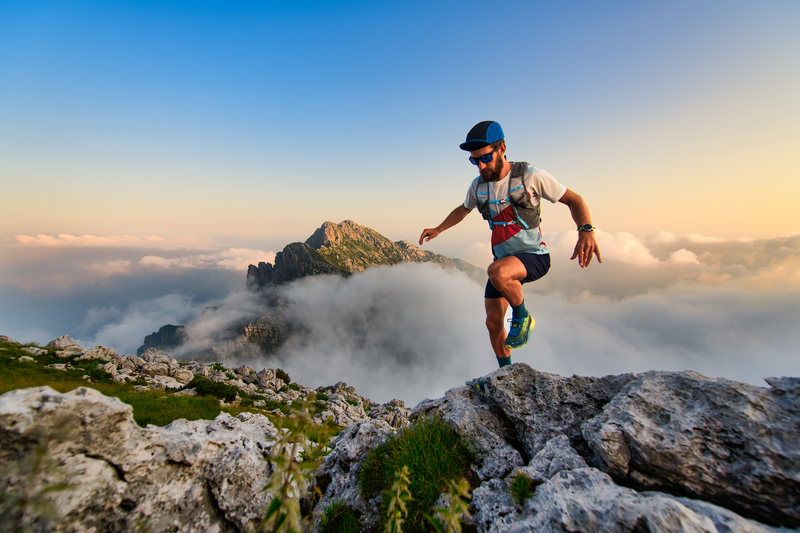
95% of researchers rate our articles as excellent or good
Learn more about the work of our research integrity team to safeguard the quality of each article we publish.
Find out more
ORIGINAL RESEARCH article
Front. Educ. , 28 January 2022
Sec. Digital Education
Volume 6 - 2021 | https://doi.org/10.3389/feduc.2021.783087
After the outbreak of novel coronavirus (COVID-19) in late December 2019, in an attempt to mitigate its development, the decision to close institutions around the world was made. To continue imparting education and delivering the learning material to their students, many institutions adopted for digital or E-learning. To support those institutions attempting to digitize their learning during this pandemic, the main aim of this study is to examine the students’ accessibility to and success of E-learning portals. Using the DeLone and McLean (D&M) Model, the study explains the differences between female and male students’ accessibility to E-learning portals. This study compares female and male student groups regarding the usage of the E-learning portal in the higher education context. Using an online google survey, the data were collected from 254 students, including males and females. The study utilized PLS-SEM to perform a multi-group analysis examining female and male student groups. The study found a significant and direct relationship of e-service quality with system use and user satisfaction for females and male student groups. System quality also supported the relationship with user satisfaction. The study further revealed a significant and positive relationship between system use and user satisfaction with E-learning portal success for females and male student groups. This study also concluded that insignificant difference exists in using the E-learning portal between female and male student in higher education institutions.
The outbreak of the COVID-19 was first identified in late December 2019 in Wuhan, one of China’s nine most populous cities. The World Health Organization (WHO) declared COVID-19 a pandemic on March 11, 2020 (Huang et al., 2020). As of late December 2019, there were at least 266 recorded cases of the disease in Hubei, China. However, as Wuhan is the business hub and significant rail interchange, the virus spread to other major cities in China. By mid-January 2020. COVID-19 had spread across borders between people through direct or indirect contact or contaminated surfaces and objects (Chaplin, 2020). The pandemic outbreak has had overarching consequences beyond the spread of the disease itself, including quarantine leading to economic, political, cultural, and social disruptions. The impacts on the education system brought about the closure of schools, colleges, and universities worldwide. The closure disrupted the learning processes of more than 1.6 children across the globe (United Nations, 2020).
Like other nations, the government of Afghanistan announced the closure of all private and public higher education institutions (HEIs) across the country from March 14 to April 17, 2020, which was later extended to late May 2020 (UNDP Afghanistan, 2020). This crisis stimulated innovative approaches within the education system. To curb educational impact during the pandemic, various means of distance education, including radio, television, taking learning packages home, and online learning networks, have been used (Warangadinata et al., 2020). With the distinctive rise of e-learning, technology has been the most critical lifeline for education worldwide, where teaching and learning take place on digital platforms. Technology-based learning covers the term e-learning that includes learning through various electronic educational technologies and online networks that aim to facilitate the independent learning of students (Basak et al., 2018).
Most universities worldwide offer some type of online courses for their students on and off campuses during this pandemic. In Afghanistan, the Ministry of Higher Education (MoHE) issued several guidelines for operations during COVID-19 time. Consistent with these guidelines, many private and public universities started online courses from late March 2020 (Aljazeera English, 2020).
In terms of the use of technology and web environment for e-learning, authors have emphasized on considering the gender as the differences exist in their learning style (Garland and Martin, 2005; Cuadrado-García et al., 2010; Hung et al., 2010). However, previous studies have shown inconclusive findings on the gender and e-learning. For instance, on the one hand, studies have demonstrated that gender has a profound impact on the success of e-learning portals as females and males differ in their use of technology and satisfaction (Lu and Chiou, 2010; He and Freeman, 2014; Ramírez-Correa et al., 2015). On the other hand, some research studies suggest that gender has no effect on satisfaction or attitudes towards e-learning (Cuadrado-García et al., 2010; Hung et al., 2010), or on teaching results (Kay and Knaack, 2008; Chu and Chu, 2010). These inconsistent findings with regards to the relationship between gender differences and e-learning call for further research to validate if gender difference affect the e-learning portal (ELP) success in the higher education context of Afghanistan. In addition, authors have noted lack of gender related research in the field of web-based learning (Ramírez-Correa et al., 2015). Thus, exploring the success of the e-learning platform from both female and male universities’ students’ perspectives is essential. To fill this need, this comparative study examines if the usage of female and male university students of the E-learning platform is different during the pandemic.
In this study, the D&M model was tested on female and male university students in Afghanistan. Many private universities and some public universities have been offering online and blended education options in Afghanistan. The present study emphases the satisfaction of the female and male users (students) and e-learning system usage leading to e-learning portal success in Afghan universities. Many scholars have claimed that user satisfaction and e-learning system usage are significant determinants of ELP success (Cidral et al., 2018; Selvaraj 2019). Giving centrality to ELP success, this study considers students enrolled in Afghan universities currently using the E-learning portals. Based on research, a knowledge gap exists in e-learning systems literature after the pandemic and closure of HEIs. The study investigated the effect of e-service quality (ESQ), information quality (IQ), and system quality (SQ) on user satisfaction (US) and system use (SU), which subsequently result in ELP success.
This study is divided into five major sections. Section 1 introduces the subject matter; section 2 briefly reviews extant literature about how the learning curve has shifted towards online portals by using the DeLone and McLean Model. Section 3 contains a description of the research method and the overall data collection and analysis processes. Section 4 contains a Multi-Group Analysis (MGA) and the interpretation of the results. Lastly, Section 5 presents a holistic discussion related to comparing female and male groups.
Over the last 2 decades, technological advancement has modernized the overall educational system across the world. The shift towards online education is one of the most powerful trends in global education. This literature review section presents information on the shift towards online education and its extensive use during the COVID-19 pandemic. It further provides a general definition of Information System (IS) success covering various evaluation perspectives by discussing the DeLone and McLean (D&M) model.
The rapid evolution of technology has made distance education easily accessible in all disciplines, and it is popular among students for its flexibility, convenience, and customized learning experience (McBrien et al., 2009; Arpaci et al., 2020). The term e-learning is illustrated via a variety of terms. Most of these terms like blended learning, computer-mediated learning, online learning, M-learning, open learning, and web-based learning refer to a technological device connected to a network that facilitates learning anywhere and anytime (Cojocariu et al., 2014; Al-Maroof and Al-Emran, 2021). Through online education, teaching-learning has become more innovative, flexible, and independent. Online learning can occur in either synchronous or asynchronous environments using various technologies and devices such as the worldwide web, chat, email, texts, video, and audio conferencing over computer networks (Indira and Sakhshi, 2017; Al-Nuaimi et al., 2021). Synchronous online learning is a real-time interaction between educators and learners through live lectures, where a possibility of instant feedback exists. Asynchronous learning is a flexible, student-centered approach, where educational materials are available on various systems and forums. Instant feedback or live discussion is not possible in an asynchronous learning environment (Hrastinski, 2008). The benefits and limitations of these two approaches depend on the affordances of the various media. Synchronous learning offers social interaction, whereas asynchronous learning provides independent learning (McBrien et al., 2009).
