- 1University of Education, Freiburg, Germany
- 2Chemnitz University of Technology, Chemnitz, Germany
The disfluency effect postulates that intentionally inserted desirable difficulties can have a beneficial effect on learning. Nevertheless, there is an ongoing discussion about the emergence of this effect since studies could not replicate this effect or even found opposite effects. To clarify boundary effects of the disfluency effect and to investigate potential social effects of disfluency operationalized through handwritten material, three studies (N1 = 97; N2 = 102; N3 = 103) were carried out. In all three experiments, instructional texts were manipulated in terms of disfluency (computerized font vs. handwritten font). Learning outcomes and cognitive load were measured in all experiments. Furthermore, metacognitive variables (Experiment 2 and 3) and social presence (Experiment 3) were measured. Results were ambiguous, indicating that element interactivity (complexity or connectedness of information within the learning material) of the learning material is a boundary condition that determines the effects of disfluency. When element interactivity is low, disfluency had a positive effect on learning outcomes and germane processes. When element interactivity increases, disfluency had negative impacts on learning efficiency (Experiment 2 and 3) and extraneous load (Experiment 3). In contrast to common explanations of the disfluency effect, a disfluent font had no metacognitive benefits. Social processes did not influence learning with disfluent material as well.
Introduction
When managing complex and challenging learning tasks in multimedia environments, instructors should be aware that a high cognitive load hampers learning progress (Sweller et al., 2019). In this vein, research on designing appropriate instructional materials and procedures for multimedia learning has gained a lot of attention over the years. The aim is to support the learner to concentrate solely on learning and to eliminate unnecessary burdens (or unnecessary cognitive load) as far as possible. Nevertheless, there is an ongoing research on positive effects of designing learning material in a way that it is intentionally difficult to perceive. One approach is providing texts with a difficult-to-read font. What sounds paradox, however, has been established as the disfluency effect in educational research (Alter et al., 2007). The intentional insertion of disfluency, in this case, a difficult-to-read font can improve memorization skills because of metacognitive processes. This study focuses on investigating the disfluency effect operationalized through a handwritten text font. Disfluency is defined and discussed in the context of desirable difficulty, cognitive load, and social processes. Three experiments outline the role of disfluent materials and causal mechanisms of text design on learning processes.
Literature Review
Disfluency as Desirable Difficulty
The concept of disfluency can be traced back to research from James (1950) as well as Kahneman and Frederick (2002), who stated that human processing consists of two distinct cognitive systems. System one is quick, effortless, associative, and intuitive while system two is slow, effortful, analytic, and deliberate (Kühl and Eitel, 2016). Depending on the perceived task difficulty, one of the two systems is activated (Alter et al., 2007). If the learner perceives the information as easy to process, system one operates (Alter and Oppenheimer, 2009). Accordingly, system two will be stimulated when the information processing is perceived as difficult and requires deeper analytic processing. In this case, the learner invests more mental effort (Eitel et al., 2014). Alter et al. (2007) have shown that experienced difficulty motivated learners to process tasks more analytically. In this vein, intentionally inserted desirable difficulties (e.g., an illegible learning material) can have a beneficial effect on learning (Pashler et al., 2007; Bjork, 2013). When learners are facing a challenging design of the learning material, they invest more mental effort while processing the information more deeply (Strukelj et al., 2016). As difficult perceived tasks engage the learner to activate more elaboration strategies (Alter et al., 2007; Xie et al., 2018).
Adding difficulties to the learning material is also possible by manipulating the legibility of the text-font presented (e.g., Alter and Oppenheimer, 2009; Beege et al., 2021). It is assumed that “making text slightly harder-to-read fosters retention and understanding” (Eitel and Kühl, 2016, p. 108). When learners are confronted with hard-to-read learning materials, such difficulties motivate to process the information more deeply than it would be the case in easy-to-read learning materials (Xie et al., 2018). “With disfluent learning material learners perceive the task as more difficult and metacognitively regulate their learning approach by activating system two” (Seufert et al., 2017, p. 222). The cognitive task of encoding a text, therefore, relates to a metacognitive experience. Thus, metacognitive activities should be taken into account. Three main types of judgments are measured when discussing metacognitive activities in learning contexts (Nelson and Narens, 1990): ease of learning (EOL) judgments are made at the beginning of the learning period, after seeing the material for the first time which affects the allocation of study-time (Son and Kornell, 2008). Ease of learning judgments are particularly affected by the text font since no information about the complexity of the learning content is available at the time the judgment is made. Judgments of learning (JOL) are made after learning from the text and predict future memory performance (Dunlosky and Metcalfe, 2009). Finally, retrospective confidence (RC) assesses the confidence of the performance in a learning test (Dinsmore and Parkinson, 2013). These variables are used to calculate metacognitive accuracy scores to determine how accurate learners assume their performance in relation to their abilities and how learners adapt their strategies during learning (Pieger et al., 2016). Pieger and colleagues pointed out that disfluency enhanced absolute metacognitive accuracy, since more analytic metacognitive processes were initiated. Perceptual fluency might be a dominant cue for improving monitoring processes which led to more accurate judgements of learning performance.
The impetus for this field of research was provided by Diemand-Yauman et al. (2011) who investigated the effect of disfluency on memory performances more closely. Across two experiments with different samples (university students and high school students) they confirmed that texts with hard-to-read fonts (e.g., Hattenschweiler) led to better learning performances than presenting text with easy-to-read fonts (e.g., Arial). In another study, Eitel et al. (2014) tried to apply the disfluency effect to multimedia learning across four experiments. However, a benefit for disfluent material could be found only in the first experiment. The manipulation of the text and the pictures in Experiment 1 is of particular interest. The disfluent text was presented in the same way as in the study by Diemand-Yauman et al. (2011). Pictures in a less legible format were operationalized as a low-quality photocopy. An ANCOVA with spatial ability as covariate showed that participants with disfluent text outperformed participants with fluent text regarding transfer. However, no significant differences between the groups could be detected for retention. In line with assumptions of desirable difficulties, learners receiving less legible texts invested significantly more mental effort than learners confronted with legible texts. However, the Experiments 2, 3, and 4 were not able to replicate the learning-beneficial effect of disfluency.
Making texts perceptually harder-to-read can also be implemented by handwritten texts. For instance, a study by Geller et al. (2018) examined the impact of cursive text on students’ performance in a recognition memory task. Participants studied the learning material (word list) either with text in type-print, easy-to-read cursive, and hard-to-read cursive. The results confirmed that “cursive words were better remembered than type-print words, indicating that cursive script serves as a desirable difficulty” (Geller et al., 2018, p. 1114). However, the difference between easy-to-read and hard-to-read cursive script did not reach significance. The degree of disfluency, therefore, does not seem to be sufficient as a theoretical explanatory approach. Two additional experiments confirmed that the memory effect is also stable across different list designs and after a 24 h delayed learning test.
To sum up, empirical evidence for the learning beneficial effect of hard-to-encode instructional materials is not conclusive. Several experimental studies came to debilitating results and could not find any benefit for disfluency (e.g., Faber et al., 2017; İli̇c and Akbulut, 2019; Rummer et al., 2016; Yue et al., 2013). A meta-analysis by Xie et al. (2018) also questions the robustness of the disfluency effect in text-based educational settings. In this vein, no significant effects of perceptual disfluency were found on recall (d = –0.01) and transfer (d = 0.03) outcomes. Furthermore, the theoretical foundation, postulated by Alter et al. (2007) is questioned as well. Thompson et al. (2013) found that perceptual fluency had no impact on metacognitive judgements and metacognitive accuracy. In consequence, boundary conditions or further explanations have to be taken into account to explain these inconsistent findings.
Disfluency as Extraneous Cognitive Load
An explanation for the rather inconsistent findings in disfluency research can be provided by considering the Cognitive Load Theory (CLT; Sweller, 1988, Sweller, 2010), which represents a well-established and empirically verified framework. The goal is to provide instructional guidelines and design recommendations that efficiently use the limited working memory to promote learning (Paas et al., 2003; Sweller et al., 2019). The load imposed on the cognitive structures can be divided into three types (intrinsic, extraneous, and germane cognitive load; Paas et al., 2003; Sweller et al., 1998). However, recent publications assumed a two-factor model including intrinsic and extraneous load (e.g., Sweller et al., 2019; Jiang and Kalyuga, 2020). First, intrinsic cognitive load (ICL) can be defined as the internal complexity of the learning material (Kalyuga, 2011). It is determined by the task’s inherent element interactivity and the learner’s domain-specific prior knowledge (Sweller et al., 2019). The concept of element interactivity relates to the complexity of the information to be learned and can be classified on a continuum between low to high. More concretely, interactivity refers to the number of elements that must be processed simultaneously in working memory (Sweller, 1994). Working memory load is not only caused by the task’s inherent complexity, but also by a suboptimal design of the learning material. An inappropriate designed instructional format imposes extraneous cognitive load (ECL) and does not contribute to learning (Paas and Sweller, 2014). To facilitate schema construction and automation, extraneous processing should ideally be avoided (De Jong, 2010). Consequently, the instructional designer can manipulate the ECL while preparing learning materials (Klepsch et al., 2017). Intrinsic and extraneous cognitive load are in an additive relationship to each other and should be considered accordingly in the design (Paas and Sweller, 2014). When appropriate design principles ensure a reduction of the ECL, working memory capacities are freed for managing the tasks immanent element interactivity. However, meaningful learning is also possible, when comparatively low ICL does not require many working memory resources. In this case, high levels of ECL can be managed (Paas and Sweller, 2014). The third component, germane cognitive load (GCL), has experienced a redefinition over the years. Whereas older publications assumed that the GCL describes the required working memory capacities for managing the intrinsic load, current research attributes the GCL as a redistribution function (Sweller et al., 2019) and as active processing (i.e., mental effort; Jiang and Kalyuga, 2020). More precisely, GCL does not contribute to the whole load, but it rather allocates working memory capacities to activities being relevant for learning (Kalyuga, 2011).
