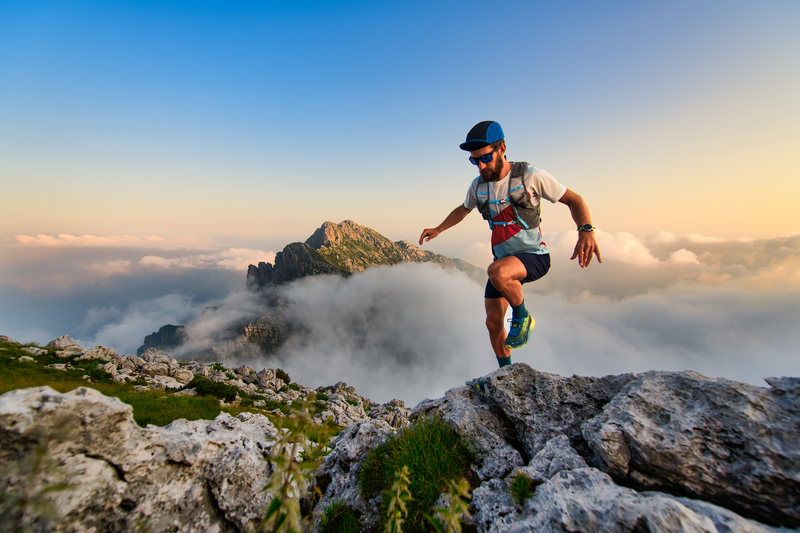
94% of researchers rate our articles as excellent or good
Learn more about the work of our research integrity team to safeguard the quality of each article we publish.
Find out more
PERSPECTIVE article
Front. Clim. , 18 December 2024
Sec. Climate and Decision Making
Volume 6 - 2024 | https://doi.org/10.3389/fclim.2024.1499765
This article is part of the Research Topic Concept Papers from the World Climate Research Programme: The Future of Climate Research View all 10 articles
The World Climate Research Programme (WCRP) envisions a future where actionable climate information is universally accessible, supporting decision makers in preparing for and responding to climate change. In this perspective, we advocate for enhancing links between climate science and decision-making through a better and more decision-relevant understanding of climate impacts. The proposed framework comprises three pillars: climate science, impact science, and decision-making, focusing on generating seamless climate information from sub-seasonal, seasonal, decadal to century timescales informed by observed climate events and their impacts. The link between climate science and decision-making has strengthened in recent years, partly owing to undeniable impacts arising from disastrous weather extremes. Enhancing decision-relevant understanding involves utilizing lessons from past extreme events and implementing impact-based early warning systems to improve resilience. Integrated risk assessment and management require a comprehensive approach that encompasses good knowledge about possible impacts, hazard identification, monitoring, and communication of risks while acknowledging uncertainties inherent in climate predictions and projections, but not letting the uncertainty lead to decision paralysis. The importance of data accessibility, especially in the Global South, underscores the need for better coordination and resource allocation. Strategic frameworks should aim to enhance impact-related and open-access climate services around the world. Continuous improvements in predictive modeling and observational data are critical, as is ensuring that climate science remains relevant to decision makers locally and globally. Ultimately, fostering stronger collaborations and dedicated investments to process and tailor climate data will enhance societal preparedness, enabling communities to navigate the complexities of a changing climate effectively.
Global warming is causing a wide range of weather and climate extremes to become more frequent, intense and widespread (Seneviratne et al., 2021). Every region of the world is experiencing changes in climatic impact-drivers, such as heatwaves, floods, droughts, fire weather, sea level rise, and storm surges (IPCC AR6 2021, 2022). Climate change will continue to cause unprecedented extreme events, such as record-breaking heat waves (Fischer et al., 2021), and will lead to increases in the occurrence of compound events (Raymond et al., 2022). We are thus challenged with “imagining the unimaginable” for better risk preparedness and management.
The World Climate Research Programme (WCRP) envisions a future in which actionable climate information is available for all regions of the world, with climate services providing decision makers with tools enabling them to prepare for, respond to, and build resilience to climate change alongside reducing anthropogenic emissions. Weather and climate information is crucial for climate risk management, as well as for adaptation and mitigation, and needs to be made (or remain) accessible for everyone. The quickly evolving field of climate services is concerned with making climate information accessible and useful to decision makers (Jacob et al., 2024), but there is no one-size-fits-all approach to climate services. Context, user involvement, and collaboration among scientists, practitioners, and users determine the type and usefulness of climate services for adaptation, mitigation, and risk management (Ranasinghe et al., 2021).
Around the time of the first Intergovernmental Panel on Climate Change (IPCC) report (early 1990s) climate science was a relatively young field, with a focus on global scales and far-distant time horizons (e.g., global mean temperature projections out to 2,100 or 2,300). While useful for some [e.g., in the context of sea level rise (van Dorland et al., 2023; van der Wiel et al., 2024)], these temporal and spatial scales exceed the scope and directives of many decision makers. In the last decade, climate science has evolved substantially to also include regional climate change, climate extremes and their attribution, and has advanced in near-to-medium term climate predictions (IPCC, 2021). The severe and widespread impacts of climate extremes in recent years have alerted many decision makers to the topic of climate change and piqued their interest in becoming better prepared for (or adapted to) the consequences of climate change, but actual action in terms of proactive adaptation and mitigation at the scale needed is limited (e.g., Schleussner et al., 2024; Theokritoff et al., 2023; Thomas et al., 2021).
Here we focus on aspects that could improve the links between recent advances in climate science and decision-making through a better and more decision-relevant understanding of climate-related impacts (Figure 1). Impacts, such as damaged infrastructure, lost lives, destroyed ecosystems or livelihoods, describe the actual consequences of climate change that have already occurred or are currently happening, so basically the realization of risk (IPCC, 2022). The framework we present here is based on three main pillars: climate and climate science, impacts and impact science, and decision-making. We argue that climate-resilient pathways can be established through processes enabling strong connections between, and collaboration across, these three pillars. This will include seamless climate information based on learning from past impacts, impact-based early warning systems, and climate prediction and projections of relevant climatic impact-drivers. Societal considerations of resilience in the face of a changing climate imply that decision-making should be front and center, and that more robust adaptation and mitigation efforts need to happen than those we see today.
Figure 1. Illustration of linking climate science and decision-making via the impacts of climate change. Three conceptual pillars form a foundation on which climate-resilient pathways are built: (i) climate science, which provides a body of knowledge and understanding of the climate system; (ii) impact sciences and a robust understanding of climatic impact-drivers; and (iii) the decision-making sphere, where a synthesis of relevant climate science data and understanding of the impacts inform action. The three pillars interact (arrows) via physical effects, transfer of learnings, and implementation of decisions.
Climatic impact-drivers (including climate extremes), or hazards in the context of risk assessments, must be connected to their respective impacts on society and ecosystems (Ruane et al., 2022). Understanding how climatic changes lead to impacts is necessary to support decision-making (e.g., heat or flood warnings, forecasts and warnings for tropical cyclones, agricultural decisions supported by sub-seasonal to seasonal predictions). A better-informed risk assessment through advances in climate science and impact science will contribute to adaptation and response strategies for climate-resilient development (IPCC, 2022). Depending on the vulnerability of populations, economic sectors or assets, impacts can be totally different for the same climatic impact-driver (e.g., in different countries or for different groups of people) or the same forecast (e.g., Tradowsky et al., 2023). Multiple climatic impact-drivers, such as extreme heat, floods, droughts and compounding events, can affect a specific sector, such as health, agriculture or infrastructure, but also specific climatic impact-drivers affect multiple sectors in unique ways (Ranasinghe et al., 2021). Moreover, impacts can also cascade across sectors and regions (Simpson et al., 2021, 2023). These compounding and cascading events make risks more complex and difficult to manage (IPCC, 2022).
For better preparedness, we can gain a lot of knowledge from current and past impacts of climate extremes and how we responded to them [e.g., through disaster forensics (Keating et al., 2016)], thus informing our decision-making related to possible future impacts. Historical observations of previous extreme events, their impacts and the translation into a warmer climate, can provide valuable insights into the types of event that could be experienced today and in the near future (e.g., Hawkins et al., 2023a; Hegdahl et al., 2020). A review of a variety of approaches to constructing such physical climate storylines can be found in Baldissera Pacchetti et al. (2023). Also, in the field of extreme events attribution, which focuses mainly on the probability of a hazard in a world with and without human-induced climate change, the storyline approach can provide some insight in the thermodynamic and dynamic processes that contributed to a past extreme event (Shepherd, 2016). However, even for large-scale heatwaves, for which event attribution seems rather straightforward, this method is challenged by the limited ability of climate models to represent relevant local processes (e.g., moisture budget, land interactions) and natural variability (Shepherd, 2016; van Oldenborgh et al., 2022). In recent years, event attribution has also been applied to impacts, with considerable challenges, but also opportunities for this emerging field, as elaborated in Perkins-Kirkpatrick et al. (2024).
Ideally, we generate seamless information over time, meaning that we combine what we have learned from the past with climate predictions or projections to inform impact forecasts tailored to specific decision makers or contexts. In this way, as new or refined insights become available, they can inform decision-making as part of adaptive pathways planning (Haasnoot et al., 2018). Such insights could include the time of emergence in climate scenarios or the time that a critical threshold is reached (e.g., Slangen et al., 2022). As time goes on, the situation can be reviewed and new decisions can be made. When this is part of an adaptive pathways plan, decision-relevant information can be identified which can be monitored for signals of change (e.g., climate changing faster/slower, adaptation thresholds being reached in the near-term). This includes accounting for and imagining high-impact low-likelihood outcomes associated with unprecedented climate extremes or compound events, which need to be included in stress-testing and storylines to support risk assessments (e.g., Sillmann et al., 2021a; IPCC, 2021).