Online learning requires careful planning and extensive resources. Moreover, E-learning students need more support than face-to-face students, and online educational systems offer the flexibility to provide additional skills development training to students who have limited exposure to technology (Sharma and Dogra, 2012). Despite being used for more than a decade, online learning is in the literature is still described as a new means of teaching and learning (Cojocariu et al., 2014). The digitalization of educational content was relatively uncommon before the current pandemic started. Only 20% of the countries worldwide had online learning resources in schools, and only 10% of the countries had digital resources available outside the schools (European Data Portal, 2020). No standard and universal digital educational curriculum existed anywhere in the world.
However, governments worldwide have put forth tremendous efforts to move to digital learning to ensure continuity of education in this time of crisis (The World Bank, 2020; Al-Nuaimi et al., 2021). To introduce successful online learning systems, understanding the perspectives of learners and accordingly introducing platform and technology is essential (Williams et al., 2020). The ideal digital educational platforms can accommodate numerous students in a video conference; instant discussions are possible with students. They can support materials through any digital device, including mobile phones, facilitate instant feedback, record lecture, and submit assignments.
At the end of January 2020, the world was alerted to the severe outbreak of COVID-19, which created a global pandemic. The world went on quarantine after wide-spread of the epidemic. Within days schools, colleges, and universities closed in more than 120 countries. This overnight shift forced institutions to adopt new technologies such as Zoom, Google Classroom, Microsoft Teams, and Webex Blackboard (Dignan, 2020; Al-Nuaimi et al., 2021).
COVID-19 presented challenges for the institutions managing online education, educators, and learners. During the demanding circumstances, one concern was how education could continue. The shift to the online system was the only practical option. Although online platforms could support the delivery of education, institutions needed to adapt teaching-learning methods to an online environment in a massive manner (Al-Emran and Teo, 2020; Carey, 2020). Delivering online courses has three primary requirements: 1) access to the Internet, 2) the correct technological device, and 3) the skills to use technology. Unfortunately, even though online education has been the only and best alternative to traditional education during the COVID-19 crisis, many nations cannot fully digitalize their education systems. Global estimates show that 826 million learners lack access to a computer; 706 million do not have the Internet, and more than 56 million lack network coverage (UNESCO, 2020).
Online education took a new turn with the appearance of Massive Open Online Courses (MOOCs) in 2012 (Calisir et al., 2014; Margaryan et al., 2015). The extensive demand and use of MOOCs are viewed as a momentous development in higher education. Millions of students benefit from the resources available on MOOCs (Arpaci et al., 2020). Many HEIs in Afghanistan have recognized the value of online platforms as an essential instructional tool to assure the continuity of education during the COVID-19 lockdown. They have developed online learning management systems (LMS) similar to MOOCs (Mohammadi, 2015). While the move to online education enabled many students to continue learning, many challenges must be overcome in Afghanistan. Among the issues is an absence of access to reliable internet services, lack of digital devices, power outages, lack of trained lecturers, the interface of LMS designs, use of the system, and student adoption of technology.
Effective and useful measurement of information systems (IS) success is necessary to understand the value of investments in the course design and delivery and their outcomes. Hellstén and Markova (2006) posited that the measure of IS success measurement is critical as it is a multidimensional concept assessed at various levels, and several factors contribute to IS success. One of these is gender, which plays a crucial role in determining that success. In terms of IS success, females and males have been found to differ concerning trust, information processing, usage, and satisfaction level (Gaitan et al., 2010). Given these differences, the present study compares the user satisfaction and system usage of female and male students and their impacts on ELP success using the DeLone and McLean Model (D&M)-IS success model.
DeLone and McLean introduced the D&M model in 1992 to measure various dimensions of IS success. The model was based initially on communications, classification of information output, and research on information systems (Mason, 1978). Later, based on the work of various researchers, IS success was seen to encompass six major interdependent dimensions, including information quality, individual impact, organizational impact, system quality, system use, and user satisfaction (Hellstén and Markova, 2006).
A decade later, the original authors refined their work based on the response to the feedback of scholars in this area. As a result, DeLone and McLean included service quality dimensions in their revised model. They replaced the individual and organizational impact with net benefits to broaden the impacts of IS to industries, groups, and nations (DeLone and McLean, 2003). Based on the communication construct of Shannon and Weaver (1949), the technical level of communication is the efficiency and accuracy of the communication system, the semantic level is about conveying the intended meaning of the message, and the effectiveness level refers to the impact of information on the receiver (Mohammadi, 2015).
Figure 1 below shows the six-dimensional D&M model. In this model, “systems quality” measures technical success, “information quality” measures semantic success, and the rest of the four components, “use,” “user satisfaction,” “individual impact,” and “organizational impact” measures “effectiveness success.”
FIGURE 1. Updated DeLone and McLean IS success model (D&MISSM). Source: DeLone and McLean (2003).
The modified version of the D&M model addressed the criticism and recommendations received from many scholars. A plethora of scientific papers cited this model, which is among the most successful and influential studies in contemporary IS research (Al-Kofahi et al., 2020). Each of the six major dimensions shown in Figure 1 has a unique set of characteristics, and their practical application depends on the organizational context (Petter et al., 2008; DeLone and McLean, 2016). In their meta-analytic review Al-Kofahi et al. (2020) argued that D&M model might be appropriate for scrutinizing the individual or organisational systems’ impact, especially on the performance’s aspect. Up until now, to assess IS, D&M model has been applied in several fields including higher education (Petter et al., 2008; DeLone and McLean, 2016; Rana and Dwivedi, 2018; Widjaja et al., 2018; Al-Kofahi et al., 2020; Al-Nuaimi and Al-Emran, 2021).
Review of the recent literature reveal that several authors have applied DeLone and Mclean framework in the education sector of different countries by focusing on its adoption or successful implementation among students (Cidral et al., 2018; Yakubu and Dasuki, 2018; Dalle et al., 2020; Dikovic et al., 2020; Fearnley and Amora, 2020; Fearnley and Amora, 2020; Hadoussa, 2020; Khand and Kalhoro, 2020; Nugroho et al., 2020; Safsouf et al., 2020; Zaineldeen et al., 2020). However, limited studies have considered the D&M-IS success from the gender perspective which is critical for its success, thusly, indicating a literature gap. In addition, scholars such as Al-Kofahi et al. (2020) called for further research on the validation of D&M-IS success model from the low-income countries such as Afghanistan which lack high level of technological advancements. Hence, responding to the research call and bridging the gap, this study examines the D&M-IS success from the gender perspective in the higher education context.
DeLone and McLean (2003) argued that the researchers who apply the D&M model must understand the information system and organization under study and accordingly select a success dimension. Concerning the DeLone and McLean’s model, the meta-analytic review of Nguyen et al. (2015) revealed that, in IS success, success is denoted in the form of individual benefits. Therefore, the current study measures individual benefits in the form of ELP success.
The SQ dimension depicts desirable characteristics of information systems, i.e., flexibility, reliability, easy to use, intuitive, and sophisticated. Several studies in the past have indicated that SQ is vital for any online learning portal’s success because it increases the user’s interest and satisfaction (Ifinedo, 2014; Mohammadi, 2015; Dalle et al., 2020; Fearnley and Amora, 2020; Hadoussa, 2020) and behavioral intention to use (i.e., system use) (Mohammadi, 2015; Yakubu and Dasuki, 2018; Dalle et al., 2020; Fearnley and Amora, 2020; Fernando, 2020; Hadoussa, 2020; Khand and Kalhoro, 2020). Since, females and males differ in their use of technology and satisfaction (Lu and Chiou, 2010; He and Freeman, 2014) as mentioned earlier. Therefore, the study proposes the following two hypotheses;
H1: The positive impact of SQ on SU will be significantly different for female and male student groups.
