- 1Chair of Chemistry Education, Faculty of Humanities, Social Sciences, and Theology, FAU Erlangen-Nuremberg, Nuremberg, Germany
- 2Chair of Inorganic Chemistry, Department of Chemistry and Chemical Biology, TU Dortmund University, Dortmund, Germany
- 3Chair of Chemistry Education, Department of Chemistry and Chemical Biology, TU Dortmund University, Dortmund, Germany
Introduction: During the COVID-19 pandemic, digital video conferencing formats temporarily became the new norm at universities. Due to social distancing, these environments were often the only way for students to work together. In the present study, we investigated how first-semester chemistry students dealt with new, challenging content, i.e., quantum theories of chemical bonding such as molecular orbital (MO) theory, in such an unfamiliar collaboration environment. Studies in the field of Computer-Supported Collaborative Learning (CSCL) suggest that small groups working on complex tasks are particularly effective when students actively build on the ideas and reasoning of their peers, i.e., when they engage in transactive talk and when they structure their work on a metacognitive level by following typical problem-solving patterns.
Methods: To operationalize these constructs, we developed a coding manual through quantitative content analysis, that we used to analyze a total of N = 77 students working together in 21 small groups on two consecutive tasks: the creation of glossaries and the construction of concept maps on MO theory. Our manual showed very good characteristics in terms of internal consistency and inter-coder reliability. Based on the data obtained, it was possible to not only describe the student’s transactive communication and problem-solving activities, but to correlate it with other variables such as knowledge development in MO theory, which allowed us to compare the two different collaborative phases as well as different treatment groups.
Results and discussion: Students showed a higher proportion of transactivity and problem-solving activities when constructing the concept maps than when creating glossaries. In terms of knowledge gains, a multiple linear regression analysis revealed that students in groups that derived consequences from their collaborative work showed greater improvements than students who did not, although the students’ prior knowledge remained the most influential factor. As for the different treatments, our data did not reveal any noticeable difference when students from a small group worked with either complementary or identical material before collaboration, neither in terms of transactive talk nor problem-solving patterns. All in all, we were able to develop and test a powerful tool to quantify transactive communication and problem-solving activities in a collaborative context.
1 Introduction
In general, digital-collaborative approaches have proven to be well suited for learning difficult scientific content (Kyndt et al., 2013). Such difficult content may, for example, include quantum mechanical theories of the chemical bond such as molecular orbital (MO) theory (Brundage et al., 2023). From a socio-constructivist point of view, the aim of collaborative learning scenarios is to engage learners in joint discourse and knowledge co-construction in order to achieve beneficial learning outcomes for each participating individual (Mercer and Howe, 2012; Roschelle and Teasley, 1995). In theory, activity within a pair or group can be considered as co-constructive when learners share and discuss the ideas which they have constructed individually before: Throughout this process, they may develop their understanding of the topics at hand, create new ideas, negotiate meanings of existing ones and integrate new information into already existing knowledge structures (Gätje and Jurkowski, 2021; Webb, 2009; Webb et al., 2014). A central characteristic of knowledge co-construction is that students may potentially construct knowledge they would not have been able to on their own (Deiglmayr and Spada, 2011).
1.1 Transactivity in collaborative learning scenarios
According to Gätje and Jurkowski (2021), one essential mechanism behind the effectiveness of collaboration is that it allows students to engage with each other’s understandings of the matter at hand, a process that Mercer and Howe (2012) call interthinking, i.e., a coordinated activity centered around establishing, maintaining and developing intersubjectivity. The latter refers to a form of cognitive agreement on the meaning of concepts within a group (Berger and Luckmann, 2011; Cooper-White, 2014; Mercer and Howe, 2012). Building on this thought, transactive communication, or dialogs in which learners build upon and develop previous contributions from group discussion, serves this purpose of co-constructing a joint solution to the task at hand from collaboration (Berkowitz and Gibbs, 1983; Vogel et al., 2023). Vogel et al. (2017) summarize multiple benefits of transactive communication in small groups: In accordance with Chi and Wylie’s (2014) ICAP framework, they argue that transactive communication has two benefits on a socio-cognitive level: First, contributions that add new ideas to the contributions of others may increase the amount of knowledge and perspectives in the group discussion. Furthermore, transactive activities can evoke socio-cognitive conflicts between learners. Resolving these conflicts can lead to a further increase in subject knowledge (Schwarz and Linchevski, 2007).
Gätje and Jurkowski (2021) also report multiple instances, in which transactivity was reported to positively relate to the results in group processes, e. g., in young adults’ discussions of moral dilemmas (Berkowitz and Gibbs, 1983), scientific problem-solving (Azmitia and Montgomery, 1993), or test performance after partner work in educational psychology (Jurkowski and Hänze, 2015). In a study conducted by Jurkowski and Hänze (2015), students who were trained in producing transactive statements outperformed their peers who did not receive such a training in a subsequent test on the topic. For that reason, the degree of transactivity within a collaborative problem-solving scenario might influence both the quality of the resulting product as well as the learning progress that individual learners make within this scenario (Gätje and Jurkowski, 2021; Vogel et al., 2023; Webb et al., 2021).
When investigating transactive talk, three distinct forms can be distinguished: self-references, low-transactive communication and high-transactive communication (Gätje and Jurkowski, 2021; Noroozi et al., 2013; Teasley, 1997; Vogel et al., 2023).
1.1.1 Self-references
Students engage in transactive self-talk when they provide a substantive justification or illustration for the ideas they put forward or the progress they make. Although several authors (e. g. Gätje and Jurkowski, 2021) argue that transactivity can only occur when students refer to the ideas that others make, we decided to follow along Berkowitz’ and Gibbs’ (1983) as well as Teasley’s (1997) broader definition of the term which includes self-referential statements into the transactive category. Bisra et al. (2018) were able to show that this form of communication might also be beneficial for the further development of learners’ conceptual understanding when working together in pairs or small groups.
1.1.2 Low-level transactivity
In low-level transactive speech acts, students ensure that they have correctly understood the thought process behind utterances from group members by paraphrasing statements or asking direct questions that aim at missing or more detailed explanations. Although, on the group level, no new knowledge is constructed in this way, these acts serve as a mechanism to integrate group knowledge into individual cognitive structures. Thus, they can be beneficial to individual learning (Gätje and Jurkowski, 2021; Zoethout et al., 2017).
1.1.3 High-level Transactivity
In contrast to low-level transactivity, students add new ideas when they execute high-level transactive speech acts and thus progress the co-construction of knowledge on the group level. Such acts include expanding on ideas, asking critical questions or pointing out mistakes, contrasting several multiple and joining together separate concepts from the group discourse (Gätje and Jurkowski, 2021; Vogel et al., 2023; Weinberger and Fischer, 2006).
1.2 Problem-solving processes in co-constructive activities
Aside from transactive argumentation, the students’ problem-solving skills also need to be considered when describing co-constructive processes (Engelmann et al., 2009; Priemer et al., 2020; Webb et al., 2021). According to Funke (2012), problem-solving requires learners to construct a mental representation of the problem, which they then try to solve by integrating their prior knowledge (Priemer et al., 2020). In this context, problems can be defined as situations in which learners need to overcome an obstacle (= problem) to achieve a goal state from a given state (Funke, 2012). This requires both subject-specific knowledge as well as metacognitive strategies (Priemer et al., 2020). According to Pólya (1957, p. 5), problem-solving in its simplest form consists of the following four steps:
1. Understanding the problem
2. Devising a plan
3. Carrying out the plan
4. Looking back
Priemer et al. (2020) document that similar steps can be found in other established problem-solving models. According to the OECD’s (2013, p. 126) widely accepted (Priemer et al., 2020) operationalization in the 2012 PISA study, problem-solving processes should start with exploring and understanding the problem. The goal here is for learners to build a mental representation of the information given to them in the problem context. In a collaborative learning situation, it is important for learners not only to understand the problem individually, but to achieve a common understanding of the problem situation at the group level (Vogel et al., 2023).
The second step in the PISA framework is the creation of a situation or problem model from the problem situation (OECD, 2013, p. 126). If necessary, the problem needs to be translated into a different representational format, e. g., by constructing tables, drawing graphics or converting the problem into a symbolic or verbal representation.
The third phase of a problem-solving process should include the planning and execution of a solution (OECD, 2013, p. 126). The planning phase involves defining or clarifying the goal, which is also reflected in our first two categories. Furthermore, students should divide the goal into sub-goals, devise a strategy to reach the goal state, and execute it.
In the fourth phase of problem-solving, learners should monitor their progress toward their (sub-)goal, detect possible obstacles and react accordingly. The final phase includes a critical reflection of the work process and product.
1.3 Research questions and hypotheses
Literature suggests that there should be a positive relation between the development of students’ learning success and the quality of their collaboration in small groups (Gätje and Jurkowski, 2021; Noroozi et al., 2013; Priemer et al., 2020; Vogel et al., 2023). However, these results should be critically examined for their applicability to our study, as many positive correlations were measured not in small groups, but in paired work (e. g., Jurkowski and Hänze, 2010; Jurkowski and Hänze, 2015). Furthermore, at the time of writing this paper, we were not aware of any studies investigating this in the context of the collaborative construction of glossaries and concept maps on quantum chemistry.
For that reason, we try to answer the following research questions throughout this paper:
1. How well do university students in each small group collaborate when they create glossaries and concept maps on molecular orbital theory together?