The disfluency assumption can be seen as a counterpart to the CLT (Eitel et al., 2014; Lehmann et al., 2016; Xie et al., 2018). Implementing handwritten texts into the learning material might not be adequate for learning in general. In particular, when handwriting is hard to read (i.e., high disfluency), encoding errors can occur (Hartel et al., 2011). Hard-to-read handwriting affects the ECL since additional cognitive resources are required to deal with the inadequate instructional design (Seufert et al., 2017). In line with the CLT, illegible fonts must first be deciphered before learning can take place. In consequence, resources not relevant to learning are expended. Accordingly, receiving learning texts with rather hard-to-read letters induce extraneous load (Beege et al., 2021). A study by Seufert et al. (2017) proved that a high level of disfluency, where the text is barely legible, impedes learning success. Moderate levels of disfluency on the other hand can be quite conducive to learning, for example in terms of lower extraneous load perceptions, higher engagement, and better recall performances. Nevertheless, the authors emphasize the ambiguity about the learning-beneficial degree of disfluency, especially concerning extraneous load. İli̇c and Akbulut (2019) could also show that the combination of disfluent texts and animations causes higher extraneous cognitive load than the same representations in a fluent form. However, there are several studies that could not detect significant effects of disfluency on extraneous load (e.g., Eitel et al., 2014; Kühl et al., 2014).
Disfluency as Social Cue
Recent findings in the field of educational research suggest considering learning not as an exclusively cognitive process. Motivational, affective, meta-cognitive, and social impacts should also be taken into account, since learning engagement is determined by these factors (e.g., Moreno et al., 2001; Mayer et al., 2003; Moreno and Mayer, 2007). A recent framework regarding multimedia learning explicitly includes social variables (CASTLM; Schneider et al., 2018).
As outlined, disfluency can be operationalized as writing instructional test in handwritten form. In this context, disfluent texts can be viewed as encounters for social processes. Triggering social responses with handwritten texts can be explained with the embodiment principle (Mayer, 2014). It is assumed that the implementation of humanlike entities can lead to the feeling of social presence and being in a social communication situation. By activating a social reaction, an increase in active cognitive processing results in better retention outcomes (Mayer et al., 2004; Mayer, 2014). Unlike other techniques (e.g., interactive learning environments; Moreno and Mayer, 2007), presenting handwritten texts is a comparatively simple possibility to induce the perception of a social event (Reeves and Naas, 1996). Accordingly, the learning environment thus fulfills two functions: First, it delivers information about a certain topic to the learner (Mayer, 2001); and second, it induces the feeling of being in a social interaction with the computer (Mayer et al., 2003). When learners receive font as human-like, the trust mechanism could also have a learning-promoting effect. For instance, a learning situation in which the instructor has written the text could be created. Thus, using disfluent text should not only be discussed in the context of desirable difficulty or cognitive load. Disfluent text, under circumstances, should be discussed considering social learning theories as well since several studies prove the beneficial effect of social cues on learning (e.g., for the voice principle, Mayer et al., 2003; for the politeness effect, McLaren et al., 2011).
Hypotheses
As outlined, operationalizing disfluency through a handwritten font can have beneficial as well as detrimental effects on learning. In three experiments, the role of disfluency in learning scenarios should be specified considering cognitive, metacognitive, and social processes.
Hypothesis 1: From the perspective of disfluency as a desirable difficulty, a handwritten text font leads to more elaborated metacognitive activities since difficulties engage to process the information more deeply than it would be the case in easy-to-read learning materials (Alter et al., 2007; Xie et al., 2018). According to explanations provided by Pieger et al. (2016), disfluency should enhance absolute metacognitive accuracy since perceptual fluency might be crucial for more accurate judgements of learning performance. Thus, absolute metacognitive accuracy should be positively influenced by a harder-to-read, handwritten text font.
H1: Learners receiving an instructional text with a handwritten (hard to read) text font achieve a higher absolute metacognitive accuracy than learners receiving an instructional text with a computerized (easy to read) text font.
Hypothesis 2: From the perspective of disfluency as extraneous cognitive load, a handwritten text font negatively affects ECL since additional cognitive resources are required to read the information (Seufert et al., 2017). Illegible fonts must first be deciphered before learning can take place. In consequence, resources not relevant to learning are expended (Beege et al., 2021).
H2: Learners receiving an instructional text with a handwritten (hard to read) text font perceive higher extraneous cognitive load than learners receiving an instructional text with a computerized (easy to read) text font.
Hypothesis 3: From the perspective of disfluency as a social cue, a handwritten text font can trigger social processes and function as a cue for perceived social presence (embodiment principle; Mayer, 2014), by activating a social reaction an increase in active cognitive processing (Mayer et al., 2004; Mayer, 2014). When learners perceive a font as human-like, the trust mechanism could also have a learning promoting effect.
H3: Learners receiving an instructional text with a handwritten (hard to read) text font perceive higher social presence than learners receiving an instructional text with a computerized (easy to read) text font.
Hypothesis 4: Because of these opposing perspectives, it is difficult to postulate an effect of using handwritten text on learning. Furthermore, research findings are ambiguous. Some studies support a disfluency effect (e.g., Diemand-Yauman et al., 2011; Geller et al., 2018). Consequently, the metacognitive or social benefits when using handwritten fonts are more significant for learning processes than the detrimental effect of the increased ECL. Other experiments found opposing effects or no effects of disfluency on learning (e.g., Faber et al., 2017; İli̇c and Akbulut, 2019), indicating that the detrimental effects of ECL are more significant for learning or at least as significant as metacognitive and social benefits. In consequence, boundary conditions seem to determine which effect occurs most dominantly. In the current experiments, the element-interactivity of the learning material was investigated as a potential moderator of the effect. In a rather simple learning environment, metacognitive benefits can unfold since the working memory has enough capacity for encoding the disfluent font and the rising ECL does not significantly influence learning. If the material has a high element-interactivity, no resources are available for encoding the disfluent font and thus, the rising ECL leads to an overload which is dominant despite possible metacognitive or social benefits. Thus, two hypotheses are outlined.
H4a: Learners receiving an instructional text with a low element interactivity and a handwritten (hard to read) text font achieve higher learning outcomes than learners receiving an instructional text with a high element interactivity and a computerized (easy to read) text font.
H4b: Learners receiving an instructional text with a high element interactivity and a handwritten (hard to read) text font achieve lower learning outcomes than learners receiving an instructional text with a low element interactivity and a computerized (easy to read) text font.
To get first insights into learning with handwritten, disfluent texts, a first exploratory experiment with a low element interactivity was carried out. Learning outcomes and cognitive load was measures (Hypothesis 2 and 4). Because of the encouraging results, two additional experiments were conducted with more dependent measures to provide detailed insight. Whereas the first experiment explored the cognitive effects of a disfluent font as well as learning outcomes, the second experiment further included metacognitive variables (Hypotheses 1, 2, and 4). The third experiment further included social variables (Hypotheses 1, 2, 3, and 4). Additionally, exploratory mediation analyses are in the focus of the third main experiment, to determine if learning outcomes are causally effected by rather cognitive, metacognitive, or social processes and thus, to provide general theoretical implications of the emergence of a disfluency effect.
Experiment 1
Methods
Participants and Design. Overall, the discussed studies on the disfluency effect provided diverse effect sizes. Studies that supported the disfluency effect reported small to medium effect sizes (e.g., Eitel and Kühl, 2016) or medium to high effect sizes (e.g., Seufert et al., 2017). To detect, at least, a medium effect size concerning an one-factorial experiment with two factor levels, an a-priori power analysis (f = 0.25; α = 0.05; 1 - ß = 0.80) revealed that 102 participants must be acquired. With respect to this analysis, 97 secondary students (48.5% female; age: M = 11.79, SD = 0.48) participated in Experiment 1. Students were in the 5th (22.7%) or 6th (77.3%) grade. Prior knowledge of the participants (M = 0.76, SD = 0.74; with a maximum of five points) was low. Students attended secondary schools (Gymnasium) in XXX.
The participants were randomly assigned to two experimental conditions (handwritten font vs. computerized font) of a between-subjects design by drawing lots. Forty-seven students were assigned to the condition with handwritten font and 50 students were assigned to the condition with computerized font. For the experimental conditions, no significant differences existed in terms of age or prior knowledge, t(95) = [0.04, 0.13]; p = [0.90, 0.97] as well as gender, χ2 = 2.95; p = 0.09.
Materials. The learning material consisted of an instructional text. The text dealt with the geography, climatic characteristics, politics, culture, and language of the country Sweden. The topic was chosen since it was not part of the curriculum of these secondary students. Thus, prior knowledge was assumed to be low. The text had 672 words and was divided into five segments, which were presented on different web-pages. On average, 134.4 words were presented per segment. The participants could click on the forward or backward buttons to navigate through the web-pages. They could navigate and re-read the segments as often as they wanted. There was a finish button on the last page. Once this button had been clicked, the learning websites could no longer be accessed. The participants decided themselves how long they wanted to learn. In the computerized condition, the text was displayed in the legible font Arial. For the handwritten condition, the text was printed and traced to ensure that the size of the letters and the arrangement of the text on the page is identical. The used handwritten font was based on the standard school writing to ensure that the font clearly perceived as written by another person and disfluent but not completely illegible. A screen example of the experimental manipulation is shown in Figure 1.
Measures. To assess prior knowledge, five open answer questions were presented (ω = 0.37). The questions covered the spectrum of knowledge that was later included in the learning text. Students were able to get one point per question (a maximum of five points). An example was: “What is the most common animal in Sweden?”.