Climate predictions and projections can provide the information needed to underpin decision-making or deliver early warning systems. Climate projections can inform adaptation and mitigation planning about medium to long-term future climate change (e.g., Zhang et al., 2024), while climate predictions can provide useful information on the sub-seasonal to decadal time scale. In particular, national climate projections provided by National Meteorological and Hydrological Services (NMHS) are often prepared with a focus on specific use cases of decision-making on adaptation and mitigation (e.g., Lowe et al., 2018; Fischer et al., 2022). To connect the different timescales, it is thus important to have both early warning systems (which predict specific events or climate conditions that are often context and place specific) and forewarning systems (which indicate the growing potential of future climate risks that may necessitate proactive planning; e.g., Haasnoot et al., 2018). Although both systems may be equipped to inform responses to the same type of extreme event (e.g., a flood), in the early warning case decision makers are under immediate pressure to respond based on the response options available, while in the latter case decision makers may consider what adaptation measures they could plausibly develop to be equipped for future responses and to build long-term resilience. Forewarning may also be linked with foresight analyses that link changes in climatic impact-drivers with shifts in vulnerability and exposure (via scenarios or storylines; e.g., Findell et al., 2023) for more comprehensive risk assessments. Ideally, both would go hand-in-hand with seamless data and information flows and opportunities for iterations and learning to support decision-making under uncertainty.
As Earth’s climate is subject to chaotic characteristics and climate models and prediction systems are not perfect, decisions need to be made under uncertainty (e.g., Cross Chapter Box DEEP in New et al., 2022). The uncertainty and usefulness of climate predictions can partly be quantified by forecast quality evaluations (e.g., Delgado-Torres et al., 2022; Meehl et al., 2021). However, the skill of predictions also varies in time related to the phase of large-scale climate drivers, which can also provide so-called “windows of opportunity” (Borchert et al., 2019a; Sgubin et al., 2021; Mariotti et al., 2020; Liu et al., 2023). For instance, we know that the state of the El Niño-Southern Oscillation can be predicted several months in advance, and its consequences, such as changes in rainfall patterns and increases in the likelihood of extreme events, are well known for many regions, as was illustrated for the 2022 Pakistan Floods by Dunstone et al. (2023). Furthermore, skillful predictions of North Atlantic sea surface temperature may enable predictions of hot European summers up to a decade ahead (Borchert et al., 2019b).
The impact of any climate impact-driver relates to the driver itself (the hazard), as well as exposure and vulnerability of the affected system. We argue that impact understanding and modeling are the connector between climate science and decision-making. Using past impacts and iterative knowledge gained through experience, combined with predictions and projections of hazard, exposure, and vulnerability changes, and taking uncertainty into account, decisions can be tailored to balance risk and impact for each individual circumstance. We outline challenges in climate sciences, impact sciences, and decision making below.
Several decades of climate science provide a robust understanding of how and why global climate is changing, and this knowledge can underpin action and decisions (Forster et al., 2023; IPCC, 2023). However, numerous challenges related to physical climate and impact sciences remain, and hinder the efficient production and uptake of climate information in decision-making. In this section we discuss these challenges with respect to the current state of knowledge, separated into the physical climate science aspects, climate impacts, and the relevance for decision-making.
Key challenges in the physical climate sciences are here divided into data-related challenges, process understanding and representation in models, and prediction and projection of changes.
Data coverage and gaps and access to data remain consistent challenges, particularly for understanding and analysis of climate extremes (Sillmann et al., 2017). The nature of the challenge depends on the climate extreme being investigated, its occurrence frequency, spatial size and distribution, and ease of observation. Generally, there is a lack of data for the Global South (e.g., Dunn et al., 2020), with research resources often missing for climate risk assessments (Otto et al., 2020a; Seneviratne et al., 2021). However, space-based data and technologies have made significant contributions in addressing these challenges, providing critical data and insights that can enhance our understanding and management of climate change impacts (Yang et al., 2013; Alexander et al., 2020; CEOS, 2023). The latter, however, should not be seen as a replacement for other forms of observations, especially in situ measurements. Data rescue and recovery, for example of non-digitized past records, can play an important role in calculating and assessing past trends and defining extreme events (Hawkins et al., 2023b). In addition, human observations (e.g., narratives) can complement instrumental records of climate extremes in regions with sparse data (Savo et al., 2024). Also, Artificial Intelligence methods are showing promising potential in infilling sparse observational data and revealing past climate extremes consistent with proxy reports or narratives (e.g., Plésiat et al., 2024). Historical records are also useful for providing context to contemporary extremes (Yule et al., 2023). Development of plausible extreme-event scenarios based on combinations of model outputs and historical records could increase scientific defensibility for emergency preparedness and planning (Dettinger et al., 2012; Huang and Swain, 2022).
Some relevant processes are still insufficiently understood, and climate models have known shortcomings in representing these relevant processes. Here we consider process representation in models, but it is worth noting that some processes related to hazards, such as surface hydrology or geomorphology, are not represented at all in meteorological or climate models (Senatore et al., 2015). Some key processes related to climate extremes have spatial scales smaller than typical model resolutions and must often be parameterized (Seneviratne et al., 2021). Representation of extremes in models depends on the accuracy of such parameterizations (e.g., Kong et al., 2022), as well as model resolution (e.g., Rowell and Berthou, 2023) and physical process understanding (Seneviratne et al., 2021). Increases in computational power have led to the availability of higher-resolution, convection-permitting simulations with benefits particularly for precipitation extremes (Kendon et al., 2021; Poschlod, 2021). The ability to run climate models at kilometer resolution represents a significant advance with wide-ranging benefits but also challenges (Schär et al., 2020; Prein et al., 2021). This being said, process understanding gaps related to extremes remain (Seneviratne et al., 2021) in areas including the scaling with temperature of short-duration and high-intensity rainfall events (Fowler et al., 2021), severe storms (Allen, 2018), and compound extreme events which can exacerbate impacts (Zscheischler et al., 2020; Poschlod et al., 2020). Increases in computational power have also enabled the creation of single model initial-condition large ensembles, which allow for a robust sampling of univariate and compound extremes (Bevacqua et al., 2023; Lang and Poschlod, 2024) and show the effect of internal climate variability on climate extremes (Suarez-Gutierrez et al., 2020; Poschlod et al., 2021). Other recent advances in process understanding and model representation have covered a wide variety of topics, including tropical-extratropical interactions (e.g., Zilli et al., 2023), ocean-land-atmosphere interactions (e.g., Dehondt et al., 2024; Freisen et al., 2022; Duan et al., 2023), extreme heat and drought in selected regions (e.g., Baier and Stohl, 2023; Sun et al., 2022), monsoon rains (e.g., Menon et al., 2022; Vg et al., 2023), wildfires (e.g., Charlton et al., 2022; Son et al., 2024), atmospheric convection (e.g., Bony et al., 2020; Klein et al., 2023; Nkrumah et al., 2023; Prein et al., 2021), and jets and eddies in the atmosphere (e.g., Garfinkel et al., 2024) and ocean (Gutierrez-Villanueva et al., 2023). There have also been improvements in understanding tipping points, which are rapid and/or irreversible changes in the climate system (Lee et al., 2021) and their interactions (Franzke et al., 2022). However, further understanding of the underlying nonlinear mechanisms is needed (Boers et al., 2022), and robust assessment of nonlinear processes requires longer observational data sets than linear processes (Findell et al., 2015). Overall, linking our understanding of climate extremes, their characteristics, and their changes, to drivers, feedbacks and compound characteristics, remains a challenge.
Predicted and projected changes in extremes, and scientific confidence in the changes, vary by region and event type, with thermodynamic changes generally better understood than dynamic changes (Seneviratne et al., 2021). Projections with relatively higher confidence include those for temperature-related extremes, drought, and fire weather in many regions, while differences between event scales and model resolutions and uncertainties in parametrizations lead to relatively lower confidence in extremes including severe storms, and heavy snowfall (Ranasinghe et al., 2021). Artificial intelligence (AI) and machine learning (ML) have opened new modeling opportunities, with ML methods used for the prediction and attribution of extremes (Reichstein et al., 2019; Salcedo-Sanz et al., 2024), and the skill of some ML-based models in sub-seasonal prediction comparable to dynamic prediction (Li et al., 2024). However, traditional Numerical Weather Prediction (NWP) models still perform better for some extreme events, especially when considering metrics for issuing warnings (Charlton-Perez et al., 2024). Ensembles of moderately high-resolution climate simulations combined with AI/ML, built on domain-specific knowledge, have been championed as an effective approach to advancing climate prediction and projection (Schneider et al., 2023) as well as early warning (Reichstein et al., 2024). One challenge is that we may need to anticipate events that have hitherto been considered implausible. Approaches that go beyond statistical assessments and standard climate model ensemble projections may be required. Such approaches include physical climate storylines (Baldissera Pacchetti et al., 2023; Doblas-Reyes et al., 2021; Harvey et al., 2023; Maraun et al., 2022; Sánchez-Benítez et al., 2022; Seneviratne et al., 2021; Hegdahl et al., 2020), iterative decision-making processes (New et al., 2022), and the UNSEEN approach (Thompson et al., 2017; Kelder et al., 2020).
Understanding the full extent of climate change impacts is complicated due to the complexity and uncertainty inherent in the climate system and their interactions with ecosystems and human societies (IPCC, 2022). Climate change impacts vary widely across regions and contexts, making it challenging to develop generalizable assessments that accurately capture the diverse range of impacts experienced globally. Furthermore, limited availability of high-quality impacts data, particularly in regions with inadequate monitoring infrastructure, poses challenges for assessing current and future impacts of climate change (e.g., Vicedo-Cabrera et al., 2021). In the last decade, there have been substantial advances in impact attribution (Hansen and Stone, 2016; Lloyd and Shepherd, 2020; Perkins-Kirkpatrick et al., 2022) and impact assessments highlighting the widespread and profound impacts of climate change across various regions and sectors, including ecosystems, water resources, agriculture, human health, and infrastructure (IPCC, 2022). In their systematic review of advances in extreme event attribution, Perkins-Kirkpatrick et al. (2024) conclude that there is a challenge and need for coordinated interdisciplinary and transdisciplinary efforts to advance impact attribution assessments and their broader applicability. We take that conclusion one step further, highlighting the need for tighter collaboration between climate science and impact science for climate-savvy decision-making.
