- 1Department of Educational Science, Saarland University, Saarbruecken, Germany
- 2Department for Teaching and Studies, Saarland University, Saarbruecken, Germany
Self-regulated learning (SRL) is positively associated with improved learning achievements during all educational phases. Despite playing an important role in conveying SRL strategies to their students, pre-service often lack knowledge about SRL and imparting it. Therefore, addressing SRL and teaching SRL strategies to students seems relevant to pre-service teacher training. The present study aims to analyze pre-service teachers’ SRL profiles in asynchronous and synchronous digital learning environments and compares their influence on training effectiveness. As part of a pre-post design, a total of N = 141 pre-service teachers participated in the study, and questionnaires on SRL strategy use and an SRL knowledge test were used. A latent profile analysis indicated a three-class solution (low, moderate, high SRL), revealing significant differences regarding SRL strategy use but not for SRL knowledge. These findings enable a person-centered approach to develop digital learning environments and provide insight into specific learner behavior.
1 Introduction
Digital learning environments are platforms or applications providing learning material that enable students to study course contents remotely, often on their terms, with or without direct monitoring by the teacher (Arguel et al., 2017; Kümmel et al., 2020). Current research suggests that digital learning environments can positively impact learning (Clark et al., 2016) by, e.g., motivating learners (Chang et al., 2017). They also offer the advantage of reaching many people at the same time. Digital learning environments are particularly suitable for teaching interdisciplinary skills on which students can work regardless of time and location (Broadbent et al., 2020). One of these interdisciplinary skills is self-regulated learning (SRL), the ability to plan and regulate thoughts, feelings, and actions during the learning process, to achieve previously set learning goals (Zimmerman, 2000). SRL comprises cognitive, motivational, and metacognitive components (Boekaerts, 1999): Cognitive components include conceptual and strategic knowledge and the ability to apply appropriate learning strategies. Motivational components include activities to initiate and sustain learning. Metacognitive components comprise planning, self-monitoring, and reflection on one’s own learning process (Boekaerts, 1999).
On the one hand, SRL is a competency that can be fostered directly or indirectly. Direct promotion of SRL often includes the impartation of specific SRL strategies and knowledge via training programs led by trained teachers. In this case, the learners are aware that they are explicitly learning SRL strategies. Indirect promotion is often unconscious and can occur, for example, by providing a learning environment that provides the possibility for SRL but does not instruct the use if SRL strategies explicitly (Dignath and Veenman, 2021). On the other hand, SRL is an essential prerequisite for successful learning in digital environments (Broadbent and Poon, 2015) where learners often work independently. Since they often have limited interaction with lecturers, learners must use SRL strategies to determine with which learning materials to engage, and when (Broadbent et al., 2021; Kizilcec et al., 2017). Research has shown that students who possess self-regulation skills are more likely to succeed in digital learning environments and complete tasks without interruption compared to those who lack these skills (Alhazbi and Hasan, 2021; Cho and Shen, 2013; Lee and Choi, 2011). It has been observed that SRL positively affects learning in all educational domains (Dent and Koenka, 2016) which is why SRL should be fostered as early as possible. Pre-service teachers are particularly relevant to supporting their students’ SRL, but they require skills to promote the construct among students. They often lack knowledge about teaching SRL strategies (Glogger-Frey et al., 2018). Therefore, promoting SRL among pre-service teachers as early as possible is crucial, to develop the necessary skills to teach their students explicit learning strategies and serve as a model for their implementation in the classroom (Peeters et al., 2014).
Digital learning environments are an ideal platform for providing pre-service teachers with SRL strategies in an adaptable way. Designing effective digital learning environments requires considering students’ individual needs for successful learning. A person-centered approach seems suitable to fulfill this requirement because individual learning profiles can be analyzed and provide a basis for future learning environments. Hence, this research aims to examine individual SRL characteristics of pre-service teachers in two digital learning environments—asynchronous e-learning and synchronous online seminar—and to compare the effectiveness of the training regarding the acquisition of SRL strategies and SRL knowledge.
2 Theoretical background
2.1 Self-regulated learning
SRL is “a process whereby learners activate and sustain cognitions, affects, and behaviors that are systematically oriented towards the attainment of personal goals” (Zimmerman, 2011, p. 1). SRL is a proactive process that demands a high level of SRL strategy use (Zimmerman, 2008). Furthermore, it is a general interdisciplinary competency that supports students in planning, implementing, and reflecting on their learning processes and functions across various disciplines (Bembenutty, 2011). Models for describing SRL distinguish between component and process models. SRL contains cognitive, metacognitive, and motivational components that component models entail (e.g., Boekaerts, 1999). In contrast to component models that focus on constituent parts of SRL, Zimmerman’s (2000) social cognitive model of SRL depicts it as a cyclical process, dividing the learning process into different phases that represent the central components of self-regulation. Due to the consideration of SRL’s different components as well as its circularity, Zimmerman’s (2000) model is the most popular model for intervention studies and also the basis for the intervention in the present study, and the following section describes it further.
2.1.1 Social cognitive model of SRL
Zimmerman (2000) divides the learning process into three phases: forethought, performance, and self-reflection. These involve cognitive, metacognitive, and motivational components. The cyclical character of SRL is due to the phases occurring sequentially and previous learning processes impact prospective strategy use.
The “forethought phase” represents the first phase of the learning process. Initially, learners set goals, plan their behavior, and choose adequate learning strategies (metacognitive component) that seem beneficial for reaching the desired goals. Moreover, learners must motivate themselves to start the learning process. One important motivational component is self-efficacy, the self-belief that one can master the requirements to solve the task (Bandura, 2006), which often determines the ability to self-motivate (motivational component). If self-efficacy for a specific task is at a high level, starting the task is highly probable.
The “performance phase” refers to the actual learning activity. Cognitive strategies, such as repeating and organizing, particularly help to consolidate acquired knowledge by integrating new information into existing cognitive structures (cognitive component). In this phase, motivational strategies play an important role in maintaining the learning process. Learning can only continue if learners can motivate themselves sufficiently (Alhazbi and Hasan, 2021), have enough volitional strategies to protect themselves from distractions and focus their attention (Dörrenbächer and Perels, 2015; Valenzuela et al., 2020; motivational component). Throughout the whole learning process, learners must self-control their actions to adapt their strategy use if necessary. Self-observation is one essential metacognitive strategy that enables learners to adjust their learning behavior in case of a discrepancy between their goals and the current state (De Bruin and van Gog, 2012). By monitoring the learning process, learners identify the learning strategies that have proved less effective and consequently merit dismissal (metacognitive component).
In the following “self-reflection phase,” learners undertake a self-evaluation by contrasting forethought phase goals with the achieved results (metacognitive component). This comparison entails a selection of productive strategies for prospective learning and a refusal of strategies that did not prove successful and/or an adaptation of goals because the initial goals turned out to be unrealistic (Zimmerman, 2000). For this reason, learners must be able to evaluate their achievements, which closely links to causal attribution (Brun et al., 2021). In terms of self-regulated learning, an internal variable attribution would be the most suitable because it promotes learners’ perception of responsibility and controllability. Different options exist for a self-reaction of the learning results. Self-satisfaction with one’s own abilities has turned out to be beneficial for learners, resulting in increased self-efficacy. All in all, the successful accomplishment and positive evaluation of a task consolidate the learner’s self-motivational beliefs and outcome expectations, causing positive emotions (motivational component)—for example, pride—to impact the choice of strategies in the proximate forethought phase (Zimmerman, 2000).
2.1.2 Pre-service teachers’ SRL
Pre-service teachers’ self-regulation ability and the use of different SRL strategies should be examined separately. The ability to self-regulate requires declarative knowledge of SRL, defined as “facts, figures, rules, relations” (Cooke et al., 2000, p. 153) about SRL as well as procedural knowledge of SRL (“procedures, sequences and actions required for task performance,” Cooke et al., 2000, p. 153). Given that procedural knowledge is difficult to operationalize, this study is focused on declarative knowledge of SRL. However, declarative knowledge of SRL and the use of SRL strategies should be differentiated, since knowledge of SRL strategies does not imply their use (Dörrenbächer-Ulrich et al., 2021; Foerst et al., 2017).
Regarding declarative SRL knowledge, research indicates that pre-service teachers’ declarative knowledge of SRL strategies is often fragmented (Lawson et al., 2019) and disorganized (Ohst et al., 2015). Furthermore, some pre-service teachers demonstrate bad-quality metacognitive strategy knowledge (Glogger-Frey et al., 2018), leading to ineffective teaching of SRL strategies. Accordingly, they seldom use SRL strategies spontaneously (Engelmann et al., 2021), or use those that are less effective (Fryer and Vermunt, 2018). Consistent with these findings, Lawson et al. (2019) suggested that pre-service teachers may not be aware of the effectiveness of their SRL strategies and, thus, do not use them or may interrupt their strategy use too early.
Regarding SRL strategy use, successful learners utilize effective learning strategies to achieve their goals (Perry et al., 2018), using not more but more varied SRL strategies than less successful learners (Nandagopal and Ericsson, 2012). Increased strategy use results in deeper information processing and more effective learning. In a study with 366 pre-service teachers, Vosniadou et al. (2021) found a positive relationship between cognitive and metacognitive strategies and academic achievement. Furthermore, there is evidence that pre-service teachers use few SRL strategies to manage their learning (De Bruin and van Merriënboer, 2017).
