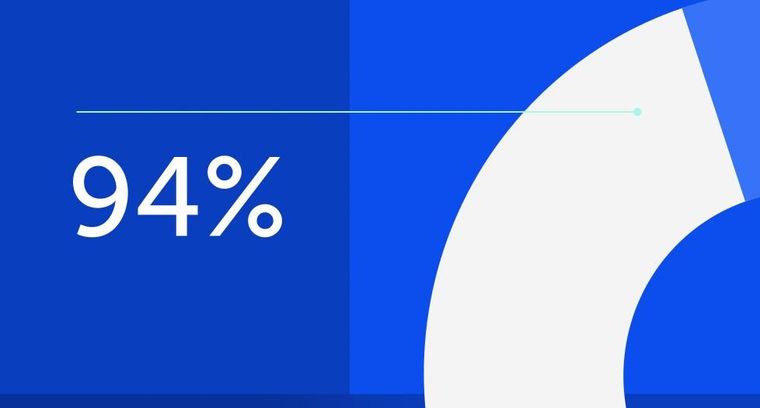
94% of researchers rate our articles as excellent or good
Learn more about the work of our research integrity team to safeguard the quality of each article we publish.
Find out more
EDITORIAL article
Front. Educ., 24 January 2022
Sec. Assessment, Testing and Applied Measurement
Volume 6 - 2021 | https://doi.org/10.3389/feduc.2021.838422
This article is part of the Research TopicRecent Approaches for Assessing Cognitive Load from a Validity PerspectiveView all 13 articles
Editorial on the Research Topic
Recent Approaches for Assessing Cognitive Load From a Validity Perspective
“Cognitive load can be defined as a multidimensional construct representing the load that performing a particular task imposes on the learner’s cognitive system” (Paas et al., 2003, p. 64). It is assumed that assessed cognitive load under various experimental conditions represents the working memory resources exerted or required during the task performance. Cognitive load is widely studied in diverse disciplines such as education, psychology, and human factors. In educational science, cognitive load is used to guide instructional designs; for instance, when developing instructional designs, overly high cognitive load should be avoided because it may hinder knowledge construction and understanding. Generally, cognitive load theory offers valuable perspectives and design principles for instruction and instructional materials (e.g., Sweller, 2005; Kirschner et al., 2006; Paas and van Merriënboer, 2020).
The present Research Topic invited contributions describing approaches to measure cognitive load and examining the validity of these approaches. “Measuring cognitive load is […] fundamentally important to education and learning” (Chandler, 2018, p.x) and several approaches have been proposed to measure cognitive load and function as indicators of learners’ working memory resources during task performance, or as input for personalized adaptive tak selection (e.g., Salden, 2006). Cognitive load measurements can also advance cognitive load theory by providing an empirical basis for testing the hypothetical effects of instructional design principles on cognitive load (Paas et al., 2003). However, Martin (2018) points out that “Finding ways to disentangle different kinds of load and successfully measure them in valid and reliable ways remains a major challenge for the research field” (p.38). The contributions of the present Research Topic address this challenge and, hence, contribute to the development of a fundamentally important area of cognitive load research.
In this editorial piece, we first provide a summary of recent approaches to measure cognitive load, use the assessment triangle (National Research Council, 2001) as a framework to systemize existing and forthcoming research in the field of cognitive load measurement, then we analyze the studies published in this Research Topic based on the assessment triangle.
Assessment of cognitive load has been a significant direction in cognitive load theory and research (e.g., Paas et al., 2003; Paas et al., 2008; Brünken et al., 2010). Although several classifications of cognitive load measurement methods have been proposed (e.g., analytic vs. empirical, Xie and Salvendy, 2000; direct vs. indirect, Brünken et al., 2010), the subjective-objective classification (see Paas et al., 2003) is most frequently used. Whereas subjective methods are based on self-reported data, objective methods use observations of performance, behavior, or physiological conditions. The self-reported data are preferably collected after each learning unit or test task with rating scales for perceived mental effort (e.g., Paas, 1992), perceived task difficulty (e.g., Kalyuga et al., 1999), or both (e.g., Krell, 2017; Ouwehand et al.). Subjective measures are considered less sensitive to fluctuations in cognitive load and are generally used to estimate overall cognitive load. This means that students give one rating for a whole learning unit or test task or a series of learning units or test tasks. Although it has been argued that it is not possible to differentiate between different types of cognitive load (i.e., intrinsic, extraneous, germane) with the conventional rating scales, recent research has shown that the scales can be adapted to differentiate between the different types of cognitive load successfully (e.g., Leppink et al., 2013; Leppink et al., 2014).