Using a variety of data sources can help form a better picture of extreme events and their impacts: for example, observations and reanalyses can be complemented by community data (e.g., Fox et al., 2023) and social media and citizen-run application data can provide impact information (e.g., Young et al., 2022). We note however that data homogeneity and quality can be adversely affected when combining (often temporally short) data from different sources and attention is required to ensure the data are meaningful in a climate context. Climatic impact-drivers must be combined with vulnerability and exposure information to properly assess climate risks (IPCC, 2022; Seneviratne et al., 2021), yet collecting such data at sufficient temporal and spatial resolutions is difficult.
Reporting on impacts may be spatially biased (Harrington and Otto, 2020) or delayed following extreme events, and disentangling individual impacts becomes challenging when multiple extremes occur simultaneously. These complexities underscore the need for comprehensive, timely, and contextually appropriate impact data to inform effective adaptation strategies (Otto et al., 2020b). Identifying and prioritizing adaptation measures to address the impacts of climate change requires a nuanced understanding of local vulnerabilities and adaptation capacities, which may vary widely across different communities, sectors and regions. Additional challenges for impact assessment and decision-making concern equitable and just distribution of adaptation resources and addressing the disproportionate impacts of climate change on vulnerable populations (IPCC, 2022). An example of a practical response to these challenges is the Brazilian adaptation platform illustrating how climatic and non-climatic factors are interrelated in generating risks to society, providing a comprehensive tool for decision makers and the public (Alves et al., 2022).
Integrating climate change impacts across different sectors and regions to provide comprehensive assessments requires interdisciplinary collaboration and the synthesis of diverse sources of information (IPCC, 2022). Generally, impacts depend on individual-, system- or sector-specific thresholds (e.g., heat tolerance thresholds in humans, plants or infrastructure) (Ruane et al., 2022). Hence, impact data need to be contextual, ensuring accurate representation of systems and the translation of biophysical or engineering impacts into socioeconomic or health-related impacts (e.g., Schwingshackl et al., 2021). A further challenge is the myriads of methods and models used to capture and analyze impacts across different sectors and climatic impact-drivers (e.g., Sillmann et al., 2021b). Including state-of-the-art climate model data into impact models to assess changes in impacts due to climate change, particularly related to climate extremes, is often not straightforward and requires better and sustained collaboration between the climate and impact science communities (e.g., Schewe et al., 2019). Large efforts are made in the Inter-Sectoral Impact Model Intercomparison Project (ISIMIP) to provide a framework for ensemble modeling of climate change impacts and impact attribution for various sectors (e.g., health, agriculture) across the world (Frieler et al., 2024).
There is increasing recognition of the urgent need to bridge the gap between physical climate science and decision-making (e.g., ARC Centre of Excellence for Climate Extremes, 2023; Galford et al., 2016; von Winterfeldt, 2013). Here we argue that impact science and a better understanding of climate change impacts (see section 2 and 3.2) can be a gateway to bridging this gap. Furthermore, bridging can only be robustly accomplished with active participation by people on both sides of the gap: decision makers working to anticipate and respond to climate impacts on one hand, and climate scientists working to better understand climatic impact-drivers on the other hand. Both require close interaction with the climate impact science community and practitioners. Together they can ensure that climate and impact information become more decision-relevant. Climate and impact information can be tailored to stakeholders considering the spatial and temporal scales associated with their decision domains (Fischer et al., 2024), for example long-term breadbasket-wide drought has a distinct impact on different food system stakeholders (Stuart et al., 2024). This requires that decision makers provide insight into the vulnerabilities of their system, the lead time for decision-making and implementation, and thus the reliability and timing of information supply and demand (e.g., Haasnoot et al., 2018). Beyond that, however, information needs to fit the needs of the decision makers in terms of responsiveness to their values and interests. Sustained engagement with stakeholders is required with sectoral and regional experts working together with physical climate scientists to co-produce useful and contextual impact-, risk- and decision-relevant information that is “fit-for-purpose.” This can help to focus science but is often very time consuming, requires new skill sets, partnerships and infrastructure (Mahon et al., 2019), and is usually not covered by current research funding schemes (Hermansen et al., 2021). In this context, the role of scientific institutions with both a mandate for climate services and sustained funding could be strengthened, for instance in public-private partnerships (Doblas-Reyes et al., 2024; Jacob et al., 2024).
Climate predictions for specific climatic impact-drivers can support decisions to prepare for them and mitigate potential negative impacts or exploit climate-related opportunities (e.g., weather conditions ideal for crop sowing or harvesting) (e.g., Dunstone et al., 2022; Solaraju-Murali et al., 2022). Besides the uncertainties related to prediction quality, the usefulness of predictions depends on user-specific contexts regarding their risk tolerance and level of acceptable uncertainty (e.g., Hinkel et al., 2019). This inherently requires an understanding of how values operate in the decision-making context. To optimize decision-making, users and scientists therefore need to be aware of the specific decision context, which will depend on the cost of action and the potential losses from inaction. Figure 2 conceptually illustrates how different levels (thresholds) of risk tolerance and prediction skill of the model system can result in different false negative, i.e., misses (how often the forecast does not predict an event that does occur), and false positive rates (how often the forecast predicts an event that does not occur in the real world), which will affect decision-making. The skill of the prediction system depends on the location and specific variable or indicator of relevance, as depicted by the green and pink lines in Figure 2B and discussed for marine heatwaves at different locations in Jacox et al. (2022).
Figure 2. Illustration of how climate prediction information can be used for decision-making [adapted from Jacox et al. (2022) Figure 4 under CC-BY-4.0]. Top panel: hazard intensity (magnitude of a climate extreme) as a function of forecast probability, indicating that more intense extremes are typically predicted with higher probability. Bottom panel: False prediction rates (false positive and false negative predictions) as a function of forecast probability, illustrated for a more skillful and a less skillful prediction (where the skill can differ between different regions, climate variables or prediction systems).
All decisions must be taken under uncertainty, and in such probabilistic settings decision makers often optimize the costs and benefits of action or inaction while taking into account the false positive (overestimation) and false negative (underestimation) rates of the prediction. In a risk-avoidance approach, action is taken at a low forecast probability threshold, meaning more events are acted on but actions risk being unnecessary when predicted events do not occur. In a risk-acceptance approach, action is taken at a high forecast probability, limiting actions to more intense events that are better predicted but risking no action being taken for unpredicted events. In the presence of deep uncertainty, event-based or physical climate storylines may be a promising alternative approach (Sillmann et al., 2021a; IPCC, 2021). To deal with uncertainties in adaptation decision-making, an adaptive approach can be taken to support decisions that are low-regret and allow for further adaptation depending on how the future unfolds (e.g., Haasnoot et al., 2018). Additionally, regarding actions under high uncertainty, insights from other scientific fields—such as Decision Theory —could provide valuable guidance (Green and Weatherhead, 2014; Gibbs, 2015; Pope et al., 2017; Delpiazzo et al., 2022). This helps to overcome decision paralysis and avoid maladaptation (IPCC, 2022). Projections on when new climate and impact information may become available can inform such adaptive plans (e.g., by indicating when and what kind of information may become available).
While advancements in climate data and models are undoubtedly necessary, an overemphasis on achieving clear, precise, and reliable climate information often also results in decision paralysis (Nissan et al., 2019). What is needed is the implementation of methodologies that allow for the achievement of fit-for-purpose climate information for the users and the context in which they are operating—information that is reliable and usable. For example, participatory methods that emphasize multi-actor knowledge and utilize climate risk storylines featuring realistic impact events have the potential to catalyze informed decisions (see also 3.2). The benefits of this approach were showcased at the 2023 WCRP Open Science Conference (OSC) with real-world examples (e.g., Jack, 2023; Duba, 2023; Mahon and Trotman, 2023) and corroborated by several authors (e.g., Taylor et al., 2021; McClure, 2023; Ndebele-Murisa et al., 2020). Further research on this approach is recommended in order to develop a comprehensive framework for its future application (see also Baldissera Pacchetti et al., 2024).
Actionable climate information is only attainable with better integration of climate and impact sciences, and decision-making (cf. Figure 1 pillars). Hence, it is essential to invest in the continuous improvement of climate models to enhance the accuracy and robustness of climate predictions. In parallel, a more detailed and quantitative understanding of climate-related impacts, that can ideally be applied seamlessly to climate predictions, is needed – as it is typically the anticipation of certain impacts as opposed to climate anomalies that will be the basis for making decisions. Prioritizing the co-production of data outputs by involving impact scientists and decision makers will ensure relevance and usefulness, while better understanding the inherent uncertainties, communicating them between pillars, and reducing them when possible. Pfleiderer et al. (2023) suggest reversing the traditional impact chain (i.e., going from emissions, to global warming levels, to physical climate impacts) to make information from climate science more actionable and tangible to decision makers. This approach includes socio-economic and value judgment dimensions by starting from a decision-relevant impact or critical threshold of interest (e.g., heat-related mortality in a city of interest) and then estimates global emission reductions that are required to avoid related climate-impact drivers (e.g., exceedance of a temperature threshold associated with heat stress) at the local level. Linking risk threshold exceedance directly to global emissions can further aid the understanding of the benefits of stringent emission reductions for societies and local decision makers (Schleussner et al., 2024).
A climatic impact-driver approach (Ruane et al., 2022) may help stakeholders identify climate conditions that elevate or reduce risk or opportunities, and underpin climate services that can meet stakeholders’ decision-making needs. Risk reduction and risk management are heavily dependent on effective early warning systems and learning from past events. The World Meteorological Organization is investing in a multi-hazard early warning system based on global collaboration and supported by foundational financing mechanisms (WMO, 2022). However, recent extreme events have shown that despite the existence of accurate forecasts and early warning systems, many people lost their lives because not all responsible authorities and individuals received the alerts, or the warnings were not always understood or heeded (Tradowsky et al., 2023). Hence, to provide credible, accessible, relevant and actionable information to underpin decisions in anticipation of certain climatic conditions and related impacts (risks or opportunities) or adaptation to climate change, we need seamless integration of weather and climate information over different time scales and a better understanding of the needs of decision makers, recognizing their diverse backgrounds, objectives, capacities and decision contexts. This needs to be combined with a better integration of information about possible impacts of hazards, particularly for hazards with intensities and frequencies that are changing with global warming. In this context, for instance, Geiger et al. (2024) proposes a strategic, methodological and technical framework for the development of impact-related services provided by National Meteorological and Hydrological Services.