A knowledge test was implemented to assess learning gain. In this vein, twelve multiple-choice questions (ω = 0.41; e.g., “The neighboring countries of Sweden are...”) and four open answer questions (ω = 0.70; e.g., “What is the capital of Sweden?”) were formulated. Multiple-choice questions refer to recognizing the learning content and open answer questions refer to the reproduction of information (e.g., Atkinson and Shiffrin, 1968). The multiple-choice questions consisted of two to four possible answers. From one to all of the answer options could be correct within a question. A participant gained one point if he or she marked the correct answer option or correctly not marked a wrong answer. Participants could reach up to 41 points for the multiple choice-question test. This approach was chosen because explicitly giving points for correctly rejecting false answers reduces blind guessing and leads to higher reliability of the knowledge test (Burton, 2005). Even if reducing guessing in knowledge tests might disadvantage learners with poor metacognitive monitoring skills (Higham and Arnold, 2007), a bias was avoided because of the randomized allocation of students to the experimental groups. The open questions could always be answered with a single or a few words or numbers. A preset of possible answers was created to ensure a rating that was as objective as possible. Overall, students were able to gain a maximum of 14 points. The low reliabilities might be explained by considering item construction. The items of both learning scales were designed to assess different sub-topics. Furthermore, items had different difficulties to create a broad variance in the answer behavior of the participants. In consequence, internal-consistency was restricted.
To measure cognitive load, the scale from Leppink et al. (2014) was implemented. The questionnaire consisted of ten items. Three items measured ICL (ω = 0.77; e.g., “The subjects in the learning environment were complicated”), three items measured ECL (ω = 0.53; e.g., “The explanations in the learning environment were unclear”) and four items measured GCL (ω = 0.85; e.g., “The learning environment improved my understanding of the topic I was working on”). Students were asked to rate these items on an 11-point Likert scale ranging from “not correct at all” to “totally correct”.
Procedure. The experiment was carried out in a computer lab parallel to normal school activity. Thus, the experiment was embedded in a school lesson and lasted 45 min. One class participated per experimental run (20–25 students). The working stations were prepared by opening the learning environment on the computer desktop (screen was turned off) and by placing the paper-pencil questionnaire on the desks. Students started with the paper-pencil questionnaire by completing the prior knowledge test. Afterward, the learning phase took place. Participants were instructed to turn on the monitor with the pre-opened learning material. Students read and navigated through the web pages. Finally, the dependent variables were assessed on the paper-pencil questionnaire in the following order: cognitive load, knowledge test, demographic questions.
Results
In the analyses of data, multivariate analyses of variance (MANOVAs) and univariate analyses of variance (ANOVAs) were conducted to assess differences between groups. For all variance analyses, disfluency (handwritten font vs. computerized font) was used as independent variable. Since no other variable (i.e., age, gender, prior knowledge) significantly differed among the experimental groups, no covariate was used for the analyses. Pre-defined test assumptions were only reported if significant violations occur. Descriptive results for all dependent variables are outlined in Table 1.
Learning Outcomes. A MANOVA was conducted with recognition (multiple-choice) and recall (open ended questions) as dependent variables. A significant main effect with a large effect size was found for disfluency; Wilk’s Λ = 0.70; F(2, 94) = 19.92, p < 0.001, ηp2 = 0.30.
Follow-up ANOVAs were conducted to get deeper insights into the significant main effect. A significant effect was found for the multiple-choice questions; F(1, 95) = 25.80, p < 0.001, ηp2 = 0.21 and the open-ended questions; F(1, 95) = 11.11, p = 0.001, ηp2 = 0.11. Students in the handwritten condition achieved higher learning outcomes than students in the condition with the computerized font.
Cognitive Load. A MANOVA was conducted with ICL, ECL and GCL as dependent variables. A significant main effect with a medium effect size was found for disfluency; Wilk’s Λ = 0.91; F(2, 93) = 3.09, p = 0.03, ηp2 = 0.09.
Follow up ANOVAs were conducted in order to get deeper insights into the significant main effect. A significant effect was found for GCL; F(1, 95) = 6.11, p = 0.02, ηp2 = 0.06. Students in the handwritten condition reported a higher GCL than students in the condition with the computerized font. No effects could be found with regard to ICL; F(1, 95) = 1.48, p = 0.23, ηp2 = 0.02 and ECL; F(1, 95) = 0.01, p = 0.93, ηp2 < 0.001.
Discussion
This first exploratory experiment was carried out to investigate the effects of a handwritten font in contrast to a computerized font. Results partly support the disfluency effect, since learning outcomes as well as active, generative processing were enhanced in the handwritten (disfluent) condition. This might be a first hint that using a handwritten font in educational settings can foster learning processes. In particular, when students are familiar with handwritten fonts (Ito et al., 2020) and if the degree of disfluency in handwritten fonts is rather low (Geller et al., 2018), handwritten fonts can foster learning. Since 5th and 6th graders often study with handwritten texts, disfluency through handwritten fonts can be effectively included in learning materials. Nevertheless, several limitations have to be discussed.
First, because of the exploratory nature of the study, several process variables were not assessed. Thus, no statements about metacognitive or social variables can be postulated. Furthermore, no effect regarding ECL could be observed. An explanation is the use of the CL questionnaire. ECL was assessed with items like “The explanations in the learning environment were unclear.” Thus, the scale was rather misleading and did not cover ECL regarding the used test font. Additionally, reliabilities of the multiple-choice questionnaire and the ECL subscale was rather low. Thus, results have to be interpreted with caution and might be explained by methodical flaws. Additionally, more data was necessary to generalize the findings across other knowledge domains and other study samples. Nevertheless, these first exploratory results were encouraging. In order to resolve the methodological problems of Experiment 1, two additional experiments were carried out.
In Experiment 2 and 3, the disfluency effect was investigated with a student sample to increase generalizability of the results. Furthermore, the learning topics were changed in the following two experiments. In Experiment 2, a mathematical topic and in Experiment 3, a natural-scientific topic was used as learning material.
Experiment 2
Methods
Participants and Design. Concerning the power-analysis conducted in Experiment 1, the acquisition of 102 participants was aimed for. One hundred and five participants (78.1% female; age: M = 23.81, SD = 10.25) participated in the experiment. Participants were university students from the XXXX. Students were enrolled in media communications (73.5%), instructional psychology (22.5%), and other fields of study (3.9%). Students got a 1-h course credit or 5€ as a reward for participating. Prior knowledge of the participants (M = 0.49, SD = 0.68; with a maximum of five points) was low.
The participants were randomly assigned to one of two experimental conditions (handwritten font vs. computerized font) of a between-subjects design by an online randomization software. Forty-seven students were assigned to the condition with handwritten font and 58 students were assigned to the condition with the computerized font. For the experimental conditions, no significant differences existed in terms of gender and field of study, χ2 = [0.11, 0.33]; p = [0.74, 0.85]. There were significant differences in terms of age, t(103) = 2.31; p = 0.02 and prior knowledge, t(103) = 2.12; p = 0.04 indicating that participants in the handwritten condition were younger and had more prior knowledge. Thus, age, as well as prior knowledge, were included as covariates in all analyses.
Materials. The learning material consisted of an instructional text which dealt with matrix calculation. Again, the topic was chosen, since prior knowledge of the participants was considered low. Furthermore, the change of subject might show to what extent the results of Experiment 1 can be generalized. The text had 725 words and was divided into eleven segments, which were presented on different pages. On average, 68.4 words were presented per segment. The material did not only consist of the instructional text. Mathematical formulas were presented to illustrate exemplary calculations. The participants could click on the forward or backward buttons to navigate through the websites. They could navigate and re-read the segments as often as they wanted and there was a finish button on the last page. Once this button had been clicked, the websites could no longer be accessed. The participants decided themselves how long they wanted to learn. In the computerized condition, the text was displayed in the legible font Times New Roman. For the handwritten condition, the text was printed and traced to ensure that the size of the letters and the arrangement of the text on the page is identical. A screen example of the experimental manipulation is shown in Figure 2.
Measures. To assess prior knowledge (ω = 0.62), six open answer questions were presented. Because of the low inter-rater reliability, one item was excluded from the analyses (new reliability: ω = 0.68). The questions covered the spectrum of knowledge that was later included in the learning text. Students were able to get one point per question (a maximum of five points). An example was: “How do you multiply matrices?”. Inter-rater reliability of two independent rater with regard to the remaining five items was high, ICC (1, k) = [0.35, 0.96], F(104, 104) = [2.10, 47.85], p < 0.001.
A knowledge test was implemented to assess learning gain. In this vein, eight multiple-choice (retention) questions (ω = 0.58; e.g., “What is a vector?”) and seven arithmetic (transfer) problems (ω = 0.52; e.g., “Multiply the following vectors”) were formulated. Retention, as well as transfer, was measured to get a deeper insight into rather basal and more complex learning processes. According to Mayer (2014), retention can be defined as “remembering” content, which has been explicitly presented in an instructional text. Transfer knowledge is defined as “understanding.” The learners had to solve novel problems that were not explicitly presented in the instructional text by using the acquired knowledge (Mayer, 2014). The multiple-choice questions were designed as in Experiment 1. Participants could reach up to 32 points. The arithmetic problems had to be solved and calculated by the participants without additional tools. Students could reach one point per correct solution. Overall, students were able to gain a maximum of seven points.
In contrast to Experiment 1, cognitive load was measured with the questionnaire from Klepsch et al. (2017), which was chosen because the ECL subscale explicitly refers to recognition of information. Furthermore, the scale was found to be valid in various learning situations (Klepsch and Seufert, 2020). Two items measured ICL (ω = 0.84; e.g., “This task was very complex”). Three items measured ECL (ω = 0.78; e.g., “During this task, it was exhausting to find the important information”). Theoretically, germane processes (GCL) are subsumed under the facet of ICL (two-factor model; Jiang and Kalyuga, 2020). In the used questionnaire, ICL items rather refer to the complexity of the learning material and GCL items refer to active processing and mental effort (Klepsch et al., 2017). Thus, the GCL facet of the questionnaire was included separately. Two items measured germane processes (ω = 0.75; e.g., “My point while dealing with the task was to understand everything correct”). The participants had to rate the items on a 7-point Likert scale ranging from 1 (completely wrong) to 7 (completely correct).