Pre-service teachers play a crucial role in supporting their students’ SRL, but they need to possess the skills necessary to promote this construct among their students. As teachers, they can aid students’ SRL development in various ways, including creating challenging assignments, granting independence in decision-making, or providing opportunities for self-evaluation (Dignath and Veenman, 2021). Pre-service teachers not only impart knowledge directly to their students but also serve as role models who use SRL strategies themselves (Peeters et al., 2014). This makes them essential for helping students acquire declarative SRL knowledge and learn how to use SRL strategies effectively. According to Buzza and Allinotte (2013), pre-service teachers who possess good SRL skills are more likely to understand the concepts related to promoting SRL. In addition, Gordon et al. (2007) showed that teachers who have good SRL skills are more likely to create a classroom environment that supports their students’ SRL by encouraging mastery-oriented learning. Therefore, promoting SRL among pre-service teachers is crucial for developing their teaching skills and modeling SRL to their students as early as possible.
2.2 Digital learning environments
Digital learning environments provide different formats for conveying knowledge. Their lack of physical encounters magnifies the important role of communication. Learning can involve distinguishing between synchronous and asynchronous communication. The real-time interaction of the instructor with the learners characterizes synchronous communication (Alhazbi and Hasan, 2021; Amiti, 2020; Shahabadi and Uplane, 2015). Synchronous technologies, e.g., video conference or live chat, enable carrying out this purpose (Giesbers et al., 2014; Watts, 2016), facilitating online instruction and learner-oriented interaction (Shahabadi and Uplane, 2015). Conversely, asynchronous communication occurs independent of time, enabling non-simultaneous participation of the instructor and the learners (Amiti, 2020). The instructor prepares educational material, and communication occurs via asynchronous technologies, such as discussion boards, email, or assessments (Alhazbi and Hasan, 2021; Reese, 2015; Watts, 2016). Both communication methods offer advantages that the next section discusses.
2.2.1 Synchronous digital learning environments
In synchronous digital learning environments, a direct interaction between instructor, learner, and peers occurs. This causes a feeling of collaboration and increases the information flow in the team (Hrastinski et al., 2010). The learners feel less isolated from the instructor and their peers (Amiti, 2020; Francescucci and Rohani, 2019), leading to increased individual participation and more intense interaction (Hrastinski, 2008). This allows for instant feedback among learners and teachers, which may leave less time for reflection but does allow for direct correction of misconceptions (Giesbers et al., 2014). In her literature review, Watts (2016) summarized 24 papers and concluded that synchronous online interactions positively impacted students’ engagement in an online learning environment, due to instant feedback and interaction. Despite high-level social interaction in synchronous learning environments, introverted students may participate more because they feel comfortable and less stressed, due to the spatial distance (Amiti, 2020). Communication in synchronous learning environments is faster and more convenient than in asynchronous digital learning environments. A study by Oztok et al. (2013) examined both synchronous chats and asynchronous forums, with 222 university students from a Canadian university. The research found that synchronous messages tend to be shorter, contain more social language, and are easier to read than asynchronous messages. Synchronous learning is well-suited for group projects since synchronous tools offer a high level of media richness that facilitates a deeper learning process (Rockinson-Szapkiw and Wendt, 2015). Additionally, synchronous communication is suitable for socializing, organizing activities, and discussing less complex tasks (Duncan et al., 2012).
2.2.2 Asynchronous digital learning environments
In asynchronous learning environments, learning is time-independent, which benefits students with different learning paces and strategies (Kim et al., 2018; So, 2016). Students must manage their learning but can work at their own pace (Broadbent and Poon, 2015). Asynchronous communication leads to increased engagement with the learning material (Alhazbi and Hasan, 2021; Watts, 2016) because learners have more time available for interacting with, processing, and reflecting on the learning content (Lucas et al., 2014). For example, the ability to pause video lectures reduces cognitive load and leads to deeper processing of the material, due to increased time on task (Nieuwoudt, 2020). Asynchronous messages in a digital learning space use more academic language and are longer than private synchronous messages, providing increased opportunities for peer interaction due to more reflective content (Oztok et al., 2013). More time spent on writing longer messages encourages reflection as well as the presentation of complex issues (Hrastinski et al., 2010). Researchers suggest using asynchronous interactions for group work, particularly when complex content requires reflection before posting (Griffiths and Graham, 2010; Watts, 2016). A study by Hrastinski (2008) examined the learning outcomes of Swedish and Argentinian students in two synchronous and two asynchronous learning environments. The researcher conducted the study over four weeks and focused on the topic of knowledge management. The study found that asynchronous learning environments led to increased cognitive participation. When students have time to reflect on a problem and construct their response instead of giving a spontaneous answer, they can engage in critical thinking and produce better-quality responses, resulting in higher cognitive achievement than synchronous learning produces (Ogbonna et al., 2019). Given that asynchronous learning involves less personal interaction with peers and does not require spontaneous reactions, this type of communication could especially suit introverted or shy learners (Amiti, 2020).
To summarize, both synchronous and asynchronous learning environments can promote learning but require different learning strategies (Shahabadi and Uplane, 2015) and the ability to self-regulate the learning process.
2.3 Self-regulated learning in digital learning environments
SRL plays an important role when it comes to learning in synchronous or asynchronous digital environments and can be seen twofold: On the one hand, SRL is an essential prerequisite for successful learning in digital learning environments, on the other hand, relevant research indicates that SRL is a fosterable skill in university students (Theobald, 2021). The next chapters provide an overview of SRL double role for learning in digital learning environments.
2.3.1 SRL as a prerequisite for learning in digital environments
Learning in digital learning environments differs from learning in analog settings because the self-contained work on online assignments requires students to plan, monitor, and evaluate their learning process. Time management, critical thinking, metacognitive strategies, and other self-regulating learning processes have proved particularly effective in enhancing learning outcomes in those environments (Broadbent and Poon, 2015), making SRL essential for successful online learning. Due to the high degree of autonomy in digital learning environments, learners must independently plan, engage with, and reflect on learning material while staying motivated (Barnard-Brak et al., 2010; Broadbent and Poon, 2015). Current research findings highlight the importance of using SRL strategy in digital learning environments. Highly self-regulated learners have a greater task completion rate (Ainscough et al., 2019) and achieve better results in digital learning environments than their less self-regulated peers (Ning and Downing, 2015). Therefore, SRL is a crucial factor and represents an important prerequisite for successful learning in those environments. Due to the context-bound nature of SRL, individual differences in motivation and learning strategy use can arise between online and traditional learners (Meijs et al., 2019), calling for more differentiated view on individual learners, for example by using a person-centered approach. Developing a research approach toward a person-centered examination of SRL has occurred by analyzing subgroups of persons with different SRL profiles, to gain insight into different behaviors during learning (Broadbent and Fuller-Tyszkiewicz, 2018). This approach involves cluster or latent profile analysis (LPA) to categorize learners into definite subgroups.
2.3.2 SRL as improvable competence for learning in digital environments
Given the positive correlation between SRL and online academic performance (Cheng et al., 2023), its development among students as early as possible is essential. Teaching pre-service teachers how to teach SRL strategies to their students in the classroom can achieve this. While digital learning environments generally demand SRL, they also provide an opportunity to promote SRL knowledge and SRL strategy use (Zheng, 2016). This is possible through a direct approach, by explicitly presenting learners with specific SRL strategies, or indirectly, by using digital learning environments that require learners to use SRL strategies but that do not encompass explicit training (Dignath and Veenman, 2021).
E-learning environments, as a type of digital learning environment, are “instruction that is delivered via a digital device that is intended to promote learning” (Clark and Mayer, 2016, p. 7), suitable for both direct and indirect promotion of SRL. However, research indicates that the implementation of SRL training can achieve higher-level academic achievement and an increase in student SRL strategy use (Kramarski and Michalsky, 2010). Even if the implementation of most SRL training occurs in analog learning environments, evidence for the promotion of SRL in digital learning environments is growing. The most common SRL training approach is the direct conveyance of SRL strategies.
Two direct SRL e-learning trainings for students were conducted by van der Beek et al. (2020) and Bellhäuser et al. (2016), strengthening the findings that SRL is promotable via e-learning and classroom seminars are comparably effective in promoting SRL. The presented studies obtained promising results in terms of course performance, knowledge of SRL, and its use, implying the promotability of SRL within direct e-learning environments. However, the studies largely neglect the student teachers target group, despite their important role as multipliers of SRL knowledge and strategy use. Alkhasawnh and Alqahtani (2019) provided direct SRL training to n = 70 education students from Saudi Arabia. They implemented an e-learning course, with SRL strategies for the experimental group and without SRL strategies for the control group. In comparison to the control group, the findings indicated an increase of SRL and course performance in the experimental group. Dörrenbächer-Ulrich et al. (2019) used e-learning training to directly promote SRL among n = 57 pre-service teachers and revealed an increase of SRL traits, especially in the motivational subscale, as well as increased SRL knowledge in the experimental condition.