Although subjective measures have been found easy to use, valid, and reliable (e.g., Paas et al., 1994; Leppink et al., 2013; Krell, 2017), cognitive load researchers have continuously searched for objective measures, which are not influenced by opinions and perceptions of people. This research has resulted in a wide range of objective techniques. The first objective method used in cognitive load research was an analytical method to analyze the effectiveness of learning from conventional goal-specific problems versus nonspecific problems based on the number of statements in working memory, the number of productions, the number of cycles to a solution, and the total number of conditions matched (Sweller, 1988). Another objective technique is based on the so-called dual-task paradigm (Bijarsari), which holds that performance on a secondary task performed in parallel with a primary task is indicative of the cognitive load imposed by the primary task (for examples see: Chandler and Sweller, 1996; Van Gerven et al., 2000; Brünken et al., 2010). An objective technique that is increasingly used in cognitive load research is based on the assumption that changes in cognitive load are reflected by physiological variables. In this category of objective techniques, measures of the heart (e.g., heart-rate variability: e.g., Minkley et al., 2018; Larmuseau et al., 2020), brain (e.g., EEG: e.g., Antonenko et al., 2010; Wang et al., 2020), eye (e.g., pupil dilation: e.g., Lee et al., 2020) and skin (e.g., galvanic skin response: e.g., Nourbakhs et al., 2012; Hoogerheide et al., 2019) have been used (for a review see, Paas, and Van Merrienboer, 2020). Psychophysiological measures are sensitive to instantaneous fluctuations in cognitive load.
Independent of the specific approach, which is employed in a study to measure cognitive load (i.e., subjective or objective), it is crucial to provide evidence that the specific measure allows inferences about the amount of cognitive capacity that a learner invested for learning or solving a task and, if applicable, for which type of cognitive load (e.g., intrinsic, extraneous, germane). In the next section, a framework for the systematic development and evaluation of educational and psychological assessments is introduced: the assessment triangle.
The assessment triangle (Figure 1) has been proposed by the US National Research Council (National Research Council, 2001) to emphasize that each educational assessment is a means to produce some data “that can be used to draw reasonable inferences about what students know” (p.42). While this statement refers to knowledge assessment, the framework also applies to broader contexts such as cognitive load assessment. The process of collecting evidence for supporting the specific inferences a researcher aims to draw is represented in the assessment triangle as a triad of construct (What is measured?), observation (How is it measured?), and interpretation (Why can we infer from observation to construct?) (Shavelson, 2010). For an assessment to be effective, each of the three elements must be considered and be in synchrony (National Research Council, 2001). Below, we explain how each dimension of the assessment triangle is reflected in the context of cognitive load research.
FIGURE 1. Assessment triangle (adapted from Shavelson, 2010).
In the case of cognitive load assessment, the construct under consideration is cognitive load. While cognitive load is generally defined as an individual’s cognitive resources used to learn or perform a task (e.g., Paas et al., 2003), further differentiations have been proposed, distinguishing between several types of cognitive load such as extraneous, intrinsic, and germane cognitive load (e.g., Paas et al., 2003) or mental load and mental effort (e.g., Krell, 2017). For example, Choi et al. (2014) propose a model of cognitive load in which three main assessment factors of cognitive load are included: mental load, mental effort, and performance; see also (Paas and Van Merriënboer, 1994).
While the basic definition of cognitive load is shared by most researchers, the theoretical distinction between different types of cognitive load is still under debate (de Jong, 2010; Sweller et al., 2019). For example, task performance is sometimes conceptualized as being one aspect of cognitive load (e.g., Choi et al., 2014) others see it as an indicator for cognitive load (e.g., Kirschner, 2002). Furthermore, the most established conceptualization of cognitive load encompassing three types of extraneous, intrinsic, and germane cognitive load is also critically discussed (Kalyuga, 2011). For a specific cognitive load assessment, it is necessary to be clear and precise as to what one aims to measure. It is argued that an assessment approach is most effective if an explicit and clear concept of the construct of interest is used as a starting point (National Research Council, 2001).
Observation refers to the data that are collected using a specific method and aimed to be interpreted as evidence to draw inferences about the construct under investigation (National Research Council, 2001)—in this case: cognitive load. Various approaches have been suggested to measure cognitive load (Brünken et al., 2010). Subjective approaches using self-reports on rating scales are frequently used to assess cognitive load, while several objective approaches are still in an earlier stage of development and evaluation (Antonenko et al., 2010; Sweller et al., 2011; Ayres et al.). However, the validity of different approaches and the extent to which they represent cognitive load are still under debate (Solhjoo et al., 2019; Mutlu-Bayraktar et al., 2020).
Taking into consideration the different types of cognitive load suggested in the literature as well, this makes it necessary to carefully decide on which measurement method to use in a given context and for a given purpose. Furthermore, researchers should be clear about what kind of measures they decided to apply and for what reasons. Related to subjective approaches for cognitive load measurement, for instance, it is not always entirely clear which construct the items are aimed to measure. For example, many researchers use category labels related to task complexity but label them broadly as measures of cognitive load (de Jong, 2010).