A nuanced, multi-evidence approach enables more robust conclusions by combining insights across observational, theoretical, and modeled lines of evidence for climatic impact-drivers and their impacts, even in cases of uncertainty or divergence. Doblas-Reyes et al. (2021) outlined key strategies to synthesize information coherently when bringing together different types of evidence. These strategies include using a systematic approach to quantify and communicate uncertainty, assessing the physical consistency and plausibility of evidence within the context of known climate processes and mechanisms, hierarchical structuring of evidence and transparent communication of all of these aspects. Furthermore, physical climate storylines are highlighted in Doblas-Reyes et al. (2021) as a valuable complementary tool for reconciling different types of evidence and addressing uncertainties in regional climate assessments. This narrative approach enriches understanding by integrating various forms of evidence, clarifying physical mechanisms, and supporting transparent communication and robust climate risk communication.
The WCRP Regional Information for Society (RIfS) Core Project and the My Climate Risk Lighthouse Activity are exploring frameworks and methodologies in this context (WCRP, 2024a, 2024b). Those efforts are complemented by the annual-to-decadal focus of the WCRP Lighthouse Activity on Explaining and Predicting Earth System Change (EPESC) (Findell et al., 2023). EPESC is looking to develop operational methodologies for integrated attribution, prediction, and projection of changes in the Earth system, particularly those that affect the frequency and intensity of climatic impact-drivers in different regions of the globe. It will be useful if this work could be integrated into the planning of future science priorities across climate and impact science communities. Since the first IPCC Assessment report, we have come a long way in terms of representation of extreme events in observations and climate models, improving prediction systems, climate change and extreme event impact attribution (Noy et al., 2024), and information available for mitigation and adaptation decision-making (IPCC, 2023). Nevertheless, there remains a need to accelerate climate science to expand its relevance and utility to decision makers, and to do so globally (WCRP, 2024c). There are large disparities in data availability and access to training and technology in some regions of the world, including a shortage of high-quality, long-term observations (e.g., Harrington and Otto, 2020; Perkins-Kirkpatrick et al., 2024) and less access to advanced technologies, such as the skills and resources to access high-performance computing facilities needed to run computationally intensive climate models (Forster et al., 2023; UNFCCC, 2023). Recent initiatives, such as FAIR principles (Findable, Accessible, Interoperable, and Reusable data), CARE Principles for Indigenous Data Governance, the Copernicus Climate Change Service (Buontempo et al., 2022) and the European Open Science Cloud (EOSC) help to advance knowledge and address societal challenges through collaborative and transparent data-sharing frameworks. Particularly, outcomes of publicly funded climate services should be treated as public goods accessible to all as highlighted in the Global Framework for Climate Services (Webber and Donner, 2016; Hewitson et al., 2017; Jacob et al., 2024). However, we also note that principles such as FAIR in themselves are not fully sufficient for equitable sharing of data as they might ignore power differentials (e.g., colonialism) and historical contexts (e.g., Indigenous knowledge) (e.g., Jennings et al., 2023).
Likewise, observational campaigns and modeling efforts need to be better integrated and coordinated, which is one of the aims of the WCRP Earth System Modeling and Observations (ESMO) Core Project, to enhance the accuracy, reliability, and accessibility of climate data and projections. While further improvements to data and models are still needed (e.g., Lacagnina et al., 2022), it is also important to focus on the vast amount of climate and impact information already available that can be used to support or trigger decision-making, as shown in the recent risk assessment report by the European Environmental Agency (EEA, 2024). In particular, it is essential to set research priorities with the scientific community in the Global South and allocate resources to foster stronger collaboration, shared and equitable leadership, and alignment with local understanding of science challenges and opportunities (WCRP, 2024c).
In many cases a substantial investment is needed in behind-the-scenes work to quality-control, process, and tailor open-access datasets to facilitate climate services (WMO, 2014). Global observation systems enhancing the real-time weather observing system, combined with advanced impact-based early warning systems (e.g., GIEWS - Global Information and Early Warning System on Food and Agriculture) (WMO, 2022), near real-time information provision during ongoing events and insights gained from post-disaster assessments (e.g., disaster forensics) can be used to sharpen our focus for seasonal and decadal predictions tailored to the needs of specific sectors, but also making the often limited (but definitely non-zero) information content of these predictions more usable. Future climate assessments will need to adopt a more risk-facing perspective, focusing on thresholds, surprises, and the potential for impacts to cascade (Sherwood et al., 2024) to ensure that society is adequately prepared and climate-resilient.
Publicly available datasets were analyzed in this study.
JS: Conceptualization, Funding acquisition, Methodology, Resources, Visualization, Writing – original draft, Writing – review & editing. TR: Conceptualization, Visualization, Writing – original draft, Writing – review & editing. KF: Conceptualization, Visualization, Writing – original draft, Writing – review & editing. MD: Conceptualization, Visualization, Writing – original draft, Writing – review & editing. LMA: Writing – review & editing. LA: Writing – review & editing. LB: Writing – review & editing. PB: Writing – review & editing. CB: Writing – review & editing. EF: Writing – review & editing. CF: Writing – review & editing. BG: Writing – review & editing. MH: Writing – review & editing. EH: Writing – review & editing. DJ: Writing – review & editing. RM: Writing – review & editing. DM: Writing – review & editing. MM: Writing – review & editing. BP: Writing – review & editing. AR: Writing – review & editing. Shampa: Writing – review & editing. TS: Writing – review & editing. NW: Writing – review & editing. ZW: Writing – review & editing. XZ: Writing – review & editing. JŽ: Writing – review & editing.
The author(s) declare that financial support was received for the research, authorship, and/or publication of this article. JS, BP, and LB were funded by the Deutsche Forschungsgemeinschaft (DFG, German Research Foundation) under Germany’s Excellence Strategy—EXC 2037: “CLICCS—Climate, Climatic Change, and Society”—Project number: 390683824, contribution to the Center for Earth System Research and Sustainability (CEN) at the University of Hamburg, JS and JZ further acknowledge funding from the European Union’s Horizon 2020 research and innovation programmes under Grant agreement no. 101003687 (PROVIDE), CF was supported by the Institute for Basic Science (IBS), Republic of Korea, under IBS-R028-D1, EH was supported by the UK National Centre for Atmospheric Science. DM acknowledges funding by the Austrian Climate Research Programme Project CHIANTI (KR19AC0K17553), AR participation was made possible by NASA Earth Sciences Division support for the GISS Climate Impacts Group, TR acknowledges funding support by QBE Insurance since March 2024. LMA was supported by São Paulo Research Foundation (FAPESP, grant 2022/08622-0), and LA was supported by Australian Research Council grant CE170100023. MD is supported by the Horizon Europe project EXPECT (Grant 101137656). CB has been supported by the Copernicus Climate Change Service, a programme funded by the European Commission and implemented by ECMWF.
We thank Cathy Raphael for help with Figure 1. JS would like to thank OpenAI’s ChatGPT v2 (training data up to October 2023) for assistance in refining text in this article.
TR declares funding received by QBE Insurance since March 2024; this funding provider was not involved in this study.
The remaining authors declare that the research was conducted in the absence of any commercial or financial relationships that could be construed as a potential conflict of interest.
The handling editor MC declared a past co-authorship with the following authors LMA and EH.
All claims expressed in this article are solely those of the authors and do not necessarily represent those of their affiliated organizations, or those of the publisher, the editors and the reviewers. Any product that may be evaluated in this article, or claim that may be made by its manufacturer, is not guaranteed or endorsed by the publisher.
Alexander, L. V., Bador, M., Roca, R., Contractor, S., Donat, M. G., and Nguyen, P. L. (2020). Intercomparison of annual precipitation indices and extremes over global land areas from in situ, space-based and reanalysis products. Environ. Res. Lett. 15:055002. doi: 10.1088/1748-9326/ab79e2
Allen, J. T. (2018). Climate change and severe thunderstorms. Oxford Res. Encycl. Clim. Sci. 30:62. doi: 10.1093/acrefore/9780190228620.013.62
Alves, L. M., Ometto, J. P. H. B., Lemos, C. M. G., Andrade, P. R., Bezerra, K. R. A., Santos, D. V., et al. (2022). AdaptaBrasil MCTI: innovative platform for analyzing climate change impacts in Brazil. GEWEX Q. Rep. 32, 8–9.
ARC Centre of Excellence for Climate Extremes (2023). Building bridges between climate scientists and decision-makers. Available at: https://climateextremes.org.au/wp-content/uploads/Building-Bridges-Between-Climate-Scientists-and-Decision-Makers.pdf (Accessed November 23, 2024).
Baier, K., and Stohl, A. (2023). “The role of moisture and heat transport for extreme droughts in the Amazon basin - a Lagrangian perspective,” in EGU General Assembly 2023 (Vienna, Austria, 23–28 April).
Baldissera Pacchetti, M., Coulter, L., Dessai, S., Shepherd, T. G., Sillmann, J., and Van Den Hurk, B. (2023). Varieties of approaches to constructing physical climate storylines: a review. WIREs Clim. Change 15:e869. doi: 10.1002/wcc.869
Baldissera Pacchetti, M., Jebeile, J., and Thompson, E. (2024). For a pluralism of climate modeling strategies. Bull. Am. Meteorol. Soc. 105, E1350–E1364. doi: 10.1175/BAMS-D-23-0169.1
Bevacqua, E., Suarez-Gutierrez, L., Jézéquel, A., Lehner, F., Vrac, M., Yiou, P., et al. (2023). Advancing research on compound weather and climate events via large ensemble model simulations. Nat. Commun. 14:2145. doi: 10.1038/s41467-023-37847-5
Boers, N., Ghil, M., and Stocker, T. F. (2022). Theoretical and paleoclimatic evidence for abrupt transitions in the earth system. Environ. Res. Lett. 17:093006. doi: 10.1088/1748-9326/ac8944
Bony, S., Semie, A., Kramer, R. J., Soden, B., Tompkins, A. M., and Emanuel, K. A. (2020). Observed modulation of the tropical radiation budget by deep convective organization and lower-tropospheric stability. AGU Adv. 1:e2019AV000155. doi: 10.1029/2019AV000155
Borchert, L. F., Düsterhus, A., Brune, S., Müller, W. A., and Baehr, J. (2019b). Forecast-oriented assessment of decadal Hindcast skill for North Atlantic SST. Geophys. Res. Lett. 46, 11444–11454. doi: 10.1029/2019GL084758
Borchert, L. F., Pohlmann, H., Baehr, J., Neddermann, N.-C., Suarez-Gutierrez, L., and Müller, W. A. (2019a). Decadal predictions of the probability of occurrence for warm summer temperature extremes. Geophys. Res. Lett. 46, 14042–14051. doi: 10.1029/2019GL085385
Buontempo, C., Burgess, S. N., Dee, D., Pinty, B., Thépaut, J. N., and Rixen, M. (2022). The Copernicus climate change service: climate science in action. Bull. Amer. Meteor. Soc. 103, E2669–E2687. doi: 10.1175/BAMS-D-21-0315.1
CEOS (2023). Earth observation handbook 2023: space data for the global stocktake. Available at: https://ceos.org/news/eohb-2023/ (Accessed November 23, 2024).