2.4 Individual differences in SRL
The SRL person-centered approach to detect individual differences is relatively new, resulting in only a few studies on the topic and ambiguous findings (Ainscough et al., 2019). For instance, researchers have found different numbers of SRL profiles among university students but only a few studies exist that focus on pre-service teachers as a target group:
A two-profile solution with competent and less competent self-regulated learners was revealed by Huang et al. (2021) with n = 68 pre-service teachers from an educational college in the United States, who were using an intelligent web browser to design technology lessons. The findings showed that competent learners were superior regarding the quality of designing good lessons.
A three-profile solution was found most often in the current literature for university students (Ainscough et al., 2019; Esnaashari et al., 2023; Lin et al., 2021). The profiles included students using many SRL strategies, referred to as a high SRL profile, students using a medium number of SRL strategies (medium SRL profile), and students using few SRL strategies (low SRL profile). For preservice teachers, a three-profile solution could be confirmed by Heikkilä et al. (2012) and Muwonge et al. (2020).
A four-profile solution was indicated for university students from Great Britain (Araka et al., 2022), Germany (Dörrenbächer and Perels, 2016), and the United States (Schwam et al., 2020). At one end of the range were “exemplary” students as well as “good self-regulators” and students with the profile “poor self-regulators” on the other end. Students with average SRL scores were located in the middle of the range. Unlike Araka et al. (2022), Schwam et al. (2020), and Dörrenbächer and Perels (2016) found an SRL profile that they labeled “conflicting SRL.” These students showed high scores on some subscales (e.g., self-efficacy) but low scores on other subscales (e.g., time-planning) related to SRL.
A five-profile solution was found by Barnard-Brak et al. (2010) in a sample of n = 279 university students in the USA. The analyses revealed that the minimal self-regulators had the lowest GPAs, and competent self-regulators had the highest.
The presented findings illustrate different numbers of profiles, based on SRL for university students and pre-service teachers, making a specific decision on the exact number of profiles difficult. The examination of SRL profiles offers insight into learners’ prerequisites, enabling a categorization of SRL competencies. This offers the possibility of promoting a lack of competencies for students with low SRL profiles or adapting learning environments to students with already high-level SRL skills. Furthermore, the SRL profiles provide a more differentiated view of students learning. For example, there could be students who are very motivated but lack the use of metacognitive strategies which could not be revealed in generalized SRL measures.
2.5 The present study
The aforementioned findings show that SRL is a prerequisite for learning in digital environments and, simultaneously, a competency that can be improved in digital learning environments. However, knowledge about learners’ individual differences in SRL is still sparse and studies analyzing students’ SRL profiles provide different numbers of profiles and neglect the special target group of pre-service teachers, raising the need for further investigation. Moreover, the examination of SRL’s promotability due to individual preferences in synchronous and asynchronous learning environments needs further research.
Therefore, the present study examines the following three research questions:
1. Are there different SRL profiles for pre-service teachers and if so, how many?
2. How does an SRL training affect pre-service teachers with different SRL profiles?
3. What are the differences between synchronous and asynchronous learning environments regarding the effectiveness of SRL training for the different SRL profiles?
To examine the research questions, SRL profiles are deduced, based on the participants’ SRL scores divided into cognitive, metacognitive, and motivational SRL components. In the second step, the training effectiveness, represented by increased SRL knowledge and strategy use, is investigated, based on the profiles. Finally, the study examines the influence of the different communication styles (synchronous, asynchronous) on the training effectiveness, depending on the SRL profile.
3 Materials and methods
3.1 Sample
The study involved N = 145 pre-service teachers from a university in southwestern Germany. Four participants were excluded from the final sample, due to numerous missing values in the pretest, leaving a total of n = 141 participants. The participants were between 20 and 49 years old, with an average age of M = 24.24 years (SD = 4.50). On average, the participating students were in their seventh semester of studies (SD = 2.09). Most participants identified as female (n = 102), n = 37 identified as male, and one participant reported a diverse gender identity. One person did not provide gender identity information.
3.2 Procedure
The study was conducted following the Declaration of Helsinki, no institutional approval was necessary by local law. We conducted a quasi-experimental study using a pre-post design with two different training conditions—asynchronous e-learning and a synchronous online seminar. Time (pretest and posttest) was measured as a within-subject factor, while training condition was measured as a between-subject factor. We selected the two groups from preexisting compulsory courses in educational science. Each course (n = 11) was randomly assigned to one of the two training conditions, though a randomized assignment of single participants was not possible. Thus, we assigned six courses to the asynchronous e-learning condition (n = 90), and five courses to the synchronous online seminar condition (n = 51). At the start of each course session, a digital online survey was conducted to collect pretest measurements and, after 6 weeks we conducted posttest measurements. Both questionnaires took about 15–20 min to complete, and participation was voluntary and unpaid. All participants confirmed their participation by informed consent, and their data was anonymized using individualized codes. During the six-week period between the measurements, the participants in the two conditions gained knowledge about SRL and the use of SRL strategies. The participants in the asynchronous e-learning condition received knowledge through an online platform, while the participants in the synchronous online seminar condition received theoretical input and exercises during weekly online classroom sessions led by a course teacher. The content was identical in both conditions; the only difference was the learning environment in which it was presented.
3.3 Intervention
The SRL training intervention occupied a period of 6 weeks in two learning environments, namely, asynchronous e-learning and a synchronous online seminar. Zimmerman (2000) SRL model provided the basis for the training content and materials. Their positive impact on SRL had been evaluated in an analog training program (anonymous authors). To ensure the attribution of the effects to the different learning environments, the same learning content was used in both conditions. Thus, all learning environments covered the whole SRL process with forethought, performance, and reflection phases. Specific SRL strategies became six modules in the e-learning environment and six online sessions in the synchronous online seminar. More detailed information appears in Table 1.
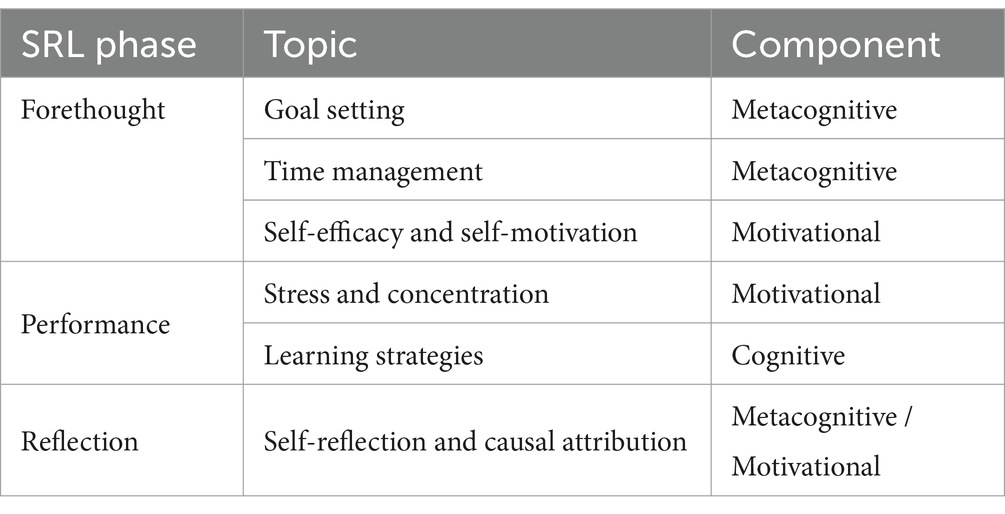
Table 1. Overview of the training topics and their classification into different SRL phases and components.
3.3.1 Synchronous online seminar
A trained instructor led the weekly synchronous online seminar using MS Teams. Each session had an equivalent structure and aimed at improving one specific SRL strategy at a time. The forethought phase covered sessions one to three. In session one, Zimmerman (2000) SRL model, its phases, and the topic “goal setting” were introduced to the students. The instructor provided theoretical input on goal definition, the SMART method (Doran, 1981), and goal orientation. After receiving the theoretical content, students completed digital worksheets referring to the presented theoretical input by working on their own or in groups. All worksheets were in PDF format and could be filled out digitally. For example, one of the exercises involved using the SMART method (Doran, 1981) to set goals. The second session covered the topic “time management” and provided such strategies as the ALPEN method (Seiwert, 2006) for organizing all the tasks of the day in five steps: Write down tasks, estimate their length, plan buffer time, make decisions, and follow-up on goal achievement. During this session, participants analyzed their daily behaviors and created a personalized weekly schedule based on their findings. The main topic of session three was “self-efficacy and self-motivation.” Participants learned about procrastination and self-efficacy. They acquired knowledge about different self-motivation strategies, such as creating the feeling of success or finding a personal benefit in doing required work. Participants utilized worksheets to rate their preferred self-motivation techniques. Additionally, they ran a personal self-experiment to observe the physical effects of self-efficacy on their own bodies.