The procedure for assessing metacognitive judgments and metacognitive accuracy was based on Pieger et al. (2016). Ease of learning (EOL) was measured by the question, “How easy or difficult will it be to learn the text?” on a scale from 0 (very difficult) to 100 (very easy). Judgments of learning (JOL) were measured twice, to assess if they could answer retention questions (“What percentage of the questions about the text will you answer correctly?”) and solve arithmetic problems (transfer performance; “What percentage of the arithmetic problems will you solve correctly?”) on a scale from 0 (no questions) to 100 (all questions). Retrospective confidence (RC) was measured by the question, “How confident are you that your answer is correct?” on a scale from 0 (unconfident) to 100 (confident). In line with the JOL questions, RC questions were implemented after the retention as well as transfer questionnaire. Metacognitive accuracy was calculated as absolute. The five metacognition scores (EOL, JOL [retention and transfer], and RC ratings [retention and transfer]) and the learning scores were z-standardized prior to analyses. Z-standardization was carried out for the whole sample in order to examine differences in metacognitive judgments and their relation to performance between the experimental groups. For the absolute accuracy calculation, the performance scores were subtracted from the five metacognition scores (Pieger et al., 2016). Non-standardized accuracy scores (differences between judgments and performance) can be found in Supplementary Appendix B.
Finally, the learning time in seconds and navigation (the number of switches between the web-pages) were tracked to get insight into the learning behavior of the participants. Concerning learning time, an efficiency score was conducted based on the formula from Van Gog and Paas (2008).
For p, performance scores (retention and transfer) were included and T was the learning time. Learning time as well as performance scores were z-standardized. Efficiency was calculated for retention and transfer performance separately.
Procedure. A computer laboratory at the university with ten identical computers was prepared before each experimental session. The online questionnaire was pre-opened at each workstation. Up to ten participants were tested simultaneously. Sight-blocking partition walls were used to ensure that the students worked independently. At the beginning of the experiment, the participants were told that the experiment was an instructional study on a science topic and were asked to answer the prior knowledge test. Then, they were given the link to the learning material and asked to take a preliminary look at the learning environment and the learning text. After 2 seconds, the participants were automatically redirected to a questionnaire and had to evaluate the EOL item. The learning phase then began. The students had to learn the material at their own pace. They were able to navigate freely between the individual learning segments. When they had finished the learning phase, the students had to rate two JOL items. They should predict how many questions they could possibly answer correctly (retention) and how many arithmetic problems they could possibly solve (transfer). Further, dependent variables were measured after finishing the learning phase. At the beginning of this questionnaire, the cognitive load was assessed. Afterward, retention and transfer were measured. One RC item had to be answered after the retention test and on RC item had to be answered after the transfer test. Finally, the students had to answer a demographic questionnaire. When all the tests had been completed, the participants could leave the room. The experiment lasted a total of 45 min.
Results
In the analyses of data, multivariate analyses of covariance (MANCOVAs) and univariate analyses of covariance (ANCOVAs) were conducted to assess differences between groups. For all variance analyses, disfluency (handwritten font vs. computerized font) was used as the independent variable. Age and prior knowledge were used as covariates in all analyses since these variables significantly differed between the experimental groups. Pre-defined test assumptions were only reported if significant violations occur. Descriptive results for all dependent variables are outlined in Table 2.
Learning Outcomes. A MANCOVA was conducted with retention (multiple-choice) and transfer (arithmetic problems) as dependent variables. Prior knowledge; Wilk’s Λ = 0.90; F(2, 100) = 5.49, p = 0.01, ηp2 = 0.10 but not age; Wilk’s Λ = 0.99; F(2, 100) = 0.34, p = 0.71, ηp2 = 0.01 was a significant covariate. A significant main effect with a large effect size was found for disfluency; Wilk’s Λ = 0.84; F(2, 100) = 9.77, p < 0.001, ηp2 = 0.16. Follow up ANCOVAs revealed a significant effect for retention; F(1, 101) = 19.67, p < 0.001, ηp2 = 0.16 but not for transfer; F(1, 101) = 0.18, p = 0.67, ηp2 = 0.002. In contrast to Experiment 1 students in the computerized font condition achieved higher retention outcomes than students in the condition with the handwritten font.
Cognitive Load. A MANCOVA was conducted with ICL, ECL, and GCL as dependent variables. Neither prior knowledge; Wilk’s Λ = 0.98; F(3, 99) = 0.78, p = 0.51, ηp2 = 0.02 nor age; Wilk’s Λ = 0.99; F(3, 99) = 0.33, p = 0.80, ηp2 = 0.01 were significant covariates. No main effect was found for disfluency; Wilk’s Λ = 0.98; F(3, 99) = 0.82, p = 0.82, ηp2 = 0.02.
Metacognition. A MANCOVA was conducted with EOL, JOL (retention and transfer), and RC (retention and transfer) as dependent variables. Prior knowledge; Wilk’s Λ = 0.80; F(5, 95) = 4.73, p = 0.001, ηp2 = 0.20 but not age; Wilk’s Λ = 0.97; F(5, 95) = 0.55, p = 0.74, ηp2 = 0.03 was a significant covariate. No main effect was found for disfluency; Wilk’s Λ = 0.99; F(5, 95) = 0.24, p = 0.95, ηp2 = 0.01.
After analyzing metacognitive measures, metacognitive accuracy scores were investigated. At first, absolute metacognitive accuracy with regard to retention performance was analyzed. A MANCOVA was conducted metacognitive accuracy scores (EOL, JOL [retention], and RC [retention]) as dependent variables. Neither prior knowledge; Wilk’s Λ = 0.98; F(3, 97) = 0.55, p = 0.65, ηp2 = 0.02 nor age; Wilk’s Λ = 0.98; F(3, 97) = 0.81, p = 0.49, ηp2 = 0.02 were significant covariates. A significant main effect with a large effect size was found for disfluency; Wilk’s Λ = 0.86; F(3, 97) = 5.34, p = 0.002, ηp2 = 0.14. Follow up ANCOVAs revealed a significant effect for all accuracy scores; F(1, 99) = [10.13, 12.20], p = [0.001, 0.002], ηp2 = [0.09, 0.11]. Students in the computerized condition had positive accuracy scores whereas students in the handwritten condition had negative scores. T-tests against zero revealed that students in the computerized condition overestimated their performance regarding all accuracy scores; t(57) = [2.66–3.22], p = [0.001–0.005], d = [0.35–0.43]. Students in the handwritten condition underestimated their performance with regard to all accuracy scores; t(46) = [-2.27–-2.41], p = [0.02–0.03], d = [-0.34–-0.35]. Second, absolute metacognitive accuracy with regard to transfer performance was analyzed. A MANCOVA was conducted metacognitive accuracy scores (EOL, JOL [transfer], and RC [transfer]) as dependent variables. Neither prior knowledge; Wilk’s Λ = 0.95; F(3, 97) = 1.84, p = 0.15, ηp2 = 0.05 nor age; Wilk’s Λ = 0.99; F(3, 97) = 0.44, p = 0.73, ηp2 = 0.01 were significant covariates. No main effect was found for disfluency; Wilk’s Λ = 0.99; F(3, 97) = 0.20, p = 0.90, ηp2 = 0.01.
Learning Time and Navigation. A MANCOVA was conducted with learning time and navigation as dependent variables. Neither prior knowledge; Wilk’s Λ = 0.98; F(3, 97) = 1.84, p = 0.15, ηp2 = 0.05 nor age; Wilk’s Λ = 0.99; F(3, 97) = 0.44, p = 0.73, ηp2 = 0.01 were significant covariates. A significant main effect with a small to medium effect size was found for disfluency; Wilk’s Λ = 0.93; F(3, 97) = 3.67, p = 0.03, ηp2 = 0.07. Follow up ANCOVAs were conducted in order to get deeper insights into the significant main effect. A significant effect was found for learning time; F(1, 98) = 6.70, p = 0.01, ηp2 = 0.06. Students in the handwritten condition learned longer than students in the condition with the computerized font. A significant effect was found for navigation; F(1, 98) = 4.72, p = 0.03, ηp2 = 0.05. Students in the handwritten condition navigated more often through the websites than students in the condition with the computerized font.
To analyze learning efficiency, a MANCOVA was conducted with learning efficiency with respect to retention and transfer as dependent variables. Prior knowledge; Wilk’s Λ = 0.90; F(2, 97) = 5.35, p = 0.01, ηp2 = 0.10 but not age; Wilk’s Λ = 0.99; F(2, 97) = 0.33, p = 0.72, ηp2 = 0.01 was a significant covariate. A significant main effect with a large effect size was found for disfluency; Wilk’s Λ = 0.80; F(2, 97) = 11.98, p < 0.001, ηp2 = 0.20. Follow up ANCOVAs showed a significant effect for retention efficiency; F(1, 98) = 24.03, p < 0.001, ηp2 = 0.20. Students in the computerized condition had a higher efficiency than students in the condition with the handwritten font. A significant effect was found for transfer efficiency; F(1, 98) = 4.99, p = 0.03, ηp2 = 0.05. Students in the computerized condition had a higher efficiency than students in the condition with the handwritten font.
