- 1Laboratory of Tropical Crop Improvement, Division of Crop Biotechnics, KU Leuven, Heverlee, Belgium
- 2Bioversity International, Heverlee, Belgium
- 3International Institute of Tropical Agriculture, Arusha, Tanzania
- 4Bioversity International, Addis Ababa, Ethiopia
- 5Farming Systems Ecology, Wageningen University, Wageningen, Netherlands
- 6Bioversity International, Kampala, Uganda
Agroecological intensification (AEI) practices relying on on-farm diversity tend to close nutrient cycles and reduce dependency on external inputs in agricultural systems. These practices improve the productivity of banana-based systems in Uganda, but their extent of implementation differs between and within regions. However, the impact of AEI practices on a broader range of objectives including environmental and nutritional objectives, is hardly quantified. Additionally, recommendations to improve the farm performance, given these options, are lacking. We, therefore, analyzed the current farm performance for these broad range of objectives and explored optimal farm reconfigurations in two Ugandan districts, one in Central Uganda and one in Southwestern (SW) Uganda. Given the heterogeneity of smallholder farms, a farm typology based on the applied AEI practices was developed. It classified the subsistence farms in Central Uganda into two extreme groups with an average of 11.0 and 16.4 AEI practices applied per farm. Farms in SW Uganda were moderately intensified (i.e., 13.0 practices). The FarmDESIGN model revealed a higher species diversity, relatively higher profitability (2,039 – 3,270 $/ha/year) and nutritional yield on farms in Central Uganda. However, relatively high soil erosion levels (0.243 – 0.240) and negative nitrogen (N) balances (−72 to −50 kg N/ha/year) were indicative of unsustainable practices. In contrast, farms in SW Uganda were less diverse and more market oriented. Their commercial orientation allowed investments in soil fertility management, resulting in more sustainable [low soil erosion level (0.172) and positive N balance (5 kg/ha/year)], but less profitable (506 $/ha/year) systems. To improve farm performance, bananas and other perennials played a key role. Explorations with Calliandra calothyrsus (Calliandra) hedgerow or Mucuna pruriens (Mucuna) cover crop increased on-farm mulch production, improved sustainability indicators and profitability. We conclude that AEI practices can improve farm performance, and a more intensive use would be beneficial. In addition, the FarmDESIGN model provides a useful tool for redesigning these farms, proposing different redesigns depending on farmers' objectives (profitability, productivity or sustainability), and for evaluating ex ante the impact of new agricultural measures on farm performance.
Introduction
East African highland bananas and ABB type cooking/beer bananas (Musa spp.) are important components of the production landscape in Uganda (Gold et al., 2002; Němečková et al., 2018), especially in the country's Central and South-western region (UBOS and MAAIF, 2010). As a cheap all year-round energy source, rich in vitamin A, B6 and C, bananas contribute to small-scale farmers' food and income security (Frison and Sharrock, 1999; Abele et al., 2007). However, over the past 16 years, banana yield decreased with 1.5 tons/ha, resulting in an average yield of 4.3 tons/ha in 2017 (FAO, 2019)1. Increased land pressure due to rising population densities (Fermont et al., 2008), soil fertility decline (Stoorvogel and Smaling, 1990; Bazira et al., 1997; Bekunda and Manzi, 2003), drought (Wairegi et al., 2010; van Asten et al., 2011) and biotic stresses of pests, diseases and weeds (Tinzaara et al., 2009, 2018) are reported to contribute to this yield decline.
Sustainable intensification of the land currently under agricultural production is required to avoid further expansion into forest- or non-cultivated land (Wairegi and van Asten, 2010). Although fertilizer application is reported to have beneficial effects on banana production (Meya et al., 2020), adoption remains low due to high prices, low availability and poor market access. The practice is also only profitable in regions with good farm gate prices (e.g., close to Kampala) and good crop responses (e.g., Southern-Uganda) (Wairegi and van Asten, 2010). Therefore, agroecological intensification (AEI) practices that enhance the profitability, sustainability and nutritional yield on smallholder farms could offer opportunities for substantial improvement of the performance of these farms. As defined by Wezel et al. (2015), “AEI is a way of improving the performance of agriculture while minimizing environmental impacts and reducing dependency on external inputs through integration of ecological principles into farm- and system management.” Besides increasing production and minimizing environmental impact, elements such as social and cultural perspectives are also included. Practices like mulching, intercropping, crop rotations, integrated soil- and nutrient management, soil- and water conservation, integrated pest management and biological control strategies and a balanced and more efficient use of fertilizers are mentioned as possible AEI strategies by different authors (Gliessman, 2000; Côte et al., 2010; Karamura et al., 2013; Vanlauwe et al., 2013; Wezel et al., 2015).
Several studies report the application of AEI practices on banana-based farms in Uganda and the Great Lakes region (Katungi et al., 2006; Jassogne et al., 2013; Mpiira et al., 2013; Ocimati et al., 2013; Ssebulime et al., 2017). Here, bananas are integrated in a wide range of cropping systems, ranging from monocultures to intercropping- and mixed farming systems (Karamura et al., 1998). While in Uganda's Southwestern region banana plots are more likely to be pure stands, crop diversity within the banana field increases toward the country's Central region. Beans (Phaseolus vulgaris) (Karamura et al., 1998), coffee (Coffea spp.) (Jassogne et al., 2013) or agroforestry species such as jackfruit (Artocarpus heterophyllus), natal fig (Ficus natalensis), albizia (Albizia coriaria) and mango (Mangifera indica) are often intercropped with banana (Mpiira et al., 2013). However, the system's performance (profitability, productivity and sustainability) in general and the effect of the different farm components on farm performance, more specifically, are not yet fully quantified. Moreover, recommendations on how to intensify banana-based systems with these AEI practices are lacking (Ssebulime et al., 2017).
Therefore, this study focuses on the quantification of current banana-based systems in terms of their profitability, sustainability (soil N balances and soil erosion levels) and nutritional yield (dietary energy, vitamin A and iron) at the farm system level. Additionally, windows of opportunities for the improvement of the systems, given the initial farm components, are explored. As smallholder farms are characterized by a large array of farm components, all interrelated, the FarmDESIGN model (Groot et al., 2012; Ditzler et al., 2019), was used to facilitate the redesign process. The different agroecological and socio-economic conditions farmers are faced with, also result in a large diversity between farms even within the same geographic region. In addition, production constraints faced by farmers determine their possibilities to adopt intensification practices. To overcome the potential challenges due to the heterogeneity among farmers, this study uses farm typologies to group farmers into more homogeneous groups on the basis of the currently applied AEI practices.