H2: The positive impact of SQ on US will be significantly different for female and male student groups.
The IQ construct encompasses output characteristics of a system such as web pages and reports. The online learning system should generate accurate, relevant, usable, complete, and understandable information within time to the end-users. These qualities of information determine the satisfaction level of users (Ifinedo, 2014; Mohammadi, 2015; Dalle et al., 2020; Fernando, 2020; Hadoussa, 2020; Khand and Kalhoro, 2020) and their behavioral intention to use (SU) E-learning portals (Mohammadi, 2015; Yakubu and Dasuki, 2018; Dalle et al., 2020; Hadoussa, 2020). As earlier mentioned, the differences exist in their use of technology and satisfaction among females and males (Lu and Chiou, 2010; He and Freeman, 2014). Hence, the following two hypotheses are proposed;
H3: The positive impact of information quality on system use will be significantly different for female and male student groups.
H4: The positive impact of information quality on user satisfaction will be significantly different for female and male student groups.
The ESQ dimension refers to the support users receive from the IS or IT departments. The service quality construct has several characteristics of service quality; however, five distinctive dimensions, namely, reliability, tangibility, responsibility, assurance, and empathy, are highly recognized as essential (Petter et al., 2008). Like previous studies, it is theorized that ESQ will influence user satisfaction (Freeze et al., 2010; Ramayaha et al., 2010; Mohammadi, 2015; Yakubu and Dasuki, 2018; Dalle et al., 2020; Fernando, 2020; Khand and Kalhoro, 2020) and behavioral intentions to use system (Ramayaha et al., 2010; Mohammadi, 2015; Dalle et al., 2020; Fernando, 2020; Hadoussa, 2020; Khand and Kalhoro, 2020) given the differences between females and males on the use of technology and satisfaction (Lu and Chiou, 2010; He and Freeman, 2014). Therefore:
H5: The positive impact of E-service quality on system use will be significantly different for female and male student groups.
H6: The positive impact of E-service quality on user satisfaction will be significantly different for female and male student groups.
The SU dimension concerns with the assessment of means in which the IS is utilized. Several researches have measured this dimension by assessing it either frequency of use or the actual usage (Urbach et al., 2010). Similar to practices in other part of the world, a collaborative portal is in place in universities in Afghanistan. The university management expects and mandate their students and staffs to use E-learning systems (ELP). This study examines the SU dimension from the perspective of accomplishing and completing work (Selim, 2007; Wang and Wang, 2009). In the up-dated D&M-IS success model, all the quality constructs (IQ, SQ, ESQ) were believed to affect the SU dimension. In the context of E-Learning portal (ELP) success, several studies have found support for the relationship between SU and the ELP success (i.e., actual usage or net benefits of E-learning systems) (Lin, 2007; Mohammadi, 2015; Yakubu and Dasuki, 2018; Khand and Kalhoro, 2020; Hadoussa, 2020). Since gender is an important variable that significantly influence the use of system and satisfaction with the e-learning system (or ELP success) (Lu and Chiou, 2010). Thus:
H7: The positive impact of the system used on the e-learning portal success will be significantly different for female and male student groups.
The US dimension measures the overall satisfaction with e-learning system (Petter and McLean, 2009) and is considered important to IS success. US characteristics captured in this study include dependability, relevance, usefulness, and effectiveness (DeLone and McLean, 2003; Pillay and Maharaj, 2014). US can be measured based on the people’s feelings who use the IS and the degree to which they believe that it their informational needs, in the learning process, have been met (Freeze et al., 2010; Harandi, 2015).
US of e-learning systems has been shown to influence actual usage or net benefits (i.e., ELP success) (Mohammadi, 2015; Yakubu and Dasuki, 2018; Fernando, 2020; Khand and Kalhoro, 2020). Further, gender being a critical element that significantly effect the use of system and satisfaction with the e-learning system (or ELP success) (Lu and Chiou, 2010). Therefore, the following hypothesis is proposed:
H8: The positive impact of user satisfaction on the e-learning portal success will be significantly different for female and male student groups.
In conclusion, the modified D&M model has been instrumental in evaluating the success of various technological applications. This model has been used to utilize to measure the success of e-government applications, e-banking, e-procurement, and several other online applications (Hsu et al., 2015; Aparicio et al., 2017; Cidral et al., 2018). Based on the D&M model, this study measures the success level of online learning portals (ELP) by comparing the satisfaction levels of female and male users and their ability to utilize an e-learning system.
As shown in Figure 2, this study measures ELP success based on SQ, IQ, ESQ, SU, and US. The conceptual frame work shows the relationship of SQ, IQ, and ESQ with SU and US and, further the relationship between SU, US, and ELP success.
This study used a quantitative approach for data collection, employing a cross-sectional survey, using a convenience sample with a Google form. The study included students as sample from different Kabul-based universities who were enrolled in their online courses both at graduate and under graduate level. To approach students for the data collection, the survey link was shared with the students through the WhatsApp group of their respective lecturers. Being in teaching profession and having a strong networking with lecturers of other universities, we contacted these lectures via telephone and emails and requested them to help us in data collection. These lecturers were briefed about the survey so that they could further provide briefing to their respective students accordingly. Since the survey included question with mandatory responses for each of the questions, thus there were no missing values in the data set.
Table 1 summarizes students enrolled either in a bachelor program or master program who participated in the online survey conducted from July to August 2020. Table 1 presents the demographic characteristics of the respondents.
Table 1 shows that most students were enrolled in the master program (i.e., 63.8%), and 36.2% were enrolled in the bachelor program. Furthermore, males and females accounted for 66.9 and 33.1%, respectively, of the total participants. Most respondents were aged between 21 and 30, representing 45.3% of the sample. Most respondents, i.e., 179 (representing 70.5%), had less than a year of experience using an E-learning portal.
Previous studies were used to develop the survey instrument for this study. Based on the objectives, the questionnaire was adopted/adapted and reworded. In total, this study used 29 items to measure SQ, IQ, ESQ, SU, US, and ELP success constructs. The SQ, IQ, ESQ, SU, and US constructs were assessed using 5, 5, 8, 3, and 4 items respectively. All items used a 5-point Likert scale with scores ranging from 1 to 5 from “strongly disagree” to “strongly agree.” The questionnaire items for these constructs (i.e., ESQ, IQ, SQ, SU, and US) were adopted from the scales that McGill et al. (2003) and Rai et al. (2002) developed.
The ELP success construct was measured using a four-item scale. Items for this construct were adopted from the scale that Freeze et al. (2010) developed. All items of ELP success used a 5-point Likert scale with scores ranging from 1 = “Poor”, 2 = “Fair”, 3 = “Satisfactory”, 4 = “Very good”, and 5 = “Excellent”.
As the study used a self-reported online survey and the data are collected using a single source in a specific timeline which is concerned with the common method bias, hence it was necessary to test CMB (Spector, 2006; Tehseen et al., 2017). To ensure there was no common method bias, the present study investigated the full collinearity approach as suggested by (Kock, 2015). According to this method, all the variables have to be regressed against a common variable, and if the VIF ≤5, then there is no bias from the single-source data. Results provided in Table 2 indicate the single-source bias was not a serious issue with the data.
This study performed normality test before selecting the appropriate technique for the data analysis. To do that Cain et al. (2017) and Hair et al. (2017), recommended the multivariate kurtosis and skewness of the data were examined through the WebPower statistical power analysis (WebPower, 2020). Mentioned in Table 3, the results indicated that Mardia’s multivariate kurtosis (ß = 75.3678, p < 0.001) and Mardia’s multivariate skewness (ß = 8.3485, p < 0.001) showed that multivariate data distribution was not normal (Cain et al., 2017).