2. a. To what extent do students engage in transactive talk, i.e., refer to their own or each other’s ideas in their argumentation?
3. b. To what extent do students structure the work process on a metacognitive level, i.e., follow established problem-solving patterns?
4. How is the quality of the collaboration in small groups (as operationalized in question 1) related to the development of the individual students’ content knowledge, taking into account their prior knowledge before they start to collaborate?
Following along the literature discussed above, we hypothesize there to be a positive relation between the development of students’ learning success and the quality of the students’ collaboration in small groups.
Similar to the idea underlying the jigsaw method (cf. Aronson and Patnoe, 2011), we hypothesize that an increase in positive interdependence among students might also lead to an increase in transactivity and meta-communication, when the students realize that they need their peers to succeed in solving the problem presented to them, i.e., to create a glossary or a concept map (Johnson and Johnson, 1999).
One way to achieve this is by distributing the information needed to address the problem among various group members, so that no one person alone, but only the group as a whole, should be able to solve the problem. We will investigate whether this division of information positively impacts collaboration in the presented context in the following research question:
1. To what extent does collaboration differ between students who work with identical or complementary material before the group process in terms of
a. … transactive talk and problem-solving activities in general?
b. … the influence of transactive talk and problem-solving activities on the development of their concept knowledge?
2 Materials and methods
First, we present the intervention study in which we conducted our analyses (section 2.1). In the second subsection 2.2, we describe the development of a coding manual through quantitative content analysis according to Döring and Bortz (2016, pp. 555–559), before we finish with a principal component analysis (PCA) of our two scales in subsection 2.3.
2.1 Design of the Intervention
The research questions were addressed within the framework of a larger intervention study focused on molecular orbital theory, which we conducted in early 2022 in Germany (Hauck et al., 2023a). Before the intervention, students attended the introductory first-semester lecture (General and Inorganic Chemistry, held by the second author of this paper). The lecture followed an Atoms-First approach, meaning that students were confronted with quantum chemical content from the beginning of their studies, starting with basic quantum mechanical principles and finishing with molecular orbital (MO) theory (Chitiyo et al., 2018; Esterling and Bartels, 2013) around December. Over the Christmas break, the students could register to participate in our intervention, which started right after the holidays. Students could withdraw at any time or object to the processing of their data without negative consequences. Prior to participation, each of them filled out a declaration of informed consent, which contained important information on data protection, data security, and the further processing of data.
In January, the students took part in five seminar sessions (see Figure 1). Due to the circumstances caused by the COVID-19 pandemic, all seminar sessions had to be held online. Accordingly, the students did not work together face-to-face, but via video conference: Although the presentations were given digitally in the first half of the semester, the students had not yet collaborated with their peers in this lecture. Furthermore, there were only few, if any, opportunities for students to get to know their fellow students before the intervention (Werner et al., 2021). For this reason, we carried out two team-building activities in the first session of the study: To get to know each other, students entered a Zoom breakout session with other members of their small group and played a question game called “3 truths, 1 lie,” where they had to make three true and one false statement about themselves. The others had to guess which statement was the false one. Whenever the students got together in small groups, they were supervised by a moderator, e. g., a student assistant or a lecturer.
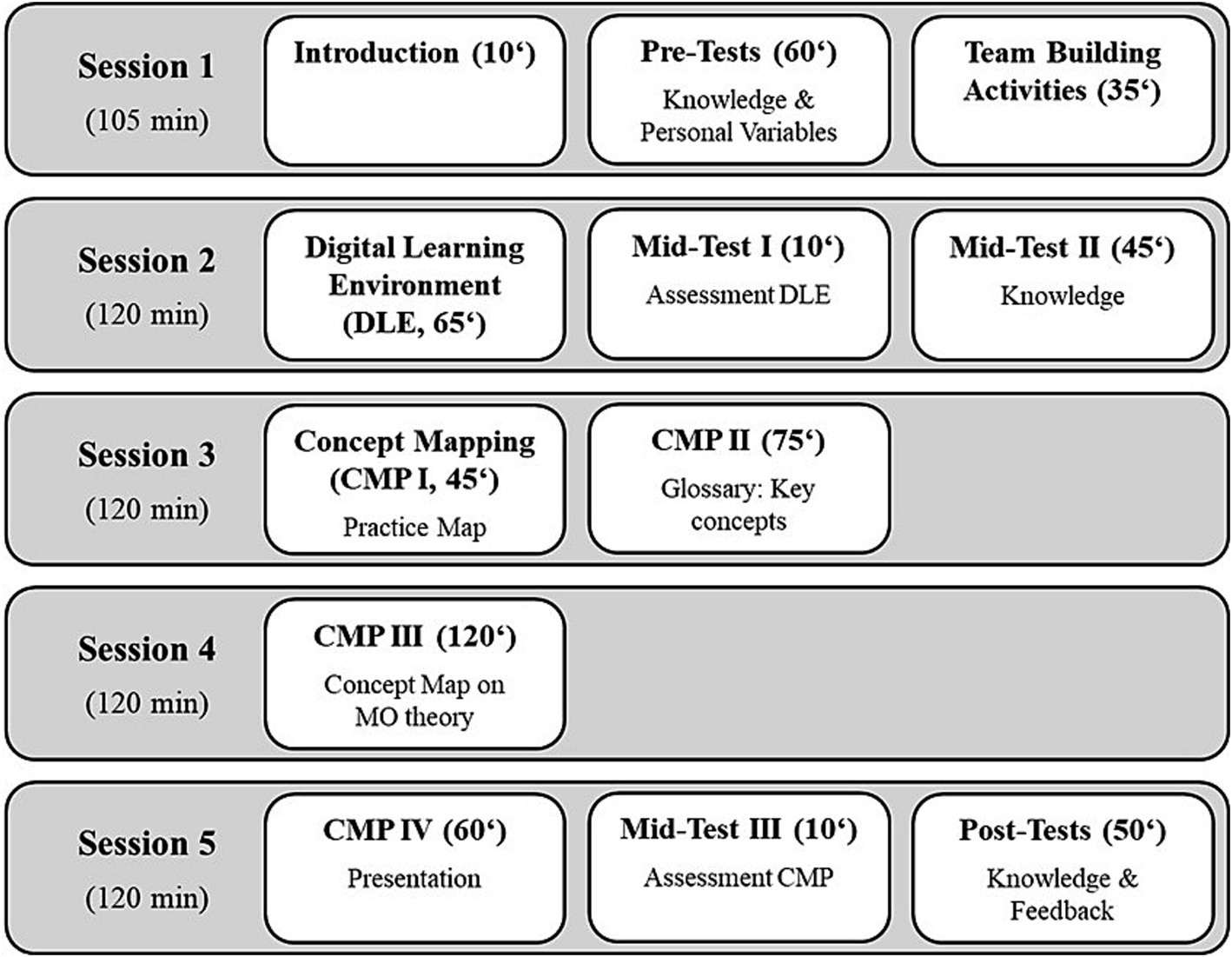
Figure 1. Structure of the five seminar sessions. In the first session, students receive an introduction, complete pre-tests, and participate in team-building activities. In the second session, they work with a digital learning environment (DLE), assess it in Mid-Test I, and answer subject knowledge questions for the second time in mid-test II. Sessions 3 to 5 are dedicated to the concept mapping process (CMP). In session 3, students first create a practice map, then select and explain key concepts of molecular orbital theory in a glossary. In session 4, they transfer, structure, and link these concepts into a concept map, which they present in session 5. Afterwards, students assess the CMP, complete a final subject knowledge questionnaire in the post-test, and provide feedback on the entire intervention.
In this particular phase, the moderator led the students through the question game, so that all of them were equally included. Later on, the moderator’s tasks were to record phases in which the students worked in small group, to help them with technical problems, and to answer questions regarding the organization of the intervention. At the end of this ice breaking activity, the students had to decide for a team name and return to the meeting from their breakout session. In the second step, the students participated in a quiz competition against other small groups: The students took turns to answer chemistry-related questions with the help of their group members.
After the students visited the regular introductory chemistry lecture, they participated in the intervention: First, they worked with interactive learning videos by themselves and then created glossaries and concept maps on MO theory to apply and structure their knowledge. During that second phase, N = 77 students were separated into small groups of 3–5 students (see Figure 1 for a detailed overview of the intervention structure) which form the sample for the research presented in this paper.
In the first session (“pre”), we assessed the students’ prior knowledge of general quantum chemistry and molecular orbital theory with a self-developed questionnaire consisting of 29 closed-ended (single-choice) and eight open-ended questions (Hauck et al., 2023b).
As learners need to have at least some amount of prior knowledge in order to effectively collaborate on complex tasks (Zambrano et al., 2019), the co-constructive phase was preceded by an individual constructive phase (Olsen et al., 2019). For that reason, the students worked on a Digital Learning Environment (DLE) by themselves in session 2. The DLE consisted of interactive learning videos which covered fundamental quantum chemical principles of MO theory (focus A), as well as the practical application of the theory (focus B), i.e., the creation and interpretation of so-called MO diagrams. The learning videos were developed based on the principles of the Cognitive Theory of Multimedia Learning (Mayer, 2014) and enriched with interactive elements to engage learners more actively and provide immediate feedback, which has shown positive effects on learning outcomes in numerous studies (Hattie, 2023). For a detailed description of the videos and their development, see Hauck et al. (2023a).