Materials and Methods
Project, Site Selection, and Study Area
The fieldwork was conducted in two Ugandan districts: Kiboga district [0° 49′ 48.8″ N, 31° 51′ 36.8″ E; 1,210 meters above sea level (m.a.s.l.)] in Central Uganda and Isingiro district [0° 50′ 43.8″ S, 31° 0′ 30.1″ E; 1,510 m.a.s.l.] in SW Uganda. The two study sites are diverse so that most findings could become applicable to other banana production zones with similar production systems and constraints in the East and Central African region. Additionally, differences in AEI practices depending on the production orientation of the banana-based farming systems can be explored since production of bananas in Kiboga is mainly oriented toward home consumption, while farmers in Isingiro mainly produce for the market.
Both districts are characterized by a mean annual temperature of 21°C. Besides, a bimodal rainfall pattern is present in both regions, consisting of two rainy seasons, one from April until May and the other from September until December. The total annual precipitation in Kiboga is 1,200 mm, while in Isingiro it is 1,140 mm (FAO, 2005)2.
Farm Typology
Dataset
In order to capture the diversity among farms considering AEI practices, 120 farm households were selected in a first round of interviews using the snowball and maximum variation sampling approach. Thus, farmers and/or key informants were interviewed to identify very and less diverse farms in their neighborhoods to capture the conventional as well as rarely adopted AEI practices. In total, 60 households each were visited in Lwamata sub-county in Kiboga district, and Rugaaga sub-county in Isingiro district. During the interviews, a preliminary questionnaire was used, to collect both qualitative and quantitative information on farm, farm location, and household and farm management, including the farm, household and livestock size, number of cultivated crop species, number and type of applied agro-ecological practices, and the production orientation, constraints and objectives.
Typology Construction
Sixteen potential key variables were selected to cluster the farms according to their AEI level (Table 1). As in Alvarez et al. (2018), multivariate statistics, executed in R (version 3.5.1), were used for the typology construction. By drawing pairs plot, boxplots and histograms, the data were checked for missing values and possible outliers, which were subsequently removed. In addition, key variables with a non-normal distribution were transformed to a more normal distribution. Out of the 120, 112 households were retained and the logarithm (log10) and square root function applied to the variables “farm size” and “tropical livestock units,” respectively. Tropical livestock units (TLU) were calculated according to FAO guidelines (Otte and Chilonda, 2002), using the conversion factors : cattle = 0.70, pigs = 0.20, sheep and goats = 0.10, and chickens = 0.01. These factors were multiplied by the number of each animal species present on farm and summed up to come up with the final TLU value. The transformed variables are represented by the codes area2 and tlu2, respectively.
In order to reduce the number of key variables into non-correlated dimensions, the Factor Analysis of Mixed Data (FAMD) was executed with the packages “factoextra” (version 1.0.5) and “FactoMineR” (version 1.41). However, highly correlated key variables resulted in a poor outcome of the factor analysis. Therefore, key variables with the lowest contribution to the dimensions were omitted during several rounds of analysis, until only seven variables were kept for the final grouping. These variables included five quantitative (farm size, tropical livestock units, number of cultivated crop species, number of applied AEI practices, number of agroforestry species) and two qualitative variables (farm location and farmer's objectives). To determine the number of dimensions kept in the analysis the Kaiser criterion was used, to keep only the dimensions with an eigenvalue larger than one. The remaining dimensions were used for further analysis to classify the farms into different groups.
The clustering analysis was performed by the HCPC function (Hierarchical Clustering on Principal Components) from the “FactoMineR” package (version 1.41). This function starts with assigning every observation to its own cluster and subsequently merges the two most similar clusters until only one cluster is left (Crawley, 2013). The optimal number of clusters was found by using Ward's method, which maximizes both intra-cluster homogeneity and inter-cluster heterogeneity (Murtagh and Legendre, 2014). In order to identify the variables that characterized the different clusters, boxplots were drawn for the quantitative variables and statistical significances between the different clusters were detected with the pairwise Wilcoxon test. Moreover, a classification tree was built using the R package “rpart” (version 4.1–13). The Margalef index (M), representing species richness, and Shannon index (H), representing both species richness and evenness, were calculated for each identified cluster, based on the following formulas, respectively:
where S is the number of crop species present on farm, A the total farm area (m2) and pi the area proportion of crop i.
Farm Redesigns
Dataset
In order to calculate the performance of each farm type and reveal windows of opportunities for farm optimization, we used the FarmDESIGN model (Groot et al., 2012; Ditzler et al., 2019). Three farms from every cluster, identified by the farm typology, were selected for a second round of interviews. This selection was based on the willingness of the farmer to participate and on the quality of the information provided by the farmer during the previous interview. Additionally, farmers as close as possible to the cluster centers, as identified by the HCPC analysis, were selected. Then, a more detailed questionnaire was used, which captured necessary input variables for the FarmDESIGN model, including household and herd composition, and for each crop, crop yield, product destination, cultivation costs, labor requirements and market prices. Additional information such as, data on environmental and crop characteristics, like soil type and climatological conditions, N fixation and nutrient composition was obtained from secondary data available within the FarmDESIGN model or from literature (Gutteridge and Shelton, 1994; Groot et al., 2012; Kongkijthavorn, 2017; de Jager, 2018; HarvestPlus, 2018; USDA, 2018; World Agroforestry Centre, 2018a,b,c).
FarmDESIGN Model
Besides being able to calculate the performance of the original farm, the FarmDESIGN model can explore redesigns that outperform the original farm by using a multi-objective Pareto-based Differential Evolution algorithm. Considering as well-objectives as constraints, the performance of Pareto-optimal redesigns cannot be improved without compromising one of the other objectives (Groot et al., 2007). For every run, the number of iterations was set at 1,000. We wanted to maximize operating profit, dietary energy yield, vitamin A yield, iron yield and to improve the farm sustainability by minimizing N losses and soil erosion (objectives). Constraints were set so that the total crop area could not increase and that each fruit species could occupy maximally 0.4 ha. Redesigns varied in the area allocated to each (mixture of) crop(s) and destination of the crop products and residues (decision variables). A summarizing table listing the objectives, decision variables and constraints used for the FarmDESIGN model is given in Table S1. In addition, two scenarios were created, in which Calliandra calothyrsus (as hedge row) and Mucuna pruriens (as cover crop) were each separately introduced on farm to evaluate the effect on farm performance.
Each time the FarmDESIGN model was run, it generated 800 Pareto-optimal redesigns. Those redesigns which outperformed the original farm in all objectives were selected. As trade-offs between objectives made it impossible to reach the best performance for each indicator simultaneously, objectives had to be ranked to select the most optimal redesign. In this study, profitability was prioritized, followed by soil quality and nutritional yield improvement. As postulated by Kansiime et al. (2018), it is believed that higher incomes allow on-farm investments, which in turn can lead to increased soil quality, if the right investments are made, and hence improved sustainability.
