 224–241. doi: 10.1080/09523987.2023.2324585
Zimmerman, B. J. (2000). “Attaining self-regulation: a social cognitive perspective” in Handbook of self-regulation. eds. M. Boekaerts, P. R. Pintrich, and M. Zeidner (San Diego, CA: Academic Press), 13–39.
Zimmerman, B. J. (2008). Investigating self-regulation and motivation: historical background, methodological developments, and future prospects. Am. Educ. Res. J. 45, 166–183. doi: 10.3102/0002831207312909
Keywords: AI, self-regulated learning, STEM education, skill training, cognitive strategies, virtual humans
Citation: Glick D, Miedijensky S and Zhang H (2024) Examining the effect of AI-powered virtual-human training on STEM majors’ self-regulated learning behavior. Front. Educ. 9:1465207. doi: 10.3389/feduc.2024.1465207
Received: 15 July 2024; Accepted: 30 October 2024;
Published: 19 November 2024.
Edited by:
Laurence Habib, Oslo Metropolitan University, NorwayReviewed by:
Leif Knutsen, Simula Metropolitan Center for Digital Engineering, NorwayCopyright © 2024 Glick, Miedijensky and Zhang. This is an open-access article distributed under the terms of the Creative Commons Attribution License (CC BY). The use, distribution or reproduction in other forums is permitted, provided the original author(s) and the copyright owner(s) are credited and that the original publication in this journal is cited, in accordance with accepted academic practice. No use, distribution or reproduction is permitted which does not comply with these terms.
*Correspondence: Danny Glick, ZGFubnkuZ2xpY2tAb3JhbmltLmFjLmls
Disclaimer: All claims expressed in this article are solely those of the authors and do not necessarily represent those of their affiliated organizations, or those of the publisher, the editors and the reviewers. Any product that may be evaluated in this article or claim that may be made by its manufacturer is not guaranteed or endorsed by the publisher.
Research integrity at Frontiers
Learn more about the work of our research integrity team to safeguard the quality of each article we publish.