Afterwards, their knowledge on the topic was tested once again through the same questionnaire (“mid”). In the following three sessions, the students created glossaries and concept maps on MO theory to apply their knowledge and link existing concepts. Finally, they were tested a final time (“post”).
As summarized in Table 1, our sample consists of 41 female, 35 male, and one non-binary student (N = 77). The overall majority of them were first-semester students which graduated at a German upper secondary school. About half of them were from a Chemical Biology program, 20 studied Chemistry, and 14 aspired to become chemistry teachers for upper secondary school. The remaining students studied chemistry as a subsidiary subject, e. g. for a degree in mathematics (B. Sc.).
In our investigation, we compared students who worked in two different treatment groups (TGs):
1. About half of the students (n = 39) worked together in 10 small groups of three to five students each to create glossaries and concept maps. Every one of them watched identical interactive videos beforehand.
2. The other half (n = 38) also created glossaries and concept maps in 11 small groups of three to five students each. However, in the preceding phase, these students viewed two complementary subsets of videos that corresponded to the two different foci of the learning environment (A, quantum chemical basics vs. B, application of MO theory). When combined, video variants A and B contained the same content as the videos that treatment group TG1 worked with. On their own, students who watched videos corresponding to focus A were lacking the information on the application of MO theory, which only their other group members who worked with video variant B had, and vice versa for the quantum chemical basics.
Within this paper, the separation of the students into these intervention groups allows us to address research question RQ3 by comparing TG1 (identical videos before the start of collaboration) and TG2 (complementary videos).
We have previously published an in-depth investigation of the students’ conceptual knowledge throughout the intervention (Hauck et al., 2023b). There, we could show that the learning videos have been very effective in influencing their knowledge of MO theory. However, creating concept maps did not lead to a significant change in conceptual knowledge when comparing mean knowledge test scores for the entire sample. Furthermore, there seemed to be no difference between the treatment groups on a macroscopic level. However, should there be a difference between the groups concerning research question RQ3a, then this would suggest that the development of each student’s subject knowledge should be investigated in more detail, which would be a further discussion point of this paper.
In the collaborative work phases, students returned to the same small groups from session 1. Once again, we created Zoom breakout sessions and assigned moderators to each of them. In contrast to the team building activity, the moderators took a more passive role: To guarantee standardized conditions, they did not answer any questions regarding the method of concept mapping or the content. For that reason, we showed a pre-recorded presentation to the students at the beginning of each seminar session. These presentations contained information on the organization of the session, methodological and technical tutorials to create glossaries in Moodle (phase “CMP II” in Figure 1) or Concept Maps via CmapTools (phase “CMP III” in Figure 1), as well as an explanation of the tasks the students had to work on during the session. After the introduction, the students had the opportunity to ask questions in plenary, before working in their breakout sessions. In each work phase, the moderator posted the task in the Zoom chat, before the students chose a group member to share their screen with and began working.
For our subsequent analyses, the moderators recorded the audio and video of the creation of the glossary (phase “CMP II” in Figure 1, 75 min) and the construction of the concept map (phase “CMP III” in Figure 1, 120 min), using the capture function in Zoom. The final data set contains 42 videos, 21 from each phase. Each of these videos will be investigated separately in its entirety, so that in the end, we will obtain 42 ratings for each of our items, i.e., two ratings per group.
2.2 Development of the coding manual through quantitative content analysis
Since the goal of this article is to relate both transactivity and problem-solving behavior to students’ subject knowledge, a quantified variable, we have chosen to investigate these constructs using quantitative content analysis (Coe and Scacco, 2017; Döring et al., 2016; Riffe et al., 2019, pp. 148–167) of the screen and audio recordings of the collaborative phases from the intervention study presented in section 2.1. We chose this method because it allows us to systematically categorize and score our qualitative material through a coding process. These quantified data can then be further processed using quantitative statistical analysis methods and integrated with other variables such as the students’ subject knowledge (Coe and Scacco, 2017; Döring et al., 2016).
In accordance with Coe and Scacco (2017), this process can generate either low-or high-inference data. The latter are produced when the objective is to make non-observable deep structures accessible for quantitative analysis, e. g. in the case of analyzing transactivity in a collaborative learning situation, as we have done. In our analysis, we followed the 12 steps of this method according to Döring and Bortz (2016, pp. 555–559).
The first four steps, the development of research questions and hypotheses; the design of the study; the sampling; and the preparation of the data for analysis have already been covered in section 2.1 as well as within this subsection.
2.2.1 Generation of categories from theory
The fifth step is to deductively design the coding manual, which we originally created in German. Following research question RQ1, we decided to develop two distinct scales: The first set of categories consists of the three transactivity categories described in section 1.1: self-references (category 1.1), low-level transactivity (1.2) and high-level transactivity (1.3). The second set contains seven categories to analyze the students’ problem-solving behavior in small groups as described in section 1.2.
We want to distinguish between groups that plan carefully in advance and those that start working without a plan and only realize during the process that some of them might have a different understanding of the task than other group members. The latter would still be better than not establishing a common understanding at all. However, this runs the risk of students working independently instead of together. For this reason, we derive the following two categories from the first problem-solving step, the exploration and understanding of the problem, i.e., the creation of a glossary or a concept map:
Students establish a common understanding of the task…
1. Before they start working on it,
2. While they are working on it.
The second step, the creation of a situation or problem mode, corresponds to the tasks the students were given (“create a glossary from the information in the interactive learning videos”; “transfer your glossary into a concept map”). Therefore, we did not include a category for this step in our coding manual.
Given that the creation of concept maps is quite difficult due to it requiring both profound conceptual as well as metacognitive knowledge (Haugwitz et al., 2010; Nesbit and Adesope, 2006), we scaffolded the third problem-solving step, i.e., the planning and execution of a solution, by including sub-goals. First, students should transfer all terms from the glossary to the concept map. Second, they were tasked to look for a way to arrange the concepts on the map and third, link them with arrows. To represent this third phase, we derived the following category:
1. Students pre-structure their work process or discuss their strategic approach before they start working on the task. On the highest level, students discuss how to proceed before beginning with a new work phase.
Category 4 operationalizes the fourth problem-solving activity, monitoring of the work process:
1. Students structure their work process or discuss their strategic approach while they are working on the task. On the highest level, students actively monitor their work process and, if necessary, react accordingly.
Finally, we included three items to investigate whether the students followed the fifth step of problem-solving, a critical reflection of their work process and product. We separated these two reflections in categories 5 and 6. In category 7, we examine the extent to which students not only reflect on their work but also draw conclusions for their future actions.
1. Students reflect their work process or their strategic approach after they have finished working on the task. On the highest level, this reflection is done in an extensive and self-critical way.
2. Students reflect on the product they created, i.e., their glossary or concept map while they are working on the task or after they have finished working on it. The criteria from the preceding item also apply for the highest level in this one.
3. Students derive consequences for future learning processes from their reflections or discussions. On the highest level, this reflection is not limited to the contents of MO theory or to the creation of glossaries and concept maps.
For each item, we derived a fundamental idea of what it should measure as well as possible positive and negative indicators from the literature presented in this article. Each item is rated on a 3-point ordinal scale from 1 (students do not exhibit the described behavior) to 3 (students fully exhibit the described behavior).
2.2.2 Inductive revision and pre-testing
During the development process, the manual was revised and tested several times, corresponding to steps 6 and 7 of the quantitative content analysis. A revision cycle always proceeded as follows: The manual was applied to 2–4 videos by several raters. These videos were recorded in a similar intervention we conducted in early 2021, in which students who had attended the same lecture a year earlier also created concept maps in small groups (cf. Hauck et al., 2021). Afterwards, the raters came together, revised ambiguous wordings, and replaced any indicators from the literature with actual student statements that fitted the item, thus creating anchor examples.
2.2.3 Rater training; analysis of reliability and finalization of the coding manual; coding of the entire sample
In the 8th and 9th step of the analysis process, we double coded a random selection of eight videos (four videos which showed the creation of a glossary and four that depicted the construction of a concept map), corresponding to 19% of our total sample to check the reliability of the manual. Using IBM’s SPSS software (version 29), we calculated Cronbach’s Alpha to assess internal consistency between the transactivity and problem-solving items. Analysis of the double coding resulted in an excellent correlation between the two coders (ICCunjust = 0.952, cf. Koo and Li, 2016). In terms of internal consistency, we obtained a good alpha-value for the transactivity scale (items 1.1 to 1.3 for both phases, α = 0.736) and an acceptable one for the problem-solving scale (items 2.1 to 2.7 for both phases, α = 0.664) according to Cortina (1993). Due to these satisfactory results, no more changes were made to the manual. Nonetheless, we discussed and eliminated the differences in the ratings of these eight videos so that they could be included in the subsequent overall sample. This was necessary to obtain a sufficiently large sample for the subsequent analyses.
Tenthly, we analyzed all remaining 34 videos, and we conducted statistical analysis using SPSS. The final two steps, i.e., the statistical analysis and the interpretation of data, will be described in the results section of this article.
An English translation of the German manual can be found in the Supplementary material of this article, including a comprehensive description of our categories as well as explanations on how to score them.
2.3 Principal component analysis of the two scales
Before we analyzed our data against the background questions of the research questions, we conducted a principal component analysis to extract common factors and to look for possible dimensional reductions. Our PCA reveals that all three transactivity items load on a singular factor (see Table 2 for the corresponding correlation matrix), so that no rotation or normalization is needed, and we can assume there to be a common underlying latent construct (Osborne, 2015).