The operating profit was calculated by subtracting both variable (for labor, manure, fertilizer and other inputs) and fixed costs (for land) from the crop and animal product revenues. To calculate the nutritional yield, expressed in persons/ha/year, the nutrient supply of the products, annually produced on one ha of land, was compared with the recommended daily reference intake multiplied by 365 days. The N balance was computed by considering both N inputs (symbiotic and non-symbiotic N fixation, atmospheric N deposition, and the N content of green manure, animal manure and fertilizers) and outputs (N content of exported crop and animal products, soil erosion and N volatilization) (Groot et al., 2012). Erosion levels were determined for each crop by the soil erosion C factor, defined as the ratio between soil losses on bare soil and those on land cultivated by every specific crop (FAO, 2001). Therefore, soil erosion C factors are dimensionless, ranging from zero to one, whereby low values are assigned to crops which cause limited soil erosion.
Due to time constraints, the outcome of the model runs was discussed with the interviewed farmers in Kiboga only during two focus group discussions. The best redesigns and scenarios were presented to the farmers with slides each representing the composition of the modeled farm, after which farmers opinions were asked.
Results
Farm Type Characterization
Rainfed cooking bananas (further referred to as bananas) were the major component in agricultural production systems in Kiboga and Isingiro. However, there are some considerable differences. In Kiboga, farms were smaller (on average 2.2 ha) compared to Isingiro, where the farm size was on average 6.8 ha. Crops like coffee, maize, beans and cassava were intensively intercropped with banana in Kiboga. Moreover, agroforestry species were present on all farms within the banana fields unlike hedges which were on 25% of the farms in that region. In Isingiro, maize, beans and cassava were also present, but farmers mainly focused on monocrops of each crop. Instead of plot diversity, these farmers invested more in soil and water-conservation practices. Here, mulch was applied on every farm, compared to 74% of the farms in Kiboga. Likewise, 80% of the farmers in Isingiro, compared to 58% in Kiboga, applied animal manure. The same trend could be noticed for the occurrence of water retention ditches: 78% in Isingiro compared to 39% in Kiboga.
To exploit the differences between farms in more depth, a multivariate analysis was executed. The first two dimensions of the Factor Analysis of Mixed Data explained together 60% of the total variability between farms, based on seven key variables. The first dimension explained 39% of the variability and was closely related to the farm location, farm size, number of agroforestry species and farmer's objectives, thus, expressing general farm information. The second dimension, explaining 21% of the variability, was mainly correlated to the number of applied AEI practices and seemed to explain farm management. The clustering analysis grouped the farms into four different farm types (Figure 1). However, since the third farm type (WC; wrongly classified) consisted of only two observations (Figure 1), these observations were classified according to the classification tree into the three remaining farm types: farm type LI (low intensified), MI (moderately intensified) and HI (highly intensified), ordered with increasing number of applied AEI practices (Figure 2).
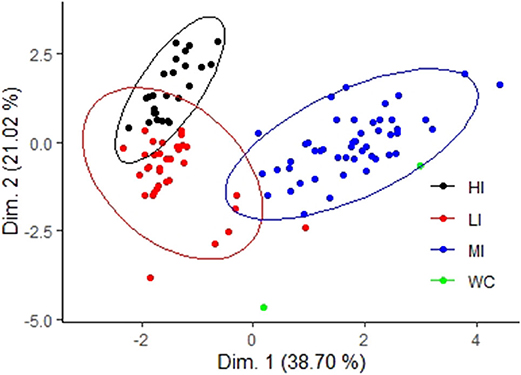
Figure 1. Hierarchical clustering of identified clusters LI, MI, HI, and WC, referring to low intensified farms, moderately intensified farms, highly intensified farms and wrongly classified farms, respectively.
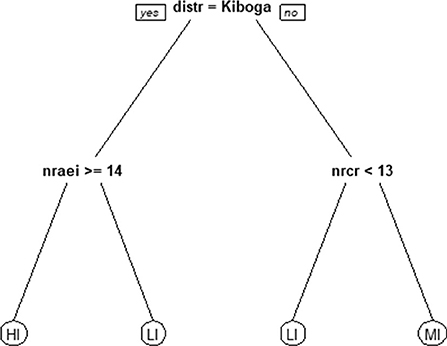
Figure 2. Classification tree, with overall accuracy of 82%, to classify the two observations from cluster WC into the three remaining clusters (LI, MI, and HI, referring to low, moderately, and highly intensified farms). Distr represents the considered district, nraei the number of applied agroecological intensification practices and nrcr the number of cultivated crop species.
Farm Type LI: Low Intensified Farms (n = 36, 32% of the Sampled Farms)
LI farms were the least intensified of the three farm types. On average, 11.0 out of the 34 AEI practices (Table S2) such as intercropping, integration of agroforestry species, application of kitchen and household waste and manual control of pests and diseases were applied in these farms (Figure 3). Additionally, LI farms were the smallest (1.41 ha; 6.3 household members), with the smallest number of cultivated crop species (11.1) including banana, coffee, maize, beans, cassava and groundnuts. Annual species covered about 49% of the land. Perennials mainly comprised banana and coffee. However, 10% of the land was allocated to trees, i.e., 7% agroforestry species and 3% fruit species (Table S3). While the fruit species [i.e., Artocarpus heterophyllus (jackfruit), Mangifera indica (mango), Persea americana (avocado)] were mainly grown as an additional food source for the farm household, agroforestry species (i.e., Eucalyptus grandis, Maesopsis eminii, Albizia coriaria, Erythrina abyssinica, and Syzigium cuminii) were valued for shade, fodder, mulch, firewood and timber. Hedges, in contrast, were not abundant. If present, hedges were used as a mulch, fodder and/or firewood source. LI farms had a Margalef index, indicating species richness, of 2.32. An index of 0 represents monocultures, while larger indices indicate more species in a determined area or the same amount of species on a smaller area. The Shannon index, a measure of both species richness and evenness mainly ranging from 1.5 to 3.5, reached a level of 2.12, indicating that the relatively high number of species were more or less evenly distributed on farm. Few animals, like chickens and pigs were on farm, resulting in the lowest TLU of 0.45 amongst all farm types. While chickens were free ranging, pigs were tethered in the farmyard. These least intensified farms were mainly located in Kiboga (83.3%) and managed by subsistence farmers who mainly produced for home consumption.