Therefore, the study used the SmartPLS (version 3.2.8) software (see Ringle et al., 2015), a non-parametric analysis that uses partial least squares to analyze a research model, which is also used when the sample size is small (Hair et al., 2017). Furthermore, a variance-based structural equation modeling (PLS-SEM) provides more reliable construct scores to use in further analysis as compared to the covariance-based structural equation modelling (PLS-CB) (Henseler et al., 2015). Furthermore, PLS-SEM is considered perfectly fit in case of explanatory research and complex model (Henseler et al., 2018; Ringle et al., 2018). Provided, this research is explanatory in nature and model is complex [Multigroup analysis (MGA)] so PLS-SEM analysis has been applied herein.
While applying PLS-SEM (MGA), first, the permutation algorithm examined if the indicators associated with each construct were invariant between females and males. Then, the measurement invariance of composite models (MICOM) examined measurement model invariance, which is also referred to as measurement equivalence (Hair et al., 2019). The MICOM procedure comprises three steps—examining 1) configural invariance, 2) compositional invariance, and 3) equality of composite mean values and variance. Next, multi-group analysis (MGA; permutation algorithm) via the SmartPLS tool with partial least squares structural equation modeling (PLS-SEM) compared the two groups (female and male) and tested whether pre-defined data groups had statistically significant differences in gender-group parameter estimates. Lastly, PLS-SEM tested the overall model. Smart PLS evaluates measurement and structural models (Hair et al., 2016).
Cronbach’s alpha, average variance (AVE), composite reliability, and factor loadings are typically used to ascertain the reliability and convergent validity (Henseler et al., 2016; Hair et al., 2017). As Table 4 shows, Cronbach’s alpha values for all constructs in all three models (i.e., full model, female model, and male model) ranged between 0.70 and 0.90 which is considered satisfactory (Hair et al., 2017). Also, the composite reliability of all constructs in complete model, female group model, male group model ranged between 0.870 and 0.990, which exceeds 0.6, the threshold of acceptability (Hair et al., 2017). In addition, all loadings of indicators were more than 0.73, which exceeds 0.70, the threshold for acceptability (Hair et al., 2017). Nonetheless, weaker outer loadings between 0.40 and 0.70 can be retained if they can explain 50% of AVE (Hair et al., 2017). The average variance extracted (AVE) of all constructs in the complete model, female group model, male group model was more than 0.60, which exceeds 0.5, the threshold for convergent validity (Henseler et al., 2016). Thus, convergent validity was ensured in this study. See also, Figures 3–5 for the full measurement model, the male measurement model, and the female measurement model respectively.
The heterotrait-monotrait (HTMT) ratio was used as recommended to establish discriminant validity because the HTMT ratio is viewed as superior to the Fornell-Larcker criterion (Henseler et al., 2015; Voorhees et al., 2016; Hair et al., 2019). HTMT offers two ways to assess discriminant validity, which are a criterion test or as a statistical test. In a criterion test, a discriminant validity problem is present when the HTMT value is greater than the HTMT0.85 value of 0.85 (Kline, 2012) or HTMT0.90 value of 0.90 (Gold et al., 2001). The second criteria test the null hypothesis (H0: HTMT ⩾1) against the alternate hypothesis (H0: HTMT <1), and if the confidence interval contains the value of 1 (i.e., H0 holds), then this indicates a lack of discriminant validity (Henseler et al., 2015). As shown in Tables 5–7 all the values were below the threshold level, HTMT.90 (Gold et al., 2001. The HTMT inference shows that the confidence interval did not have a value of 1 on any of the constructs, thus indicating discriminant validity. Therefore, discriminant validity was established.
The study applied an invariance test to measure two sets of university students (female and male). Performing an invariance test before conducting a multi-group analysis is vital. The purpose of an invariance test is to determine “whether, under different conditions of observing and studying phenomena, measurement models yield measures of the same attribute” (Henseler et al., 2015, p. 117). Afterward, a study follows three steps: configural invariance, compositional invariance, and equality of composite mean values and variances to examine measurement invariance (Henseler et al., 2016).
First, because the measurement models have the same number of constructs, identical items, identical data treatment, and identical algorithm settings, configural invariance is established for both data groups (see Tables 5–7). Second, the permutation test measured compositional invariance to assure the composite scores are the same between the groups. Last, the study assesses the equality of composite variances and means values of groups. The results of the difference between the composites’ mean and variance ratio must fall within the 95% confidence interval.
Table 6 reveals that each of the composite constructs has non-significant differences for the composite mean and variances ratio. Moreover, Table 8 shows the full measurement invariance of females and males. Therefore, the various model estimations of female and male groups students were not distinct in terms of either the content or usage of the ELP.
This study applied PLS-MGA to calculate the differences using the Welch-Satterthwaite test on female and male groups (Sarstedt et al., 2011). Furthermore, Table 9 shows the path coefficient and difference of the composite’s means. The results show that a difference exists for both males and females as indicated in the path differences; however, these differences were not statistically significant for male and female data sets. Hence, as theorized earlier that the differences exist between female and male group of students in validating the relationship among the constructs (i.e., SQ, IQ, ESQ, SU, US, and ELP) used in the present study. These findings are not in agreement with previous studies which have shown differences between gender (females and males) in the use of technology and satisfaction and further with ELP success (Lu and Chiou, 2010; Lu and Chiou, 2010; He and Freeman, 2014; Ramírez-Correa et al., 2015).
Concerning the hypotheses testing on the male group, as shown in Table 10, five variables, directly and indirectly, significantly supported ELP success. Similar to previous studies, this study found a significant positive influence of ESQ on the US (Freeze et al., 2010; Ramayaha et al., 2010; Mohammadi, 2015; Yakubu and Dasuki, 2018; Dalle et al., 2020; Fernando, 2020; Khand and Kalhoro, 2020) and SU (Ramayaha et al., 2010; Mohammadi, 2015; Dalle et al., 2020; Fernando, 2020; Hadoussa, 2020; Khand and Kalhoro, 2020) for both female and male group students. This suggests that HEIs should attempt to motivate people to use the IS by implementing them in a way that will lead to the achievement of desirable outcomes, such as the processing of information and storage (Hackney et al., 2007; D’Ambra and Rice, 2001). Additionally, aside from flexibility, the IS should also be functional and easy to use. When IS is not accurate, safe, and reliable, users will not perceive their benefits and may stop relying on them to attain the desirable results.
Besides ESQ and in agreement with previous studies, this study also found that SQ significantly affected the US (Ifinedo, 2014; Mohammadi, 2015; Dalle et al., 2020; Fearnley and Amora, 2020; Hadoussa, 2020) for both female and male group students. This finding indicates that the desirable characteristics of information systems, i.e., flexibility, reliability, easy to use, intuitive, and sophisticated lead to US which is also critical for any online learning portal’s success.
In alignment with previous studies, another finding of this study was the SU dimension and US dimension were found to influence ELP success (Lin, 2007; Mohammadi, 2015; Yakubu and Dasuki, 2018; Khand and Kalhoro, 2020; Hadoussa, 2020) for both female and male group students. This finding implies that student, faculty, and staff perceive that the current information system (ELP) is effective in terms usage and of much helpful in the learning process and fulfilling their informational needs.
Thus, the E-learning portal’s usage and success are of equal importance for the female and male students’ group in higher education institutions of Afghanistan.
The extant literature on technology adoption phenomena reveals that most previous studies mainly concentrated on either the individual level (customer), the organizational level (management) or both (Al-Kofahi et al., 2020). However, studies related to technology adoption, such as ELP success among females and student groups, are infrequently conducted, especially in Afghanistan. This is a pioneering study to test theoretical and empirical relationships between female and male student groups in higher education settings regarding e-portal implementation. The study considered the D&M model because tangible information factors may help universities in Afghanistan to strengthen their services.