The performance phase occupied sessions four and five. In session four, the topic was “stress and concentration.” Participants were taught about the SORK Model (Kanfer and Saslow, 1969), which explains how stress develops. Strategies to reduce stress were discussed, including progressive muscle relaxation and cognitive reframing. The session further covered activities to improve concentration. Worksheets guided participants in relaxation and concentration exercises, on their own or with a partner. During session five, which focused on learning strategies, the instructor presented the method of Loci and the technique of mind-mapping. Participants had the chance to practice these strategies through various exercises and worksheets provided during the session.
The reflection phase was the subject in session six, focusing on “self-reflection and causal attribution.” The theoretical content included information about causal attribution theory, along with the benefits and disadvantages of self-reflection. In this session, worksheets were used to explore participants’ own attributional patterns and reference norm orientation.
3.3.2 Asynchronous e-learning environment
The e-learning environment was implemented as a digital online course via the learning platform Moodle, with the experimenter enrolling participants. The course comprised six modules presented in blocks, and participants had a six-week period in which to complete it. It was possible to finish the course faster. The modules were structured in a fixed order, with access to the subsequent module only granted after completion of the exercises in the previous module. The content was similar to that of the synchronous online seminar condition, to enable comparability. All theoretical content appeared as digital text in different chapters. All worksheets were also provided in fillable PDF format and, upon completion, uploaded on Moodle to finish the exercise.
To summarize, both learning environments used the same learning material but differed in the presence of a course teacher (yes/no) and the communication style (seminar = synchronous, e-learning = asynchronous) for learning.
3.4 Measurement instruments
To comprise all relevant information for predicting student profiles, the self-reported use of SRL strategies was assessed. To examine the impact on the training gains, we also assessed participants’ declarative SRL knowledge.
The self-reported use of SRL strategies was measured with the SRL questionnaire by Dörrenbächer and Perels (2016) containing 56 items and covering all three phases (forethought, performance, reflection) of Zimmerman’s SRL model (2000). Thus, the instrument covered the complete cyclic SRL process. The questionnaire provided an overall score as well as subscale scores for the different components of SRL, examining cognitive, metacognitive, and motivational SRL strategies, produced using a four-point Likert scale (1 = “totally disagree,” 4 = “totally agree”). As Table 2 shows, a high level of reliability for the overall scale as well as the motivational and metacognitive component, and acceptable reliability for the cognitive component was achieved in the current sample.
To determine whether participants experienced an increase in declarative SRL knowledge, a knowledge test consisting of 10 open-ended questions, answerable with the course content, was used. For example, the questions covered Zimmerman’s SRL model structure: “Between which three phases does Zimmerman (2000) differentiate in his model of self-regulation?” or causal attribution theory: “Name dimensions which can apply to causal attribution.” A total of 28 points was possible, and a research assistant coded all answers, using a standard sample solution to determine the score for each answer.
3.5 Data analysis
To prepare the following calculations, the data set was checked for outliers, resulting in the exclusion of three persons from the analyses, due to repetitive and implausible answer patterns. Thus, the analyses used a final sample of n = 138 participants.
(1) To address the first research question, an LPA (Vermunt and Magdison, 2004) was conducted with MPlus 8.8 (Muthén and Muthén, 2017), to identify an ideal number of profiles based on the scores of the questionnaire’s SRL component subscales. The aim of the LPA was to classify pre-service teachers with similar cognitive, metacognitive, and motivational strategies into homogeneous groups that differ maximally from each other. Following the approach by Ferguson et al. (2020), various models, including different numbers of classes were compared regarding their model fit. The number of classes was examined exploratorily by comparing models until the model with k + 1 classes did not lead to a significant improvement of the model fit. The LPA was conducted with the Robust Maximum Likelihood Estimator and the Full Information Maximum Likelihood algorithm, to deal with missing data. To avoid local maxima, we used 100 solutions for stage optimization, 2,000 random starts, and 20 iterations. The fit of the competing models was determined by using adequate statistical fit indices, namely, the Bayesian Information Criterion (BIC), the Akaike Information Criterion (AIC), and the Lo–Mendell–Rubin Test (LMRT), together with entropy (E) as an indicator for classification accuracy (Marsh et al., 2009). Lower values of the BIC and AIC represent a better model fit. The LMRT compares the current model with a k-1 class model. A significant p-value indicates a better fit than the model with k-1 classes. Entropy should be above the threshold of 0.80 to ensure a satisfactory classification of persons into different groups (Asparouhov and Muthén, 2014). In addition, we considered the theoretical congruity and the number of assigned participants to each profile. Representative profiles can only be classified if more than 5% of the sample falls into them (Masyn, 2013). The classifications were transferred to IBM SPSS (version 28.0.1). Next, we validated the profiles by examining differences in SRL in the pretest, which were (as expected, due to the LPA) statistically significant.
(2) and (3): To investigate the second and third research questions, ANOVAs with post-hoc comparisons were used to analyze possible differences regarding the training gains for SRL strategy use and declarative SRL knowledge in the different learning environments.
4 Results
Descriptive results and correlations regarding all relevant variables appear in Table 3. Our findings indicate moderate-to-strong correlations among the SRL subscales but no correlation between SRL strategy use and declarative SRL knowledge.
4.1 Research question 1: pre-service teachers’ SRL profiles
The results of the LPA support a three-class solution. For the two-class solution, the entropy was above the threshold of 0.80, and it showed the highest AIC value, compared to the other models. The four-class solution showed a slight increase in the BIC and a decrease in the AIC, compared to the three-class solution. Both models had the same classification accuracy, but the LMRT p-value was significant for the three-class solution and not for the four-class solution. The five-class and six-class solutions showed slightly lower AIC values but higher BIC values than the three-class solution. The p-value was not significant for both models, indicating that the three-class solution showed the best fit to the data. The fit indices for all estimated models appear in Table 4.
Profile 1 included seven participants (5%), forming the smallest profile group of participants with low values on all SRL components. Therefore, we labeled the group “low SRL.” Profile 2 comprised 51 participants (37%), and we labeled it “high SRL” because this second group demonstrated high values on all three SRL components. Finally, most of the participants (58%) were assigned to Profile 3, showing moderate values in the motivational component and low values in the cognitive and metacognitive components. Profile 3 scored much higher on all components than Profile 1, so we labeled it “moderate SRL.” Table 5 displays the descriptive statistics for the three profiles regarding the examined SRL components. To validate the profiles, isolated univariate ANOVAs were conducted with each SRL component as a dependent variable and the profiles as an independent variable. As expected, due to the LPA, the findings indicated significant differences between all three profiles regarding all SRL components, with all Scheffé p-values <0.001. There were no significant differences between the profiles regarding declarative SRL knowledge before the intervention (F (2,135) = 2.26, p = 0.109, η2 = 0.03).
To facilitate the interpretation of the findings, we converted the means into z-scores. This standardization enables a comparison of relative divergences between the profiles, in which z-scores larger than 0.5 and smaller than-0.5 are interpreted as extreme values (Liu et al., 2014). A profile plot based on the z-scores appears in Figure 1.
4.2 Research question 2 and 3: differential training effects regarding self-reported SRL strategy use and declarative SRL knowledge
To explore whether there are differences in SRL profiles in relation to self-reported use of SRL strategies and SRL knowledge after the intervention and to examine whether the learning environment influences learning performance, we conducted two 2 (pretest-posttest)*2(e-learning-online seminar)*3 (profiles) ANOVAs. The profiles and training conditions (asynchronous e-learning, synchronous online seminar) were used as between-subjects factors, time (pretest/posttest) was used as a within-subjects factor, while the SRL strategy use and the SRL knowledge separately were the dependent variables. Table 6 shows the means and standard deviations.
For SRL strategy use, results indicate a significant interaction of time*profile, F (2,112) = 5.35, p = 0.006, η2 = 0.10, hinting at different training gains depending on the profile. Scheffé post-hoc comparisons revealed significant differences between all profiles (p < 0.001). There was no significant interaction of time*condition, F (1,112) = 0.02, p = 0.896, and no three-way interaction of time*condition*profile, F (2,112) = 0.13, p = 0.883. Figure 2 visualizes the change in self-reported SRL strategy use means after the intervention.
To further analyze the training gains of the different profiles, we conducted Wilcoxon-tests, with the profile as independent variable and the SRL strategy use as the dependent variable. The histograms of difference scores were visually inspected and showed a symmetrical distribution of values for all three profiles, confirming the requirements for the test. For the low SRL profile, z = 2.03, p = 0.043, and the moderate SRL profile, z = −3.09, p = 0.002, the SRL strategy use increased significantly. For the high SRL profile, z = −0.15, p = 0.882, there was no significant increase in strategy use after the intervention.
For the declarative SRL knowledge, results reveal a significant main effect of time (F (1,112) = 62.78, p < 0.001, η2 = 0.36). No significant time*profile interaction was found (F (2,112) = 0.14, p = 0.869, η2 = 0.00), indicating a similar training effect independent of the profile. All profiles increased their declarative SRL knowledge statistically significant after the intervention. Figure 3 visualizes the change in declarative SRL knowledge means. The results also indicate a significant interaction of time*condition, F (1,112) = 4.46, p = 0.037, η2 = 0.04, hinting at different training gains depending on the condition. The e-learning condition increased their declarative knowledge more than the seminar condition. There was no significant three-way interaction of time*condition*profile, F (2,112) = 0.15, p = 0.864, η2 = 0.00.