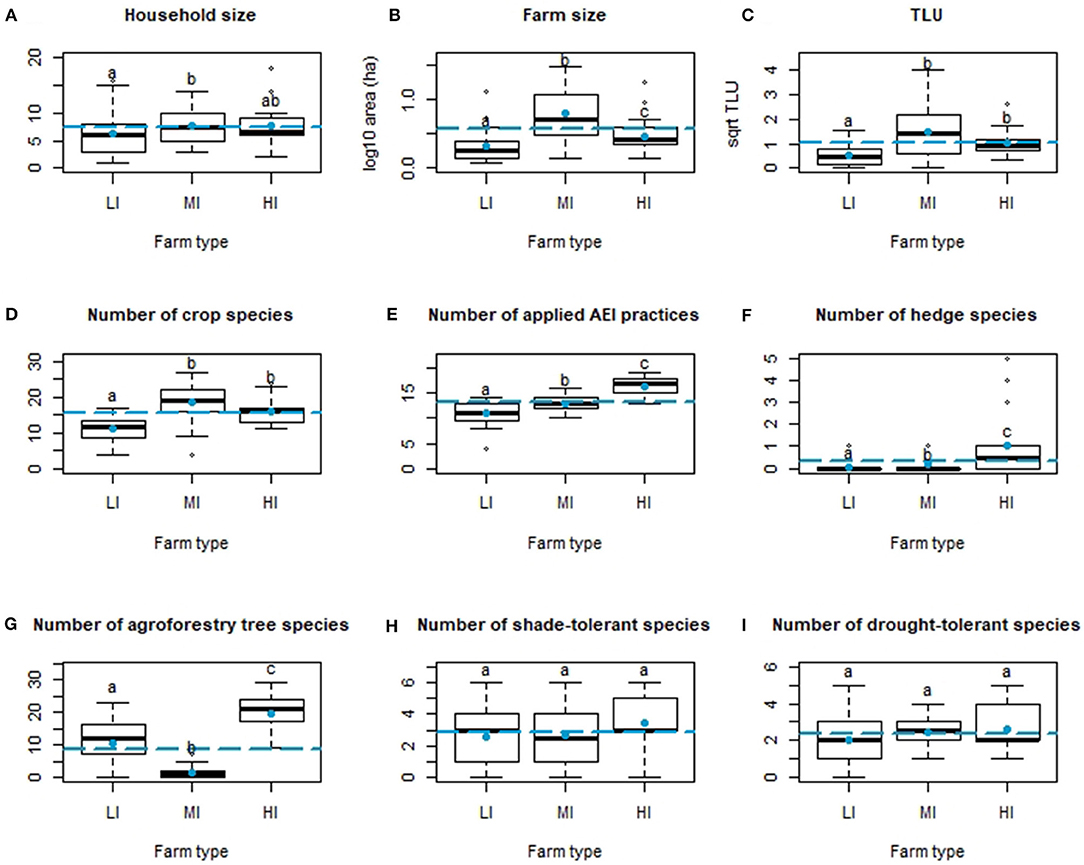
Figure 3. Boxplots of the three farm types, ordered by increasing number of applied AEI practices (LI: low intensified farms, MI: moderately intensified farms and HI: highly intensified farms), for the variables household size (A), farm size (B), tropical livestock units (C), number of cultivated crop species (D), number of applied AEI practices (E), number of hedge species (F), number of agroforestry species (G), number of shade- and drought-tolerant species (H,I). Blue points represent the cluster means, thick solid horizontal lines the median values, box outlines the interquartile range containing the middle 50% of values, whiskers the 90th percentile values, gray points the outlier values and the dashed blue line the survey means of each variable. Small letters above the whiskers of the boxplots represent the output of the pairwise Wilcoxon test. Cluster means that are significantly different at the 0.05 significance level are indicated by a different letter. Only boxplots of the same quantitative variable can be compared.
Farm Type MI: Moderately Intensified Farms (n = 50; 45% of the Sampled Farms)
In MI farms, defined as the moderately intensified farms, 13.0 AEI practices were on average applied (Figure 3). Unlike farm type LI, MI farms were the largest (7.57 ha; 7.8 household members). Although about 80% of the total land area was covered by banana, many crop species (18.8), like beans, maize, groundnut and millet, were cultivated mainly as monocrops. Crops grown for home consumption only, such as eggplants, leafy vegetables and pumpkins, were found on a very small to negligible scale, consisting of one or two plants per species and this was reflected by the species indices. The Margalef index was the smallest for all farm types and reached a level of 1.32. Moreover, the relatively small number of species was not evenly distributed (Shannon index = 0.92). Perennials other than banana occupied 1% of the land and included Carica papaya (papaya), Ricinus communis and Eucalyptus grandis. While the former two species were grown within the banana fields, the latter was allocated to a separate field to avoid possible competition for water, soil nutrients and solar radiation with the cultivated crops. Cattle, goats and chickens were managed by the farm household (TLU = 3.32). While cattle and goats were on communal grazing areas during day time and kept in kraals overnight, chickens were found in the farmyard. This resulted in a large availability of animal excreta, which was applied on the banana field(s). In addition to application of farmyard manure on banana fields, kitchen and household waste, mulch and external cow dung were applied and soil and water retention ditches dug. These farms were mainly (98%) located in Isingiro, where farmers had commercial objectives.
Farm Type HI: Highly Intensified Farms (n = 26; 23% of the Sampled Farms)
Intercropping, integration of agroforestry- and hedge species, application of kitchen and household waste, mulch, manure and even mineral fertilizers were some of the 16.4 AEI practices applied on HI farms (Figure 3). These highly intensified farms had, on average, a size of 2.59 ha (7.6 household members), of which 45% was allocated to annual crop species, such as maize, sweet potato, cassava, beans and tomato. Coffee and banana occupied together about 50% of the total area. Half of the remaining land was covered by agroforestry/hedge species while the other half with fruit tree species (i.e., jackfruit, mango and avocado). Additionally, these farms were characterized by the highest diversity of agroforestry and hedge species, with on average 19.9 and 1.0 species, respectively. The five most occurring agroforestry species were Markhamia lutea (96%), Albizia coriaria (92%), Maesopsis eminii (92%), Ficus natalensis (85%), and Vernonia amygdalina (85% of HI farms). Hedges occurred on 50% of the farms and were used by 77% of these households as a fodder, mulch and/or firewood source. Especially Calliandra calothyrsus and Morus spp. were used for these production objectives. HI farms were highly diverse with a Margalef index of 3.12. Additionally, the relative high number of species were equally divided among the available land (Shannon index = 2.49). As for all farm types, animals were kept on farm. Here, livestock mainly consisted of goats, pigs and chickens, resulting in a TLU of 1.25. While the former two were mainly zero grazed, chickens were free ranging in the farmyard. This type of farmers were all located in Kiboga district, defining themselves as subsistence farmers.
Performance of the Farms
The different compositions of the farm types resulted in contrasting farm performances, as calculated by the FarmDESIGN model using the inputs of the second round of interviews (Table 2). LI (with a profit of 2,039 $/ha/year) and HI (with a profit of 3,270 $/ha/year) farms, predominantly located in Kiboga, were more profitable compared to MI farms (with a profit of 506 $/ha/year), mainly located in Isingiro. The species rich LI and HI farms were also able to meet the dietary requirements of a relatively larger number of people compared to the less diverse MI farms. However, the relatively high soil erosion levels and negative N balances indicated unsustainable farming practices for LI and HI farms. In contrast, MI farms in Isingiro were more sustainable given the positive N balance (+ 5 kg N/ha/year) and the relatively low soil erosion level (0.172).