Regarding the problem-solving items, two different factors can be uncovered. The varimax rotated component matrix with Kaiser normalization (Kaiser, 1958, see Table 3) illustrates that the items 2.5, 2.6, and 2.7 heavily load on the first factor; items 2.1 and 2.3 load on the second one; and 2.2 and 2.4 correlate with both. This result is interesting insofar as these three sets of items correspond to the three different phases investigated: Items 2.1 and 2.3 refer to students’ activities before beginning to create their glossaries or concept maps; items 2.5 to 2.7 relate to the students’ reflections after finishing; items 2.2 and 2.4, which load on both factors, pertain to strategies while working. Although a reduction to two problem-solving factors would be possible in principle, we refrain from doing so in view of the ambiguous results for these two items, so that we retain seven separate problem-solving factors. Another reason for our decision is the potential loss of information if we reduce all seven items to two factors.
3 Results
In this section we will answer the research questions underlying this paper. Subsection 3.1 contains the descriptive results for our transactivity-and problem-solving items to subsequently address research question RQ1. The necessary data basis for RQ2 is formed by a multiple linear regression analysis in section 3.2. Finally, the chapter closes with a quantitative comparison of transactive talk and problem-solving patterns between students in treatment groups TG1 and TG2 in order to answer research questions RQ3a and RQ3b.
3.1 Descriptive statistics
The data presented here correspond to research question RQ1: Through a descriptive analysis of our ratings on the transactivity (section 3.1.1) and problem-solving (section 3.1.2) scales, we operationalize the quality of the students’ collaboration while they worked in small groups to create glossaries (CMP II phase in Figure 1) and concept maps (CMP III phase in Figure 1).
3.1.1 Transactivity scale
Table 4 illustrates the transactivity ratings for the phase in which the students created glossaries.

Table 4. Descriptive statistics for the transactivity scale (3-point ordinal scale), glossary creation (CMP II phase).
Table 5 refers to the creation of concept maps in the CMP III phase.

Table 5. Descriptive statistics for the transactivity scale (3-point ordinal scale), Concept Map creation (CMP III phase).
In both work phases, the student’s level of transactive communication was assessed as high, which can be deduced from the high mean scores: Not a single one is below 2.5 out of a maximum of 3 points and in no category in any of the two phases did fewer than two thirds of all groups receive the highest score. Furthermore, the amount of low-level transactive communication (item 1.2) was rated as low for only one group in each of the two phases. No group showed a low amount of self-referential (item 1.1) or high-level transactive (item 1.3) speech acts in either phase. For the creation of the concept map, all but one group’s high-level transactive communication was rated at the highest level.
3.1.2 Problem-solving scale
Table 6 contains the ratings for the 7 problem-solving items for the phase in which students created glossaries in small groups.
Table 7 shows the respective ratings for the concept-mapping phase, CMP III.
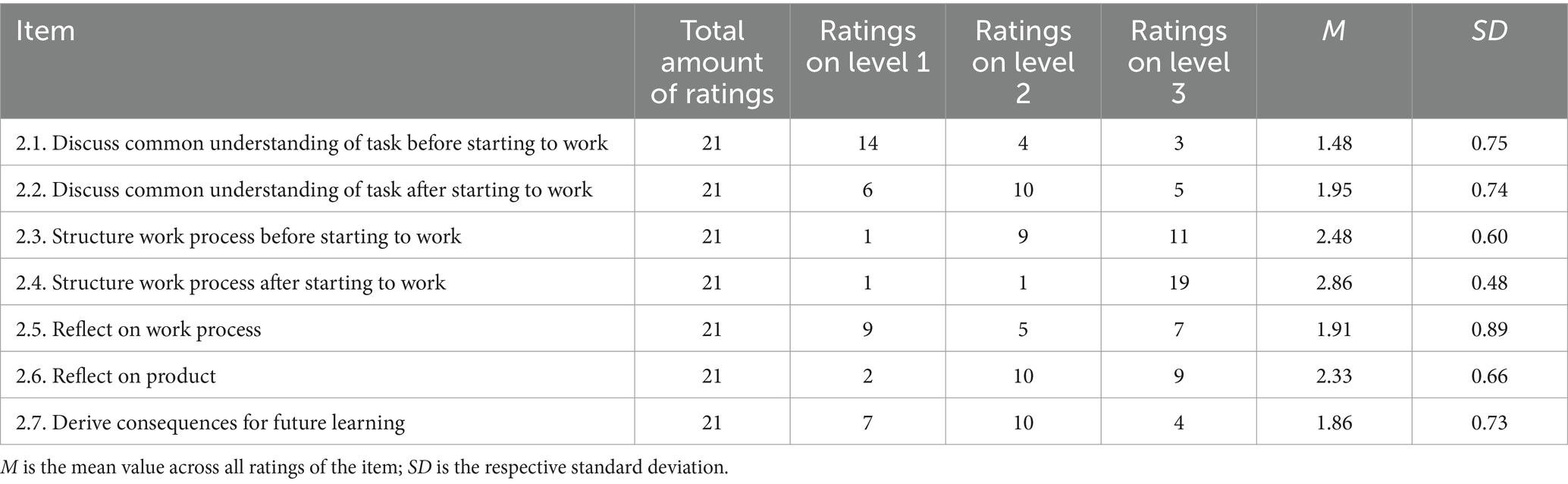
Table 7. Descriptive statistics for the problem-solving scale, concept map creation (CMP III phase).
Compared to the results on the transactivity scale, the ratings on the problem-solving scale were more heterogeneous.
While working together to create a glossary, more than half of the students frequently discussed the strategy they wanted to approach the work process with before they started (item 2.3) or during the work process (item 2.4); only one group did not do so at all. Beyond the strategic approach, scores are much lower:
About one quarter of the groups took the time to establish a common understanding of the task before they started working on it (item 2.1); one quarter only partly did so; the remaining half of all groups immediately began their work. If we take a look at the same activity during the work process, the mean values are even lower with only one group frequently negotiating their understanding of the task while working on it (item 2.2). The same could be observed for the reflection of the work process which only 3 student groups did in an extensive and self-critical way. Similar effects were shown for the in-depth reflection of the glossaries (item 2.6) and the discussion of consequences for future learning processes beyond the scope of MO theory and the creation of glossaries itself (item 2.7), which only one group did.
Apart from item 2.1 (establishing a common understanding of the task before beginning to work on it), all item’s mean values increase by about half a point on the respective scale when creating the concept maps, which means that students show a larger frequency of problem-solving strategies compared to the creation of the glossaries beforehand. Once again, the highest mean ratings were given for items 2.3 and 2.4, covering the discussion of the strategic approach to the task before and during the work process.
3.2 Regression analysis
With the analyses presented in this subsection, we aim at answering research question RQ2: Following up on the results from sections 3.1 and 3.2, we investigate whether the quality of collaboration within their small groups influences the individual students’ knowledge development throughout the creation of glossaries and concept maps.
For that reason, we conducted a multiple linear regression (MLR) analysis: Independent variables consisted of the students’ content knowledge prior to collaboration (“mid”-knowledge, MK, cf. Figure 1), the averaged transactivity scores (TS) across all three items and both collaborative work phases as well as all seven respective problem-solving scores, which we also averaged across both phases to reduce the complexity of our model against the background of the rather small sample size of only 21 groups. As the dependent variable, we chose the students’ content knowledge at the end of the intervention (“post”-knowledge, PK).
After calculating the MLR, we checked our data set for possible outliers. All studentized residuals were within the acceptable range from –3 to 3 (Pope, 1976). None of the leverages exceeded the limit calculated with the formula given by Igo (2010). All Cook distances are smaller than 1 (Cook, 1979). Linear independency of residuals was indicated by a Durbin-Watson value of 2.086 (Durbin and Watson, 1951). Furthermore, our studentized residuals were normally distributed according to a non-significant Shapiro-Wilks test (p = 0.660). Using the variance inflation factor (VIF) criterion according to Kim (2019), none of our items exceeded a value of 10, so that we assume there to be no significant multicollinearity between factors which could limit the power of the regression analysis.
The R2 for the overall model was 0.722 (adjusted R2 = 0.685), indicating a high goodness-of-fit (Cohen, 1988). Furthermore, an analysis of variance [ANOVA, F(9) = 19.329, p < 0.001] shows that the independent variables we selected are able to statistically significantly predict the students’ learning outcomes at the end of the intervention.
Table 8 shows an overview of the regression coefficients, showing that only the students ‘mid’-knowledge and the scores for item 2.7 (groups discuss consequences for future learning) remain as significant influences in the model, whereas the ‘mid’-knowledge is by far the stronger predictor of the two. Neither the transactivity score nor other problem-solving categories significantly predict the student’s post-test results.
3.3 Comparison of treatment groups
In the third and final subchapter, we address the third research question RQ3a: By comparing two different treatment groups TG1 (which we designed in a way that all students work with identical learning videos in the preceding DLE phase) and TG2 (in which different students from the same small group watched different videos beforehand), we examine whether there are differences in transactive talk or problem-solving patterns whether students enter collaboration after watching identical (TG1) or complementary (TG2) videos before beginning to collaborate by calculating unpaired Welch-tests (cf. Rasch et al., 2011; Ruxton, 2006) for each variable for the creation of glossaries (Table 9), concept maps (Table 10), as well as for the average values between the two (Table 11). Although there are marginal differences between some variables on the descriptive level, our data indicates that none of them are statistically significant. Since there are no differences between the groups in any category that could explain possible differences in the influence of transactive communication or problem-solving activities on the development of expertise, research question RQ3b cannot be answered satisfactorily on the basis of the present data set.