Interpretation refers to the extent to which valid inferences can be drawn from data (e.g., self-reports on rating scales) to (the level of) an individual’s invested cognitive resources. The interpretation of data as evidence for an individual’s cognitive load means generalizing from a specific form of data (e.g., subjective ratings on single items) to the more global construct of cognitive load (or a specific type, such as mental effort). This is an essential step for the operationalization of the construct. However, the interpretation of measured data may be questioned, for example, if this interpretation is made only based on subjective ratings on items assessing mental effort. This is because cognitive load—as proposed by Choi et al. (2014)—is not only composed of the assessment factor of mental effort but also of mental load and performance. This demonstrates that the evaluation of the validity of the proposed interpretation of test scores is critical and complex.
In more general terms, it is proposed to consider different sources of evidence to support the claim that the proposed inferences from data to an individual’s cognitive load are valid. This is why “the evidence required for validation is the evidence needed to evaluate the claims being made” (Kane, 2015, p.64).
In the Standards for Educational and Psychological Testing, the authors elaborate on different “sources of evidence that might be used in evaluating the validity of a proposed interpretation of test scores for a particular use” (p.13). These sources of validity evidence are: Evidence based on test content, on response processes, on internal structure, and on relations to other variables (American Educational Research Association et al., 2014). Gathering evidence based on test content hereby means analyzing the relation “between the content of a test and the construct it is intended to measure” (American Educational Research Association et al., 2014, p.14). Sources of evidence based on test content often consist of expert judgments. Concerning the assessment of cognitive load, it is necessary, for example, to ask why a specific approach (e.g., subjective) has been chosen and to what extent this decision influences the intended interpretation of the obtained data. Furthermore, quality criteria such as objectivity and reliability are necessary prerequisites for the valid interpretation of test scores (American Educational Research Association et al., 2014). The current concept of validity includes aspects of reliability and fairness in testing as part of the criteria that offer evidence of a sufficient internal structure. Gathering evidence based on response processes takes into account individuals’ reasoning while answering the tasks to evaluate the extent to which the proposed inference on an individual’s cognitive resources is valid. For this purpose, research methods like interviews and think-aloud protocols are typically employed. Gathering evidence based on relations to other variables means considering relevant external variables, for example, data from other assessments (e.g., convergent and discriminant evidence) or categorical variables such as different subsamples (e.g., known groups). For example, a comparison between subjective and objective measures has been proposed as a source of validity evidence for subjective measures and as a way to learn about what the different measures are measuring (Leppink et al., 2013; Korbach et al., 2018; Solhjoo et al., 2019).
Based on the considerations above, validity can be seen as an integrated evaluative judgment on the extent to which the appropriateness and quality of interpretations based on obtained data (e.g., subjective ratings or other diagnostic procedures) are supported by empirical evidence and theoretical arguments. Hence, “validity refers to the degree to which evidence and theory support the interpretations of test scores for proposed uses of tests” (American Educational Research Association et al., 2014, p.11). Therefore, the validation of an instrument is not a routine procedure but is carried out through theory-based research, with which different interpretations of test data can be legitimized or even falsified (Hartig et al., 2008). Kane (2013) further argued that researchers have to critically demonstrate the validity of test interpretations based on a variety of evidence, especially by considering the evidence that potentially threatens the intended interpretation (“falsificationism”).
In the following, the 12 contributions of this Research Topic are analyzed and discussed based on the assessment triangle and the above thoughts on validity.
Minkley et al. based their study on the cognitive load framework Choi et al. (2014) and investigated relationships between causal and assessment factors of cognitive load in samples of secondary school students. The study aimed to test the assumed convergence between subjective (self-reported mental load and mental effort) and objective (heart rate) measures of cognitive load and to provide evidence for the assumed relationships between assessment factors of cognitive load (mental load and mental effort) and related causal factors in terms of learner characteristics (self-concept, interest and perceived stress). From their findings, the authors conclude that it is still unclear if objective measures can be validly interpreted as an indicator for an individual’s cognitive load and in which contexts. The authors emphasize the need for a clear theoretical framework of cognitive load, including the different objective measures.
Andersen and Makransky, in their contribution, evaluated an adapted version of the widely used Cognitive Load Scale by Leppink et al. (2013) called Multidimensional Cognitive Load Scale for Physical and Online Lectures (MCLS-POL). In three studies, the authors provide validity evidence based on test content (utilizing theoretical considerations and previous studies), on internal structure (through psychometric analyses), and on relations to other variables (using group comparisons). Overall, the authors conclude that their findings provide evidence for the validity of the MCLS-POL but that some minor limitations should be considered in future studies (e.g., some subscales with only a few items).