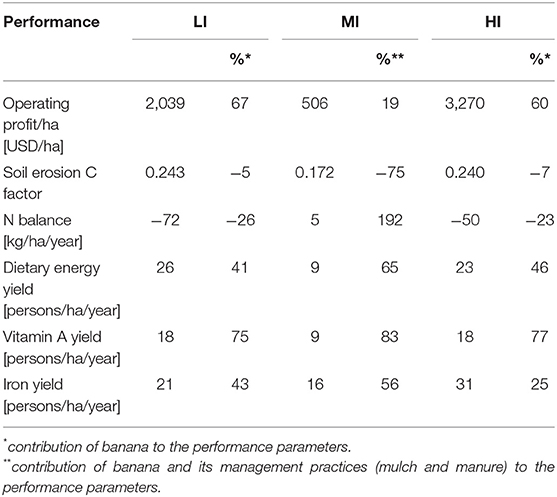
Table 2. Performance of the farm types and the contribution of banana to the performance parameters (%), as calculated by the FarmDESIGN model.
As the major farm component, bananas were paramount in those farms (Table 2). They were the major vitamin A supplier and provided 60 to 67% of the total profit in Kiboga, but only 19% in Isingiro. If income from sales was considered, bananas provided 31% of the total farm income in the latter region, although still reaching lower levels than expected. The sale of tomato and cassava contributed 24 and 25% to the total farm income in Isingiro, respectively (data not shown). However, bananas were responsible for the sustainability of MI farms by reducing soil erosion by 75% and improving the N balance by 192%, meaning that more nitrogen is added to the banana plot than is removed by its harvest.
Farm Redesigns
An Optimal Redesign
In order to improve the farm performances (profitability, productivity and/or sustainability), redesigns of each farm type were generated by multi-objective optimization by first varying the area allocated to every species, already present on the farm and, hence, without introducing new species. As an illustration, out of the clouds of redesigns, one Pareto-optimal redesign which outperformed the original farm in terms of all objectives was chosen for each farm type (Table 3A). Unlike for farm type MI, whereby all objectives could be enhanced, farm composition changes did not profoundly improve the N balance in farm type LI, while in farm type HI N balance improved but still remained negative. Nevertheless, profits could be doubled and even quadrupled. Also, the nutritional parameters were improved.
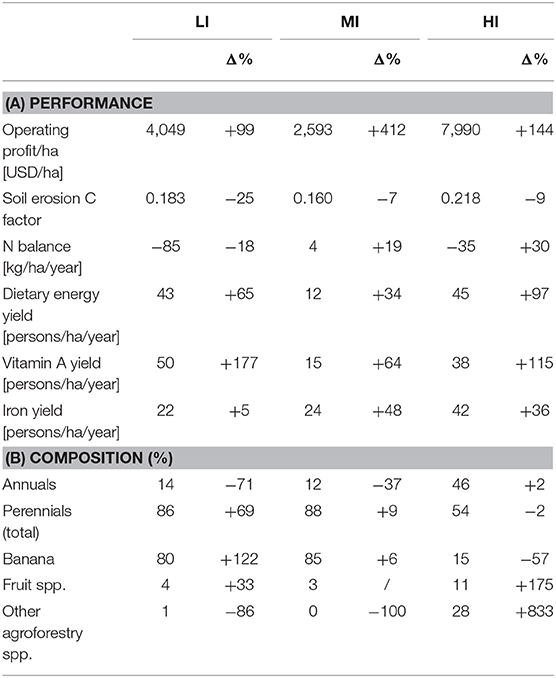
Table 3. Performance (A) and composition (B) of the best redesigns for each farm type, as calculated by the FarmDESIGN model, and the percentage change in objective (A) and farm components (B) relative to the original farm performance.
To obtain these performances, perennials seemed to be important, covering 54% to 88% of the total farm area (Table 3B). While for farm type LI and MI, banana was the main perennial on farm, agroforestry tree species and other perennials comprised the largest area allocated to perennials on farm type HI. Erythrina abyssinica, Pennisetum purpureum, and Maesopsis eminii were the most prominent perennial species here, comprising each 86%, 4% and 3% of the total area under perennial species other than banana, respectively (data not shown). Considering annuals, cassava occupied the largest area in all redesigns (56, 22, and 43% of the total area under annuals on farm type LI, MI, and HI, respectively). The rest of the area was mainly allocated to maize, tomato, peas and cabbages. The detailed composition of the original farm types and the best redesigns can be found in Tables S3, S4, respectively.
For a given farm type, the model generated a wide range of redesigns (Table 4A), allowing farmers to choose a redesign in line with their own preferred ranking of objectives. However, trade-offs between objectives impeded the existence of compositions which performed better for all objectives simultaneously than the original farm composition. Increased nutritional yield, for instance, was related to a reduced N balance. Likewise, a positive N balance on farm type HI was associated with a lower operating profit. Moreover, the variation of the performance was not equally large for all farm types. For farm type LI, only three better performing but similar redesigns were identified by the model.
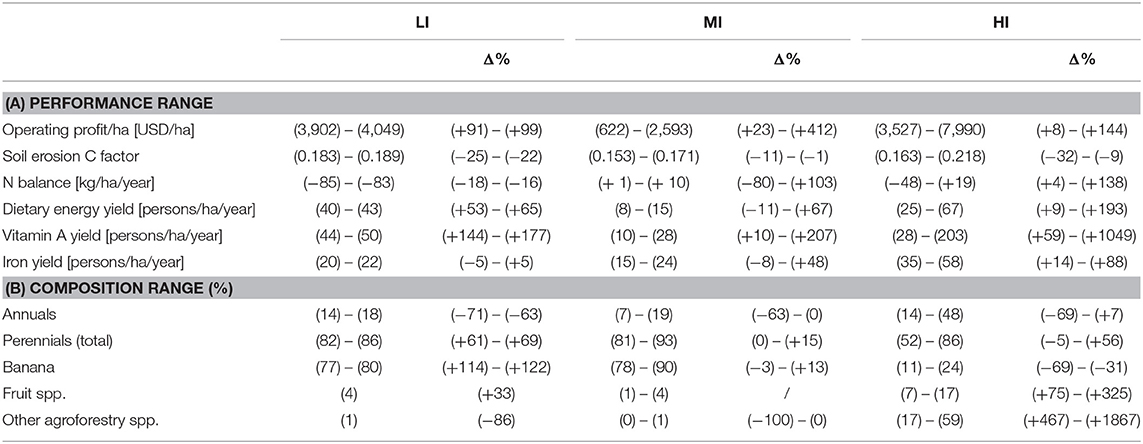
Table 4. Performance range (A) and composition range (B) of the redesigns outperforming the original farm, as calculated by the FarmDESIGN model, and the percentage change in objective (A) and farm components (B) relative to the original farm performance.