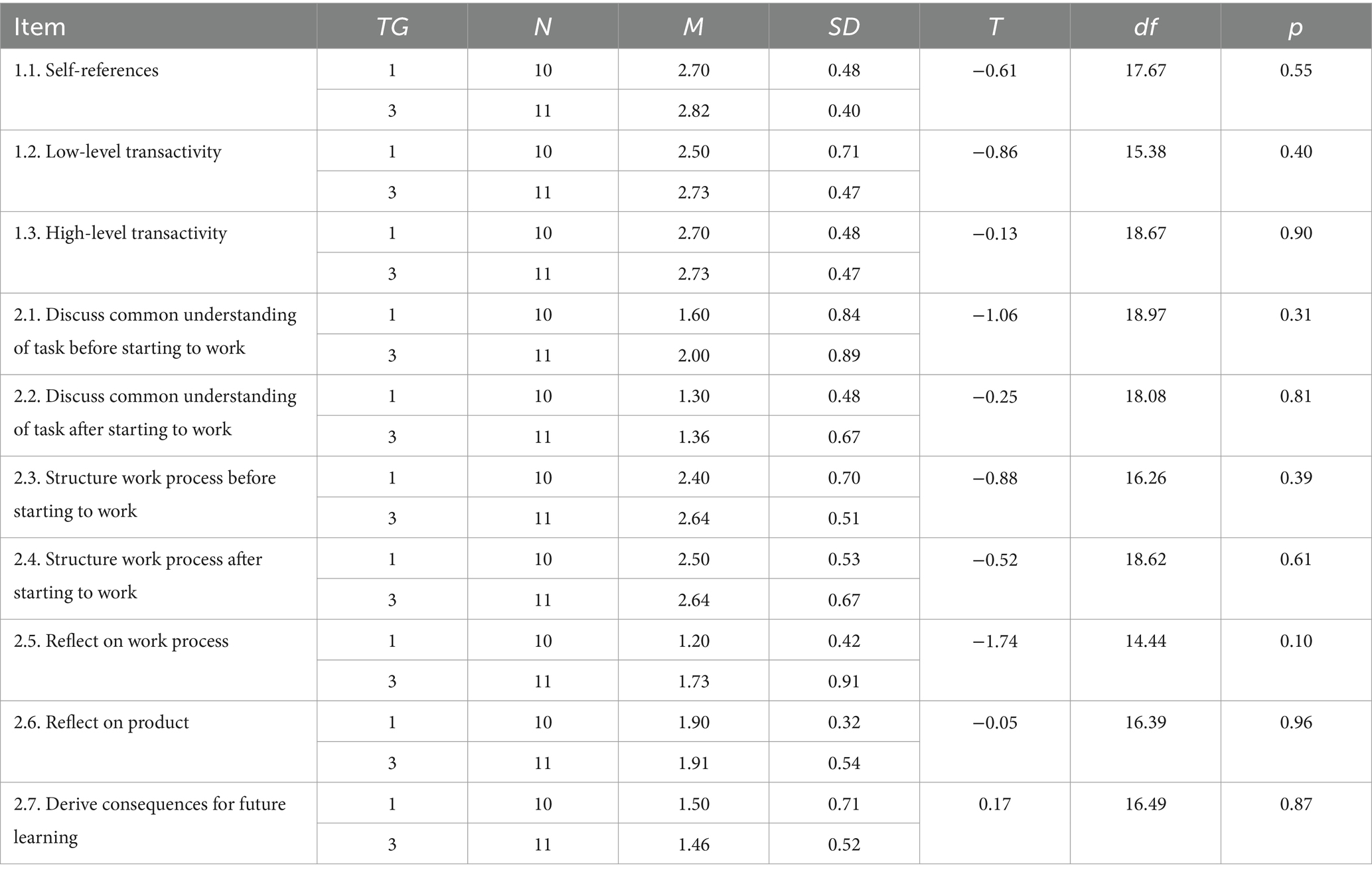
Table 9. Comparison of treatment groups TG1 and TG2 when creating glossaries (CMP II phase), unpaired Welch-tests.
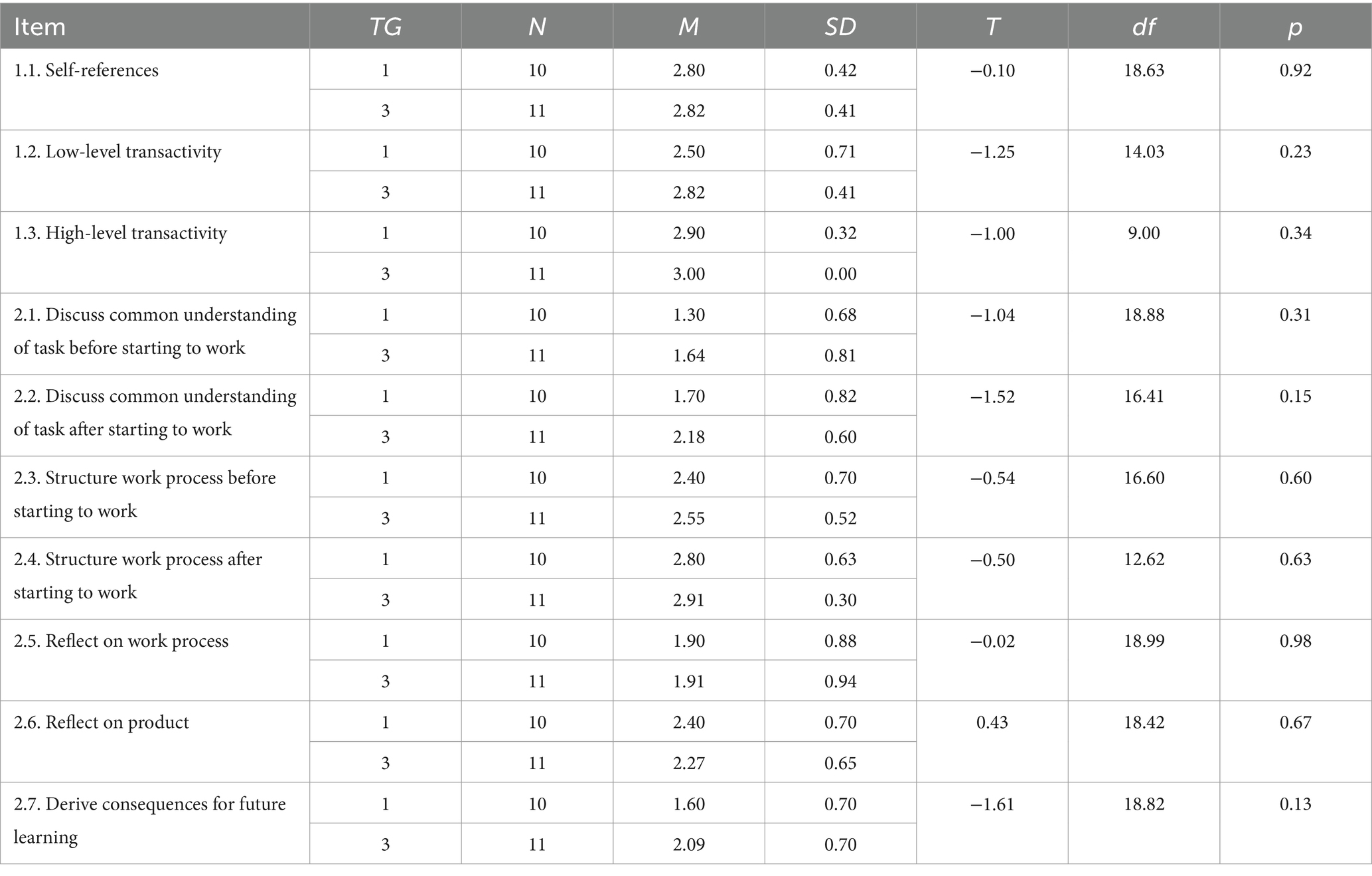
Table 10. Comparison of treatment groups TG1 and TG2 when creating concept maps (CMP III phase), unpaired Welch-tests.