Discussion
The second experiment was carried out to shed more light on the emergence of a potential disfluency effect. Interestingly, the results of Experiment 1 could not be replicated. In Experiment 1, learning scores as well as GCL was enhanced in the disfluent condition. These results were in line with the disfluency effect. In contrast, results of Experiment 2 mostly contradicted the disfluency effect. Students in the disfluent (handwritten) condition achieved worse retention outcomes and related to the learning time, students in the disfluent condition had lower accuracy scores than students in the fluent condition. No main effects of the metacognitive monitoring scores could be obtained but metacognitive accuracy scores showed that participants in the disfluent condition underestimated their learning skills at the beginning, during, and after learning. A disfluent font might discourage learners to invest effort into schema construction. Learners were discouraged by encoding this illegible information and consequently, learners might invest less effort into learning because they rated their learning success too low compared to their abilities. Learners were less focused which might lead to longer learning time and the need for additional navigation. This interpretation contradicts the common explanation of the disfluency effect pointing out that using disfluent fonts encourages learners to invest more effort and to use more elaborated learning strategies (Alter et al., 2007). Another explanation would be that, in line with common explanations, learners were encouraged to invest more effort in encoding the font because they realized that there might be a gap between the learning task and their cognitive skills. Though, the additional effort which was invested in encoding consumed too many cognitive resources and not enough resources for schema construction left. Nevertheless, students did not benefit from their additional study time which can be interpreted as a labor-in-vain effect (Nelson and Leonesio, 1988). In line with Experiment 1, no effects regarding ECL could be found. Cognitive effects were more likely to be unconscious or the findings cannot be explained explicitly concerning cognitive variables.
The differences between the results from Experiment 1 and 2 and the pre-study might be explained considering the new learning material. In Experiment 1, a learning material with comparative low element interactivity was used. Information could be learned, recalled, and applied without considering other information from the material. Furthermore, the information was rather surface knowledge since it was adapted to younger learners. The material might be so easy that learners were not engaged. The disfluent font made learners an effort to learn the material whereas students in the computerized condition did not invest many resources in learning the material and the disfluency effect occurred. In Experiment 2, the material was complex and had a high element interactivity. The presentation of this material in a disfluent font overtaxed learners because they have already invested a lot of resources in learning the complex content. The overtaxed learners underestimated their performance and did not compensate for the negative effects of the font through an adequate effort in learning. Altogether, using a disfluent font led to unfavorable learning conditions and learning behavior.
Overall, Experiment 1 and 2 pointed out that there might be no general disfluency effect. Boundary conditions seemed to determine if a handwritten text font has rather positive or negative effects on learning. Furthermore, social effects of handwritten learning material were not investigated in the first two experiments. In consequence, a third experiment was carried out 1) to investigate social variables in addition to cognitive and metacognitive variables, 2) to get additional insights in causal indirect effects on learning by conducting mediation analyses with these multidisciplinary process variables, and 3) to further investigate the influence of element interactivity on learning with disfluent material. Since Experiment 1 used low-interactivity material and Experiment 2 used high-element interactivity material, an instructional text with medium element interactivity was used as learning material.
Experiment 3
Methods
Participants and Design. Again, the acquisition of 102 participants was aimed for. One hundred and three participants (74.8% female; age: M = 22.71, SD = 2.97) participated in the experiment. Participants were university students from the XXXX. Students were enrolled in media communications (58.3%), instructional psychology (32.0%), and other fields of study (9.7%). Students got a 1-h course credit or they took part in a raffle for a 20€ voucher. Prior knowledge of the participants (M = 0.50, SD = 1.04; with a maximum of thirteen points) was low.
The participants were randomly assigned to two experimental conditions (handwritten font vs. computerized font) of a between-subjects design by an online randomization software. Fifty-seven students were assigned to the condition with handwritten font and 46 students were assigned to the condition with computerized font. For the experimental conditions, no significant differences existed in terms of gender and field of study, χ2 = [0.03, 1.54]; p = [0.46, 0.86] as well as age and prior knowledge; t(101) = [0.29, 1.03]; p = [0.31, 0.78]. In consequence, no covariates had to be included in further analyses.
Materials. The learning material consisted of an instructional text. The text dealt with the chemical process of pyrolysis. The topic was chosen since prior knowledge of the participants was considered low. The element interactivity of the material was higher than in Experiment 1 since not only basal facts that did not depend on each other were taught. The element interactivity of the material was further not as high as in Experiment 2 since single paragraphs displayed information that depends on each other but subtopics were self-contained. In Experiment 2, all information was necessary to understand the learning material to the end. Consequently, in comparison to Experiment 1 and 2, the material of Experiment 3 had a medium element interactivity. The text had 676 words and was divided into ten segments which were presented on different pages. On average, 67.7 words were presented per segment. The material did not only consist of an instructional text. One table (summarizing information about the different types of pyrolysis) and one figure (illustrating the yield of ethylene) were additionally included in the learning material. The participants could click on the forward button to navigate through the websites and there was a finish button on the last page. Once this button had been clicked, the websites could no longer be accessed. The participants decided how long they wanted to learn themselves. In the computerized condition, the text was displayed in the legible font Arial. For the handwritten condition, the text was printed and traced to ensure that the size of the letters and the arrangement of the text on the page is identical. A screen example of the experimental manipulation is shown in Figure 3.
Measures. To assess prior knowledge (ω = 0.71), three open answer and three single choice questions were presented. The questions covered the spectrum of knowledge that was later included in the learning text. Students were able to get three to four points per open answer question and one point per single choice question (a maximum of thirteen points). An example was: “Where is pyrolysis used in practice?”. Inter-rater reliability of two independent rater with regard to the three open answers was high, ICC (1, k) = [0.55, 0.79], F(102, 102) = [3.51, 8.52], p < 0.001 or perfect (ICC = 1).
A knowledge test was implemented to assess learning gain. In this vein, a retention test (ω = 0.65; e.g., “What characterizes the thermo-chemical process of pyrolysis?”) consisting of eight multiple-choice questions and two open-answer questions, as well as a transfer test (ω = 0.65; e.g., “please specify, if the following chemical equations are a pyrolysis. Please substantiate your decision”) consisting of two multiple-choice questions and five open-answer questions was implemented. The scoring of the multiple-choice questions was similar to Experiment 1 and 2. For the open-answer questions, participants had to remember information that was explicitly in the text (retention) or decide if presented chemical equations are a pyrolysis and explain their decision (transfer). Inter-rater reliability of two independent rater and the three open-answer questions was high, ICC (1, k) = [0.77, 0.94], F(102, 102) = [8.85, 34.27], p < 0.001. In sum, students were able to gain 67 points for retention and 18 points for transfer.
Measurement of cognitive load (ICL: ω = 0.71; ECL: ω = 0.83; GCL: ω = 0.63), metacognitive variables and learning time was nearly identical to Experiment 2. The only difference from Experiment 2 is that only one JOL and RC score was assessed after learning phase, since no arithmetic problems had to be solved. Again, non-standardized accuracy scores are displayed in Supplementary Appendix B.
In addition to Experiment 2, social presence (ω = 0.73) was measured with a self-created scale based on a scale from Bailenson et al. (2004). Five items (e.g., “The text was impersonal”) measured if the participants had the subjective feeling of a social context. Students had to rate the items on a 7-point Likert scale ranging from 1 (absolutely wrong) to 7 (absolutely correct).
Procedure. The procedure was largely identical to the procedure of Experiment 2. The only difference was that the experiment was completely carried out online. In line with Experiment 2, students 1) were instructed, 2) answered the prior knowledge questions, 3) completed the learning phase and the metacognitive items, 4) completed the questionnaire concerning the dependent and demographic variables. The experiment lasted a total of 45 min.
Results
In the analyses of data, multivariate analyses of variance (MANOVAs) and univariate analyses of variance (ANOVAs) were conducted to assess differences between groups. Furthermore, mediation analyses were conducted to get a deeper insight into the causal processes during learning. Mediator analyses were carried out using PROCESS (Model 4; Hayes, 2017) with a bootstrap-sample of N = 5,000. For all variance analyses, disfluency (handwritten font vs. computerized font) was used as the independent variable. No covariates were used. Pre-defined test assumptions were only reported if significant violations occur. Descriptive results for all dependent variables are outlined in Table 3.
Learning Outcomes. A MANOVA was conducted with retention and transfer as dependent variables. No main effect could be found for disfluency; Wilk’s Λ = 0.97; F(2, 99) = 1.71, p = 0.19, ηp2 = 0.03.
Cognitive Load. A MANOVA was conducted with ICL, ECL, and GCL as dependent variables. A significant main effect with a medium effect size was found for disfluency; Wilk’s Λ = 0.89; F(3, 99) = 4.10, p = 0.01, ηp2 = 0.11.
A follow up ANOVA revealed a significant effect for ECL; F(1, 101) = 8.51, p = 0.004, ηp2 = 0.08. Participants in the handwritten font condition reported a higher ECL than students in the computerized condition. No effects were found regarding ICL; F(1, 101) = 0.01, p = 0.92, ηp2 < 0.001 and GCL; F(1, 101) = 0.12, p = 0.73, ηp2 = 0.001.
Metacognition. A MANOVA was conducted with EOL, JOL, and RC as dependent variables. A significant main effect with a high effect size was found for disfluency; Wilk’s Λ = 0.71; F(3, 99) = 13.21, p < 0.001, ηp2 = 0.29.
A follow up ANOVA revealed a significant effect for EOL; F(1, 101) = 38.051, p < 0.001, ηp2 = 0.27. Participants in the handwritten font condition reported a lower EOL (harder to learn) than students in the computerized condition. No effects were found regarding JOL; F(1, 101) = 3.42, p = 0.07, ηp2 = 0.03 and RC; F(1, 101) = 0.26, p = 0.61, ηp2 = 0.003.
At first, absolute metacognitive accuracy with regard to retention performance was analyzed. A MANOVA was conducted metacognitive accuracy scores (EOL, JOL, and RC) as dependent variables. A significant main effect with a large effect size was found for disfluency; Wilk’s Λ = 0.85; F(3, 94) = 5.69, p = 0.001, ηp2 = 0.15. Follow up ANOVAs revealed a significant effect for EOL accuracy; F(1, 96) = 12.20, p = 0.002, ηp2 = 0.11. In contrast to Experiment 2, students in the computerized condition had negative accuracy scores whereas students in the handwritten condition had positive scores. T-tests against zero indicated that students in the computerized condition underestimated their performance; t(44) = -2.55, p = 0.01, d = -0.38 whereas students in the handwritten condition overestimated their performance; t(52) = 2.62, p = 01, d = 0.36. No differences regarding JOL accuracy; F(1, 96) = 1.01, p = 0.32, ηp2 = 0.01 and RC accuracy; F(1, 96) = 0.05, p = 0.82, ηp2 = 0.001 could be observed.