Similar to the best performing composition (Table 3) perennials accounted for more than 50% of the total farm area in these redesigns (Table 4B), with at least 80% of land allocated to banana on farm types LI and MI. In contrast, agroforestry tree species, specifically E. abyssinica accounted for the largest area under perennials on farm type HI. To increase the area under perennials, the area under annuals had to decrease in most cases. Major annual crops were cassava, maize and beans on farm type LI, cassava, tomato, groundnuts, beans and peas on farm type MI and cassava and cabbages on farm type HI (data not shown).
Explorations of New Species on Farm
Besides changing the compositions of the initial components, new species, which potentially reduce the dependency on external inputs, can be added to the model to investigate their effect on the farm performance. In a first scenario Calliandra calothyrsus, a N fixing species with high biomass production (8.5 tons DM/ha/year; Kongkijthavorn, 2017), was integrated as a hedge around farmers' fields in the model. Explorations by the FarmDESIGN model revealed that with exception of the N balance for farm type LI, all redesigns with C. calothyrsus outperformed the original farm type for all objectives considered (Table 5A). Again, perennials played a key role (Table 5B): i.e., banana on farm type LI and MI, occupying 80% and 87% of the total area, respectively, and other agroforestry species on farm type HI, covering 52% of the total area. Here, E. abyssinica was the most prominent, comprising 91% of the area allocated to agroforestry species. Important annuals were cassava on farm type LI, tomato and Solanum potato on farm type MI and cabbages and sweet potato on farm type HI, covering 69, 27, 13, 18, and 18% of the area allocated to annuals, respectively (data not shown).
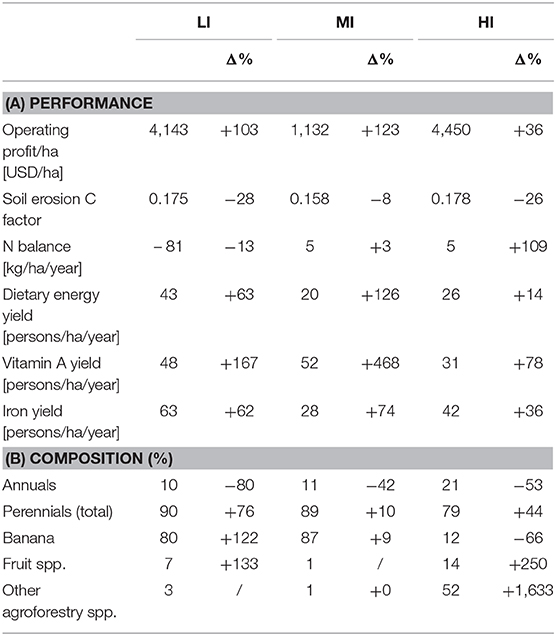
Table 5. Performance (A) and composition (B) of the best redesign with C. calothyrsus integrated on farm, as calculated by the FarmDESIGN model, and the percentage change in objectives (A) and farm components (B) relative to the original farm performance.
In a second scenario, the N fixing cover crop Mucuna pruriens was integrated below the banana canopy on farm type MI. This introduction alone, without changing any of the current farm components, reduced soil erosion and farm profitability by 5% and tripled the N balance.
Discussion
Farms differ in terms of land accessibility, resource endowment, production and consumption decisions, experience and management skills of the owners (Tittonell et al., 2010; Alvarez et al., 2014). These differences influence the interest and capacity of farmers to adopt AEI strategies that can potentially improve the farm performance (Kuivanen et al., 2016). Therefore, the classification of farmers in more homogenous groups facilitates tailor-made recommendations and allows to increase adoption rates of new technologies by farmers.
Farm Type Characterization
In this research, farm typology revealed different farm management strategies across both districts. These differences can be explained by the difference in farm size. With an average farm size of 7.57 ha, MI farmers cultivated their crops as pure stands to reach the household dietary requirements. Moreover, the practice of intercropping did not fit within their mindset, as, according to these farmers, species integrated within the banana plot compete for water, soil nutrients and solar radiation. Therefore, E. grandis was cultivated in a separate field, while beans were planted at the border of the banana plot and only specific species, like papaya and R. communis, were grown within the field. The latter two species are characterized by a limited above-ground branching habit resulting in limited competition for light (CABI, 2018)3. However, below-ground, papaya is characterized by an extensive root system in the first 30 cm of the soil layer, which can potentially result in competition with the banana plants, while R. communis has a tap root system with prominent lateral roots, therefore, avoiding competition with other crops (CABI, 2018)3. The limited available land in Kiboga (farm types LI and HI) forced farmers to increase the production intensity by intercropping, integrating fruit- and other agroforestry species to meet family dietary needs and keep their land productive. Integration of several crops on the same piece of land has been reported to be a smallholder strategy to increase land- and other resource-use efficiency (Sileshi et al., 2007) and minimize risks of crop failures (Dapaah et al., 2003).
In addition, farm size is reported to be linked to market participation, i.e., the larger the farm size the more these farms are involved in sales (Tittonell et al., 2010; Chapoto et al., 2013; Kuivanen et al., 2016), suggesting that MI farmers are more oriented toward the market than type LI and HI farmers. Moreover, profits from sales can, on the one hand, be invested in soil amendments, such as mulch and animal manure, to compensate for nutrient losses, and, on the other hand, in expansion of the herd (Kuivanen et al., 2016). Besides, the large farm size enables farmers to keep more livestock to provide manure and fertilize the fields. In addition, animals can be kept as a form of insurance. During periods of cash shortage, they might be sold to pay medical bills and school fees. Among all animal species, cattle are the most valuable. Indeed, MI farmers owned the largest cattle herds, while in Kiboga, where farms were smaller and market participation was less, livestock mainly consisted of small ruminants. These differences in management practices were reflected by the farm performances. While farmers in Isingiro invested in soil fertility by buying mulch and animal manure, farmers in Kiboga did not, resulting in less profitable but more sustainable farms in Isingiro, compared to profitable but unsustainable farms in Kiboga. These results are supported by findings of Kansiime et al. (2018), who showed that labor and fertilizers were more efficiently used on farms with a commercial orientation, suggesting that the expectation to generate farm income motivates farmers to produce more efficiently.
In Kiboga the number of applied AEI practices differed significantly between both farm types (cf. 11.0 practices on farm type LI and 16.4 on farm type HI). The fewer number of AEI practices on LI farms may be due to the small land LI farmers owned. Tittonell et al. (2010) showed that poor resource-endowed (land and labor) farmers face constraints, such as education and health for several generations. This limits their interest and possibilities to adopt new intensification strategies. Hence, lack of knowledge or adequate labor forces could be given as some of the reasons for the lower adoption of AEI practices on LI farms compared to HI farms.
Farm Redesigns
The current performance of the different farms urged for improvement measures in terms of all objectives considered, i.e., operating profit, sustainability in terms of soil erosion and N balance, and nutritional yield. Farmers' information, acquired during the interviews, revealed that income from sales of commonly cultivated crops, like banana, coffee, maize, beans and groundnuts, was relatively low. Additionally, commonly eaten food products by the Ugandan population are rich in carbohydrates, while vitamins and minerals are often lacking. Fruits and vegetables showed potential to increase farm profitability and nutritional yield.