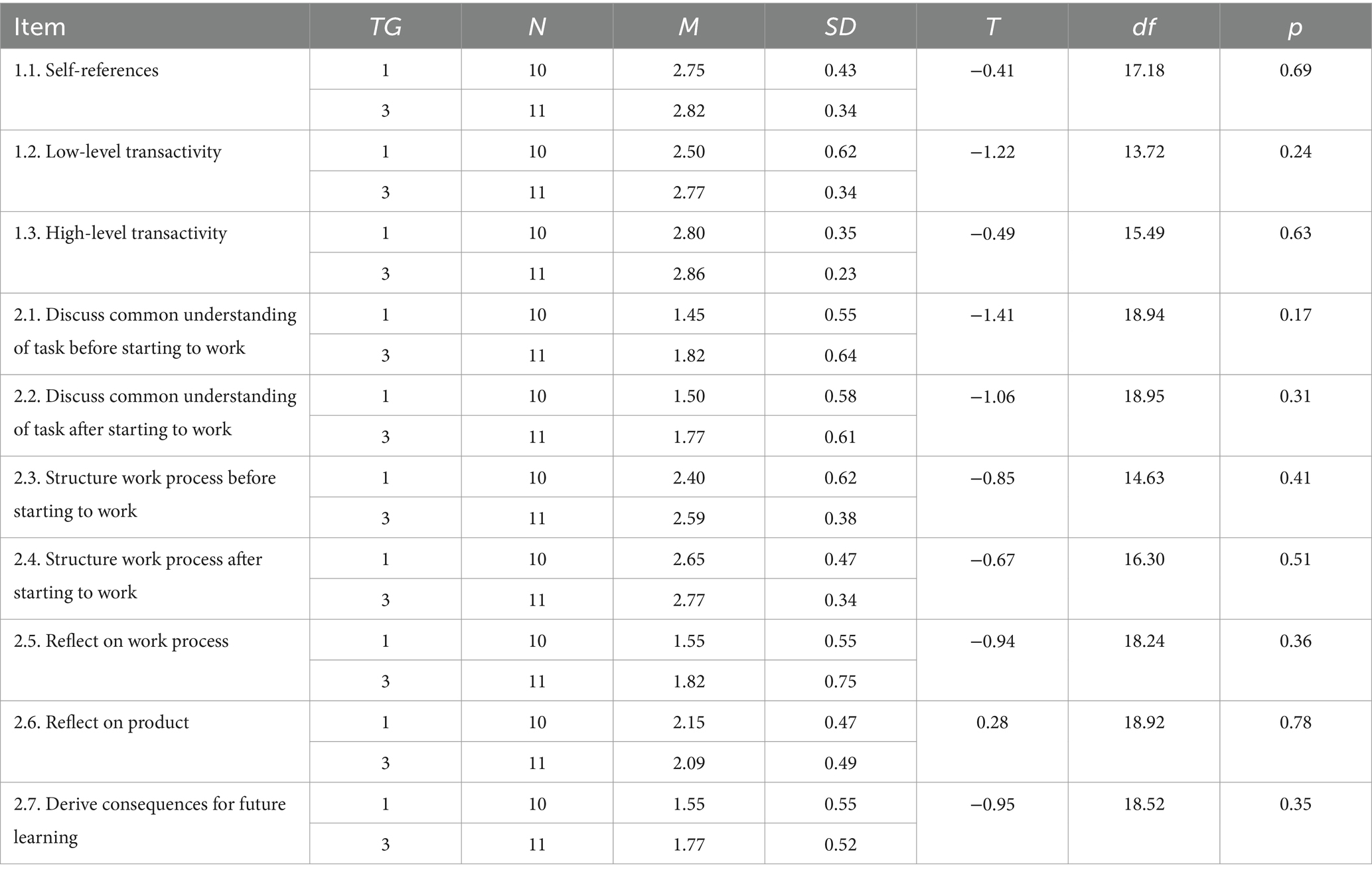
Table 11. Comparison of treatment groups TG1 and TG2, mean values of the creation of glossaries (CMP II phase) and concept maps (CMP III phase), unpaired Welch-tests.
4 Discussion
Throughout this paper, we described the successful development and implementation of a coding manual to quantify university students’ transactive communication and problem-solving activities while they created glossaries and concept maps on a difficult chemistry topic, molecular orbital theory.
In section 2.2.3, we were able to demonstrate a sufficient degree of reliability through satisfactory values for the inter-coder correlation and the internal consistency of our two scales, so that we can conclude that the manual is suitable for use in practice and to answer the three research questions underlying the structure of this paper.
With research question RQ1, we wanted to investigate how well students in our intervention were able to engage in transactive talk (RQ1a) and to what extent they followed established problem-solving patterns to structure their work on a meta-cognitive level (RQ1b).
Regarding RQ1a, we were able to measure very high amounts of transactive talk across all phases. There was only one group in which low-level transactive talk was rated on the lowest level in both phases.
From our perspective as lecturers, this is a very desirable result, because students exhibit desired behavior when they justify their own viewpoints in group discussions, ask each other questions or paraphrase the ideas of group members and expand on the contributions of their fellow students in a co-constructive manner. From our perspective as researchers, these results must be taken with a grain of salt, as the resulting ceiling effects could dilute further analyses due to an underestimation of variance within the sample (Jennings and Cribbie, 2016). To counteract this effect, several approaches are conceivable. Firstly, the 3-point scale could be refined to achieve better resolution in the higher rating range. Secondly, the analyzed material could be divided into shorter segments, allowing to better identify and differentiate phases with higher and lower degrees of transactive communication, for example. The quantitative data obtained from coding with the manual could subsequently be correlated with other data within the scope of research questions RQ2 and RQ3.
In relation to question 1b, the results indicated that students may have already learned or at least intuitively followed some problem-solving patterns, especially when it comes to strategizing before and during their work process (items 2.3 and 2.4). On the other hand, the participants in our study tended to start working on their problems immediately without clarifying the task.
Few groups actually took the time to conduct a comprehensive process-level reflection, which could have resulted from time constraints some students reported in their feedback to the intervention (cf. Hauck et al., 2023a). With regard to the creation of glossaries, this came as a surprise to us regarding the fact that the students had to use them as a foundation to create their concept maps in the succeeding seminar session.
This underlines the importance of appropriate scaffolding in collaborative problem-solving, especially because students had to start their studies under COVID-19 conditions and thus had to work together on a very difficult topic in an unfamiliar online environment with peers they probably did not know well before (Weber et al., 2022; Werner et al., 2021). This argument is supported by the observation that our ratings for the second collaborative phase (CMP III), in which students created concept maps from their glossaries, improved in all categories that students had previously struggled with, except for the creation of a shared task understanding before starting the work (item 2.1). Another plausible explanation would be that the students perceived the creation of glossaries only as a preparatory task for the creation of concept maps and therefore considered it less important. It would therefore be helpful to more explicitly emphasize the importance of the glossary for the subsequent work.
The MLR we conducted to answer RQ2 resulted in two possible predictor variables from our set of items: The influence of the students’ prior knowledge is not surprising, but a well-executed reflection on the collaboration process (item 2.7) as a predictor of the students’ knowledge gain is a major finding of this paper. It appears that students, who learn a lot, are more likely to reflect at the end of the collaboration and derive consequences for their future actions. Vice versa, it could also mean that groups, in which reflection on the task is raised to an additional meta-level, gain more from the collaboration at the end. This is an important result, as in the context of digital learning, the question of how to precisely structure collaborative scenarios to maximize learning effectiveness often arises (Chen et al., 2018; Sung et al., 2017). If certain patterns were to prove predictive of the success of individual groups beyond this study, they could be encouraged through the use of targeted scaffolds.
Against the background of these results, some methodological limitations must be discussed. First, the mean differences in conceptual knowledge between the two points of measurement are very small to a level of statistical non-significance – especially when comparing the mean values (cf. Hauck et al., 2023b). This might hinder an analysis of possible moderators such as the amount of transactive talk or adequate problem-solving behaviour. Furthermore, this might have also affected the comparison of treatment groups (RQ3a and RQ3b) who did not show any significant difference in neither conceptual knowledge, conceptual knowledge development, amount of transactive talk on any level nor in any form of problem-solving activity we measured with our manual at any measurement point. Possible reasons for this could be that the students used the creation of glossaries and concept maps primarily to consolidate the large amount of knowledge they had acquired through the previously viewed interactive learning videos (cf. Hauck et al., 2023b).
This consolidation may have had a positive impact on their long-term knowledge retention, particularly as a result of creating concept maps (see also Haugwitz et al., 2010; Nesbit and Adesope, 2006). However, follow-up testing would be required to test this hypothesis. In our design, this was not possible as our intervention ended right before the students took the exam in the corresponding course.
Second, we coded transactive talk and problem-solving activities at the group level, whereas the students’ subject knowledge was assessed for each individual participant. Although the higher resolution which could be achieved by investigating individual students’ behavior might allow for a more detailed analysis, we refrained from doing so because of several reasons: On the one hand, our approach was sufficient to answer the research questions presented in this paper. On the other hand, it allowed us to bypass technological limitations resulting from the video conferencing environment: To protect the students’ personal data, we did not record their faces in the conference. As they took part using their own devices, audio quality was poor for some students, so that not every statement could be attributed to the exact person who had made it. Furthermore, fixating on individual students might neglect the influence of their group members’ behavior. Since we analyzed both the small groups and the individual students within these groups, we cannot ensure that the individual observations are independent of one another. This limitation could be addressed by using a multilevel regression model (instead of the uni-level model we utilized), which, however, would require a larger sample size than is available in our study (cf. Maas and Hox, 2004). Nevertheless, our linear regression model allows us to capture the overall dependencies between content knowledge and the influencing variables we proposed.
Third, our data set is limited to a single cohort of students at our university. To test the generalizability of our results, studies on other content, in higher semesters, and at other universities or schools would need to be conducted.