Second, absolute metacognitive accuracy with regard to transfer performance was analyzed. A MANOVA was conducted metacognitive accuracy scores (EOL, JOL, and RC) as dependent variables. A significant main effect with a large effect size was found for disfluency; Wilk’s Λ = 0.77; F(3, 93) = 9.47, p < 0.001, ηp2 = 0.23. Follow up ANOVAs revealed a significant effect for EOL accuracy; F(1, 95) = 27.84, p < 0.001, ηp2 = 0.23 and JOL accuracy; F(1, 95) = 8.15, p = 0.01, ηp2 = 0.08. Again, t-tests against zero indicated that students in the computerized condition underestimated their performance; t[43, 44] = [-3.55, -2.19], p = [<0.001, 0.03], d = [-0.54, -0.33] whereas students in the handwritten condition overestimated their performance; t(52) = [1,87, 3.91], p = [< 001, 0.03], d = [0.26, 0.58]. No effect could be found for RC accuracy; F(1, 95) = 3.51, p = 0.06, ηp2 = 0.04 but descriptively, the direction of the effect is similar.
Learning Time. Levene’s test indicated unequal variances; F(1, 101) = 7.44, p = 0.01. Thus, an U test was conducted. No effect for disfluency could be found; U = 1,201.50, p = 0.47.
To analyze learning efficiency, a MANOVA was conducted with learning efficiency with respect to retention and transfer as dependent variables. A significant main effect with a medium effect size was found for disfluency; Wilk’s Λ = 0.91; F(2, 94) = 4.40, p = 0.02, ηp2 = 0.09. Follow up ANOVAs were conducted in order to get deeper insights into the significant main effect. A significant effect was found for retention efficiency; F(1, 95) = 7.53, p = 0.01, ηp2 = 0.07. Students in the computerized condition had a higher efficiency than students in the condition with the handwritten font. No effect was found for transfer efficiency; F(1, 95) = 0.08, p = 0.78, ηp2 = 0.001.
Social Presence. An ANOVA was conducted with social presence as dependent variable. A significant effect with a large effect size was found for disfluency; F(1, 101) = 29.12, p < 0.001, ηp2 = 0.22. Students in the handwritten font condition reported higher presence scores than students in the computerized condition.
Mediation Models. Mediation models were conducted to get deeper insights into how cognitive, metacognitive, and social variables influence learning with disfluent material. Concerning the previous findings, ECL, EOL, and social presence were used as mediators and learning outcomes (retention and transfer) were used as dependent variables. All variables were z-standardized.
For retention as dependent variable (see Figure 4), the mediator analysis showed no significant direct effect of disfluency on retention (β = –0.06; SE = 0.10; p = 0.54). When ECL, EOL and social presence were considered as mediator, this effect remained non-significant (β = 0.13; SE = 0.13; p = 0.32). As already outlined, using a handwritten font instead of a computerized font led to a higher ECL (β = 0.27; SE = 0.10; p = 0.01), a lower EOL (β = –0.51; SE = 0.09; p < 0.001) and a higher social presence (β = 0.48; SE = 0.09; p < 0.001). ECL had a negative impact on retention (β = –0.26; SE = 0.11; p = 0.02). Social presence (β = –0.14; SE = 0.12; p = 0.23) and EOL (β = 0.11; SE = 0.12; p = 0.39) had no impact on retention performance.
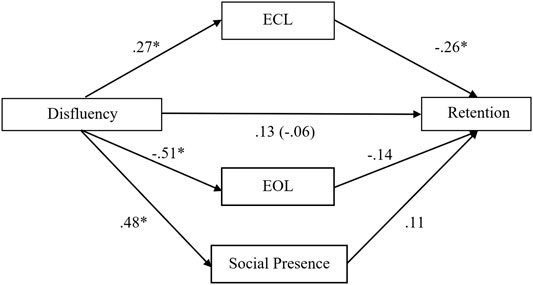
FIGURE 4. Indirect influence of disfluency on retention (β values are displayed; *p < 0.05); Mediators: ECL (extraneous cognitive load), EOL (ease of learning), social presence.
For transfer as dependent variable (see Figure 5), the mediator analysis showed no significant direct effect of disfluency on retention (β = 0.16; SE = 0.10; p = 0.11). When ECL, EOL and social presence were considered as mediator, this effect remained non-significant (β = 0.19; SE = 0.14; p = 0.16). As already outlined, using a handwritten font instead of a computerized font led to a higher ECL (β = 0.27; SE = 0.10; p = 0.01), a lower EOL (β = –0.52; SE = 0.09; p < 0.001) and a higher social presence (β = 0.47; SE = 0.09; p < 0.001). ECL (β = –0.09; SE = 0.12; p = 0.43), social presence (β = 0.03; SE = 0.12; p = 0.22), and EOL (β = 0.04; SE = 0.13; p = 0.77) had no impact on transfer performance.
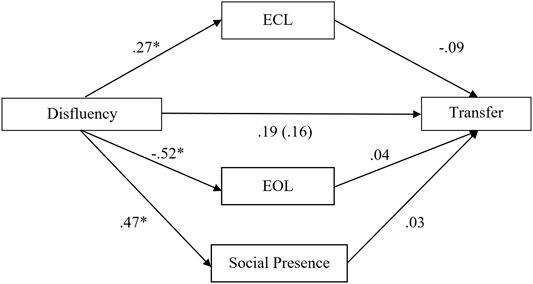
FIGURE 5. Indirect influence of disfluency on transfer (β values are displayed; *p < 0.05); Mediators: ECL (extraneous cognitive load), EOL (ease of learning), social presence.
Discussion
Again, the results are rather ambiguous. In contrast to the previous experiments but in line with the hypotheses, a handwritten font increased ECL. Nevertheless, in Experiment 3, no main effect on learning could be observed but related to the learning time, students in the disfluent condition had a lower retention efficiency (but not transfer efficiency) than students in the fluent condition. Furthermore, in contrast to Experiment 2, participants in the disfluent condition overestimated their learning skills at the beginning and during learning. Even if learners in the disfluent condition had a higher EOL they did not metacognitively adapt their learning strategy to the increased demand through the illegible font. Furthermore, the experienced ECL might have such negative effects that the adaptation was insufficient. An additional hint for this explanation can be derived from the mediation analyses. Even if the mediation analyses detected no direct effect, these effects can be interpreted because the missing direct effect is not a gatekeeper for interpretation (Hayes, 2009). The only significant mediation could be observed concerning ECL and retention. Disfluency enhanced ECL which in consequence reduced retention outcomes. This indicated that disfluency had negative impacts on retention and retention efficiency because of cognitive factors and not because of metacognitive or social factors. Disfluency was, indeed, capable of increasing the perception of social presence but social presence did not influence learning outcomes. Nevertheless, overall, the implications of Experiment 3 are restricted since only mediation effects concerning retention but not transfer could be observed.
General Discussion
Overall, serval differences between the three experiments could be observed. In hypothesis 1, it was assumed that a disfluent font should prevent learners from overestimating their learning performance and foster monitoring processes (Alter et al., 2007; Xie et al., 2018). The results of the current experiments were mixed. Learners in the disfluent condition underestimated their performance in contrast to participants in the fluent condition (Experiment 2). This effect is reversed in Experiment 3. The metacognitive benefits which are postulated to arise from learning with disfluent material cannot be supported in general. In consequence, hypothesis 1 has to be rejected. In hypothesis 2, it was assumed that a disfluent font negatively enhances ECL since additional cognitive resources are required to read and decipher the information (Seufert et al., 2017; Beege et al., 2021). This effect could only be observed in Experiment 3. In Experiment 1 and 2, no effects on ECL occurred. In consequence, hypothesis 2 can only partially be supported. Hypothesis 3 took the social perspective into account. Disfluency operationalized through a handwritten font can trigger social processes and act as a cue for perceived social presence (embodiment principle; Mayer, 2014). Concerning the results of Experiment 3, learners in the handwritten font condition reported higher social presence scores than participants in the computerized font condition. Thus, hypothesis 3 can be supported. Nevertheless, activating a social reaction should also increase cognitive processing and foster learning outcomes (e.g., Mayer et al., 2004; Mayer, 2014). This could not be supported by the data of Experiment 3, since mediation analyses pointed out that social presence had no effects on learning outcomes. Hypothesis 4 dealt with the influence of disfluency on learning outcomes in dependence of the element interactivity of the learning material. It was assumed that either an effect of desirable difficulty (e.g., Diemand-Yauman et al., 2011; Geller et al., 2018), no general effect (e.g., Faber et al., 2017), or a learning inhibiting effect, based on arguments of the CLT (e.g., Lehmann et al., 2016; Xie et al., 2018), could be found in the dependence or the element interactivity. Indeed, the results of the current studies indicate that boundary conditions determine the effectiveness or harmfulness of disfluent learning material. When learning with materials with low element interactivity (Experiment 1), beneficial effects of disfluency on learning outcomes and germane processing could be shown. Nevertheless, further process variables were not investigated in Experiment 1 and thus, further explanations cannot be taken from the data. If the element interactivity increases (Experiment 3), no general effects on learning could be observed. Nevertheless, investigating efficiency revealed that disfluency had detrimental effects on efficiency regarding retention. Overall, inducing ECL through disfluency might have rather suppressing effects on learning when the element interactivity increases. When the element interactivity is high (Experiment 2), disfluency had clearly negative effects on learning and learning efficiency. Thus, implications from the cognitive load perspective might be especially relevant for learning with complex material. Thus, hypothesis 4 could be supported. Nevertheless, it has to be discussed that these explanations have to be viewed with caution. At first, element interactivity was not investigated as a separate experimental factor. In consequence, not only element interactivity but also the learning material as a whole differed between all experiments. This approach was chosen to ensure content equivalence within the single experiments. Nevertheless, this led to the problem, that element interactivity cannot be clearly separated from the effects of the use of different learning materials. Different fields of knowledge might have a crucial influence on processing superficial aspects of the material like the font and in consequence, the written information. Results can thus, give first insights in the effect of element interactivity on learning, but results are rather exploratory and implications can only be drawn with caution. Furthermore, results are ambiguous, even within single experiments. For example, in Experiment 2, disfluency hindered learning performance indicating that disfluent material induced an unproductive load. Nevertheless, no effect on ECL could be observed. Furthermore, in Experiment 3, effects only occurred for retention processes. Transfer and transfer efficiency were not affected through disfluency. In consequence, the complexity of the learning process has to be considered. Rather basal memorization processes seemed to be stronger influenced by superficial structural changes of the learning material. More complex knowledge application processes were not or rather weakly influenced by changes in the legibility of the material.