This study mainly found that ESQ and SQ both have a significant impact on US. Also, ESQ is an essential element that encourages the frequency of system usage. In this respect, it is clear that the study provides significant information that might be used as the basis for assessing an IS (such as ELP) and its use in the higher education sector. Based on the findings presented here, researchers can conduct further investigations to determine the positive and significant relationships among the various elements of the DeLone and McLean framework (Cho et al., 2015; Tilahun and Fritz, 2015). Also, studies can be undertaken to investigate how the system’s nature and the implementation areas can affect the project’s success. The aftermath of these studies might be utilized as the foundation for forecasting how users will respond to the implementation of an IS and the degree to which they will use it in their everyday lives.
This study’s noteworthy contribution is the behavior of the model, which changes when the model is tested for two separate groups of samples (i.e., female and male). The D&M model yields different results when tested on two sets of data simultaneously. That is to say, the theoretical relationship between the variables changes when the model was examined for two different subgroups. This, as a result, confirms the effectiveness of the SU and US with the ELP for both female and male students of HEIs of Afghanistan.
This study offers several implications concerning E-learning Portal technology not only for institutions of higher educations in Afghanistan but also for HEIs in other countries as well. In fact, several researchers have highlighted the validation of IS success model in the high-income countries and a lack of research low-income countries (Borena and Negash, 2016; Al-Kofahi et al., 2020), thereby representing a gap for scholars from the low-income nations such as Afghanistan (Al-Kofahi et al., 2020). Thus, the Afghanistan’s higher education sector provides a suitable context for conducting this study to assess if the IS success model works the same way in a country that has medium or low level of adoption of IS with a low level of technological advancement. The results provided in this study show that the implemented information system (ELP) had been successful and provided users with a way through which they might undertake educational activities in the institution.
This study will be useful for the Government of Afghanistan and the policymakers in the higher education setting such as the MoHE of Afghanistan and the top management in the HEIs in developing the policies and programs related to the ELP success in the country. If the aforementioned stake holders understand the nature of the IS (such as ELP) and the way users evaluate them, they will be in a better position in making sufficient allocations of resources to improve the SQ and ESQ (DeLone and McLean, 2016). In addition, this will make the stakeholders capable of analyzing the internal and external factors that will determine how users respond to IS projects. In the higher education sector, head of the faculty department should be motivated to evaluate and scrutinize the reliability of IS and forecast their potential effect on the basis of different tools such as the D&M-framework (D’Ambra and Rice, 2001; Burton-Jones and Straub, 2003; Burton-Jones and Straub, 2006). The dimensions of IS success might help them develop plans that are required during the execution of the IS projects. Moreover, they will realize and understand the changes that need to be made to ensure that the projects are a success.
The COVID-19 outbreak, within a short period, has changed the operational habits of organizations around the globe in general, especially the higher education environment. Institutions of higher education are anticipated to offer online courses to their students in the future. This study offers an optimistic view about ELP’s value to improve student access and improve the quality of teaching and learning in the higher education context of Afghanistan. The IS can also assist HEIs to be innovative and responsive to the changing demands of students and the changing educational environments. COVID-19 will have a long-term impact on the higher education sector. If the pandemic dwells longer, it might result in a permanent change from face-to-face education to an online education system.
This study provides suggestions to the HEIs such as making the ELP accessible 24/7, provision of error-free information, the robustness of the server, quality in contents, updated information, quality of information, user-friendly designs of the students’ portal, well-organized data, training module materials associated with e-learning portal use for new users, and time to time feedback from the users will enhance the acceptability and durability of the ELP.
This study was designed to compare female and male student groups about the ELP success in the HEIs. It highlights the perspectives of female and male students on the adaptability of ELP services.
The current study found that ESQ impacts US and SU and further the effect of SQ on US, which, if of high quality, will eventually lead to the success of the ELP. The use of an e-learning system can not only help in decreasing the cost of education but permit the expansion of education beyond national borders as well. In the next 5–10 years, education may become borderless. Therefore, the study provides top management of the HEIs with suggestions for developing an understanding of ESQ, IQ, SQ, SU, and US related to ELP success.
This study underscores the differences, not significant though, between female and male students in the successful use of ELP in Afghanistan. A small sample size in overall and especially of the female group students could be one major reason for insignificant results regarding the differences of female and male group students on ELP success. The less participation of females in the survey has been due the overall low participation of females in the higher education sector in Afghanistan (Katawazai, 2021; Noori, 2021) and, thus, is one major limitation of this study. Although, gender has been found to influence the IS success in the previous studies (Lu and Chiou, 2010). Future studies, however, may consider a larger sample size to investigate the impact of gender on the ELP success. In addition, this study mainly focused on the students’ perceptions of the ELP success neglecting the instructors’ and institutional perspectives, which could be interesting to discover for a comparative purpose. Future research can be carried out by comparing the students’ opinions, opinion of the academic and administrative staff, individually as well collectively. This will surely be beneficial to scrutinize and understand probable issues from the use of ELP in the higher education sector.
Moreover, this study only used the six dimensions of the updated D&M model as highlighted in the previous literature (DeLone and McLean, 2003). Future researchers, however, may consider some other variables like technology infrastructure support, technical expertise, attitude toward ELP, user characteristics, and motivation. Also, this study used cross sectional research design which limit the generalizability of results. Therefore, future studies can employ a longitudinal research design to test the relationship empirically between theoretical constructs for better generalizability in the context of HEIs.
The original contributions presented in the study are included in the article/Supplementary Material, further inquiries can be directed to the corresponding author.
The studies involving human participants were reviewed and approved by Kardan University Ethics Committe. Written informed consent for participation was not required for this study in accordance with the national legislation and the institutional requirements.
MS has started the initiation of the manuscript and take the main responsibilities, He wrote the Abstract, Introduction and the discussion Part. MN, did the Methodology section. HG, give the idea and took the responsibility of literature review, and technical help. TSM, has technically contributed in managing the manuscript and particularly in addressing the manuscript. KUK, helped in the manuscript in statistical analysis, and re-interpret the results according to reviewer comments and suggestion. His active participation and wide experience in addressing the comments really helped in the manuscript acceptance.
HG the corresponding author will pay the fees for Publication.
The authors declare that the research was conducted in the absence of any commercial or financial relationships that could be construed as a potential conflict of interest.
All claims expressed in this article are solely those of the authors and do not necessarily represent those of their affiliated organizations, or those of the publisher, the editors and the reviewers. Any product that may be evaluated in this article, or claim that may be made by its manufacturer, is not guaranteed or endorsed by the publisher.
Al-Emran, M., and Teo, T. (2020). Do knowledge Acquisition and Knowledge Sharing Really Affect E-Learning Adoption? an Empirical Study. Educ. Inf. Technol. 25 (3), 1983–1998. doi:10.1007/s10639-019-10062-w
Al-Kofahi, M. K., Hassan, H., Mohamad, R., Intan, T. P., and Com, M. (2020). Information Systems success Model: A Review of Literature. Int. J. Innovation, Creativity Change 12.
Al-Maroof, R. A., and Al-Emran, M. (2021). Research Trends in Flipped Classroom: a Systematic Review. Recent Adv. Intell. Syst. Smart Appl., 253–275. doi:10.1007/978-3-030-47411-9_15
Al-Nuaimi, M. N., and Al-Emran, M. (2021). Learning Management Systems and Technology Acceptance Models: A Systematic Review. Edu. Inf. Tech., 1–35.
Al-Nuaimi, M. N., Al-Kabi, M. N., and Al-Emran, M. (2021). Digitizing Learning during the Outbreak of COVID-19 Pandemic: Lessons Learned from the Most Infected Countries. Emerging Tech. During Era COVID-19 Pandemic 348, 291–303. doi:10.1007/978-3-030-67716-9_18
Aljazeera English (2020). Afghan Schools, Universities to Remain Closed until September. June 8. Available at: https://www.aljazeera.com/news/2020/6/8/afghan-schools-universities-to-remain-closed-until-september.