Charlton, C., Stephenson, T., Taylor, M. A., and Campbell, J. (2022). Evaluating skill of the Keetch–Byram drought index, vapour pressure deficit and water potential for determining bushfire potential in Jamaica. Atmos. 13:1267. doi: 10.3390/atmos13081267
Charlton-Perez, A. J., Dacre, H. F., Driscoll, S., Gray, S. L., Harvey, B., Harvey, N. J., et al. (2024). Do AI models produce better weather forecasts than physics-based models? A quantitative evaluation case study of storm Ciarán. NPJ Clim. Atm. Sci. 7:93. doi: 10.1038/s41612-024-00638-w
Dehondt, C., Braconnot, P., Fromang, S., and Marti, O. (2024). “Feedbacks between turbulent air-sea fluxes and their role in the adjustment of the earth climate system,” in EGU General Assembly 2024 (Vienna, Austria, 14–19 April).
Delgado-Torres, C., Donat, M. G., Gonzalez-Reviriego, N., Caron, L.-P., Athanasiadis, P. J., Bretonnière, P.-A., et al. (2022). Multi-model forecast quality assessment of CMIP6 decadal predictions. J. Clim. 35, 4363–4382. doi: 10.1175/JCLI-D-21-0811.1
Delpiazzo, E., Bosello, F., Mazzoli, P., Bagli, S., Luzzi, V., and Dalla Valle, F. (2022). Co-evaluation of climate services. A case study for hydropower generation. Clim. Serv. 28:100335. doi: 10.1016/j.cliser.2022.100335
Dettinger, M. D., Martin Ralph, F., Hughes, M., Das, T., Neiman, P., Cox, D., et al. (2012). Design and quantification of an extreme winter storm scenario for emergency preparedness and planning exercises in California. Nat. Hazards 60, 1085–1111. doi: 10.1007/s11069-011-9894-5
Doblas-Reyes, F. J., Clair, L. S., Baldissera Pacchetti, M., Checchia, P., Cortekar, J., Klostermann, J. E. M., et al. (2024). Standardisation of equitable climate services by supporting a community of practice. Clim. Serv. 36:100520. doi: 10.1016/j.cliser.2024.100520
Doblas-Reyes, F. J., Sörensson, A. A., Almazroui, M., Dosio, A., Gutowski, W. J., Haarsma, R., et al. (2021). “Linking global to regional climate change” in Climate change 2021: The physical science basis. Contribution of working group I to the sixth assessment report of the intergovernmental panel on climate change. eds. V. Masson-Delmotte, P. Zhai, A. Pirani, S. L. Connors, and C. Péan (Cambridge: Cambridge University Press), 1363–1512.
Duan, S. Q., Findell, K. L., and Fueglistaler, S. A. (2023). Coherent mechanistic patterns of tropical land hydroclimate changes. Geophys. Res. Lett. 50:e2022GL102285. doi: 10.1029/2022GL102285
Duba, T. (2023). “Sustainable small scale fisheries livelihood under uncertainty: participatory scenario planning for community adaptation response to climate change impacts,” in Poster Cluster 36 WCRP Open Science Conference 2023 (Kigali, Rwanda, 26 October).
Dunn, R. J. H., Alexander, L. V., Donat, M. G., Zhang, X., Bador, M., Herold, N., et al. (2020). Development of an updated global land in situ-based data set of temperature and precipitation extremes: HadEX3. J. Geophys. Res. Atmos. 125:e2019JD032263. doi: 10.1029/2019JD032263
Dunstone, N., Lockwood, J., Solaraju-Murali, B., Reinhardt, K., Tsartsali, E. E., Athanasiadis, P. J., et al. (2022). Towards useful decadal climate services. Bull. Am. Meteorol. Soc. 103, E1705–E1719. doi: 10.1175/BAMS-D-21-0190.1
Dunstone, N., Smith, D. M., Hardiman, S. C., Davies, P., Ineson, S., Jain, S., et al. (2023). Windows of opportunity for predicting seasonal climate extremes highlighted by the Pakistan floods of 2022. Nat. Commun. 14:6544. doi: 10.1038/s41467-023-42377-1
EEA (2024). European climate risk assessment. Available at: https://www.eea.europa.eu/publications/european-climate-risk-assessment (Accessed November 23, 2024).
Findell, K. L., Gentine, P., Lintner, B. R., and Guillod, B. P. (2015). Data length requirements for observational estimates of land–atmosphere coupling strength. J. Hydrometeorol. 16, 1615–1635. doi: 10.1175/JHM-D-14-0131.1
Findell, K. L., Sutton, R., Caltabiano, N., Brookshaw, A., Heimbach, P., Kimoto, M., et al. (2023). Explaining and predicting earth system change: a world climate research programme call to action. Bull. Am. Meteorol. Soc. 104, E325–E339. doi: 10.1175/BAMS-D-21-0280.1
Fischer, A. M., Bessembinder, J., Fung, F., Hygen, H. O., and Jacobs, K. (2024). Editorial: generating actionable climate information in support of climate adaptation and mitigation. Front. Clim. 6:1444157. doi: 10.3389/fclim.2024.1444157
Fischer, E. M., Sippel, S., and Knutti, R. (2021). Increasing probability of record-shattering climate extremes. Nat. Clim. Chang. 11, 689–695. doi: 10.1038/s41558-021-01092-9
Fischer, A. M., Strassmann, K. M., Croci-Maspoli, M., Hama, A. M., Knutti, R., Kotlarski, S., et al. (2022). Climate scenarios for Switzerland CH2018 – approach and implications. Clim. Serv. 26:100288. doi: 10.1016/j.cliser.2022.100288
Forster, P., Naik, V., Stammer, D., Cleugh, H., and Caltabiano, N. (Eds.) (2023). A WCRP vision for accessible, useful and reliable climate modeling systems: report of the future of climate modeling workshop. Geneva: WCRP publication.
Fowler, H. J., Lenderink, G., Prein, A. F., Westra, S., Allan, R. P., Ban, N., et al. (2021). Anthropogenic intensification of short-duration rainfall extremes. Nat. Rev. Earth Environ. 2, 107–122. doi: 10.1038/s43017-020-00128-6
Fox, S., Crawford, A., McCrystall, M., Stroeve, J., Lukovich, J., Loeb, N., et al. (2023). Extreme Arctic weather and community impacts in Nunavut: a case study of one winter’s storms and lessons for local climate change preparedness. Weather Clim. Soc. 15, 881–892. doi: 10.1175/WCAS-D-23-0006.1
Franzke, C. L. E., Ciullo, A., Gilmore, E. A., Matias, D. M., Nagabhatla, N., Orlov, A., et al. (2022). Perspectives on tipping points in integrated models of the natural and human earth system: cascading effects and telecoupling. Environ. Res. Lett. 17:015004. doi: 10.1088/1748-9326/ac42fd
Freisen, P. F., Arblaster, J. M., Jakob, C., and Rodríguez, J. M. (2022). Investigating tropical versus extratropical influences on the southern hemisphere tropical edge in the unified model. J. Geophys. Res. Atmos. 127:e2021JD036106. doi: 10.1029/2021JD036106
Frieler, K., Volkholz, J., Lange, S., Schewe, J., Mengel, M., Del Rocío Rivas López, M., et al. (2024). Scenario setup and forcing data for impact model evaluation and impact attribution within the third round of the inter-sectoral impact model intercomparison project (ISIMIP3a). Geosci. Model Dev. 17, 1–51. doi: 10.5194/gmd-17-1-2024
Galford, G. L., Nash, J., Betts, A. K., Carlson, S., Ford, S., Hoogenboom, A., et al. (2016). Bridging the climate information gap: a framework for engaging knowledge brokers and decision makers in state climate assessments. Clim. Chang. 138, 383–395. doi: 10.1007/s10584-016-1756-4
Garfinkel, C. I., Keller, B., Lachmy, O., White, I., Gerber, E. P., Jucker, M., et al. (2024). Impact of parameterized convection on the storm track and near-surface jet response to global warming: implications for mechanisms of the future poleward shift. J. Clim. 37, 2541–2564. doi: 10.1175/JCLI-D-23-0105.1
Geiger, T., Röösli, T., Bresch, D. N., Erhardt, B., Fischer, A. M., Imgrüth, D., et al. (2024). How to provide actionable information on weather and climate impacts?–A summary of strategic, methodological, and technical perspectives. Front. Clim. 6:1343993. doi: 10.3389/fclim.2024.1343993
Gibbs, M. T. (2015). Pitfalls in developing coastal climate adaptation responses. Clim. Risk Manag. 8, 1–8. doi: 10.1016/j.crm.2015.05.001
Green, M., and Weatherhead, E. K. (2014). Coping with climate change uncertainty for adaptation planning: an improved criterion for decision making under uncertainty using UKCP09. Clim. Risk Manag. 1, 63–75. doi: 10.1016/j.crm.2013.11.001
Gutierrez-Villanueva, M. O., Chereskin, T. K., and Sprintall, J. (2023). Compensating transport trends in the Drake Passage frontal regions yield no acceleration in net transport. Nat. Commun. 14:7792. doi: 10.1038/s41467-023-43499-2
Haasnoot, M., Van’t Klooster, S., and van Alphen, J. (2018). Designing a monitoring system to detect signals to adapt to uncertain climate change. Glob. Environ. Chang. 52, 273–285. doi: 10.1016/j.gloenvcha.2018.08.003
Hansen, G., and Stone, D. (2016). Assessing the observed impact of anthropogenic climate change. Nat. Clim. Chang. 6, 532–537. doi: 10.1038/nclimate2896
Harrington, L. J., and Otto, F. E. L. (2020). Reconciling theory with the reality of African heatwaves. Nat. Clim. Chang. 10, 796–798. doi: 10.1038/s41558-020-0851-8
Harvey, B., Hawkins, E., and Sutton, R. (2023). Storylines for future changes of the North Atlantic jet and associated impacts on the UK. Int. J. Climatol. 43, 4424–4441. doi: 10.1002/joc.8095
Hawkins, E., Burt, S., McCarthy, M., Murphy, C., Ross, C., Baldock, M., et al. (2023b). Millions of historical monthly rainfall observations taken in the UK and Ireland rescued by citizen scientists. Geosci. Data J. 10, 246–261. doi: 10.1002/gdj3.157
Hawkins, E., Compo, G. P., and Sardeshmukh, P. D. (2023a). ESD ideas: translating historical extreme weather events into a warmer world. Earth Syst. Dynam. 14, 1081–1084. doi: 10.5194/esd-14-1081-2023
Hegdahl, T. J., Engeland, K., Müller, M., and Sillmann, J. (2020). An event-based approach to explore selected present and future Atmospheric River–induced floods in Western Norway. J. Hydrometeorol. 21, 2003–2021. doi: 10.1175/JHM-D-19-0071.1
Hermansen, E. A. T., Sillmann, J., Vigo, I., and Whittlesey, S. (2021). The EU needs a demand-driven innovation policy for climate services. Clim. Serv. 24:100270. doi: 10.1016/j.cliser.2021.100270
Hewitson, B., Waagsaether, K., Wohland, J., Kloppers, K., and Kara, T. (2017). Climate information websites: an evolving landscape. WIREs Clim. Change 8:e470. doi: 10.1002/wcc.470
Hinkel, J., Church, J. A., Gregory, J. M., Lambert, E., Le Cozannet, G., Lowe, J., et al. (2019). Meeting user needs for sea level rise information: A decision analysis perspective. Earth’s Future 7, 320–337. doi: 10.1029/2018EF001071
Huang, X., and Swain, D. L. (2022). Climate change is increasing the risk of a California megaflood. Sci. Adv. 8:eabq0995. doi: 10.1126/sciadv.abq0995
IPCC (2021). Climate change 2021: The physical science basis. Contribution of working group I to the sixth assessment report of the intergovernmental panel on climate change. Cambridge: Cambridge University Press.