Implications
On the theoretical side, there is a long and ongoing discussion on the emergence of the disfluency effect (e.g., Rummer et al., 2016; Faber et al., 2017; Geller et al., 2018; İli̇c and Akbulut, 2019). Because of the ambiguous results, researchers need to identify boundary conditions of the emergence of the disfluency effect, for example, the degree of disfluency (Seufert et al., 2017). The current experiments contribute to this discussion by considering element interactivity as moderator. Further, the current investigation operationalized disfluency as a handwritten font to investigate the potential social benefits of illegible fonts. The results of the experiments indicate that the learning fostering as well as learning inhibiting effects are rather based on cognitive factors than on metacognitive or social processes.
On the practical side, designers should be aware that the complexity of the learning material can influence how handwritten fonts are processed. This is especially important in situations where handwritten instructions are heavily used, for example in the classroom or university lecturers when the lecturer draws or writes on the board while teaching. Furthermore, element interactivity is usually medium to high in instructional situations since learners are constantly being thought new information based on previous instructions. Thus, in general, implications from the cognitive load theory should be considered to reduce additional ECL while learning.
Limitations and Future Directions
At first, the handwritten font has to be discussed. For the current study, standard school writing was used to ensure that the font is slightly disfluent but not illegible. Nevertheless, the perception of handwriting can differ in many variables like aesthetics and legibility. Even legibility can arise from many factors like serifs, tilt, or thickness of letters. Thus, it is hardly possible to provide generalized implications for the use of handwritten instructional material. Future studies could specify the effects of different characteristics of handwritten fonts by explicitly manipulate them in experimental studies.
Second, the current studies investigated learning of different materials dealing with different learning domains. In consequence, implications across multiple learning materials can be stated but element interactivity was not investigated with the same learning material restricting comparability. Future studies could explicitly manipulate element interactivity by using one learning material and increase complexity across experimental conditions to further investigate element interactivity as a moderator of the disfluency effect.
Third, the study assessed global metacognitive judgements in order to strengthen economics of the study. Nevertheless, further studies should consider measuring item-by-item judgements, because item-by-item judgements might be more accurate and rely more on metacognitive beliefs (e.g., Bjork et al., 2013).
Finally, the study was carried out with two university students and one young secondary student sample. Yet, handwritten learning materials are heavily used in nearly all educational stages. Thus, investigating primary school students and older secondary school students is important to further specify the role of handwritten materials on learning processes.
Data Availability Statement
The raw data supporting the conclusions of this article will be made available by the authors, without undue reservation.
Ethics Statement
Ethical review and approval was not required for the study on human participants in accordance with the local legislation and institutional requirements. Written informed consent to participate in this study was provided by the participants’ legal guardian/next of kin.
Author Contributions
MB: Conceptualization; Methodology; Investigation; Data Curation; Formal Analysis; Validation; Project Administration; Writing - Original Draft FK: Writing - Original Draft; Data Curation; Writing - Review & Editing SS: Writing - Review & Editing SN: Writing - Review & Editing GR: Writing - Review & Editing; Supervision.
Funding
The article processing charge was funded by the Baden-Württemberg Ministry of Science, Research and Culture and the University of Education, Freiburg in the funding programme Open Access Publishing.
Conflict of Interest
The authors declare that the research was conducted in the absence of any commercial or financial relationships that could be construed as a potential conflict of interest.
Publisher’s Note
All claims expressed in this article are solely those of the authors and do not necessarily represent those of their affiliated organizations, or those of the publisher, the editors and the reviewers. Any product that may be evaluated in this article, or claim that may be made by its manufacturer, is not guaranteed or endorsed by the publisher.
Supplementary Material
The Supplementary Material for this article can be found online at: https://www.frontiersin.org/articles/10.3389/feduc.2021.678798/full#supplementary-material
References
Alter, A. L., Oppenheimer, D. M., Epley, N., and Eyre, R. N. (2007). Overcoming Intuition: Metacognitive Difficulty Activates Analytic Reasoning. J. Exp. Psychol. Gen. 136, 569–576. doi:10.1037/0096-3445.136.4.569
Alter, A. L., and Oppenheimer, D. M. (2009). Uniting the Tribes of Fluency to Form a Metacognitive Nation. Pers Soc. Psychol. Rev. 13, 219–235. doi:10.1177/1088868309341564
Atkinson, R. C., and Shiffrin, R. M. (1968). Human Memory: A Proposed System and its Control Processes, Academic Press. Psychol. Learn. Motiv. 2, 89–195. doi:10.1016/s0079-7421(08)60422-3
Bailenson, J. N., Aharoni, E., Beall, A. C., Guadagno, R. E., Dimov, A., and Blascovich, J. (2004). “Comparing Behavioral and Self-Report Measures of Embodied Agents’ Social Presence in Immersive Virtual Environments,” in Proceedings of the 7th Annual International Workshop on PRESENCE, Valencia, Spain, October 13–15, 2004, 1864–1105.
Beege, M., Nebel, S., Schneider, S., and Rey, G. D. (2021). The Effect of Signaling in Dependence on the Extraneous Cognitive Load in Learning Environments. Cogn. Process. 22 (2), 209–225. doi:10.1007/s10339-020-01002-5
Bjork, R. A., Dunlosky, J., and Kornell, N. (2013). Self-regulated Learning: Beliefs, Techniques, and Illusions. Annu. Rev. Psychol. 64, 417–444. doi:10.1146/annurev-psych-113011-143823
Bjork, R. A. (2013). “Desirable Difficulties Perspective on Learning,” in Encyclopedia of the Mind. Editor H. Pashler (Thousand Oaks, CA: Sage Publication), 134–146.
Burton, R. F. (2005). Multiple‐choice and True/false Tests: Myths and Misapprehensions. Assess. Eval. Higher Educ. 30, 65–72. doi:10.1080/0260293042003243904
De Jong, T. (2010). Cognitive Load Theory, Educational Research, and Instructional Design: Some Food for Thought. Instr. Sci. 38, 105–134. doi:10.1007/s11251-009-9110-0
Diemand-Yauman, C., Oppenheimer, D. M., and Vaughan, E. B. (2011). Fortune Favors the Bold (And the Italicized): Effects of Disfluency on Educational Outcomes. Cognition 118, 111–115. doi:10.1016/j.cognition.2010.09.012
Dinsmore, D. L., and Parkinson, M. M. (2013). What Are Confidence Judgments Made of? Students' Explanations for Their Confidence Ratings and what that Means for Calibration. Learn. Instruction 24, 4–14. doi:10.1016/j.learninstruc.2012.06.001
Eitel, A., and Kühl, T. (2016). Effects of Disfluency and Test Expectancy on Learning with Text. Metacognition Learn. 11, 107–121. doi:10.1007/s11409-015-9145-3
Eitel, A., Kühl, T., Scheiter, K., and Gerjets, P. (2014). Disfluency Meets Cognitive Load in Multimedia Learning: Does Harder-To-Read Mean Better-To-Understand? Appl. Cognit. Psychol. 28, 488–501. doi:10.1002/acp.3004
Faber, M., Mills, C., Kopp, K., and D'Mello, S. (2017). The Effect of Disfluency on Mind Wandering during Text Comprehension. Psychon. Bull. Rev. 24, 914–919. doi:10.3758/s13423-016-1153-z
Geller, J., Still, M. L., Dark, V. J., and Carpenter, S. K. (2018). Would Disfluency by Any Other Name Still Be Disfluent? Examining the Disfluency Effect with Cursive Handwriting. Mem. Cognit 46, 1109–1126. doi:10.3758/s13421-018-0824-6
Hartel, M. J., Staub, L. P., Röder, C., and Eggli, S. (2011). High Incidence of Medication Documentation Errors in a Swiss university Hospital Due to the Handwritten Prescription Process. BMC Health Serv. Res. 11, 1–16. doi:10.1186/1472-6963-11-199
Hayes, A. F. (2009). Beyond Baron and Kenny: Statistical Mediation Analysis in the New Millennium. Commun. Monogr. 76, 408–420. doi:10.1080/03637750903310360
Hayes, A. F. (2017). Introduction to Mediation, Moderation, and Conditional Process Analysis: A Regression-Based Approach. New York, NY: Guilford publications.
Higham, P. A., and Arnold, M. M. (2007). Beyond Reliability and Validity: The Role of Metacognition in Psychological Testing. New Dev. Psychol. Test., 139–162. doi:10.1016/j.jmpt.2009.06.003
İli̇c, U., and Akbulut, Y. (2019). Effect of Disfluency on Learning Outcomes, Metacognitive Judgments and Cognitive Load in Computer Assisted Learning Environments. Comput. Hum. Behav. 99, 310–321. doi:10.1016/j.chb.2019.06.001
Ito, R., Hamano, K., Nonaka, K., Sugano, I., Nakamura, S., Kake, A., et al. (2020). “Comparison of the Remembering Ability by the Difference between Handwriting and Typeface,” in International Conference on Human-Computer Interaction, Copenhagen, Denmark, July 19–24, 2020 (Cham: Springer), 526–534. doi:10.1007/978-3-030-50726-8_69
James, W. D. (1950). The Principles of Psychology. New York, NY: Dover. Original work published 1890.