Apart from other forms of statistical techniques or follow-up studies, several approaches are conceivable to overcome the above-mentioned limitations of our study, especially with regard to the possible loss of information during our coding process. One possibility would be to increase the resolution by splitting the units of analysis into narrower segments, e.g., 5-min intervals. So far, we have only awarded one score per category for the creation of glossaries (75 min) and for the creation of concept maps (120 min). Even if this approach was practicable for us and efficient in terms of research economics, the coarser resolution is accompanied by a loss of information. The same point of criticism arises from our purely quantitative research approach. By bundling different speech acts into one category, it is no longer possible to recognize from the data in retrospect which group, for example, asked a particularly large number of critical questions. Similarly, interesting dialog patterns cannot be captured by a single score alone. We therefore suggest that the use of coding manuals such as this one should be accompanied by qualitative methods, for example in a mixed methods setting (cf. Tashakkori and Teddlie, 2016).
Nonetheless, most of these limitations can be attributed to external variables that are beyond the scope of the study presented in this paper. In the context of our quantitative study, the manual itself proved to be a powerful tool that allowed us to quantify several dimensions of computer-supported collaborative learning in two different forms of activity on an especially difficult chemistry topic.
By identifying new appropriate indicators and anchor examples, the manual could easily be applied to other topics, including non-chemistry ones, as it encompasses all the tools necessary to quantify transactive communication and problem-solving activities in collaborative settings. The integration into other methodological contexts, e. g. as part of a mixed-methods study, is also viable, as is the use of the manual in secondary schools or other contexts and age groups beyond the university level.
Data availability statement
The datasets presented in this article are not readily available because in the context of the written consent form, we assured the students that their data will only be stored on devices belonging to our university. Hence, we are not permitted to disclose the data publicly. Requests to access the datasets should be directed to IM, aW5zYS5tZWxsZUB0dS1kb3J0bXVuZC5kZQ==.
Ethics statement
Ethical approval was not required for the studies involving humans because the study was developed in accordance to the European General Data Protection Regulation (GDPR) and conducted with university students: Each participant filled out a declaration of informed consent, which contained important information on data protection, data security, and the further processing of data. The participation in the study was voluntary for the students. Non-participation did not result in any disadvantages for them. The studies were conducted in accordance with the local legislation and institutional requirements. The participants provided their written informed consent to participate in this study.
Author contributions
DH: Conceptualization, Data curation, Formal analysis, Investigation, Methodology, Project administration, Software, Validation, Visualization, Writing - original draft, Writing - review & editing. AS: Conceptualization, Resources, Supervision, Validation, Writing – review & editing. IM: Conceptualization, Formal analysis, Funding acquisition, Methodology, Project administration, Resources, Supervision, Validation, Writing – original draft, Writing – review & editing.
Funding
The author(s) declare that financial support was received for the research, authorship, and/or publication of this article. This project was part of the “Qualitätsoffensive Lehrerbildung,” a joint initiative of the Federal Government and the Länder which aimed to improve the quality of teacher training. The programme was funded by the Federal Ministry of Education and Research, funding code 01JA2001.
Acknowledgments
The authors acknowledge the use of OpenAI’s generative AI technologies ChatGPT 4o and DeepL Write for some translations. We are grateful to Niels Reimann for his support during manual revision and double coding as well as for rating the collaborative phases with the final version of the manual and to Rita Elisabeth Krebs for proofreading the manuscript and the English translation of the manual. Furthermore, we thank Jana-Sabrin Blome, who investigated criteria for student collaboration with videos from another iteration of our study in her Master’s thesis (Blome, 2021). Some of these criteria have been retained and included in the manual. Finally, we wish to thank all students who participated in our study.
Conflict of interest
The authors declare that the research was conducted in the absence of any commercial or financial relationships that could be construed as a potential conflict of interest.
Publisher’s note
All claims expressed in this article are solely those of the authors and do not necessarily represent those of their affiliated organizations, or those of the publisher, the editors and the reviewers. Any product that may be evaluated in this article, or claim that may be made by its manufacturer, is not guaranteed or endorsed by the publisher.
Supplementary material
The Supplementary material for this article can be found online at: https://www.frontiersin.org/articles/10.3389/feduc.2024.1423330/full#supplementary-material
References
Aronson, E., and Patnoe, S. (2011). Cooperation in the classroom: The jigsaw method. London: Pinter and Martin.
Azmitia, M., and Montgomery, R. (1993). Friendship, transactive dialogues, and the development of scientific reasoning. Soc. Dev. 2, 202–221. doi: 10.1111/j.1467-9507.1993.tb00014.x
Berger, P. L., and Luckmann, T. (2011). The social construction of reality: A treatise in the sociology of knowledge. New York, NY: Open Road Media Integrated Media.
Berkowitz, M., and Gibbs, J. (1983). Measuring the developmental features of moral discussion. Merrill-Palmer Q. 29, 399–410.
Bisra, K., Liu, Q., Nesbit, J. C., Salimi, F., and Winne, P. H. (2018). Inducing self-explanation: a Meta-analysis. Educ. Psychol. Rev. 30, 703–725. doi: 10.1007/s10648-018-9434-x
Blome, J.-S. (2021). Digitale kollaborative Lernprozesse im Chemieanfangsstudium-Entwicklung und Erprobung eines Kodiermanuals [digital collaborative learning processes in undergraduate chemistry studies-development and implementation of a coding manual]. [unpublished master‘s thesis]. Dortmund: TU Dortmund University.
Brundage, M. J., Malespina, A., and Singh, C. (2023). Peer interaction facilitates co-construction of knowledge in quantum mechanics. Phys. Rev. Phys. Educ. Res. 19:201333. doi: 10.1103/PhysRevPhysEducRes.19.020133
Chen, J., Wang, M., Kirschner, P. A., and Tsai, C.-C. (2018). The role of collaboration, computer use, learning environments, and supporting strategies in CSCL: a meta-analysis. Rev. Educ. Res. 88, 799–843. doi: 10.3102/0034654318791584
Chi, M. T. H., and Wylie, R. (2014). The ICAP framework: linking cognitive engagement to active learning outcomes. Educ. Psychol. 49, 219–243. doi: 10.1080/00461520.2014.965823
Chitiyo, G., Potter, D. W., and Rezsnyak, C. E. (2018). Impact of an atoms-first approach on student outcomes in a two-semester general chemistry course. J. Chem. Educ. 95, 1711–1716. doi: 10.1021/acs.jchemed.8b00195
Coe, K., and Scacco, J. M. (2017). Content analysis, quantitative. Int. Encycl. Commun. Res. Methods 21, 1–11. doi: 10.1002/9781118901731.iecrm0045
Cohen, J. (1988). Statistical power analysis for the behavioral sciences. Hillsdale, NJ: L. Erlbaum Associates.
Cook, R. D. (1979). Influential observations in linear regression. J. Am. Stat. Assoc. 74:169. doi: 10.2307/2286747
Cooper-White, P. (2014). “Intersubjectivity” in Encyclopedia of psychology and religion. ed. D. A. Leeming (New York, NY: Springer), 882–886.
Cortina, J. M. (1993). What is coefficient alpha? An examination of theory and applications. J. Appl. Psychol. 78, 98–104. doi: 10.1037/0021-9010.78.1.98
Deiglmayr, A., and Spada, H. (2011). Training for fostering knowledge co-construction from collaborative inference-drawing. Learning Instr. 21, 441–451. doi: 10.3929/ethz-b-000130818
Döring, N., and Bortz, J. (2016). Forschungsmethoden und evaluation in den Sozial-und Humanwissenschaften [research methods and evaluation in the social and human sciences]. Berlin: Springer Berlin Heidelberg.
Döring, N., Reif, A., and Poeschl, S. (2016). How gender-stereotypical are selfies? A content analysis and comparison with magazine adverts. Comput. Hum. Behav. 55, 955–962. doi: 10.1016/j.chb.2015.10.001
Durbin, J., and Watson, G. S. (1951). Testing for serial correlation in least squares regression. Biometrika 38, 159–178. doi: 10.1093/biomet/38.1-2.159
Engelmann, T., Tergan, S.-O., and Hesse, F. W. (2009). Evoking knowledge and information awareness for enhancing computer-supported collaborative problem solving. J. Exp. Educ. 78, 268–290. doi: 10.1080/00220970903292850
Esterling, K. M., and Bartels, L. (2013). Atoms-first curriculum: a comparison of student success in general chemistry. J. Chem. Educ. 90, 1433–1436. doi: 10.1021/ed300725m
Funke, J. (2012). “Complex problem solving” in Encyclopedia of the sciences of learning: With 68 tables. ed. N. M. Seel (New York, NY: Springer), 682–685.
Gätje, O., and Jurkowski, S. (2021). When students interlink ideas in peer learning: linguistic characteristics of transactivity in argumentative discourse. Int. J. Educ. Res. Open 2:100065. doi: 10.1016/j.ijedro.2021.100065
Hattie, J. (2023). Visible learning, the sequel: A synthesis of over 2, 100 meta-analyses relating to achievement. London: Routledge Taylor and Francis Group.
Hauck, D. J., Melle, I., and Steffen, A. (2021). Molecular orbital theory—teaching a difficult chemistry topic using a CSCL approach in a first-year university course. Educ. Sci. 11:485. doi: 10.3390/educsci11090485
Hauck, D. J., Steffen, A., and Melle, I. (2023a). “A digital collaborative learning environment to support first-year students in learning molecular orbital theory” in Project-Based Education And Other Student-Activation Strategies And Issues In Science Education. eds. M. Rusek, M. Tóthova, and D. Koperová (Prague: Charles University, Faculty of Education), 178–192.