In order to improve the farm performance, the FarmDESIGN model was run for each farm type. Optimal redesigns, i.e., compositions which outperformed the original farm in almost all objectives, with positive N balances attributed more than 50% of the total farm area to agroforestry species. The limited available land on farm type LI seemed to be the biggest limitation for improving the N balance. Since a large area needed to be assigned to crops and fruits to improve both profitability and nutritional yield, not enough space was left for agroforestry species, necessary to maintain adequate soil fertility levels.
However, concerns of farmers in Isingiro may be appropriate, as some agroforestry species can exert above- and/or below-ground competition with intercropped species. Agroforestry species with complementary characteristics to banana, i.e., a limited above-ground branching habit or a canopy with sufficient light transmission and a low competitive or deep root system, offer opportunities to improve the system. Of the commonly integrated species in Kiboga, only A. coriaria and F. natalensis are suitable companion species to banana. As a N fixing species with a deep tap root and sparse canopy, A. coriaria produces good decomposable litter (Buyinza et al., 2019; Ssebulime et al., 2019). Also F. natalensis produces litter with a sufficient decomposition rate (Ssebulime et al., 2019). In contrast, while the dense canopy of M. lutea and fruit species, like jackfruit, mango and avocado, prevent sufficient solar radiation transmission to the crops underneath, the extensive and competitive root system of papaya and M. eminii compete for soil nutrients (Wajja-Musukwe et al., 2008; World Agroforestry Centre, 2018b)4. However, species unsuitable as upper canopy species, can also be planted in hedgerows to avoid above-ground competition through heavy foliage. Additionally, pruning can improve solar radiation transmission, although, as above- and below-ground biomass is balanced (Van Noordwijk and Purnomosidhi, 1995), it also changes the root system, leading to a reduced rooting depth and increased root branching in the topsoil (Schroth, 1995). Despite potential competition with crops, integrating agroforestry species can have many advantages. They might recycle nutrients leached to deeper soil layers, aggregate soil particles and, thus, increase the water holding capacity and water infiltration and reduce erosion (Giller and Wilson, 1991).
Besides the scientific prove that an agroecological intensification practice is effective, farmers' willingness to adopt the practice is also of utmost importance. Therefore, two feedback sessions with farmers in Kiboga were organized, one for each farm type. All farmers showed great interest in integrating M. pruriens as a cover crop underneath the banana canopy. Since weeds, lack of mulch and labor forces were a big concern, M. pruriens can offer a solution here. Some farmers, who wanted to keep small ruminants, were more interested in integrating C. calothyrsus as a hedge around their homestead and field. These findings suggest that future research should focus on a more in-depth exploration of these redesigns in close collaboration with the farmers (Le Gal et al., 2011).
Model Perspectives
Various tools for the analysis and exploration of strategic improvements in farming systems have been developed in the last decades, each using different programming techniques and algorithms (Dogliotti et al., 2005; Tittonell et al., 2007a,b; Groot et al., 2012). An advantage of the FarmDESIGN model is the generation of a large set of alternative farm designs, providing rapid insights into the consequences of a range of reconfigurations on farm performance (Groot et al., 2012). Moreover, synergies and trade-offs among different objectives are revealed by the Pareto-based multi-objective optimization algorithm (Groot and Rossing, 2011). This makes the model a useful tool to support farmers in their management-related decision making and facilitates the discussion on alternative production systems between researchers and farmers.
It should be noted that all calculations were purely based on farmers' estimations. Therefore, detailed measurement of essential model inputs and confirmation of outputs by on-farm trials will be necessary to validate the FarmDESIGN results. However, estimates as reported in this study, can be a good reflection of reality. Additionally, animal feed balance, nutrient cycles and efficiency calculations in the model allow to check the correctness of the estimated material flows at the core of the model. Hence, the FarmDESIGN model is a suitable tool to determine the overall performance given the entangled system variables and reduces the need for risky, costly and time-consuming trial and error experiments.
Conclusion
As the agroecological and socio-economic constraints faced by farmers differ a lot between and even within the same geographic locations, the adoption potential of new technologies depends on each individual. Farm size, location and orientation (market vs. home-consumption) were the major factors influencing the number and type of adopted AEI practices on farm. While in Kiboga (Central Uganda, 1,210 m.a.s.l.; 1,200 mm/year; small to medium sized farms; home-consumption) farms tended to be more diverse, farmers in Isingiro (SW Uganda; 1,510 m.a.s.l.; 1,140 mm/year; large sized farms; market oriented) mainly focused on banana monocultures. Unlike in Kiboga, farmers in Isingiro invested in mulch and animal manure and controlled soil erosion by digging water retention ditches, resulting in a more sustainable farming system. In contrast, farms in Kiboga were relatively profitable, but soil quality parameters forecast unsustainable farming practices. Banana, as the major cash crop, was of key importance for the overall farm performance, in particular for the operating profit, vitamin A yield and reduction of soil erosion. Agroforestry species were fundamental to improve the N balance, requiring a coverage of at least 50% to obtain positive N balances. Composition changes, suggested by the FarmDESIGN model, allowed to double or even quadruple the operating profit, increase the nutritional yield by 5% to 180% and improve the sustainability by 10% to 30%. We conclude that the FarmDESIGN model is a useful tool to evaluate ex ante the impact of integrating theoretical promising species on farm performance and shows potential to be used as a tool in support of agricultural policy measures.
Data Availability Statement
The raw data supporting the conclusions of this article will be made available by the authors, without undue reservation, to any qualified researcher.
Author Contributions
WO, RS, RR, and GB supervised the experiments. CG and WO performed the experiments and analyzed the data. CG, WO, RS, and JG wrote the manuscript. All authors reviewed and approved the final manuscript.
Funding
The authors thank all donors who supported this work through their contributions to the CGIAR Fund (http://www.cgiar.org/who-we-are/cgiar-fund/fund-donors-2/), and in particular to the CGIAR Research Program Roots, Tubers and Bananas (RTB-CRP). We acknowledge VLIR-UOS for the financial support during the research stay of CG in Uganda. We are grateful for the financial support from the Belgian Directorate General for Development through the Consortium for Improving Agriculture-based Livelihoods in Central Africa (CIALCA).
Conflict of Interest
The authors declare that the research was conducted in the absence of any commercial or financial relationships that could be construed as a potential conflict of interest.
Acknowledgments
We would like to thank Francis Kwezi, Frederik Mabirizi, and Perex Mujuzi for the smooth organization of the fieldwork. The interviewed farmers in Kiboga and Isingiro are gratefully acknowledged for their participation in this study.