IPCC (2022). Summary for policymakers,” in climate change 2022: Impacts, adaptation and vulnerability. Contribution of working group II to the sixth assessment report of the intergovernmental panel on climate change. Cambridge: Cambridge University Press, 3–33.
IPCC (2023). “Sections” in Climate change 2023: Synthesis report. Contribution of working groups I, II, and III to the sixth assessment report of the intergovernmental panel on climate change. eds. H. Lee and J. Romero (Cambridge: Cambridge University Press), 35–115.
Jack, C. (2023). “Mind the step: climate data as a barrier to effective decision making,” in Presentation Session 35, WCRP Open Science Conference 2023 (Kigali, Rwanda, 25 October).
Jacob, D., St Clair, A. L., Mahon, R., Marsland, S., Murisa, M. N., and Buontempo, C. (2024). Co-production of climate services. under review
Jacox, M. G., Alexander, M. A., Amaya, D., Becker, E., Bograd, S. J., Brodie, S., et al. (2022). Global seasonal forecasts of marine heatwaves. Nature 604, 486–490. doi: 10.1038/s41586-022-04573-9
Jennings, L., Anderson, T., Martinez, A., Sterling, R., Chavez, D. D., Garba, I., et al. (2023). Applying the ‘CARE principles for indigenous data governance to ecology and biodiversity research. Nat. Ecol. Evol. 7, 1547–1551. doi: 10.1038/s41559-023-02161-2
Keating, A., Venkateswaran, K., Szoenyi, M., MacClune, K., and Mechler, R. (2016). From event analysis to global lessons: disaster forensics for building resilience. Nat. Hazards Earth Syst. Sci. 16, 1603–1616. doi: 10.5194/nhess-16-1603-2016
Kelder, T., Müller, M., Slater, L. J., Marjoribanks, T. I., Wilby, R. L., Prudhomme, C., et al. (2020). Using UNSEEN trends to detect decadal changes in 100-year precipitation extremes. NPJ Clim. Atmos. Sci. 3:47. doi: 10.1038/s41612-020-00149-4
Kendon, E. J., Prein, A. F., Senior, C. A., and Stirling, A. (2021). Challenges and outlook for convection-permitting climate modelling. Philos. Trans. R. Soc. A Math. Phys. Eng. Sci. 379:20190547. doi: 10.1098/rsta.2019.0547
Klein, C., Barton, E., and Taylor, C. (2023). “Changes in land surface effects on organised convection in a convection-permitting climate projection,” in EGU General Assembly 2023 (Vienna, Austria, 24–28 April 2023).
Kong, X., Wang, A., Bi, X., Sun, B., and Wei, J. (2022). The hourly precipitation frequencies in the Tropical-Belt version of WRF: sensitivity to cumulus parameterization and radiation schemes. J. Clim. 35, 285–304. doi: 10.1175/JCLI-D-20-0854.1
Lacagnina, C., Doblas-Reyes, F., Larnicol, G., Buontempo, C., Obregón, A., Costa-Surós, M., et al. (2022). Quality management framework for climate datasets. Data Sci. J. 21:10. doi: 10.5334/dsj-2022-010
Lang, A., and Poschlod, B. (2024). Updating catastrophe models to today’s climate – an application of a large ensemble approach to extreme rainfall. Clim. Risk Manag. 44:100594. doi: 10.1016/j.crm.2024.100594
Lee, J.-Y., Marotzke, J., Bala, G., Cao, L., Corti, S., Dunne, J. P., et al. (2021). “Future global climate: scenario-based projections and near-term information” in Climate change 2021: The physical science basis. Contribution of working group I to the sixth assessment report of the intergovernmental panel on climate change. eds. V. Masson-Delmotte, P. Zhai, A. Pirani, and S. L. Connors (Cambridge: Cambridge University Press), 553–672.
Li, H., Chen, L., Zhong, X., Wu, J., Chen, D., Xie, S.-P., et al. (2024). A machine learning model that outperforms conventional global subseasonal forecast models. Res. Square 1:4493. doi: 10.21203/rs.3.rs-5104493/v1
Liu, Y., Donat, M. G., England, M. H., Alexander, L. V., Hirsch, A. L., and Delgado-Torres, C. (2023). Enhanced multi-year predictability after El Niño and La Niña events. Nat. Commun. 14:6387. doi: 10.1038/s41467-023-42113-9
Lloyd, E. A., and Shepherd, T. G. (2020). Environmental catastrophes, climate change, and attribution. Ann. N. Y. Acad. Sci. 1469, 105–124. doi: 10.1111/nyas.14308
Lowe, J. A., Bernie, D., Bett, P., Bricheno, L., Brown, S., Calvert, D., et al. (2018). UKCP18 science overview report. Exeter: Met Office.
Mahon, R., Greene, C., Cox, S.-A., Guido, Z., Gerlak, A. K., Petrie, J.-A., et al. (2019). Fit for purpose? Transforming national meteorological and hydrological services into national climate service centers. Clim. Serv. 13, 14–23. doi: 10.1016/j.cliser.2019.01.002
Mahon, R., and Trotman, A. (2023). “Co-producing climate services in the global south: successes, challenges and lessons learnt from Caribbean small island developing states,” in Poster Cluster 40, WCRP Open Science Conference 2023 (Kigali, Rwanda, 26 October).
Maraun, D., Knevels, R., Mishra, A. N., Truhetz, H., Bevacqua, E., Proske, H., et al. (2022). A severe landslide event in the alpine foreland under possible future climate and land-use changes. Commun. Earth Environ. 3:87. doi: 10.1038/s43247-022-00408-7
Mariotti, A., Baggett, C., Barnes, E. A., Becker, E., Butler, A., Collins, D. C., et al. (2020). Windows of opportunity for skillful forecasts subseasonal to seasonal and beyond. Bull. Am. Meteorol. Soc. 101, E608–E625. doi: 10.1175/BAMS-D-18-0326.1
McClure, A. (2023). Enablers of transdisciplinary collaboration for researchers working on climate risks in African cities. Sustain. Sci. 19, 259–273. doi: 10.1007/s11625-023-01426-w
Meehl, G. A., Richter, J. H., Teng, H., Capotondi, A., Cobb, K., Doblas-Reyes, F., et al. (2021). Initialized earth system prediction from subseasonal to decadal timescales. Nat. Rev. Earth Environ. 2, 340–357. doi: 10.1038/s43017-021-00155-x
Menon, A., Turner, A. G., Volonté, A., Taylor, C. M., Webster, S., and Martin, G. (2022). The role of mid-tropospheric moistening and land-surface wetting in the progression of the 2016 Indian monsoon. Q. J. R. Meteorol. Soc. 148, 3033–3055. doi: 10.1002/qj.4183
Ndebele-Murisa, M. R., Mubaya, C. P., Pretorius, L., Mamombe, R., Iipinge, K., Nchito, W., et al. (2020). City to city learning and knowledge exchange for climate resilience in southern Africa. PLoS One 15:e0227915. doi: 10.1371/journal.pone.0227915
New, M., Reckien, D., Viner, D., Adler, C., Cheong, S.-M., Conde, C., et al. (2022). “Decision-making options for managing risk” in climate change 2022: Impacts, adaptation and vulnerability. Contribution of working group II to the sixth assessment report of the intergovernmental panel on climate change. eds. H. O. Pörtner, D. C. Roberts, M. Tignor, E. S. Poloczanska, K. Mintenbeck, and A. Alegría (Cambridge: Cambridge University Press), 2539–2654.