Klepsch and Seufert evaluated items that have been formulated to measure active (“making an effort”) and passive (“experiencing load”) aspects of cognitive load. The authors report on two empirical studies, which are based on theoretical considerations concerning the relationship between active and passive aspects of cognitive load and intrinsic, extraneous, and germane load, as well as established load-inducing instructional design principles (e.g., the split-attention principle). Hence, the authors address validity evidence based on test content, internal structure, and relations to other variables. The findings suggest that it is possible to distinguish between active and passive aspects of load and that this can be related to the three types of cognitive load (i.e., the active load is associated with GCL, while the passive load is associated with ICL and—less strongly—with ECL). The items were not able, however, to entirely provide the expected measures of active and passive load in the different load-inducing instructional settings.
Zu et al. investigated how learner characteristics affect the validity of a subjective assessment instrument developed to assess extraneous, intrinsic, and germane cognitive load. In three experiments, the authors asked students to sort the items of the instrument and provide reasons for their groupings (experiment 1), administered the instrument alongside an electric circuit knowledge test before and after an instructional unit on electric circuits (experiment 2), and provided students with a test including different load-inducing problems and asked them to fill out the instrument subsequently (experiment 3). Overall, the findings provide validity evidence based on test content from the target population’s view (experiment 1) and based on relations to other variables (i.e., known-groups comparison) from experiment 3; experiment 2, however, shows that the instrument’s internal structure varied depending on the students’ level of content knowledge. The authors discuss that content knowledge might moderate how students self-perceived their cognitive load. This emphasizes that learner characteristics have to be considered to draw valid inferences from self-reports on learners’ cognitive load.
Ehrich et al. propose a new cognitive load index, which is derived from item response theory (IRT) estimates of relative task difficulty. The authors argue that the proposed index combines key assessment factors of cognitive load (i.e., mental load, mental effort, and performance); hence, providing theoretical arguments as validity evidence based on test content. Empirically, Ehrich et al. administered a version of “Australia’s National Assessment Program—Literacy and Numeracy” test to calculate raw test scores from which relative task difficulty, that is, the proposed cognitive load index, was estimated. For this measure, they provide validity evidence based on internal structure (as the IRT model shows appropriate fit in the given context) and on relations to other variables. For the latter, the authors illustrate that students’ scores on two standardized assessments (numeracy and literacy) predicted the cognitive load index as expected.
Bijarsari presents in her theoretical article current taxonomies of dual tasks for capturing cognitive load. She argues that there is a lack of standardization of dual tasks over study settings and task procedures, which—in turn—results in a lack of validity of dual-task approaches and comparability between studies. Based on a review of three dual-task taxonomies, Bijarsari proposes a “holistic taxonomy of dual-task settings,” which includes parameters relevant to the design of a dual-task in a stepwise order, guiding researchers in the selection of the secondary task based on the chosen path.
Martin et al. administered the “Load Reduction Instruction Scale-Short” (LRIS-S) to students in high school science classrooms and applied multilevel latent profile analysis to identify student and classroom profiles based on students’ reports on the LRIS-S and their accompanying psychological challenge and threat orientations. The authors explicitly adopted a within- and between-network construct validity approach on both the student and the classroom level. The analysis suggested five instructional-motivational profiles (student-level within-network), which also showed differences in persistence, disengagement, and achievement (student-level between-network). At the classroom level, the authors identified three instructional-psychological profiles among classrooms (within-network) with different levels of persistence, disengagement, and achievement (between-network). Hence, the authors consider learner characteristics (motivational constructs) and environment characteristics (classrooms) and adopt a validity approach that considers evidence based on internal structure and on relations to other variables on both the student level and the classroom level.
Ayres et al., in their review, analyzed a sample of 33 experiments that used physiological measures of intrinsic cognitive load. The findings show that physiological measures related to four main categories were used in the analyzed studies (heart and lungs, eyes, skin, brain). For evaluation of the validity of the measures, the authors considered construct validity and sensitivity (i.e., the potential to detect changes in intrinsic cognitive load across tasks with different levels of complexity). The findings propose that the vast majority of physiological measures had “some level of validity” (p.13) but varied in terms of sensitivity. However, subjective measures, which were also applied in some of the studies, had the highest levels of validity. The authors conclude that a combination of physiological and subjective measures is most effective for validly and sensitively measuring intrinsic cognitive load.