Aparicio, M., Bacao, F., and Oliveira, T. (2017). Grit in the Path to E-Learning success. Comput. Hum. Behav. 66, 388–399. doi:10.1016/j.chb.2016.10.009
Arpaci, I., Al-Emran, M., and Al-Sharafi, M. A. (2020). The Impact of Knowledge Management Practices on the Acceptance of Massive Open Online Courses (MOOCs) by Engineering Students: A Cross-Cultural Comparison. Telematics Inform. 54, 101468. doi:10.1016/j.tele.2020.101468
Borena, B., and Negash, S. (2016). IT Infrastructure Role in the success of a Banking System: The Case of Limited Broadband Access. Inf. Tech. Dev. 22 (2), 265–278. doi:10.1080/02681102.2014.979392
Burton-Jones, A., and Straub, D. (2003). Reconceptualizing System Usage. Computer Information Systems Department Working Paper No. CIS 03-01, Georgia State University.
Burton-Jones, A., and Straub, D. W. (2006). Reconceptualizing System Usage: An Approach and Empirical Test. Information systems research 17 (3), 228–246.
Cain, M. K., Zhang, Z., and Yuan, K. H. (2017). Univariate and Multivariate Skewness and Kurtosis for Measuring Nonnormality: Prevalence, Influence and Estimation. Behav. Res. Methods 49 (5), 1716–1735. doi:10.3758/s13428-016-0814-1
Calisir, F., Altin Gumussoy, C., Bayraktaroglu, A. E., and Karaali, D. (2014). Predicting the Intention to Use a Web-Based Learning System: Perceived Content Quality, Anxiety, Perceived System Quality, Image, and the Technology Acceptance Model. Hum. Factors Man. 24 (5), 515–531. doi:10.1002/hfm.20548
Carey, K. (2020). Is Everybody Ready for the Big Migration to Online College? Actually, No. The New York Times, March 13. Available at: https://www.nytimes.com/2020/03/13/upshot/coronavirus-online-college-classes-unprepared.html.
Chaplin, S. (2020). COVID ‐19: a Brief History and Treatments in Development. Prescriber 31 (5), 23–28. doi:10.1002/psb.1843
Cho, K. W., Bae, S. K., Ryu, J. H., Kim, K. N., An, C. H., and Chae, Y. M. (2015). Performance Evaluation of Public Hospital Information Systems by the Information System success Model. Healthc. Inform. Res. 21 (1), 43–48. doi:10.4258/hir.2015.21.1.43
Chu, R. J., and Chu, A. Z. (2010). Multi-level Analysis of Peer Support, Internet Self-Efficacy and E-Learning Outcomes - the Contextual Effects of Collectivism and Group Potency. Comput. Edu. 55 (1), 145–154. doi:10.1016/j.compedu.2009.12.011
Cidral, W. A., Oliveira, T., Di Felice, M., and Aparicio, M. (2018). E-learning success Determinants: Brazilian Empirical Study. Comput. Edu. 122 (1), 273–290. doi:10.1016/j.compedu.2017.12.001
Cojocariu, V.-M., Lazar, I., Nedeff, V., and Lazar, G. (2014). SWOT Anlysis of E-Learning Educational Services from the Perspective of Their Beneficiaries. Proced. - Soc. Behav. Sci. 116, 1999–2003. doi:10.1016/j.sbspro.2014.01.510
Cuadrado-García, M., Ruiz-Molina, M. E., and Montoro-Pons, J. D. (2010). Are There Gender Differences in E-Learning Use and Assessment? Evidence from an Interuniversity Online Project in Europe. Procedia-Social Behav. Sci. 2 (2), 367–371.
Dalle, J., Hastuti, D., Mahmud, M., Prasetia, I., and Baharuddin, B. (2020). DeLone and McLean Model Evaluation of Information System success: A Case Study of Master Program of Civil Engineering Universitas Lambung Mangkurat. Int. J. Adv. Sci. Tech. 29 (4), 1909–1919.
D’Ambra, J., and Rice, R. E. (2001). Emerging Factors in user Evaluation of the World Wide Web. Information and management 38 (6), 373–384.
DeLone, W. H., and McLean, E. R. (2016). Information Systems success Measurement. FNT Inf. Syst. 2 (1), 1–116. doi:10.1561/2900000005
DeLone, W. H., and McLean, E. R. (2003). The DeLone and McLean Model of Information System success: A Ten-Year Update. J. Manage. Inf. Syst. 19 (4), 9–30. doi:10.1080/07421222.2003.11045748
Dignan, L. (2020). Online Learning Gets its Moment Due to COVID-19 Pandemic: Here’s How Education Will Change, March 22. [Online]. ZDNet. Available at: https://www.zdnet.com/article/online-learning-gets-its-moment-due-to-covid-19-pandemic-heres-how-education-will-change/.
Dikovic, M., Etinger, D., and Golja, D. (2020). “E-learning as a Tool for Competencies Development: university Students ‘point of View,” in Proceedings of INTED conference, 1389–1398. March.
European Data Portal. (2020). Education during COVID-19; Moving towards E-Learning, June 22. Available at: https://www.europeandataportal.eu/en/impact-studies/covid-19/education-during-covid-19-moving-towards-e-learning.
Fearnley, M. R., and Amora, J. T. (2020). Learning Management System Adoption in Higher Education Using the Extended Technology Acceptance Model. ije 8 (2), 89–106. doi:10.22492/ije.8.2.05
Fernando, E. (2020). “Factors Influence the success of E-Learning Systems for Distance Learning at the university,” in 2020 International Conference on Information Management and Technology (ICIMTech) (IEEE), 294–299. August. doi:10.1109/icimtech50083.2020.9211163
Freeze, R. D., Alshare, K. A., Lane, P. L., and Wen, H. J. (2010). IS success Model in E-Learning Context Based on Students’ Perceptions. J. Inf. Syst. Edu. 21 (2), 173–184. Retrieved from: https://www.researchgate.net/publication/228365490_IS_Success_Model_in_E-Learning_Context_Based_on_Students'_Perceptions.
Gaitan, J. A., Cataluna, F. J. R., and Correa, P. E. R. (2010). “Gender Influence in Perception and Adoption of E-Learning Platforms,” in DNCOCO'10: Proceedings Of The 9th WSEAS International Conference On Data Networks, Communications, Computers, 30–35. Retrieved from: http://www.wseas.us/e-library/conferences/2010/Faro/DNCOCO/DNCOCO-04.pdf.
Garland, D., and Martin, B. N. (2005). Do gender and Learning Style Play a Role in How Online Courses Should Be Designed. J. interactive Online Learn. 4 (2), 67–81.
Gold, A. H., Malhotra, A., and Segars, A. H. (2001). Knowledge Management: an Organizational Capabilities Perspective. J. Manage. Inf. Syst. 18 (1), 185–214. doi:10.1080/07421222.2001.11045669
Hackney, R., Jones, S., and Lösch, A. (2007). Towards an E-Government Efficiency Agenda: the Impact of Information and Communication Behaviour on E-Reverse Auctions in Public Sector Procurement. European Journal of Information Systems 16 (2), 178–191.
Hadoussa, S. (2020). Evaluation of E-Learning System on Higher Education Institutions in KSA: a Survey at Saudi Electronic University. Ijtel 12 (2), 180–199. doi:10.1504/ijtel.2020.106285
Hair, J. F., Sarstedt, M., Ringle, C. M., and Gudergan, S. P. (2017). Advanced Issues in Partial Least Squares Structural Equation Modeling. SAGE Publications.