5 Discussion
The aim of the present study was to examine how the individual SRL characteristics of pre-service teachers influence the effectiveness of two digital learning environments—an asynchronous e-learning environment and a synchronous online seminar—by applying a person-oriented approach. Furthermore, we compared the two different communication styles (synchronous and asynchronous) regarding self-reported SRL strategy use and SRL knowledge.
5.1 Pre-service teachers’ SRL profiles
The first research question examined the number of different SRL profiles of pre-service teachers. The results indicate a three-class solution, revealing a low, a moderate, and a high SRL profile. This is in accordance with the findings by Ainscough et al. (2019), Chen et al. (2023), Esnaashari et al. (2023), and Muwonge et al. (2020), who also revealed a three-profile solution for SRL with low, moderate, and high specifications. In our sample, the low-profile group was the smallest group. Most persons were categorized into the moderate profile. For both the moderate-and the low-profile groups, the motivational component is particularly emphasized, but the profiles differ most regarding the cognitive and metacognitive components. This is in accordance with the findings by Veenman et al. (2006) and Hirt et al. (2021), revealing that persons with low SRL also use fewer cognitive strategies, and persons with high SRL use more cognitive and metacognitive strategies. It must be mentioned that our sample included significantly more female than male participants, reflecting the current gender distribution in teacher education (Katsarova, 2020). Although females and males only marginally differ in their SRL (Virtanen and Nevgi, 2010), this could have influenced the distribution of the profiles. Because of a small advantage of females regarding SRL strategies, the number of high and medium profiles could be slightly overestimated due to the smaller number of males in the sample. The findings strengthen the need for SRL training for pre-service teachers because more than 60% of the participants were categorized with a low or medium SRL profile, revealing room for improvement.
5.2 Differential training effects regarding self-reported SRL strategy use and declarative SRL knowledge
The second research question investigated whether an SRL training has a different effect on pre-service teachers with different SRL profiles. The results indicate a significant (self-reported) increase in SRL strategy use for the low and moderate profiles after the training, but not for the high SRL profile. Thus, the training seems to be especially effective for persons included in the low profile; they made the highest gains in SRL strategy use, leading to an approximation of the moderate profile in terms of a compensation effect. This is in line with Esnaashari et al. (2023) who conducted a 12-week blended learning course which led to an increase in SRL strategy use among university students with a low SRL profile. The students categorized with a low SRL profile had similar levels of SRL strategy use as those with average SRL profiles after the course. In contrast to the present study, Dörrenbächer and Perels (2016) found that moderate SRL profile students benefited more from an eight-week training intervention than low or high-profile students, demonstrating a compensation effect as well for students with a moderate SRL profile.
All profiles increased their declarative SRL knowledge significantly after the intervention, whereupon the low profile achieved scores far below the moderate-and high-profile groups. Despite a strong knowledge increase in all groups, persons in the low-profile group still scored less than half of the possible points in the declarative knowledge test (35%) whereas the moderate-and high-profile groups reached 50% correct answers. This result confirms the training’s effectiveness but also reveals that the participants could not use the whole training content to increase their declarative SRL knowledge. One reason for this could have been the training duration. The six weeks could have been too short for the dense amount of knowledge we presented to participants. This is in line with the meta-analysis by Chen (2022), who strengthens the assumption that students need appropriate time to master self-regulation skills, especially when the interventions are conducted in the field.
Another reason could be the fit of the questions and training content. Information provided in the training could have answered all questions; however, the sheer amount of the information could have prevented processing it deeply enough. According to the theory of multimedia learning by Mayer (2005), this could have led to a high cognitive load during learning. In future learning environments, important information should be highlighted visually.
The participants’ motivation to participate and their interest in the topic could also be a cause for the results. Students with motivated SRL profiles tend to have higher academic achievement and study intentions compared to students with non-motivated SRL profiles (Broadbent and Fuller-Tyszkiewicz, 2018). The study was carried out in different compulsory elective courses, which may have caused low motivation and interest in some participants, restricting maximum training gains.
Interestingly, the declarative SRL knowledge did not correlate with the self-reported SRL scales, suggesting a discrepancy between SRL knowledge and self-reported SRL strategy use (Dörrenbächer-Ulrich et al., 2021). In our sample, high-level SRL knowledge does not imply high-level use of SRL. It could be possible that persons with high-level SRL knowledge also use many SRL strategies but are unaware of using them and, therefore, cannot report it on a questionnaire.
5.3 Differences regarding asynchronous and synchronous learning environments
Our third research question referred to the differences between synchronous and asynchronous learning environments regarding SRL training. For SRL strategy use, there was no significant time*condition interaction, indicating no differences between asynchronous and synchronous learning environments regarding SRL strategy use after the training. The participants in both conditions increased their strategy use, indicating that both communication types are sufficient to support SRL strategy training and that in our sample, SRL strategy use does not depend on whether a course teacher is present.
Concerning declarative SRL knowledge, the results indicated a significant time*condition interaction. In our sample, participants in the asynchronous e-learning condition showed higher training gains than students in the seminar condition, independent of the participants’ respective profiles. One cause for this could be that the students had more time to reflect on the material in the asynchronous e-learning environment, which led to more interaction with the material and, hence, deeper processing of information than in the synchronous seminar condition (Lucas et al., 2014). Regarding SRL knowledge acquisition, the communication style seems to play a significant role. Given that SRL knowledge is a comprehensive and complex topic, the results suggest asynchronous environments as more adequate for teaching this topic. The automated feedback in the asynchronous e-learning environment seems sufficient to increase declarative SRL knowledge in our sample without actively involving a course teacher. This could be caused by the complexity of the learning content and the profile distribution. In our sample, the participants were able to cope with the information and exercises provided successfully, indicating a reasonable difficulty in the tasks. Because only seven persons were categorized in the low SRL profile, the SRL skills in the sample were high enough to learn without a course teacher. For future studies, it would be interesting to investigate whether a course teacher has a positive effect when applying a more complex task.
5.4 Limitations
Although the study expands previous knowledge on individual learner characteristics of pre-service teachers and their impact on SRL training using differing digital learning environments, there are several limitations that need to be addressed.
One limiting factor is the e-learning environment. Although the students had 6 weeks to complete the e-learning course, faster completion was possible. Some students may have finished the course early; some learners procrastinated and binge-learned the entire content within the last days of the available time. The assessment only registered course completion, not how much time the participants spent in the course to completion. Furthermore, a task was considered completed if the corresponding material was uploaded to Moodle. The assignments were checked visually for reasonable sentences and paragraphs, to prevent cheating on the completion, but the content was not checked for adequacy. This leaves the possibility that learners may have worked on the tasks superficially. Another limiting factor was methodological issues. The sample size is rather small for conducting an LPA. Spurk et al. (2020) recommended at least 500 participants for an accurate categorization in the LPA—difficult to realize—and called for further investigations with a larger sample. Moreover, the gender distribution in the sample causes a limitation of the study. There are significantly more female than male participants, because teaching is still a predominantly female working area with 72% female teachers in the EU (Katsarova, 2020). In future samples a balanced number of females and males would be desirable. Furthermore, the reliability of the cognitive subscale of SRL is questionable and could have led to unreliable measurement (van Griethuijsen et al., 2015). Moreover, the present findings are based on self-report data. It cannot be ruled out that the participants provided untrue answers due to social desirability. Another limitation is the small number of subjects in Profile 1 (low SRL), which may not be representative of the whole population of pre-service teachers, limiting the generalizability of the findings. Due to the small group size, an incidental finding of this profile cannot be ruled out Moreover, the current study relies on quasi-randomized data of participants from different courses at the same university with different course teachers. This nested data structure may have impacted the findings and could be analyzed using multi-level model analysis. The different course teachers worked with the same course material but without a calibration with each other which could have caused an instructor bias. It must be mentioned critically that the current approach only includes profiles that represent different levels of SRL rather than a continuous spectrum, neglecting other specifications of SRL.
5.5 Implications
The presented results illustrate implications for practical application and research. Pre-service teachers are crucial in modeling strategy use in the classroom for their future students (Peeters et al., 2014). In order to effectively teach students about using SRL strategies, pre-service teachers first need to develop their own SRL skills (Karlen et al., 2023). To achieve this, they could undergo adaptive SRL training during their studies. This training should be tailored to the individual needs of the learners based on their SRL profile and provide adaptive learning content. Based on our findings, asynchronous learning environments are suitable for declarative SRL knowledge improvement and for SRL strategy use, both, synchronous and asynchronous learning environments are adequate. Concerning this matter, either pure asynchronous learning environments or a combination of asynchronous and synchronous learning parts (Giesbers et al., 2014; Amiti, 2020) seem reasonable, to promote SRL strategy use and declarative SRL knowledge simultaneously. A combined use would ensure optimal exploitation of the advantages of both communication types. One way of implementing this is to impart declarative SRL knowledge to pre-service teachers as early as possible in their studies by means of an asynchronous e-learning training environment. Subsequently, the application of SRL strategies can be encouraged through, for example, a synchronous practical seminar that requires SRL strategy use in the classroom. This approach may also help to reduce the discrepancy between SRL knowledge and SRL strategy use. In the course of this approach, pre-service teachers should also be made aware that SRL can enhance students’ academic success and that they can facilitate their students’ future learning endeavors.