Kastaun et al. examined the validity of a subjective (i.e., self-report) instrument to assess extraneous, intrinsic, and germane cognitive load during inquiry learning. Validity is evaluated by investigating relationships between causal (e.g., cognitive abilities) and assessment (e.g., eye-tracking metrics) factors about the scores on the cognitive load instrument. In two studies, secondary school students investigated a biological phenomenon and selected one of four multimedia scaffolds. Cognitive-visual and verbal abilities, reading skills, and spatial abilities were assessed as causal factors of cognitive load, and the learners indicated their representation preference by selecting one scaffold. In sum, the authors considered validity evidence based on test content and on relations to other variables, explicitly stating four validity assumptions: 1) the three scales have a sufficient internal consistency, 2) the three subjective measures detect different cognitive load levels for students in grades 9 and 11, 3) there are theoretically sound relationships between the three subjective measures and causal factors as well as 4) assessment factors. The findings consistently support assumptions 1) and 2) but only partially assumptions 3) and 4).
Thees et al. investigated the validity of two established subjective measures of cognitive load in the learning context of technology-enhanced STEM laboratory courses. Engineering students performed six experiments (presented in two different spatial arrangements) examining basic electric circuits and, immediately after the experimentation, answered both instruments. The authors analyzed various sources of validity evidence, including the instruments’ internal structure and relation to other variables (i.e., group comparison). The intended three-factorial internal structure could not be found, and several subscales showed insufficient internal consistency. Only one instrument showed the expected group differences. Based on these findings, the authors suggest a combination of items from both instruments as a more valid instrument, which, however, still has low reliability in the subscale for the extraneous cognitive load.
Ouwehand et al. investigated how visual characteristics of rating scales influenced the validity of subjective cognitive load measures. They compared four rating scale measures differing in visual appearance (two numerical scales and two pictorial scales), which asked participants to rate mental load and mental effort after working on simple and complex tasks. The authors address validity evidence on test content (by asking the respondents to comment on the scales in an open-ended question) and on relations to other variables (by comparing resulting measures between scale type and task complexity). The findings show that all scales revealed expected differences in mental load and mental effort between simple and complex tasks; however, numerical scales provided expected relationships between cognitive load measures and performance on complex tasks more clearly than visual scales, while the opposite was found for simple tasks. In sum, this study hints that subtleties in measurements (i.e., item surface features such as visual appearance) can influence findings and, hence, could be a potential threat to the valid interpretation of test scores.
Schnaubert and Schneider investigated the relationship between perceived mental load and mental effort and comprehension and metacomprehension under different design conditions of multimedia material. The authors varied the design of the learning material (text-picture integrated, split attention, active integration) and tested for direct and indirect effects of mental load and mental effort on metacomprehension judgments. Beyond indirect effects via comprehension, both mental load and mental effort were directly related to metacomprehension (which differed between the multimedia design conditions). Based on their findings, the authors discuss that subjectivity (i.e., subjective experience of cognitive processes) needs to be considered more explicitly for validly assessing cognitive load with subjective methods.
To summarize, the present Research Topic includes two theoretical papers (i.e., literature reviews) and ten empirical studies. The theoretical papers show, for the specific areas of analysis, that there is a lack of validity in and comparability between most studies using dual tasks to capture cognitive load (Bijarsari) and that the validity and sensitivity are limited for most physiological approaches (Ayres et al.). Both studies illustrate the need for further research in terms of conceptual clarification and methods development and evaluation, respectively. Compared to other constructs, such as general cognitive abilities (e.g., Liepmann et al., 2007) or domain-specific competencies (e.g., Krüger et al., 2020), standardized representative validation studies for cognitive load assessments are highly needed but widely missing. Hence, instead of evaluating new methods of cognitive load assessment, further systematic study of the different existing methods is needed. For example, to investigate under what conditions and for whom a specific subjective method works well and why would be more critical than just applying an existing or a new method. In addition, the measurement methods are typically considered one of several dependent variables in complex learning environments, which consist of many other elements (Choi et al., 2014). Therefore, it is challenging to disentangle the differential effects on the cognitive load measures. More fundamental research into the methods is needed to get a detailed picture of the factors affecting the obtained measures. For instance, Ouwehand et al. demonstrated that research on item surface features could provide valuable insights on the validity of subjective ratings. Such fundamental research should precede more applied research.
Of the ten empirical studies, one used cognitive load measures to investigate the proposed relationship between causal and assessment factors of cognitive load. Based on their findings, Minkley et al. specifically emphasize the need for precise conceptual integration of the various objective measures for cognitive load. The remaining nine empirical studies provide studies on the validity of newly developed cognitive load measures (e.g., active and passive load; Klepsch and Seufert) or on the validity of established measures adapted to new contexts (e.g., technology-enhanced STEM laboratory courses; Thees et al.). Studies of the latter type show that published scales should be evaluated before they can be validly used in new contexts.