Jiang, D., and Kalyuga, S. (2020). Confirmatory Factor Analysis of Cognitive Load Ratings Supports a Two-Factor Model. Tqmp 16, 216–225. doi:10.20982/tqmp.16.3.p216
Kalyuga, S. (2011). Cognitive Load Theory: How Many Types of Load Does it Really Need?. Educ. Psychol. Rev. 23 (1), 1–19. doi:10.1007/s10648-010-9150-7
Kahneman, D., and Frederick, S. (2002). “Representativeness Revisited: Attribute Substitution in Intuitive Judgment,” in Heuristics and Biases. Editors T. Gilovich, D. Griffin, and D. Kahneman (Cambridge: Cambridge University Press), 49–81. doi:10.1017/cbo9780511808098.004
Klepsch, M., Schmitz, F., and Seufert, T. (2017). Development and Validation of Two Instruments Measuring Intrinsic, Extraneous, and Germane Cognitive Load. Front. Psychol. 8, 1997. doi:10.3389/fpsyg.2017.01997
Klepsch, M., and Seufert, T. (2020). Understanding Instructional Design Effects by Differentiated Measurement of Intrinsic, Extraneous, and Germane Cognitive Load. Instr. Sci. 48, 45–77. doi:10.1007/s11251-020-09502-9
Kühl, T., Eitel, A., Damnik, G., and Körndle, H. (2014). The Impact of Disfluency, Pacing, and Students' Need for Cognition on Learning with Multimedia. Comput. Hum. Behav. 35, 189–198. doi:10.1016/j.chb.2014.03.004
Kühl, T., and Eitel, A. (2016). Effects of Disfluency on Cognitive and Metacognitive Processes and Outcomes. Metacognition Learn. 11, 1–13. doi:10.1007/s11409-016-9154-x
Lehmann, J., Goussios, C., and Seufert, T. (2016). Working Memory Capacity and Disfluency Effect: an Aptitude-Treatment-Interaction Study. Metacognition Learn. 11, 89–105. doi:10.1007/s11409-015-9149-z
Leppink, J., Paas, F., Van Gog, T., van Der Vleuten, C. P. M., and van Merriënboer, J. J. G. (2014). Effects of Pairs of Problems and Examples on Task Performance and Different Types of Cognitive Load. Learn. Instruction 30, 32–42. doi:10.1016/j.learninstruc.2013.12.001
Mayer, R. E., Fennell, S., Farmer, L., and Campbell, J. (2004). A Personalization Effect in Multimedia Learning: Students Learn Better when Words Are in Conversational Style rather Than Formal Style. J. Educ. Psychol. 96, 389–395. doi:10.1037/0022-0663.96.2.389
Mayer, R. E., Sobko, K., and Mautone, P. D. (2003). Social Cues in Multimedia Learning: Role of Speaker's Voice. J. Educ. Psychol. 95, 419–425. doi:10.1037/0022-0663.95.2.419
Mayer, R. E. (2014). The Cambridge Handbook of Multimedia Learning. 2 ed. Cambridge, MA: Cambridge University Press.
McLaren, B. M., DeLeeuw, K. E., and Mayer, R. E. (2011). A Politeness Effect in Learning with Web-Based Intelligent Tutors. Int. J. Human-Computer Stud. 69, 70–79. doi:10.1016/j.ijhcs.2010.09.001
Moreno, R., Mayer, R. E., Spires, H. A., and Lester, J. C. (2001). The Case for Social agency in Computer-Based Teaching: Do Students Learn More Deeply when They Interact with Animated Pedagogical Agents? Cogn. Instruction 19, 177–213. doi:10.1207/S1532690XCI1902_02
Moreno, R., and Mayer, R. (2007). Interactive Multimodal Learning Environments. Educ. Psychol. Rev. 19, 309–326. doi:10.1007/s10648-007-9047-2
Nelson, T. O., and Leonesio, R. J. (1988). Allocation of Self-Paced Study Time and the "Labor-In-Vain Effect". J. Exp. Psychol. Learn. Mem. Cogn. 14, 676–686. doi:10.1037//0278-7393.14.4.676
Nelson, T. O., and Narens, L. (1990). “Metamemory: A Theoretical Framework and New Findings,” in The Psychology of Learning and Motivation. Editor G. H. Bower (Cambridge: Academic Press), 125–173. doi:10.1016/s0079-7421(08)60053-5
Paas, F., and Sweller, J. (2014). “Implications of Cognitive Load Theory,” in The cambridge Handbook of Multimedia Learning. Editor R. E. Mayer (Cambridge: Cambridge University Press), 27–43.
Paas, F., Tuovinen, J. E., Tabbers, H., and Van Gerven, P. W. M. (2003). Cognitive Load Measurement as a Means to advance Cognitive Load Theory. Educ. Psychol. 38, 63–71. doi:10.1207/S15326985EP3801_8
Pashler, H., Rohrer, D., Cepeda, N. J., and Carpenter, S. K. (2007). Enhancing Learning and Retarding Forgetting: Choices and Consequences. Psychon. Bull. Rev. 14, 187–193. doi:10.3758/BF03194050
Pieger, E., Mengelkamp, C., and Bannert, M. (2016). Metacognitive Judgments and Disfluency - Does Disfluency lead to More Accurate Judgments, Better Control, and Better Performance? Learn. Instruction 44, 31–40. doi:10.1016/j.learninstruc.2016.01.012
Reeves, B., and Nass, C. I. (1996). The media Equation: How People Treat Computers, Television, and New media like Real People and Places. Cambridge: Cambridge University Press.
Rummer, R., Schweppe, J., and Schwede, A. (2016). Fortune Is Fickle: Null-Effects of Disfluency on Learning Outcomes. Metacognition Learn. 11, 57–70. doi:10.1007/s11409-015-9151-5
Schneider, S., Beege, M., Nebel, S., and Rey, G. D. (2018). Theoretischer Beitrag: Soziale Prozesse beim Lernen mit digital präsentierten Lernmaterialien. Psychol. Erziehung Unterricht 65, 257–274. doi:10.2378/peu2018.art09d
Seufert, T., Wagner, F., and Westphal, J. (2017). The Effects of Different Levels of Disfluency on Learning Outcomes and Cognitive Load. Instr. Sci. 45, 221–238. doi:10.1007/s11251-016-9387-8
Son, L. K., and Kornell, N. (2008). “Research on the Allocation of Study Time: Key Studies from 1890 to the Present (And beyond),” in Handbook of Metamemory and Memory. Editors J. Dunlosky, and R. A. Bjork (Hove: Psychology Press), 333–351.
Strukelj, A., Scheiter, K., Nyström, M., and Holmqvist, K. (2016). Exploring the Lack of a Disfluency Effect: Evidence from Eye Movements. Metacognition Learn. 11, 71–88. doi:10.1007/s11409-015-9146-2
Sweller, J. (1988). Cognitive Load during Problem Solving: Effects on Learning. Cogn. Sci. 12, 257–285. doi:10.1016/0364-0213(88)90023-710.1207/s15516709cog1202_4
Sweller, J. (1994). Cognitive Load Theory, Learning Difficulty, and Instructional Design. Learn. Instruction 4, 295–312. doi:10.1016/0959-4752(94)90003-5
Sweller, J. (2010). “Cognitive Load Theory: Recent Theoretical Advances,” in Cognitive Load Theory. Editor J. L. Plass, R. Moreno, and R. Brünken. Cambridge University Press, 29–47. doi:10.1017/CBO9780511844744.004
Sweller, J., van Merriënboer, J. J. G., and Paas, F. (2019). Cognitive Architecture and Instructional Design: 20 Years Later. Educ. Psychol. Rev. 31, 261–292. doi:10.1007/s10648-019-09465-5
Sweller, J., Van Merrienboer, J. J. G., and Paas, F. G. W. C. (1998). Cognitive Architecture and Instructional Design. Educ. Psychol. Rev. 10, 251–296. doi:10.1023/A:1022193728205
Thompson, V. A., Turner, J. A., Pennycook, G., Ball, L. J., Brack, H., Ophir, Y., et al. (2013). The Role of Answer Fluency and Perceptual Fluency as Metacognitive Cues for Initiating Analytic Thinking. Cognition 128, 237–251. doi:10.1016/j.cognition.2012.09.012
Van Gog, T., and Paas, F. (2008). Instructional Efficiency: Revisiting the Original Construct in Educational Research. Educ. Psychol. 43, 16–26. doi:10.1080/00461520701756248
Xie, H., Zhou, Z., and Liu, Q. (2018). Null Effects of Perceptual Disfluency on Learning Outcomes in a Text-Based Educational Context: A Meta-Analysis. Educ. Psychol. Rev. 30, 745–771. doi:10.1007/s10648-018-9442-x
Keywords: disfluency, handwritten font, metacognition, element interactivity, social presence
Citation: Beege M, Krieglstein F, Schneider S, Nebel S and Rey GD (2021) Is There a (Dis-)Fluency Effect in Learning With Handwritten Instructional Texts? Evidence From Three Studies. Front. Educ. 6:678798. doi: 10.3389/feduc.2021.678798
Received: 10 March 2021; Accepted: 24 November 2021;
Published: 14 December 2021.
Edited by:
Giseli Donadon Germano, São Paulo State University, BrazilCopyright © 2021 Beege, Krieglstein, Schneider, Nebel and Rey. This is an open-access article distributed under the terms of the Creative Commons Attribution License (CC BY). The use, distribution or reproduction in other forums is permitted, provided the original author(s) and the copyright owner(s) are credited and that the original publication in this journal is cited, in accordance with accepted academic practice. No use, distribution or reproduction is permitted which does not comply with these terms.
*Correspondence: Maik Beege, bWFpay5iZWVnZUBwaC1mcmVpYnVyZy5kZQ==