For future studies, we recommend a longer training period, to enable an increase of SRL strategy use or a reduction of training content. In addition, utilizing Moodle as a training platform enables the generation of log and process data. This can provide a more comprehensive understanding of SRL by offering multiple measurement points, which allows for the detection of profile changes throughout the intervention. Our study contributed to the examination of different SRL profiles of pre-service teachers and their impact on different digital learning environments. Because pre-service teachers are important multiplicators in the classroom but are often neglected as a target group, we call for more studies regarding pre-service teacher SRL training in different learning environments to develop ideal learning opportunities for future teachers.
6 Conclusion
The findings of the present study underline the importance of a person-centered approach regarding digital learning environments, to fulfill pre-service teachers’ individual needs. The results indicate that SRL training for pre-service teachers with different SRL profiles is effective in general, especially for declarative SRL knowledge, but needs further adaptation to support students with already high-level SRL skills.
Data availability statement
The raw data supporting the conclusions of this article will be made available by the authors, without undue reservation.
Ethics statement
Ethical approval was not required for the studies involving humans because the study was conducted following the Declaration of Helsinki, no institutional approval was necessary by local law. The studies were conducted in accordance with the local legislation and institutional requirements. The participants provided their written informed consent to participate in this study.
Author contributions
NB: Conceptualization, Data curation, Formal analysis, Methodology, Visualization, Writing – original draft, Writing – review & editing. MB: Supervision, Writing – original draft, Writing – review & editing. LD-U: Resources, Supervision, Writing – original draft, Writing – review & editing. FP: Funding acquisition, Project administration, Supervision, Writing – original draft, Writing – review & editing.
Funding
The author(s) declare that financial support was received for the research, authorship, and/or publication of this article. The project (SaLUt, 01JA1906A) is part of the “Qualitätsoffensive Lehrerbildung,” a joint initiative of the Federal Government and the Länder, which aims to improve the quality of teacher training. The program is funded by the Federal Ministry of Education and Research.
Conflict of interest
The authors declare that the research was conducted in the absence of any commercial or financial relationships that could be construed as a potential conflict of interest.
Publisher’s note
All claims expressed in this article are solely those of the authors and do not necessarily represent those of their affiliated organizations, or those of the publisher, the editors and the reviewers. Any product that may be evaluated in this article, or claim that may be made by its manufacturer, is not guaranteed or endorsed by the publisher.
References
Ainscough, L., Leung, R., Colthorpe, K., and Langfield, T. (2019). ‘Characterizing university students’ self-regulated learning behavior using dispositional learning analytics’, 5th international conference on higher education advances (HEAd’19).
Alhazbi, S., and Hasan, M. A. (2021). The role of self-regulation in remote emergency learning: comparing synchronous and asynchronous online learning. Sustain. For. 13:11070. doi: 10.3390/su131911070
Alkhasawnh, S., and Alqahtani, M. A. M. (2019). Fostering students’ self-regulated learning through using a learning management system to enhance academic outcomes at the University of Bisha. TEM J. 8, 662–669. doi: 10.18421/TEM82-47
Amiti, F. (2020). Synchronous and asynchronous e-learning. Eur. J. Open Educ. E Learn. Stud. 5, 60–70. doi: 10.46827/ejoe.v5i2.3313
Araka, E., Oboko, R., Maina, E., and Gitonga, R. (2022). Using educational data mining techniques to identify profiles in self-regulated learning: an empirical evaluation. Int. Rev. Res. Open Distrib. Learn. 23, 131–162. doi: 10.19173/irrodl.v22i4.5401
Arguel, A., Lockyer, L., Lipp, O. V., Lodge, J. M., and Kennedy, G. (2017). Inside out: detecting learners’ confusion to improve interactive digital learning environments. J. Educ. Comput. Res. 55, 526–551. doi: 10.1177/0735633116674732
Asparouhov, T., and Muthén, B. (2014). Auxiliary variables in mixture modeling: three-step approaches using M plus. Struct. Equ. Model. Multidiscip. J. 21, 329–341. doi: 10.1080/10705511.2014.915181
Bandura, A. (2006). “Guide for constructing self-efficacy scales” in Self-efficacy beliefs of adolescents. eds. F. Pajares and T. Urdan (Grennwich: Information Age Publishing), 307–337.
Barnard-Brak, L., Paton, V. O., and Lan, W. Y. (2010). Profiles in self-regulated learning in the online learning environment. Int. Rev. Res. Open Distrib. Learn. 11, 61–80. doi: 10.19173/irrodl.v11i1.769
Bellhäuser, H., Lösch, T., Winter, C., and Schmitz, B. (2016). Applying a web-based training to foster self-regulated learning—effects of an intervention for large numbers of participants. Internet High. Educ. 31, 87–100. doi: 10.1016/j.iheduc.2016.07.002
Bembenutty, H. (2011). Introduction: self-regulation of learning in postsecondary education. New Dir. Teach. Learn. 2011, 3–8. doi: 10.1002/tl.439
Boekaerts, M. (1999). Self-regulated learning: where we are today. Int. J. Educ. Res. 31, 445–457. doi: 10.1016/S0883-0355(99)00014-2
Broadbent, J., and Fuller-Tyszkiewicz, M. (2018). Profiles in self-regulated learning and their correlates for online and blended learning students. Educ. Technol. Res. Dev. 66, 1435–1455. doi: 10.1007/s11423-018-9595-9
Broadbent, J., Panadero, E., Lodge, J. M., and de Barba, P. (2020). “Technologies to enhance self-regulated learning in online and computer-mediated learning environments” in Handbook of research in educational communications and technology. eds. M. J. Bishop, E. Boling, J. Elen, and V. Svihla (Cham: Springer), 37–52.
Broadbent, J., and Poon, W. L. (2015). Self-regulated learning strategies & academic achievement in online higher education learning environments: a systematic review. Internet High. Educ. 27, 1–13. doi: 10.1016/j.iheduc.2015.04.007
Broadbent, J., Sharman, S., Panadero, E., and Fuller-Tyszkiewicz, M. (2021). How does self-regulated learning influence formative assessment and summative grade? Comparing online and blended learners. Internet High. Educ. 50:100805. doi: 10.1016/j.iheduc.2021.100805
Brun, L., Pansu, P., and Dompnier, B. (2021). The role of causal attributions in determining behavioral consequences: a meta-analysis from an intrapersonal attributional perspective in achievement contexts. Psychol. Bull. 147, 701–718. doi: 10.1037/bul0000331
Buzza, D., and Allinotte, T. (2013). Pre-service teachers’ self-regulated learning and their developing concepts of SRL. Brock Educ. J. 23, 58–76. doi: 10.26522/brocked.v23i1.353
Chang, C.-C., Liang, C., Chou, P. N., and Lin, G. Y. (2017). Is game-based learning better in flow experience and various types of cognitive load than non-game-based learning? Perspective from multimedia and media richness. Comput. Huma. Behav. 71, 218–227. doi: 10.1016/j.chb.2017.01.031
Chen, J. (2022). The effectiveness of self-regulated learning (SRL) interventions on L2 learning achievement, strategy employment and self-efficacy: a meta-analytic study. Front. Psychol. 13:1021101. doi: 10.3389/fpsyg.2022.1021101
Chen, J., Lin, C. H., Chen, G., and Fu, H. (2023). Individual differences in self-regulated learning profiles of Chinese EFL readers: a sequential explanatory mixed-methods study. Stud. Second. Lang. Acquis. 45, 955–978. doi: 10.1017/S0272263122000584
Cheng, Z., Zhang, Z., Xu, Q., Maeda, Y., and Gu, P. (2023). A meta-analysis addressing the relationship between self-regulated learning strategies and academic performance in online higher education. J. Comput. High. Educ. doi: 10.1007/s12528-023-09390-1
Cho, M.-H., and Shen, D. (2013). Self-regulation in online learning. Distance Educ. 34, 290–301. doi: 10.1080/01587919.2013.835770
Clark, R. C., and Mayer, R. E. (2016). “E-learning: promise and pitfalls” in E-learning and the science of instruction. eds. R. C. Clark and R. E. Mayer (San Francsico: Wiley), 7–28.