Nissan, H., Goddard, L., de Perez, E. C., Furlow, J., Baethgen, W., Thomson, M. C., et al. (2019). On the use and misuse of climate change projections in international development. WIREs Clim. Change 10:e579. doi: 10.1002/wcc.579
Nkrumah, F., Klein, C., Quagraine, K. A., Berkoh-Oforiwaa, R., Klutse, N. A. B., Essien, P., et al. (2023). Classification of large-scale environments that drive the formation of mesoscale convective systems over southern West Africa. Weather Clim. Dynam. 4, 773–788. doi: 10.5194/wcd-4-773-2023
Noy, I., Stone, D., and Uher, T. (2024). Extreme events impact attribution: a state of the art. Cell Rep. Sust. 1:100101. doi: 10.1016/j.crsus.2024.100101
Otto, F. E. L., Harrington, L. J., Frame, D., Boyd, E., Lauta, K. C., Wehner, M., et al. (2020a). Toward an inventory of the impacts of human-induced climate change. Bull. Am. Meteorol. Soc. 101, E1972–E1979. doi: 10.1175/BAMS-D-20-0027.1
Otto, F. E. L., Harrington, L., Schmitt, K., Philip, S., Kew, S., van Oldenborgh, G. J., et al. (2020b). Challenges to understanding extreme weather changes in lower income countries. Bull. Am. Meteorol. Soc. 101, E1851–E1860. doi: 10.1175/BAMS-D-19-0317.1
Perkins-Kirkpatrick, S. E., Alexander, L. V., King, A. D., Kew, S. F., Philip, S. Y., Barnes, C., et al. (2024). Frontiers in attributing climate extremes and associated impacts. Front. Clim. 6:1455023. doi: 10.3389/fclim.2024.1455023
Perkins-Kirkpatrick, S. E., Stone, D. A., Mitchell, D. M., Rosier, S., King, A. D., Lo, Y. T. E., et al. (2022). On the attribution of the impacts of extreme weather events to anthropogenic climate change. Environ. Res. Lett. 17:024009. doi: 10.1088/1748-9326/ac44c8
Pfleiderer, P., Frölicher, T., Kropf, C. M., Lamboll, R., Lejeune, Q., Lourenco, T. C., et al. (2023). Reversal of the impact chain for actionable climate information. Available at: https://eartharxiv.org/repository/view/5883/ (Accessed November 23, 2024).
Plésiat, É., Dunn, R. J. H., Donat, M. G., and Kadow, C. (2024). Artificial intelligence reveals past climate extremes by reconstructing historical records. Nat. Commun. 15:9191. doi: 10.1038/s41467-024-53464-2
Pope, E. C. D., Buontempo, C., and Economou, T. (2017). Quantifying how user-interaction can modify the perception of the value of climate information: a Bayesian approach. Clim. Serv. 6, 41–47. doi: 10.1016/j.cliser.2017.06.006
Poschlod, B. (2021). Using high-resolution regional climate models to estimate return levels of daily extreme precipitation over Bavaria. Nat. Hazards Earth Syst. Sci. 21, 3573–3598. doi: 10.5194/nhess-21-3573-2021
Poschlod, B., Ludwig, R., and Sillmann, J. (2021). Ten-year return levels of sub-daily extreme precipitation over Europe. Earth Syst. Sci. Data 13, 983–1003. doi: 10.5194/essd-13-983-2021
Poschlod, B., Zscheischler, J., Sillmann, J., Wood, R. R., and Ludwig, R. (2020). Climate change effects on hydrometeorological compound events over southern Norway. Weather Clim. Extr. 28:100253. doi: 10.1016/j.wace.2020.100253
Prein, A. F., Rasmussen, R. M., Wang, D., and Giangrande, S. E. (2021). Sensitivity of organized convective storms to model grid spacing in current and future climates. Philos. Trans. R. Soc. A Math. Phys. Eng. Sci. 379:20190546. doi: 10.1098/rsta.2019.0546
Ranasinghe, R., Ruane, A. C., Vautard, R., Arnell, N., Coppola, E., Cruz, F. A., et al. (2021). “Climate change information for regional impact and for risk assessment” in Climate change 2021: The physical science basis. Contribution of working group I to the sixth assessment report of the intergovernmental panel on climate change. eds. V. Masson-Delmotte, P. Zhai, A. Pirani, and S. L. Connors (Cambridge: Cambridge University Press), 1767–1926.
Raymond, C., Suarez-Gutierrez, L., Kornhuber, K., Pascolini-Campbell, M., Sillmann, J., and Waliser, D. E. (2022). Increasing spatiotemporal proximity of heat and precipitation extremes in a warming world quantified by a large model ensemble. Environ. Res. Lett. 17:035005. doi: 10.1088/1748-9326/ac5712
Reichstein, M., Benson, C., Camps-Valls, G., Vinuesa, R., et al. (2024). Early warning of complex climate risk with integrated artificial intelligence. Nat. Sust. 17, 1–36. doi: 10.21203/rs.3.rs-4248340/v1
Reichstein, M., Camps-Valls, G., Stevens, B., Jung, M., Denzler, J., Carvalhais, N., et al. (2019). Deep learning and process understanding for data-driven earth system science. Nature 566, 195–204. doi: 10.1038/s41586-019-0912-1
Rowell, D. P., and Berthou, S. (2023). Fine-scale climate projections: what additional fixed spatial detail is provided by a convection-permitting model? J. Clim. 36, 1229–1246. doi: 10.1175/JCLI-D-22-0009.1
Ruane, A. C., Vautard, R., Ranasinghe, R., Sillmann, J., Coppola, E., Arnell, N., et al. (2022). The climatic impact-driver framework for assessment of risk-relevant climate information. Earth’s. Future 10:e2022EF002803. doi: 10.1029/2022EF002803
Salcedo-Sanz, S., Pérez-Aracil, J., Ascenso, G., Del Ser, J., Casillas-Pérez, D., Kadow, C., et al. (2024). Analysis, characterization, prediction, and attribution of extreme atmospheric events with machine learning and deep learning techniques: a review. Theor. Appl. Climatol. 155, 1–44. doi: 10.1007/s00704-023-04571-5
Sánchez-Benítez, A., Goessling, H., Pithan, F., Semmler, T., and Jung, T. (2022). The July 2019 European heat wave in a warmer climate: storyline scenarios with a coupled model using spectral nudging. J. Clim. 35, 2373–2390. doi: 10.1175/JCLI-D-21-0573.1
Savo, V., Kohfeld, K. E., Sillmann, J., Morton, C., Bailey, J., Haslerud, A. S., et al. (2024). Using human observations with instrument-based metrics to understand changing rainfall patterns. Nat. Commun. 15:9563. doi: 10.1038/s41467-024-53861-7
Schär, C., Fuhrer, O., Arteaga, A., Ban, N., Charpilloz, C., Di Girolamo, S., et al. (2020). Kilometer-scale climate models: prospects and challenges. Bull. Am. Meteorol. Soc. 101, E567–E587. doi: 10.1175/BAMS-D-18-0167.1
Schewe, J., Gosling, S. N., Reyer, C., Zhao, F., Ciais, P., Elliott, J., et al. (2019). State-of-the-art global models underestimate impacts from climate extremes. Nat. Commun. 10:1005. doi: 10.1038/s41467-019-08745-6
Schleussner, C.-F., Ganti, G., Lejeune, Q., Zhu, B., Pfleiderer, P., Prütz, R., et al. (2024). Overconfidence in climate overshoot. Nature 634, 366–373. doi: 10.1038/s41586-024-08020-9
Schneider, T., Behera, S., Boccaletti, G., Deser, C., Emanuel, K., Ferrari, R., et al. (2023). Harnessing AI and computing to advance climate modelling and prediction. Nat. Clim. Chang. 13, 887–889. doi: 10.1038/s41558-023-01769-3
Schwingshackl, C., Sillmann, J., Vicedo-Cabrera, A. M., Sandstad, M., and Aunan, K. (2021). Heat stress indicators in CMIP6: estimating future trends and exceedances of impact-relevant thresholds. Earth’s Fut. 9:e2020EF001885. doi: 10.1029/2020EF001885
Senatore, A., Mendicino, G., Gochis, D. J., Yu, W., Yates, D. N., and Kunstmann, H. (2015). Fully coupled atmosphere-hydrology simulations for the Central Mediterranean: impact of enhanced hydrological parameterization for short and long time scales. J. Adv. Model. Earth Syst. 7, 1693–1715. doi: 10.1002/2015MS000510
Seneviratne, S. I., Zhang, X., Adnan, M., Badi, W., Dereczynski, C., Luca, A. D., et al. (2021). “Weather and climate extreme events in a changing climate” in Climate change 2021 – The physical science basis: Working group I contribution to the sixth assessment report of the intergovernmental panel on climate change. eds. V. Masson-Delmotte, P. Zhai, A. Pirani, S. L. Connors, C. Péan, and S. Berger (Cambridge: Cambridge University Press), 1513–1766.