Hair, J. F., Risher, J. J., Sarstedt, M., and Ringle, C. M. (2019). When to Use and How to Report the Results of PLS-SEM. Ebr 31 (1), 2–24. doi:10.1108/EBR-11-2018-0203
Hair, J., Sarstedt, M., Hopkins, L., and Kuppelwieser, G. V. (2016). Partial Least Squares Structural Equation Modeling (PLS-SEM) an Emerging Tool in Business Research. Eur. Business Rev. 26 (2), 106–121. doi:10.1108/EBR-10.2013-0128
Harandi, S. R. (2015). Effects of E-Learning on Students' Motivation. Proced. - Soc. Behav. Sci. 181, 423–430. doi:10.1016/j.sbspro.2015.04.905
He, J., and Freeman, L. A. (2014). Are Men More Technology-Oriented Than Women? the Role of Gender on the Development of General Computer Self-Efficacy of College Students. J. Inf. Syst. Edu. 21 (2), 203–211. Retrieved from: https://www.researchgate.net/publication/220890820_Are_Men_More_TechnologyOriented_Than_Women_The_Role_of_Gender_on_the_Development_of_General_Comuter_Self-Efficacy_of_College_Students.
Hellstén, S. M., and Markova, M. (2006). The DeLone and McLean Model of Information Systems Success – Original and Updated Models. Available at: http://www.cs.tut.fi/∼ihtesem/s2006/teoriat/esitykset/IS_success_model_Markova&Hellsten_311006.pdf.
Henseler, J., Müller, T., and Schuberth, F. (2018). New Guidelines for the Use of PLS Path Modeling in Hospitality, Travel and Tourism Research. Application of Partial Least Squares: Structural Equation Modeling (PLS-SEM) in Tourism And Hospitality Research, 17e33.
Henseler, J., Ringle, C. M., and Sarstedt, M. (2015). A New Criterion for Assessing Discriminant Validity in Variance-Based Structural Equation Modeling. J. Acad. Mark. Sci. 43 (1), 115–135. doi:10.1007/s11747-014-0403-8
Henseler, J., Ringle, C. M., and Sarstedt, M. (2016). Testing Measurement Invariance of Composites Using Partial Least Squares. Int. Marketing Rev. 33, 405–431. doi:10.1108/IMR-09-2014-0304
Hrastinski, S. (2008). Asynchronous and Synchronous E-Learning. A Study of Asynchronous and Synchronous E-Learning Methods Discovered that Each Supports Different Purposes. Educause Quarterly. [Online]. Available at: https://er.educause.edu/articles/2008/11/asynchronous-and-synchronous-elearning.
Hsu, P.-F., Yen, H. R., and Chung, J.-C. (2015). Assessing ERP post-implementation success at the Individual Level: Revisiting the Role of Service Quality. Inf. Manage. 52 (8), 925–942. doi:10.1016/j.im.2015.06.009
Huang, C., Wang, Y., Li, X., Ren, L., Zhao, J., Hu, Y., et al. (2020). Clinical Features of Patients Infected with 2019 Novel Coronavirus in Wuhan, China. Lancet 395 (10223), 497–506. doi:10.1016/2FS0140-6736(20)30183-5doi:10.1016/S0140-6736(20)30183-5
Hung, M.-L., Chou, C., Chen, C.-H., and Own, Z.-Y. (2010). Learner Readiness for Online Learning: Scale Development and Student Perceptions. Comput. Edu. 55 (3), 1080–1090. doi:10.1016/j.compedu.2010.05.004
Ifinedo, P. (2014). Information Systems Security Policy Compliance: An Empirical Study of the Effects of Socialisation, Influence, and Cognition. Inf. Manage. 51 (1), 69–79. doi:10.1016/j.im.2013.10.001
Indira, D., and Sakshi, A. (2017). Online Learning. International Education and Research Journal 3 (8), 32–34.
Katawazai, R. (2021). Implementing Outcome-Based Education and Student-Centered Learning in Afghan Public Universities: the Current Practices and Challenges. Heliyon 7 (5), e07076. doi:10.1016/j.heliyon.2021.e07076
Kay, R. H., and Knaack, L. (2008). A Formative Analysis of Individual Differences in the Effectiveness of Learning Objects in Secondary School. Comput. Edu. 51 (3), 1304–1320. doi:10.1016/j.compedu.2008.01.001
Khand, Z. H., and Kalhoro, M. R. (2020). Testing and Validating DeLone and MacLean IS Model: ERP System Success in Higher Education Institutions of Pakistan. Eng. Technol. Appl. Sci. Res. 10 (5), 6242–6248. doi:10.48084/etasr.3762
Kline, E., Wilson, C., Ereshefsky, S., Tsuji, T., Schiffman, J., Pitts, S., et al. (2012). Convergent and Discriminant Validity of Attenuated Psychosis Screening Tools. Schizophr Res. 134 (1), 49–53. doi:10.1016/j.schres.2011.10.001
Kock, N. (2015). Common Method Bias in PLS-SEM. Int. J. e-Collaboration (Ijec) 11 (4), 1–10. doi:10.4018/ijec.2015100101
Kumar Basak, S., Wotto, M., Bélanger, P., E-learning, , M-learning, , and D-learning, (2018). E-learning, M-Learning and D-Learning: Conceptual Definition and Comparative Analysis. E-learning and digital media 15 (4), 191–216. doi:10.1177/2042753018785180
Lin, H. F. (2007). Measuring Online Learning Systems Success: Applying the Updated DeLone and McLean Model. Cyberpsychology and behavior 10 (6), 817–820.
Lu, H.-P., and Chiou, M.-J. (2010). The Impact of Individual Differences on E-Learning System Satisfaction: A Contingency Approach. Br. J. Educ. Tech. 41 (2), 307–323. doi:10.1111/j.1467-8535.2009.00937.x
Margaryan, A., Bianco, M., and Littlejohn, A. (2015). Instructional Quality of Massive Open Online Courses (MOOCs). Computers and Education 80, 77–83.
Mason, R. O. (1978). Measuring Information Output: A Communication Systems Approach. Information Management 1 (5), 219–234.
McBrien, J. L., Cheng, R., and Jones, P. (2009). Virtual Spaces: Employing a Synchronous Online Classroom to Facilitate Student Engagement in Online Learning. Int. Rev. Res. Open Distributed Learn. 10 (3), 1–17. doi:10.19173/irrodl.v10i3.605
McGill, T., Hobbs, V., and Klobas, J. (2003). User Developed Applications and Information Systems Success. Inf. Resour. Manage. J. 16 (1), 24–45. doi:10.4018/irmj.2003010103
Mohammadi, H. (2015). Investigating Users' Perspectives on E-Learning: An Integration of TAM and IS success Model. Comput. Hum. Behav. 45, 359–374. doi:10.1016/j.chb.2014.07.044
Nguyen, T. D., Nguyen, T. M., and Cao, T. H. (2015). “Information Systems Success: A Literature Review,” in Paper presented at International Conference on Future Data and Security Engineering. Nha Trang, Vietnam. Springer, 242–256. doi:10.1007/978-3-319-26135-5_18
Noori, A. Q. (2021). The Impact of COVID-19 Pandemic on Students' Learning in Higher Education in Afghanistan. Heliyon 7 (10), e08113. doi:10.1016/j.heliyon.2021.e08113
Nugroho, K., Murdowo, S., Ahmadi, F., and Suminar, T. (2020). Mobile Cloud Learning Based on User Acceptance Using DeLone and McLean Model for Higher Education. Int. J. Adv. Comput. Sci. Appl. 11 (1), 171–178. doi:10.14569/ijacsa.2020.0110122
Petter, S., DeLone, W., and McLean, E. R. (2008). Measuring Information Systems success: Models, Dimensions, Measures, and Interrelationships. Eur. J. Inf. Syst. 17 (1), 236–263. doi:10.1057/ejis.2008.15
Petter, S., and McLean, E. R. (2009). A Meta-Analytic Assessment of the DeLone and McLean IS success Model: an Examination of IS success at the Individual Level. Inf. Manage. 46, 159–166. doi:10.1016/j.im.2008.12.006
Pillay, K., and Maharaj, M. S. (2014). Social media and mobile Communications Adoption Patterns of South African Civil Society Organisations. South Afr. J. Inf. Manage. 16 (1), 1–8. doi:10.4102/sajim.v16i1.595
Rai, A., Lang, S. S., and Welker, R. B. (2002). Assessing the Validity of IS Success Models: An Empirical Test and Theoretical Analysis. Information Systems Research 13 (1), 50–69.