Clark, D. B., Tanner-Smith, E. E., and Killingsworth, S. S. (2016). Digital games, design, and learning: a systematic review and meta-analysis. Rev. Educ. Res. 86, 79–122. doi: 10.3102/0034654315582065
Cooke, N. J., Salas, E., Cannon-Bowers, J. A., and Stout, R. J. (2000). Measuring team knowledge. Hum. Fact. Ergon. Soc. 42, 151–173. doi: 10.1518/001872000779656561
De Bruin, A. B. H., and van Gog, T. (2012). Improving self-monitoring and self-regulation: from cognitive psychology to the classroom. Learn. Instr. 22, 245–252. doi: 10.1016/j.learninstruc.2012.01.003
De Bruin, A. B. H., and van Merriënboer, J. J. G. (2017). Bridging cognitive load and self-regulated learning research: a complementary approach to contemporary issues in educational research. Learn. Instr. 51, 1–9. doi: 10.1016/j.learninstruc.2017.06.001
Dent, A. L., and Koenka, A. C. (2016). The relation between self-regulated learning and academic achievement across childhood and adolescence: a meta-analysis. Educ. Psychol. Rev. 28, 425–474. doi: 10.1007/s10648-015-9320-8
Dignath, C., and Veenman, M. V. J. (2021). The role of direct strategy instruction and indirect activation of self-regulated learning—evidence from classroom observation studies. Educ. Psychol. Rev. 33, 489–533. doi: 10.1007/s10648-020-09534-0
Doran, G. T. (1981). There’s a SMART way to write management’s goals and objectives. Manag. Rev. 70, 35–36.
Dörrenbächer, L., and Perels, F. (2015). Volition completes the puzzle: development and evaluation of an integrative trait model of self-regulated learning. Front. Learn. Res. 3, 14–36. doi: 10.14786/flr.v3i4.179
Dörrenbächer, L., and Perels, F. (2016). Self-regulated learning profiles in college students: their relationship to achievement, personality, and the effectiveness of an intervention to foster self-regulated learning. Learn. Individ. Differ. 51, 229–241. doi: 10.1016/j.lindif.2016.09.015
Dörrenbächer-Ulrich, L., Rascopp, S., and Perels, F. (2019). ‘Evaluation einer Lernwerkstatt zum selbstregulierten Lernen für angehende Lehrkräfte – Förderung von Kompetenzen zur Gestaltung individualisierten Lernens [Evaluation of a learning workshop for self-regulated learning for prospective teachers - promoting skills for designing individualized learning]‘, Herausforderung Lehrer*innenbildung - Zeitschrift zur Konzeption. Gestaltung und Diskussion 2, 323–345. doi: 10.4119/HLZ-2454
Dörrenbächer-Ulrich, L., Weißenfels, M., Russer, L., and Perels, F. (2021). Multimethod assessment of self-regulated learning in college students: different methods for different components? Instr. Sci. 49, 137–163. doi: 10.1007/s11251-020-09533-2
Duncan, K., Kenworthy, A., and McNamara, R. (2012). The effect of synchronous and asynchronous participation on students’ performance in online accounting courses. Acc. Educ. 21, 431–449. doi: 10.1080/09639284.2012.673387
Engelmann, K., Bannert, M., and Melzner, N. (2021). Do self-created metacognitive prompts promote short-and long-term effects in computer-based learning environments? Res. Pract. Technol. Enhanc. Learn. 16, 1–21. doi: 10.1186/s41039-021-00148-w
Esnaashari, S., Gardner, L. A., Arthanari, T. S., and Rehm, M. (2023). Unfolding self-regulated learning profiles of students: a longitudinal study. J. Comput. Assist. Learn. 39, 1116–1131. doi: 10.1111/jcal.12830
Ferguson, S. L., Moore, G., and Hull, D. M. (2020). Finding latent groups in observed data: a primer on latent profile analysis in Mplus for applied researchers. Int. J. Behav. Dev. 44, 458–468. doi: 10.1177/0165025419881721
Foerst, N. M., Klug, J., Jöstl, G., Spiel, C., and Schober, B. (2017). Knowledge vs. action: discrepancies in university students’ knowledge about and self-reported use of self-regulated learning strategies. Front. Psychol. 8:1288. doi: 10.3389/fpsyg.2017.01288
Francescucci, A., and Rohani, L. (2019). Exclusively synchronous online (VIRI) learning: the impact on student performance and engagement outcomes. J. Mark. Educ. 41, 60–69. doi: 10.1177/0273475318818864
Fryer, L. K., and Vermunt, J. D. (2018). Regulating approaches to learning: testing learning strategy convergences across a year at university. Br. J. Educ. Psychol. 88, 21–41. doi: 10.1111/bjep.12169
Giesbers, B., Rienties, B., Tempelaar, D., and Gijselaers, W. (2014). A dynamic analysis of the interplay between asynchronous and synchronous communication in online learning: the impact of motivation. J. Comput. Assist. Learn. 30, 30–50. doi: 10.1111/jcal.12020
Glogger-Frey, I., Ampatziadis, Y., Ohst, A., and Renkl, A. (2018). Future teachers’ knowledge about learning strategies: Misconcepts and knowledge-in-pieces. Think. Skills Creat. 28, 41–55. doi: 10.1016/j.tsc.2018.02.001
Gordon, S. C., Dembo, M. H., and Hocevar, D. (2007). Do teachers' own learning behaviors influence their classroom goal orientation and control ideology? Teach. Teach. Educ. 23, 36–46. doi: 10.1016/j.tate.2004.08.002
Griffiths, M., and Graham, C. (2010). Using asynchronous video to achieve instructor immediacy and closeness in online classes: experiences from three cases. Int. J. E Learn. 9, 325–340.
Heikkilä, A., Lonka, K., Nieminen, J., and Niemivirta, M. (2012). Relations between teacher students’ approaches to learning, cognitive and attributional strategies, well-being, and study success. High. Educ. 64, 455–471. doi: 10.1007/s10734-012-9504-9
Hirt, C. N., Karlen, Y., Merki, K. M., and Suter, F. (2021). What makes high achievers different from low achievers? Self-regulated learners in the context of a high-stakes academic long-term task. Learn. Indiv. Differ. 92:102085. doi: 10.1016/j.lindif.2021.102085
Hrastinski, S. (2008). The potential of synchronous communication to enhance participation in online discussions: a case study of two e-learning courses. Inf. Manag. 45, 499–506. doi: 10.1016/j.im.2008.07.005
Hrastinski, S., Keller, C., and Carlsson, S. A. (2010). Design exemplars for synchronous e-learning: a design theory approach. Comput. Educ. 55, 652–662. doi: 10.1016/j.compedu.2010.02.025
Huang, L., Li, S., Poitras, E. G., and Lajoie, S. P. (2021). Latent profiles of self-regulated learning and their impacts on teachers’ technology integration. Br. J. Educ. Technol. 52, 695–713. doi: 10.1111/bjet.13050
Kanfer, F. H., and Saslow, G. (1969). “Behavioral diagnosis” in Behavior therapy: Appraisal and status. ed. D. Franks (New York: McGraw Hill), 417–444.
Karlen, Y., Hirt, C. N., Jud, J., Rosenthal, A., and Eberli, T. D. (2023). Teachers as learners and agents of self-regulated learning: the importance of different teachers competence aspects for promoting metacognition. Teach. Teach. Educ. 125:104055. doi: 10.1016/j.tate.2023.104055
Katsarova, I. (2020). ‘Teaching: a woman’s world’, European Parliament Research Service (EPRS). Available at: https://www.europarl.europa.eu/RegData/etudes/ATAG/2020/646191/EPRS_ATA (Accessed November 14, 2024).
Kim, D., Yoon, M., Jo, I. H., and Branch, R. M. (2018). Learning analytics to support self-regulated learning in asynchronous online courses: a case study at a women’s university in South Korea. Comput. Educ. 127, 233–251. doi: 10.1016/j.compedu.2018.08.023
Kizilcec, R. F., Pérez-Sanagustín, M., and Maldonado, J. J. (2017). Self-regulated learning strategies predict learner behavior and goal attainment in massive open online courses. Comput. Educ. 104, 18–33. doi: 10.1016/j.compedu.2016.10.001
Kramarski, B., and Michalsky, T. (2010). Preparing preservice teachers for self-regulated learning in the context of technological pedagogical content knowledge. Learn. Instr. 20, 434–447. doi: 10.1016/j.learninstruc.2009.05.003
Kümmel, E., Moskaliuk, J., Cress, U., and Kimmerle, J. (2020). Digital learning environments in higher education: a literature review of the role of individual vs. social settings for measuring learning outcomes. Educ. Sci. 10:78. doi: 10.3390/educsci10030078
Lawson, M. J., Vosniadou, S., van Deur, P., Wyra, M., and Jeffries, D. (2019). Teachers’ and students’ belief systems about the self-regulation of learning. Educ. Psychol. Rev. 31, 223–251. doi: 10.1007/s10648-018-9453-7
Lee, Y., and Choi, J. (2011). A review of online course dropout research: implications for practice and future research. Educ. Technol. Res. Dev. 59, 593–618. doi: 10.1007/s11423-010-9177-y
Lin, L., Gong, Y., and Xu, N. (2021). Online self-regulated learning profiles: a study of Chinese as a foreign language learners. Front. Psychol. 12:797786. doi: 10.3389/fpsyg.2021.797786
Liu, W. C., Wang, C. K. J., Kee, Y. H., Koh, C., Lim, B. S. C., and Chua, L. (2014). College students’ motivation and learning strategies profiles and academic achievement: a self-determination theory approach’. Educ. Psychol. 34, 338–353. doi: 10.1080/01443410.2013.785067
Lucas, M., Gunawardena, C., and Moreira, A. (2014). Assessing social construction of knowledge online: a critique of the interaction analysis model. Comput. Hum. Behav. 30, 574–582. doi: 10.1016/j.chb.2013.07.050
Marsh, H. W., Muthén, B., Asparouhov, T., Lüdtke, O., Robitzsch, A., Morin, A. J. S., et al. (2009). Exploratory structural equation modeling, integrating CFA and EFA: application to students’ evaluations of university teaching. Struct. Equ. Model. Multidiscip. J. 16, 439–476. doi: 10.1080/10705510903008220
Masyn, K. (2013). “Latent class analysis and finite mixture modeling” in The Oxford handbook of quantitative methods in psychology. ed. T. D. Little (New York: Oxford University Press), 551–611.