Sgubin, G., Swingedouw, D., Borchert, L. F., Menary, M. B., Noël, T., Loukos, H., et al. (2021). Systematic investigation of skill opportunities in decadal prediction of air temperature over Europe. Clim. Dyn. 57, 3245–3263. doi: 10.1007/s00382-021-05863-0
Shepherd, T. G. (2016). A common framework for approaches to extreme event attribution. Curr. Clim. Chang. Rep. 2, 28–38. doi: 10.1007/s40641-016-0033-y
Sherwood, S. C., Hegerl, G., Braconnot, P., Friedlingstein, P., Goelzer, H., Harris, N. R. P., et al. (2024). Uncertain pathways to a future safe climate. Earth’s Fut. 12:e2023EF004297. doi: 10.1029/2023EF004297
Sillmann, J., Shepherd, T. G., van den Hurk, B., Hazeleger, W., Martius, O., Slingo, J., et al. (2021a). Event-based storylines to address climate risk. Earth’s Fut. 9:e2020EF001783. doi: 10.1029/2020EF001783
Sillmann, J., Aunan, K., Emberson, L., Büker, P., Van Oort, B., O’Neill, C., et al. (2021b). Combined impacts of climate and air pollution on human health and agricultural productivity. Environ. Res. Lett. 16:093004. doi: 10.1088/1748-9326/ac1df8
Sillmann, J., Thorarinsdottir, T., Keenlyside, N., Schaller, N., Alexander, L. V., Hegerl, G., et al. (2017). Understanding, modeling and predicting weather and climate extremes: challenges and opportunities. Weather Clim. Extr. 18, 65–74. doi: 10.1016/j.wace.2017.10.003
Simpson, N. P., Mach, K. J., Constable, A., Hess, J., Hogarth, R., Howden, M., et al. (2021). A framework for complex climate change risk assessment. One Earth 4, 489–501. doi: 10.1016/j.oneear.2021.03.005
Simpson, N. P., Williams, P. A., Mach, K. J., Berrang-Ford, L., Biesbroek, R., Haasnoot, M., et al. (2023). Adaptation to compound climate risks: a systematic global stocktake. iScience 26:105926. doi: 10.1016/j.isci.2023.105926
Slangen, A. B. A., Haasnoot, M., and Winter, G. (2022). Rethinking Sea-level projections using families and timing differences. Earth’s Fut. 10:e2021EF002576. doi: 10.1029/2021EF002576
Solaraju-Murali, B., Bojovic, D., Gonzalez-Reviriego, N., Nicodemou, A., Terrado, M., Caron, L.-P., et al. (2022). How decadal predictions entered the climate services arena: an example from the agriculture sector. Clim. Serv. 27:100303. doi: 10.1016/j.cliser.2022.100303
Son, R., Stacke, T., Gayler, V., Nabel, J. E. M. S., Schnur, R., Alonso, L., et al. (2024). Integration of a deep-learning-based fire model into a global land surface model. J. Adv. Model. Earth Syst. 16:e2023MS003710. doi: 10.1029/2023MS003710
Stuart, L., Hobbins, M., Niebuhr, E., Ruane, A. C., Pulwarty, R., Hoell, A., et al. (2024). Enhancing global food security: opportunities for the American meteorological society. Bull. Am. Meteorol. Soc. 105, E760–E777. doi: 10.1175/BAMS-D-22-0106.1
Suarez-Gutierrez, L., Müller, W. A., Li, C., and Marotzke, J. (2020). Hotspots of extreme heat under global warming. Clim. Dyn. 55, 429–447. doi: 10.1007/s00382-020-05263-w
Sun, J., Liu, S., Cohen, J., and Yu, S. (2022). Influence and prediction value of Arctic Sea ice for spring Eurasian extreme heat events. Commun. Earth Environ. 3:172. doi: 10.1038/s43247-022-00503-9
Taylor, A., Jack, C., McClure, A., Bharwani, S., Ilunga, R., and Kavonic, J. (2021). Understanding and supporting climate-sensitive decision processes in southern African cities. Curr. Opin. Environ. Sustain. 51, 77–84. doi: 10.1016/j.cosust.2021.03.006
Theokritoff, E., van Maanen, N., Andrijevic, M., Thomas, A., Lissner, T., and Schleussner, C.-F. (2023). Adaptation constraints in scenarios of socio-economic development. Sci. Rep. 13:19604. doi: 10.1038/s41598-023-46931-1
Thomas, A., Theokritoff, E., Lesnikowski, A., Reckien, D., Jagannathan, K., Cremades, R., et al. (2021). Global evidence of constraints and limits to human adaptation. Reg. Environ. Chang. 21:85. doi: 10.1007/s10113-021-01808-9
Thompson, V., Dunstone, N. J., Scaife, A. A., Smith, D. M., Slingo, J. M., Brown, S., et al. (2017). High risk of unprecedented UK rainfall in the current climate. Nat. Commun. 8:107. doi: 10.1038/s41467-017-00275-3
Tradowsky, J. S., Philip, S. Y., Kreienkamp, F., Kew, S. F., Lorenz, P., Arrighi, J., et al. (2023). Attribution of the heavy rainfall events leading to severe flooding in Western Europe during July 2021. Clim. Chang. 176:90. doi: 10.1007/s10584-023-03502-7
UNFCCC (2023). “Bridging the research gap: levelling the climate research field and empowering global south climate change adaptation and disaster risk reduction,” in UN Climate Change Conference (Dubai, United Arab Emirates, Nov/Dec 2023), COP 28, global Climate Action (GCA). Implementation Lab, Marrakech Partnership for Global Climate Action, global climate Action Hub, Action Lab 1 – Al Shaheen.
van der Wiel, K., Beersma, J., van den Brink, H., Krikken, F., Selten, F., Severijns, C., et al. (2024). KNMI’23 climate scenarios for the Netherlands: storyline scenarios of regional climate change. Earth’s Fut. 12:e2023EF003983. doi: 10.1029/2023EF003983
van Dorland, R., Beersma, J., Bessembinder, J., Bloemendaal, N., van den Brink, H., Brotons Blanes, B., et al. (2023). KNMI national climate scenarios 2023 for the Netherlands. Technical report no. WR 23-02. De Bilt: Royal Netherlands Meteorological Institute.
van Oldenborgh, G. J., Wehner, M. F., Vautard, R., Otto, F. E. L., Seneviratne, S. I., Stott, P. A., et al. (2022). Attributing and projecting heatwaves is hard: we can do better. Earth’s Future 10:e2021EF002271. doi: 10.1029/2021EF002271
Vg, K., Rao, S. A., and Pillai, P. A. (2023). “Impact of bay of Bengal mesoscale eddies on Indian summer monsoon rainfall,” in Copernicus Meetings.
Vicedo-Cabrera, A. M., Scovronick, N., Sera, F., Royé, D., Schneider, R., Tobias, A., et al. (2021). The burden of heat-related mortality attributable to recent human-induced climate change. Nat. Clim. Chang. 11, 492–500. doi: 10.1038/s41558-021-01058-x
von Winterfeldt, D. (2013). Bridging the gap between science and decision making. Proc. Natl. Acad. Sci. 110, 14055–14061. doi: 10.1073/pnas.1213532110
WCRP (2024a). Regional information for society (RIfS). Available at: https://www.wcrp-rifs.org
WCRP (2024b). My climate risk lighthouse activity. Available at: https://www.wcrp-climate.org/my-climate-risk
WCRP (2024c). “Kigali declaration: climate science for a sustainable future for all,” in WCRP Open Science Conference (Kigali, Rwanda, 23–27 October 2023).
Webber, S., and Donner, S. D. (2016). Climate service warnings: cautions about commercializing climate science for adaptation in the developing world. WIREs Clim. Change 8:e424. doi: 10.1002/wcc.424
WMO (2014). Implementation plan of the global framework for climate services (GFCS). Available at: https://library.wmo.int/idurl/4/55761
WMO (2022). Global Observing System (GOS). Available at: https://wmo.int/activities/global-observing-system-gos/global-observing-system-gos
Yang, J., Gong, P., Fu, R., Zhang, M., Chen, J., Liang, S., et al. (2013). The role of satellite remote sensing in climate change studies. Nat. Clim. Chang. 3, 875–883. doi: 10.1038/nclimate1908
Young, J. C., Arthur, R., Spruce, M., and Williams, H. T. P. (2022). Social sensing of flood impacts in India: A case study of Kerala 2018. Int. J. Disaster Risk Reduc. 74:102908. doi: 10.1016/j.ijdrr.2022.102908
Yule, E. L., Hegerl, G., Schurer, A., and Hawkins, E. (2023). Using early extremes to place the 2022 UK heat waves into historical context. Atmos. Sci. Lett. 24:e1159. doi: 10.1002/asl.1159
Zhang, Y., Ayyub, B. M., Fung, J. F., and Labe, Z. M. (2024). Incorporating extreme event attribution into climate change adaptation for civil infrastructure: methods, benefits, and research needs. Res. Cities Struct. 3, 103–113. doi: 10.1016/j.rcns.2024.03.002
Zilli, M. T., Hart, N. C. G., Coelho, C. A. S., Chadwick, R., de Souza, D. C., Kubota, P. Y., et al. (2023). Characteristics of tropical–extratropical cloud bands over tropical and subtropical South America simulated by BAM-1.2 and HadGEM3-GC3.1. Q. J. R. Meteorol. Soc. 149, 1498–1519. doi: 10.1002/qj.4470
Keywords: climate extremes, climate impacts, climate risk, decision making, climate service, climate change
Citation: Sillmann J, Raupach TH, Findell KL, Donat M, Alves LM, Alexander L, Borchert L, Borges de Amorim P, Buontempo C, Fischer EM, Franzke CL, Guan B, Haasnoot M, Hawkins E, Jacob D, Mahon R, Maraun D, Morrison MA, Poschlod B, Ruane AC, Shampa, Stephenson T, van der Wel N, Wang Z, Zhang X and Županić J (2024) Climate extremes and risks: links between climate science and decision-making. Front. Clim. 6:1499765. doi: 10.3389/fclim.2024.1499765
Received: 21 September 2024; Accepted: 26 November 2024;
Published: 18 December 2024.
Edited by:
Matthew Collins, University of Exeter, United KingdomReviewed by:
Karin Van Der Wiel, Royal Netherlands Meteorological Institute, NetherlandsCopyright © 2024 Sillmann, Raupach, Findell, Donat, Alves, Alexander, Borchert, Borges de Amorim, Buontempo, Fischer, Franzke, Guan, Haasnoot, Hawkins, Jacob, Mahon, Maraun, Morrison, Poschlod, Ruane, Shampa, Stephenson, van der Wel, Wang, Zhang and Županić. This is an open-access article distributed under the terms of the Creative Commons Attribution License (CC BY). The use, distribution or reproduction in other forums is permitted, provided the original author(s) and the copyright owner(s) are credited and that the original publication in this journal is cited, in accordance with accepted academic practice. No use, distribution or reproduction is permitted which does not comply with these terms.
*Correspondence: Jana Sillmann, amFuYS5zaWxsbWFubkB1bmktaGFtYnVyZy5kZQ==
Disclaimer: All claims expressed in this article are solely those of the authors and do not necessarily represent those of their affiliated organizations, or those of the publisher, the editors and the reviewers. Any product that may be evaluated in this article or claim that may be made by its manufacturer is not guaranteed or endorsed by the publisher.
Research integrity at Frontiers
Learn more about the work of our research integrity team to safeguard the quality of each article we publish.