Besides proposing specific cognitive load measures, the empirical studies in this Research Topic also provide valuable findings for cognitive load assessment in general. For example, Zu et al. show that learner characteristics should be considered to interpret subjective cognitive load measurements validly. All studies in this Research Topic found—to a greater or lesser extent—next to supportive evidence also evidence that potentially threatens the validity of the investigated measures. For example, Thees et al. could not find the assumed three-factorial internal structure of their data, and several of their subscales showed insufficient internal consistency. Generally, it is likely that the unsolved issue of how to conceptualize cognitive load (e.g., two vs. three types; Kalyuga, 2011) highly influences cognitive load measures and their validity—at least if scholars do not evaluate the appropriateness of the selected approach for their study context. Furthermore, it is also crucial for researchers to reflect on the consequences of new developments in cognitive load theory in the research on cognitive load measurement. One example is the recently identified possibility of working memory resource depletion, which may occur following extensive mental effort (Chen et al., 2018).
Concluding, the studies collected in this Research Topic illustrate the need for further research on the validity of interpretations of data as indicators for cognitive load. Such research, to be systematic and theory-guided, can be fruitfully framed within the assessment triangle (Figure 1).
Conceptualization, MK; writing—original draft preparation, MK; writing—review and editing, MK, KX, GR, FP.
The authors declare that the research was conducted in the absence of any commercial or financial relationships that could be construed as a potential conflict of interest.
All claims expressed in this article are solely those of the authors and do not necessarily represent those of their affiliated organizations, or those of the publisher, the editors and the reviewers. Any product that may be evaluated in this article, or claim that may be made by its manufacturer, is not guaranteed or endorsed by the publisher.
American Educational Research Association (2014). “American Psychological Association, & National Council on Measurement in Education,” in Standards for Educational and Psychological Testing (Washington, DC: American Educational Research Association).
Antonenko, P., Paas, F., Grabner, R., and van Gog, T. (2010). Using Electroencephalography to Measure Cognitive Load. Educ. Psychol. Rev. 22, 425–438. doi:10.1007/s10648-010-9130-y
Brünken, R., Seufert, T., and Paas, F. (2010). “Cognitive Load Measurement,” in Cognitive Load Theory. Editors J. Plass, R. Moreno, and R. Brünken (New York: Cambridge University Press), 181–202.
Chandler, P. (2018). “Foreword,” in Cognitive Load Measurement and Application. Editor R. Zheng (New York, NY: Routledge).
Chandler, P., and Sweller, J. (1996). Cognitive Load while Learning to Use a Computer Program. Appl. Cognit. Psychol. 10 (2), 151–170. doi:10.1002/(sici)1099-0720(199604)10:2<151:aid-acp380>3.0.co;2-u
Chen, O., Castro-Alonso, J. C., Paas, F., and Sweller, J. (2018). Extending Cognitive Load Theory to Incorporate Working Memory Resource Depletion: Evidence from the Spacing Effect. Educ. Psychol. Rev. 30, 483–501. doi:10.1007/s10648-017-9426-2
Choi, H.-H., van Merriënboer, J. J. G., and Paas, F. (2014). Effects of the Physical Environment on Cognitive Load and Learning: towards a New Model of Cognitive Load. Educ. Psychol. Rev. 26, 225–244. doi:10.1007/s10648-014-9262-6
de Jong, T. (2010). Cognitive Load Theory, Educational Research, and Instructional Design: Some Food for Thought. Instr. Sci. 38, 105–134. doi:10.1007/s11251-009-9110-0
Hartig, J., Frey, A., and Jude, N. (2008). “Validität,” in Testtheorie und Fragebogenkonstruktion. Editors H. Moosbrugger, and A. Kelava (Springer), 135–163. doi:10.1007/978-3-540-71635-8_7
Hoogerheide, V., Renkl, A., Fiorella, L., Paas, F., and Van Gog, T. (2019). Enhancing Example-Based Learning: Teaching on Video Increases Arousal and Improves Problem-Solving Performance. J. Educ. Psychol. 111, 45–56. doi:10.1037/edu0000272
Kalyuga, S., Chandler, P., and Sweller, J. (1999). Managing Split-Attention and Redundancy in Multimedia Instruction. Appl. Cognit. Psychol. 13 (4), 351–371. doi:10.1002/(sici)1099-0720(199908)13:4<351:aid-acp589>3.0.co;2-6
Kalyuga, S. (2011). Cognitive Load Theory: How many Types of Load Does it Really Need. Educ. Psychol. Rev. 23, 1–19. doi:10.1007/s10648-010-9150-7
Kane, M. T. (2013). Validating the Interpretations and Uses of Test Scores. J. Educ. Meas. 50, 1–73. doi:10.1111/jedm.2013.50.issue-110.1111/jedm.12000
Kane, M. (2015). “Validation Strategies. Delineating and Validating Proposed Interpretations and Uses of Test Scores,” in Handbook of Test Development. Editors M. Raymond, S. Lane, and T. Haladyna (New York: Routledge), 64–80.