Mayer, R. E. (2005). “Cognitive theory of multimedia learning” in The Cambridge handbook of multimedia learning. ed. R. E. Mayer (New York: Cambridge University Press), 31–48.
Meijs, C., Neroni, J., Gijselaers, H. J. M., Leontjevas, R., Kirschner, P. A., and de Groot, R. H. M. (2019). Motivated strategies for learning questionnaire part B revisited: new subscales for an adult distance education setting. Internet High. Educ. 40, 1–11. doi: 10.1016/j.iheduc.2018.09.003
Muwonge, C. M., Ssenyonga, J., Kibedi, H., and Schiefele, U. (2020). Use of self-regulated learning strategies among teacher education students: a latent profile analysis. Soc. Sci. Hum. Open 2:100037. doi: 10.1016/j.ssaho.2020.100037
Nandagopal, K., and Ericsson, K. A. (2012). An expert performance approach to the study of individual differences in self-regulated learning activities in upper-level college students. Learn. Individ. Differ. 22, 597–609. doi: 10.1016/j.lindif.2011.11.018
Nieuwoudt, J. E. (2020). Investigating synchronous and asynchronous class attendance as predictors of academic success in online education. Australas. J. Educ. Technol. 36, 15–25. doi: 10.14742/ajet.5137
Ning, H. K., and Downing, K. (2015). A latent profile analysis of university students’ self-regulated learning strategies. Stud. High. Educ. 40, 1328–1346. doi: 10.1080/03075079.2014.880832
Ogbonna, C. G., Ibezim, N. E., and Obi, C. A. (2019). Synchronous versus asynchronous e-learning in teaching word processing: an experimental approach. S. Afr. J. Educ. 39, 1–15. doi: 10.15700/saje.v39n2a1383
Ohst, A., Glogger, I., Nückles, M., and Renkl, A. (2015). Helping preservice teachers with inaccurate and fragmentary prior knowledge to acquire conceptual understanding of psychological principles. Psychol. Learn. Teach. 14, 5–25. doi: 10.1177/1475725714564925
Oztok, M., Zingaro, D., Brett, C., and Hewitt, J. (2013). Exploring asynchronous and synchronous tool use in online courses. Comput. Educ. 60, 87–94. doi: 10.1016/j.compedu.2012.08.007
Peeters, J., de Backer, F., Reina, V. R., Kindekens, A., Buffel, T., and Lombaerts, K. (2014). The role of teachers’ self-regulatory capacities in the implementation of self-regulated learning practices. Proc. Soc. Behav. Sci. 116, 1963–1970. doi: 10.1016/j.sbspro.2014.01.504
Perry, N. B., Calkins, S. D., Dollar, J. M., Keane, S. P., and Shanahan, L. (2018). Self-regulation as a predictor of patterns of change in externalizing behaviors from infancy to adolescence. Dev. Psychopathol. 30, 497–510. doi: 10.1017/S0954579417000992
Reese, S. A. (2015). Online learning environments in higher education: Connectivism vs. dissociation. Educ. Inf. Technol. 20, 579–588. doi: 10.1007/s10639-013-9303-7
Rockinson-Szapkiw, A., and Wendt, J. (2015). Technologies that assist in online group work: a comparison of synchronous and asynchronous computer mediated communication technologies on students’ learning and community. J. Educ. Multimedia Hypermedia 24, 263–279.
Schwam, D., Greenberg, D., and Li, H. (2020). Individual differences in self-regulated learning of college students enrolled in online college courses. Am. J. Dist. Educ. 35, 133–151. doi: 10.1080/08923647.2020.1829255
Seiwert, L. (2006). Das 1x1 des Zeitmanagement: Zeiteinteilung, Selbstbestimmung, Lebensbalance [the 1x1 of time management: Time management, self-determination, life balance.]. Munich: Springer.
Shahabadi, M. M., and Uplane, M. (2015). Synchronous and asynchronous e-learning styles and academic performance of e-learners. Procedia Soc. Behav. Sci. 176, 129–138. doi: 10.1016/j.sbspro.2015.01.453
So, S. (2016). Mobile instant messaging support for teaching and learning in higher education. Internet High. Educ. 31, 32–42. doi: 10.1016/j.iheduc.2016.06.001
Spurk, D., Hirschi, A., Wang, M., Valero, D., and Kauffeld, S. (2020). Latent profile analysis: a review and “how to” guide of its application within vocational behavior research. J. Vocat. Behav. 120:103445. doi: 10.1016/j.jvb.2020.103445
Theobald, M. (2021). Self-regulated learning training programs enhance university students’ academic performance, self-regulated learning strategies, and motivation: a meta-analysis. Contemp. Educ. Psychol. 66:101976. doi: 10.1016/j.cedpsych.2021.101976
Valenzuela, R., Codina, N., Castillo, I., and Pestana, J. V. (2020). Young university students’ academic self-regulation profiles and their associated procrastination: autonomous functioning requires self-regulated operations. Front. Psychol. 11:354. doi: 10.3389/fpsyg.2020.00354
Van der Beek, S., Bellhäuser, H., Karlen, Y., and Hertel, S. (2020). New ways in fostering self-regulated learning at university: how effective are web-based courses when compared to regular attendance-based courses? Zeitschrift Für Pädagogische Psychol. 34, 117–129. doi: 10.1024/1010-0652/a000254
van Griethuijsen, R., Van Eijck, M. W., Van Eijck, R. M. W., Brok, D. P., Haste, H., Skinner, N. C., et al. (2015). Global patterns in students’ views of science and interest in science. Res. Sci. Educ. 45, 581–603. doi: 10.1007/s11165-014-9438-6
Veenman, M. V. J., Van Hout-Wolters, B. H. A. M., and Afflerbach, P. (2006). Metacognition and learning: conceptual and methodological considerations. Metacogn. Learn. 1, 3–14. doi: 10.1007/s11409-006-6893-0
Vermunt, J. K., and Magdison, J. (2004). “Latent class analysis” in The sage encyclopedia of social science research methods. eds. M. Lewis-Beck, A. Bryman, and T. F. Liao (New York: Sage), 549–553.
Virtanen, P., and Nevgi, A. (2010). Disciplinary and gender differences among higher education students in self-regulated learning strategies. Educ. Psychol. 30, 323–347. doi: 10.1080/01443411003606391
Vosniadou, S., Darmawan, I., Lawson, M. J., van Deur, P., Jeffries, D., and Wyra, M. (2021). Beliefs about the self-regulation of learning predict cognitive and metacognitive strategies and academic performance in pre-service teachers. Metacogn. Learn. 16, 523–554. doi: 10.1007/s11409-020-09258-0
Watts, L. (2016). Synchronous and asynchronous communication in distance learning. Q. Rev. Dist. Educ. 17, 23–32.
Zheng, L. (2016). The effectiveness of self-regulated learning scaffolds on academic performance in computer-based learning environments: a meta-analysis. Asia Pac. Educ. Rev. 17, 187–202. doi: 10.1007/s12564-016-9426-9
Zimmerman, B. J. (2000). “Attaining self-regulation: a social cognitive perspective” in Handbook of self-regulation. eds. M. Boekaerts, P. R. Pintrich, and M. Zeidner (San Diego: Academic Press), 749–768.
Zimmerman, B. J. (2008). Investigating self-regulation and motivation: historical background, methodological developments, and future prospects. Am. Educ. Res. J. 45, 166–183. doi: 10.3102/0002831207312909
Keywords: digital learning environment, self-regulated learning, teacher education, synchronous learning, asynchronous learning
Citation: Barz N, Benick M, Dörrenbächer-Ulrich L and Perels F (2024) Fostering self-regulated learning using synchronous or asynchronous digital learning environments: a latent profile analysis of pre-service teachers’ individual differences. Front. Educ. 9:1445182. doi: 10.3389/feduc.2024.1445182
Edited by:
Orna Heaysman, Achva Academic College, IsraelReviewed by:
Yinghui Shi, Central China Normal University, ChinaJoseline Santos, Bulacan State University, Philippines
Copyright © 2024 Barz, Benick, Dörrenbächer-Ulrich and Perels. This is an open-access article distributed under the terms of the Creative Commons Attribution License (CC BY). The use, distribution or reproduction in other forums is permitted, provided the original author(s) and the copyright owner(s) are credited and that the original publication in this journal is cited, in accordance with accepted academic practice. No use, distribution or reproduction is permitted which does not comply with these terms.
*Correspondence: Nathalie Barz, bmF0aGFsaWUuYmFyekB1bmktc2FhcmxhbmQuZGU=