Hauck, D. J., Steffen, A., and Melle, I. (2023b). Supporting first-year students in learning molecular orbital theory through a digital learning unit. Chem. Teacher Int. 5, 155–164. doi: 10.1515/cti-2022-0040
Haugwitz, M., Nesbit, J. C., and Sandmann, A. (2010). Cognitive ability and the instructional efficacy of collaborative concept mapping. Learn. Individ. Differ. 20, 536–543. doi: 10.1016/j.lindif.2010.04.004
Igo, R. P. (2010). “Influential data points” in Encyclopedia of research design. ed. N. J. Salkind (Los Angeles, CA: SAGE), 600–602.
Jennings, M. A., and Cribbie, R. A. (2016). Comparing pre-post change across groups: guidelines for choosing between difference scores, ANCOVA, and residual change scores. J. Data Sci. 14, 205–230. doi: 10.6339/JDS.201604_14(2).0002
Johnson, D. W., and Johnson, R. T. (1999). Making cooperative learning work. Theory Pract. 38, 67–73. doi: 10.1080/00405849909543834
Jurkowski, S., and Hänze, M. (2015). How to increase the benefits of cooperation: effects of training in transactive communication on cooperative learning. Br. J. Educ. Psychol. 85, 357–371. doi: 10.1111/bjep.12077
Kaiser, H. F. (1958). The varimax criterion for analytic rotation in factor analysis. Psychometrika 23, 187–200. doi: 10.1007/BF02289233
Kim, J. H. (2019). Multicollinearity and misleading statistical results. Korean J. Anesthesiol. 72, 558–569. doi: 10.4097/kja.19087
Koo, T. K., and Li, M. Y. (2016). A guideline of selecting and reporting Intraclass correlation coefficients for reliability research. J. Chiropr. Med. 15, 155–163. doi: 10.1016/j.jcm.2016.02.012
Kyndt, E., Raes, E., Lismont, B., Timmers, F., Cascallar, E., and Dochy, F. (2013). A meta-analysis of the effects of face-to-face cooperative learning. Do recent studies falsify or verify earlier findings? Educ. Res. Rev. 10, 133–149. doi: 10.1016/j.edurev.2013.02.002
Maas, C. J. M., and Hox, J. J. (2004). Robustness issues in multilevel regression analysis. Statistica Neerlandica 58, 127–137. doi: 10.1046/j.0039-0402.2003.00252.x
Mayer, R. E. (2014). “Cognitive theory of multimedia learning,” in the Cambridge handbook of multimedia learning, ed. R. E. Mayer (Cambridge: Cambridge University Press), 43–71.
Mercer, N., and Howe, C. (2012). Explaining the dialogic processes of teaching and learning: the value and potential of sociocultural theory. Learn. Cult. Soc. Interact. 1, 12–21. doi: 10.1016/j.lcsi.2012.03.001
Nesbit, J. C., and Adesope, O. O. (2006). Learning with concept and knowledge maps: a meta-analysis. Rev. Educ. Res. 76, 413–448. doi: 10.3102/00346543076003413
Noroozi, O., Weinberger, A., Biemans, H. J., Mulder, M., and Chizari, M. (2013). Facilitating argumentative knowledge construction through a transactive discussion script in CSCL. Comput. Educ. 61, 59–76. doi: 10.1016/j.compedu.2012.08.013
OECD (2013). PISA 2012 assessment and analytical framework: Mathematics, reading, science, problem solving and financial literacy; programme for international student assessment. Paris: OECD.
Olsen, J. K., Rummel, N., and Aleven, V. (2019). It is not either or: an initial investigation into combining collaborative and individual learning using an ITS. Comput. Supp. Learning 14, 353–381. doi: 10.1007/s11412-019-09307-0
Osborne, J. W. (2015). What is rotating in exploratory factor analysis? Prac. Assessm. Res. Eval. 20:60. doi: 10.7275/HB2G-M060
Pope, A. J. (1976). The statistics of residuals and the detection of outliers. Washington, DC: NOAA.
Priemer, B., Eilerts, K., Filler, A., Pinkwart, N., Rösken-Winter, B., Tiemann, R., et al. (2020). A framework to foster problem-solving in STEM and computing education. Res. Sci. Technol. Educ. 38, 105–130. doi: 10.1080/02635143.2019.1600490
Rasch, D., Kubinger, K. D., and Moder, K. (2011). The two-sample t test: pre-testing its assumptions does not pay off. Stat Papers 52, 219–231. doi: 10.1007/s00362-009-0224-x
Riffe, D., Lacy, S., Watson, B. R., and Fico, F. (2019). Analyzing media messages: Using quantitative content analysis in research. New York, NY: Routledge Taylor and Francis Group.
Roschelle, J., and Teasley, S. D. (1995). “The construction of shared knowledge in collaborative problem solving” in Computer supported collaborative learning. ed. C. O’Malley (Berlin: Springer), 69–97.
Ruxton, G. D. (2006). The unequal variance t-test is an underused alternative to Student’s t-test and the Mann–Whitney U test. Behav. Ecol. 17, 688–690. doi: 10.1093/beheco/ark016
Schwarz, B. B., and Linchevski, L. (2007). The role of task design and argumentation in cognitive development during peer interaction: the case of proportional reasoning. Learn. Instr. 17, 510–531. doi: 10.1016/j.learninstruc.2007.09.009
Sung, Y.-T., Yang, J.-M., and Lee, H.-Y. (2017). The effects of Mobile-computer-supported collaborative learning: Meta-analysis and critical synthesis. Rev. Educ. Res. 87, 768–805. doi: 10.3102/0034654317704307
Tashakkori, A., and Teddlie, C. (2016). SAGE handbook of mixed methods in social and behavioral research. Thousand Oaks, CA: SAGE.
Teasley, S. D. (1997). “Talking about reasoning: how important is the peer in peer collaboration?” in Discourse, tools and reasoning: Essays on situated cognition. eds. L. B. Resnick, R. Säljö, C. Pontecorvo, and B. Burge (Berlin: Springer), 361–384.
Vogel, F., Wecker, C., Kollar, I., and Fischer, F. (2017). Socio-cognitive scaffolding with computer-supported collaboration scripts: a meta-analysis. Educ. Psychol. Rev. 29, 477–511. doi: 10.1007/s10648-016-9361-7
Vogel, F., Weinberger, A., Hong, D., Wang, T., Glazewski, K., Hmelo-Silver, C. E., et al. (2023). “Transactivity and knowledge co-construction in collaborative problem solving,” in Proceedings of the 16th international conference on computer-supported collaborative learning-CSCL 2023 (International Society of the Learning Sciences), 337–346.
Webb, N. M. (2009). The teacher’s role in promoting collaborative dialogue in the classroom. Br. J. Educ. Psychol. 79, 1–28. doi: 10.1348/000709908X380772
Webb, N. M., Franke, M. L., Ing, M., Wong, J., Fernandez, C. H., Shin, N., et al. (2014). Engaging with others’ mathematical ideas: interrelationships among student participation, teachers’ instructional practices, and learning. Int. J. Educ. Res. 63, 79–93. doi: 10.1016/j.ijer.2013.02.001
Webb, N. M., Ing, M., Burnheimer, E., Johnson, N. C., Franke, M. L., and Zimmerman, J. (2021). Is there a right way? Productive patterns of interaction during collaborative problem solving. Educ. Sci. 11:50214. doi: 10.3390/educsci11050214
Weber, M., Schulze, L., Bolzenkötter, T., Niemeyer, H., and Renneberg, B. (2022). Mental health and loneliness in university students during the COVID-19 pandemic in Germany: a longitudinal study. Front. Psych. 13:848645. doi: 10.3389/fpsyt.2022.848645
Weinberger, A., and Fischer, F. (2006). A framework to analyze argumentative knowledge construction in computer-supported collaborative learning. Comput. Educ. 46, 71–95. doi: 10.1016/j.compedu.2005.04.003
Werner, A. M., Tibubos, A. N., Mülder, L. M., Reichel, J. L., Schäfer, M., Heller, S., et al. (2021). The impact of lockdown stress and loneliness during the COVID-19 pandemic on mental health among university students in Germany. Sci. Rep. 11:22637. doi: 10.1038/s41598-021-02024-5
Zambrano, R. J., Kirschner, F., Sweller, J., and Kirschner, P. A. (2019). Effects of prior knowledge on collaborative and individual learning. Learn. Instr. 63:101214. doi: 10.1016/j.learninstruc.2019.05.011
Keywords: computer-supported collaborative learning, transactive talk, problem-solving, quantitative content analysis, molecular orbital theory
Citation: Hauck DJ, Steffen A and Melle I (2024) Analyzing first-semester chemistry students’ transactive talk and problem-solving activities in an intervention study through a quantitative coding manual. Front. Educ. 9:1423330. doi: 10.3389/feduc.2024.1423330
Edited by:
Christin Siegfried, University of Education Weingarten, GermanyReviewed by:
Karin Heinrichs, University of Education Upper Austria, AustriaValentina Reitenbach, University of Wuppertal, Germany
Copyright © 2024 Hauck, Steffen and Melle. This is an open-access article distributed under the terms of the Creative Commons Attribution License (CC BY). The use, distribution or reproduction in other forums is permitted, provided the original author(s) and the copyright owner(s) are credited and that the original publication in this journal is cited, in accordance with accepted academic practice. No use, distribution or reproduction is permitted which does not comply with these terms.
*Correspondence: Insa Melle, aW5zYS5tZWxsZUB0dS1kb3J0bXVuZC5kZQ==