Ramayah, T., Ahmad, N. H., and Lo, M. C. (2010). The Role of Quality Factors in Intention to Continue Using an E-Learning System in Malaysia. Procedia-Social and Behavioral Sciences 2 (2), 5422–5426.
Ramírez-Correa, P. E., Arenas-Gaitán, J., and Rondán-Cataluña, F. J. (2015). Gender and Acceptance of E-Learning: a Multi-Group Analysis Based on a Structural Equation Model Among College Students in Chile and Spain. PloS one 10 (10), e0140460.
Rana, N. P., and Dwivedi, Y. K. (2018). An Empirical Examination of Antecedents Determining Students' Usage of Clickers in a Digital Marketing Module. Ijbis 27 (1), 86–104. doi:10.1504/ijbis.2018.088572
Ringle, C. M., Sarstedt, M., Mitchell, R., and Gudergan, S. P. (2018). Partial Least Squares Structural Equation Modeling in HRM Research. Int. J. Hum. Resource Manage. 31, 1617–1643. doi:10.1080/09585192.2017.1416655
Safsouf, Y., Mansouri, K., and Poirier, F. (2020). An Analysis to Understand the Online Learners' Success in Public Higher Education in Morocco. JITE:Research 19, 087–112. doi:10.28945/4518
Sarstedt, M., Henseler, J., and Ringle, C. M. (2011). “Multigroup Analysis in Partial Least Squares (PLS) Path Modeling: Alternative Methods and Empirical Results,” in Measurement and Research Methods in International marketingAdvances in International Marketing. Editors M. Sarstedt, M. Schwaiger, and C.R. Taylor (Emerald Group Publishing Limited), 22, 195–218. doi:10.1108/s1474-7979(2011)0000022012
Selim, H. M. (2007). Critical success Factors for E-Learning Acceptance: Confirmatory Factor Models. Comput. Edu. 49, 396–413.
Selvaraj, C. (2019). Success of E-Learning Systems in Management Education in Chennai City-Using User’s Satisfaction Approach. The Online J. Distance Edu. E-learning 7 (2), 124–132. Retrieved from: https://tojdel.net/journals/tojdel/articles/v07i02/v07i02-07.pdf.
Shannon, C. E., and Weaver, W. (2002). A Mathematical Model of Communication. Urbana, IL; University of Illinois Press 11.
Sharma, V., and Dogra, J. (2012). Study of New Paradigm Shift towards Online Learning and Analysis of E-Management Education. Int. J. Marketing Manage. Res. 3 (1), 1–12. Retrieved from: https://www.researchgate.net/publication/326149112_Study_of_New_Paradigm_Shift_wards_Online_Learning_and_analysis_of_E-Management_Education.
Spector, P. E. (2006). Method Variance in Organizational Research. Organizational Res. Methods 9 (2), 221–232. doi:10.1177/1094428105284955
Tehseen, S., Ramayah, T., and Sajilan, S. (2017). Testing and Controlling for Common Method Variance: A Review of Available Methods. J. Manage. Sci. 4 (2), 146–175. doi:10.20547/jms.2014.1704202
The World Bank. (2020). How Countries Are Using Edtech (Including Online Learning, Radio, Television, Texting) to Support Access to Remote Learning during the COVID-19 Pandemic. Available at: https://www.worldbank.org/en/topic/edutech/brief/how-countries-are-using-edtech-to-support-remote-learning-during-the-covid-19-pandemic.
Tilahun, B., and Fritz, F. (2015). Modeling Antecedents of Electronic Medical Record System Implementation success in Low-Resource Setting Hospitals. BMC Med. Inform. Decis. Mak 15, 61–65. doi:10.1186/s12911-015-0192-0
UNDP Afghanistan. (2020). Afghanistan COVID-19 Impact: Short Term Disruptions and Policy Considerations, June 18. Available at: https://reliefweb.int/report/afghanistan/afghanistan-covid-19-impact-short-term-disruptions-and-policy-considerations.
UNESCO. (2020). Startling Digital Divides in Distance Learning Emerge, April 21. Available at: https://en.unesco.org/news/startling-digital-divides-distance-learning-emerge.
United Nations. (2020). Policy Brief: Education during COVID-19 and beyond. Available at: : https://unsdg.un.org/resources/policy-brief-education-during-covid-19-and-beyond.
Urbach, N., Smolnik, S., and Riempp, G. (2010). “Studying Knowledge Management System success in System Administration,” in 42nd Hawaii International Conference on System Sciences (HICSS), 1–7.
Voorhees, C. M., Brady, M. K., Calantone, R., and Ramirez, E. (2016). Discriminant Validity Testing in Marketing: an Analysis, Causes for Concern, and Proposed Remedies. J. Acad. Mark. Sci. 44 (1), 119–134. doi:10.1007/s11747-015-0455-4
Wang, W.-T., and Wang, C.-C. (2009). An Empirical Study of Instructor Adoption of Web-Based Learning Systems. Comput. Edu. 53, 761–774. doi:10.1016/j.compedu.2009.02.021
Warangadinata, W., Maimunah, I., Dewi, Eva., and Rofiq, Z. (2020). Student’s Response on Learning in the Early COVID-19 Pandemic. TADRIS: J. Edu. Teach. Train. 5 (1). Retrieved from: http://ejournal.radenintan.ac.id/index.php/tadris/article/view/6153.
WebPower (2020). My Analysis. Available at: https://webpower.psychstat.org/login.php.
Widjaja, A. E., Chen, J. V., and Gonchig, B. (2018). Investigating Factors Affecting Central Bank Information Systems Success. Int. J. Tech. Hum. Interaction 14 (4), 43–62. doi:10.4018/ijthi.2018100103
Williams, D., Consalvo, M., Caplan, S., and Yee, N. (20202009). Looking for Gender: Gender Roles and Behaviors Among Online Gamers. J. Commun. 59, 700–725. doi:10.1111/j.1460-2466.2009.01453.x
Yakubu, N., and Dasuki, S. (2018). Assessing eLearning Systems success in Nigeria: An Application of the DeLone and McLean Information Systems success Model. JITE:Research 17, 183–203. doi:10.28945/4077
Keywords: COVID-19, E-learning portal success, gender, higher education institutions, Afghanistan, PLS-MGA
Citation: Shams MS, Niazi MM, Gul H, Mei TS and Khan KU (2022) E-Learning Adoption in Higher Education Institutions During the COVID-19 Pandemic: A Multigroup Analysis. Front. Educ. 6:783087. doi: 10.3389/feduc.2021.783087
Received: 28 September 2021; Accepted: 23 December 2021;
Published: 28 January 2022.
Edited by:
Ibrahim Arpaci, Bandirma Onyedi Eylül University, TurkeyReviewed by:
Mostafa Al-Emran, British University in Dubai, United Arab EmiratesCopyright © 2022 Shams, Niazi, Gul, Mei and Khan. This is an open-access article distributed under the terms of the Creative Commons Attribution License (CC BY). The use, distribution or reproduction in other forums is permitted, provided the original author(s) and the copyright owner(s) are credited and that the original publication in this journal is cited, in accordance with accepted academic practice. No use, distribution or reproduction is permitted which does not comply with these terms.
*Correspondence: Habib Gul, habibgul544@yahoo.com
Disclaimer: All claims expressed in this article are solely those of the authors and do not necessarily represent those of their affiliated organizations, or those of the publisher, the editors and the reviewers. Any product that may be evaluated in this article or claim that may be made by its manufacturer is not guaranteed or endorsed by the publisher.
Research integrity at Frontiers
Learn more about the work of our research integrity team to safeguard the quality of each article we publish.