Kirschner, P. A. (2002). Cognitive Load Theory: Implications of Cognitive Load Theory on the Design of Learning. Learn. Instruction 12, 1–10. doi:10.1016/s0959-4752(01)00014-7
Kirschner, P. A., Sweller, J., and Clark, R. E. (2006). Why Minimal Guidance during Instruction Does Not Work: An Analysis of the Failure of Constructivist, Discovery, Problem-Based, Experiential, and Inquiry-Based Teaching. Educ. Psychol. 41, 75–86. doi:10.1207/s15326985ep4102_1
Korbach, A., Brünken, R., and Park, B. (2018). Differentiating Different Types of Cognitive Load: A Comparison of Different Measures. Educ. Psychol. Rev. 30, 503–529. doi:10.1007/s10648-017-9404-8
Krell, M. (2017). Evaluating an Instrument to Measure Mental Load and Mental Effort Considering Different Sources of Validity Evidence. Cogent Educ. 4, 1280256. doi:10.1080/2331186X.2017.1280256
Krüger, D., Hartmann, S., Nordmeier, V., and Upmeier zu Belzen, A. (2020). “Measuring Scientific Reasoning Competencies,” in Student Learning in German Higher Education. Editors O. Zlatkin-Troitschanskaia, H. Pant, M. Toepper, and C. Lautenbach (Springer), 261–280. doi:10.1007/978-3-658-27886-1_13
Larmuseau, C., Cornelis, J., Lancieri, L., Desmet, P., and Depaepe, F. (2020). Multimodal Learning Analytics to Investigate Cognitive Load during Online Problem Solving. Br. J. Educ. Technol. 51 (5), 1548–1562. doi:10.1111/bjet.12958
Lee, J. Y., Donkers, J., Jarodzka, H., Sellenraad, G., and van Merriënboer, J. J. G. (2020). Different Effects of Pausing on Cognitive Load in a Medical Simulation Game. Comput. Hum. Behav. 110, 106385. doi:10.1016/j.chb.2020.106385
Leppink, J., Paas, F., Van der Vleuten, C. P., Van Gog, T., and Van Merriënboer, J. J. (2013). Development of an Instrument for Measuring Different Types of Cognitive Load. Behav. Res. Methods 45, 1058–1072. doi:10.3758/s13428-013-0334-1
Leppink, J., Paas, F., Van Gog, T., Van der Vleuten, C. P. M., and van Merriënboer, J. J. G. (2014). Effects of Pairs of Problems and Examples on Task Performance and Different Types of Cognitive Load. Learn. Instruction 30, 32–42. doi:10.1016/j.learninstruc.2013.12.001
Liepmann, D., Beauducel, A., Brocke, B., and Amthauer, R. (2007). Intelligenz-Struktur-Test 2000 R (I-S-T 2000 R). Göttingen: Hogrefe.
Martin, S. (2018). “A Critical Analysis of the Theoretical Construction and Empirical Measurement of Cognitive Load,” in Cognitive Load Measurement and Application. Editor R. Zheng (New York, NY: Routledge), 29–44.
Minkley, N., Kärner, T., Jojart, A., Nobbe, L., and Krell, M. (2018). Students' Mental Load, Stress, and Performance when Working with Symbolic or Symbolic-Textual Molecular Representations. J. Res. Sci. Teach. 55, 1162–1187. doi:10.1002/tea.21446
Mutlu-Bayraktar, D., Ozel, P., Altindis, F., and Yilmaz, B. (2020). Relationship between Objective and Subjective Cognitive Load Measurements in Multimedia Learning. Interactive Learn. Environments, 1–13. doi:10.1080/10494820.2020.1833042
Nourbakhsh, N., Wang, Y., Chen, F., and Calvo, R. A. (2012). Using Galvanic Skin Response for Cognitive Load Measurement in Arithmetic and reading tasks. in”Proceedings of the 24th Conference on Australian Computer-Human Interaction OzCHI. New York, NY: Association for Computing Machinery.
National Research Council (2001). Knowing what Students Know: The Science and Design of Educational Assessment. Washington, DC: National Academy Press. doi:10.1145/2414536.2414602
Paas, F., Ayres, P., and Pachman, M. (2008). “Assessment of Cognitive Load in Multimedia Learning Environments: Theory, Methods, and Applications,” in Recent Innovations in Educational Technology that Facilitate Student Learning. Editors D. Robinson, and G. Schraw (Charlotte, NC: Information Age Publishing), 11–35.
Paas, F. G., Van Merriënboer, J. J., and Adam, J. J. (1994). Measurement of Cognitive Load in Instructional Research. Percept Mot. Skills 79, 419–430. doi:10.2466/pms.1994.79.1.41910.2466/pms.1994.79.1.419
Paas, F. G. W. C. (1992). Training Strategies for Attaining Transfer of Problem-Solving Skill in Statistics: A Cognitive-Load Approach. J. Educ. Psychol. 84, 429–434. doi:10.1037/0022-0663.84.4.429
Paas, F., Tuovinen, J. E., Tabbers, H., and Van Gerven, P. W. M. (2003). Cognitive Load Measurement as a Means to advance Cognitive Load Theory. Educ. Psychol. 38, 63–71. doi:10.1207/S15326985EP3801_8
Paas, F., and Van Merriënboer, J .J. G. (1994). Instructional Control of Cognitive Load in the Training of Complex Cognitive Tasks. Educ. Psychol. Rev. 6, 51–71. doi:10.1007/bf02213420
Paas, F., and van Merriënboer, J. J. G. (2020). Cognitive-load Theory: Methods to Manage Working Memory Load in the Learning of Complex Tasks. Curr. Dir. Psychol. Sci. 29, 394–398. doi:10.1177/0963721420922183
Salden, R. J. C. M., Paas, F., and van Merriënboer, J. J. G. (2006). Personalised Adaptive Task Selection in Air Traffic Control: Effects on Training Efficiency and Transfer. Learning and Instruction 16, 350–362.
Shavelson, R. J. (2010). On the Measurement of Competency. Empirical Res. Voc Ed. Train. 2, 41–63. doi:10.1007/bf03546488
Solhjoo, S., Haigney, M. C., McBee, E., van Merriënboer, J. J. G., Schuwirth, L., Artino, A. R., et al. (2019). Heart Rate and Heart Rate Variability Correlate with Clinical Reasoning Performance and Self-Reported Measures of Cognitive Load. Sci. Rep. 9, 14668. doi:10.1038/s41598-019-50280-3
Sweller, J., Ayres, P., and Kalyuga, S. (2011). “Measuring Cognitive Load,” in Cognitive Load Theory. Editors J. Sweller, P. Ayres, and S. Kalyuga (New York, NY: Springer), 71–85. doi:10.1007/978-1-4419-8126-4_6
Sweller, J. (1988). Cognitive Load during Problem Solving: Effects on Learning. Cogn. Sci. 12 (2), 257–285. doi:10.1207/s15516709cog1202_4
Sweller, J. (2005). “Implications of Cognitive Load Theory for Multimedia Learning,” in The Cambridge Handbook of Multimedia Learning. Editor R. E. Mayer (New York, NY: Cambridge University Press), 19–30. doi:10.1017/cbo9780511816819.003
Sweller, J., van Merriënboer, J. J. G., and Paas, F. (2019). Cognitive Architecture and Instructional Design: 20 Years Later. Educ. Psychol. Rev. 31, 261–292. doi:10.1007/s10648-019-81909465-5
Van Gerven, W. M., Fred, G. W. C., Paas, F., Van Merriënboer, J. J. G., and Schmidt, H. G. (2000). Cognitive Load Theory and the Acquisition of Complex Cognitive Skills in the Elderly: Towards an Integrative Framework. Educ. Gerontol. 26, 503–521. doi:10.1080/03601270050133874
Wang, J., Antonenko, P., Keil, A., and Dawson, K. (2020). Converging Subjective and Psychophysiological Measures of Cognitive Load to Study the Effects of Instructor‐Present Video. Mind, Brain Educ. 14 (3), 279–291. doi:10.1111/mbe.12239
Keywords: cognitive load, mental load, mental effort, measurement, assessment triangle, validity, subjective and objective measures
Citation: Krell M, Xu KM, Rey GD and Paas F (2022) Editorial: Recent Approaches for Assessing Cognitive Load From a Validity Perspective. Front. Educ. 6:838422. doi: 10.3389/feduc.2021.838422
Received: 17 December 2021; Accepted: 31 December 2021;
Published: 24 January 2022.
Edited and reviewed by:
Gavin T. L. Brown, The University of Auckland, New ZealandCopyright © 2022 Krell, Xu, Rey and Paas. This is an open-access article distributed under the terms of the Creative Commons Attribution License (CC BY). The use, distribution or reproduction in other forums is permitted, provided the original author(s) and the copyright owner(s) are credited and that the original publication in this journal is cited, in accordance with accepted academic practice. No use, distribution or reproduction is permitted which does not comply with these terms.
*Correspondence: Moritz Krell, a3JlbGxAbGVpYm5pei1pcG4uZGU=
Disclaimer: All claims expressed in this article are solely those of the authors and do not necessarily represent those of their affiliated organizations, or those of the publisher, the editors and the reviewers. Any product that may be evaluated in this article or claim that may be made by its manufacturer is not guaranteed or endorsed by the publisher.
Research integrity at Frontiers
Learn more about the work of our research integrity team to safeguard the quality of each article we publish.