Supplementary Material
The Supplementary Material for this article can be found online at: https://www.frontiersin.org/articles/10.3389/fsufs.2020.00087/full#supplementary-material
Footnotes
1. ^http://www.fao.org/faostat/en/#data/CC
2. ^http://www.fao.org/land-water/land/land-governance/land-resources-planning-toolbox/category/details/en/c/1032167/
4. ^http://www.worldagroforestry.org/treedb2/speciesprofile.php?Spid=17981
References
Abele, S., Twine, E., and Legg, C. (2007). Food Security in Eastern Africa and the Great Lakes. Ibadan, Nigeria: IITA.
Alvarez, S., Paas, W., Descheemaeker, K., Tittonell, P., and Groot, J. C. J., (Eds.). (2014). Constructing Typologies, a Way to Deal with Farm Diversity: General Guidelines for the Humidtropics. Report for the CGIAR Research Program on Integrated Systems for the Humid Tropics. Plant Sciences Group, Wageningen University, Netherlands.
Alvarez, S., Timler, C. J., Michalscheck, M., Paas, W., Descheemaeker, K., Tittonell, P., et al. (2018). Capturing farm diversity with hypothesis-based typologies: an innovative methodological framework for farming system typology development. PLoS ONE 13:e0194757. doi: 10.1371/journal.pone.0194757
Bazira, H., Bekunda, M. A., and Tenywa, J. S. (1997). Decomposition characteristics of mixed grass and banana residues and their effects on banana plant performance. African Crop. Sci. Conf. Proc. 3, 421–428.
Bekunda, M., and Manzi, G. (2003). Use of the partial nutrient budget as an indicator of nutrient depletion in the highlands of southwestern Uganda. Nutr. Cycl. Agroecosystems 67, 187–195. doi: 10.1023/A:1025509400226
Buyinza, J., Muthuri, C. W., Downey, A., Njoroge, J., Denton, M. D., and Nuberg, I. K. (2019). Contrasting water use patterns of two important agroforestry tree species in the Mt elgon region of Uganda. Aust. For. 82, 1–9. doi: 10.1080/00049158.2018.1547944
Côte, F., Tomekpe, K., Staver, C., Depigny, S., Lescot, T., and Markham, R. (2010). Agro-ecological intensification in banana and plantain (Musa spp.): an approach to develop more sustainable cropping systems for both smallholder farmers and large-scale commercial producers. Acta Hortic. 879, 457–464. doi: 10.17660/ActaHortic.2010.879.50
CABI (2018). Invasive Species Compendium. CABI. Available online at: https://www.cabi.org/isc/ (accessed November 13, 2018).
Chapoto, A., Mabiso, A., and Bonsu, A. (2013). Agricultural Commercialization, Land Expansion, and Homegrown Large-Scale Farmers: Insights from Ghana. IFPRI Discussion Paper 1286. Washington, DC: International Food Policy Research Institute (IFPRI). doi: 10.2139/ssrn.2343154
Dapaah, H. K., Asafu-Agyei, J. N., Ennin, S. A., and Yamoah, C. (2003). Yield stability of cassava, maize, soya bean and cowpea intercrops. J. Agric. Sci. 140, 73–82. doi: 10.1017/S0021859602002770
de Jager, T. I. (2018). Diverging perspectives on diversification - a multi-objective pareto-based optimisation for smallholder farmers under banana xanthomonas wilt (BXW) pressure in Uganda (dissertation). Farming Systems Ecology Group, Wageningen University: Netherlands.
Ditzler, L., Komarek, A. M., Chiang, T. W., Alvarez, S., Chatterjee, S. A., Timler, C., et al. (2019). A model to examine farm household trade-offs and synergies with an application to smallholders in Vietnam. Agric. Syst. 173, 49–63. doi: 10.1016/j.agsy.2019.02.008
Dogliotti, S., Van Ittersum, M. K., and Rossing, W. A. H. (2005). A method for exploring sustainable development options at farm scale: a case study for vegetable farms in South Uruguay. Agric. Syst. 86, 29–51. doi: 10.1016/j.agsy.2004.08.002
FAO (2001). Strategic Environmental Assessment: an Assessment of the Impact of Cassava Production and Processing on the Environment and Biodiversity. Vol 5. Rome, Italy: Food and Agriculture Organization of the United Nations, International Fund for Agricultural Development.
FAO (2005). New_locClim: Local Climate Estimator. Available online at: http://www.fao.org/land-water/land/land-governance/land-resources-planning-toolbox/category/details/en/c/1032167/ (accessed March 11, 2019).
FAO (2019). FAOSTAT. Available online at: http://www.fao.org/faostat/en/#data/CC (accessed January 15, 2020).
Fermont, A. M., van Asten, P. J. A., and Giller, K. E. (2008). Increasing land pressure in East Africa : the changing role of cassava and consequences for sustainability of farming systems. Agric. Ecosyst. Environ. 128, 239–250. doi: 10.1016/j.agee.2008.06.009
Frison, E., and Sharrock, S. (1999). “The economic, social and nutritional importance of banana in the world,” in Bananas and Food Security, eds. C. Picq, E. Fouré, and E. A. Frison (Montpellier, France: International Network for the Improvement of Banana and Plantain), 21–35.
Giller, K. E., and Wilson, K. J. (1991). Nitrogen Fixation in Tropical Cropping Systems. Wallingford: CAB International.
Gliessman, S. R. (2000). Agroecology: Ecological Processes in Sustainable Agriculture, ed. E. Engels Boca Raton, FL: Lewis Publishers.
Gold, C. S., Kiggundu, A., Abera, A. M. K., and Karamura, D. (2002). Diversity, distribution and farmer preference of musa cultivars in Uganda. Exp. Agric. 38, 39–50. doi: 10.1017/S0014479702000145
Groot, J. C. J., Oomen, G. J. M., and Rossing, W. A. H. (2012). Multi-objective optimization and design of farming systems. Agric. Syst. 110, 63–77. doi: 10.1016/j.agsy.2012.03.012
Groot, J. C. J., and Rossing, W. A. H. (2011). Model-aided learning for adaptive management of natural resources: an evolutionary design perspective. Methods Ecol. Evol. 2, 643–650. doi: 10.1111/j.2041-210X.2011.00114.x
Groot, J. C. J., Rossing, W. A. H., Jellema, A., Stobbelaar, D. J., Renting, H., and Van Ittersum, M. K. (2007). Exploring multi-scale trade-offs between nature conservation, agricultural profits and landscape quality-a methodology to support discussions on land-use perspectives. Agric. Ecosyst. Environ. 120, 58–69. doi: 10.1016/j.agee.2006.03.037
Gutteridge, R. C., and Shelton, H. M. (1994). Forage Tree Legumes in Tropical Agriculture, eds R. C. Gutteridge and H. M. Shelton Wallingford: CAB International.
HarvestPlus (2018). Food Composition Table. Available online at: https://www.harvestplus.org/node/562 (accessed October 1, 2018).
Jassogne, L., Nibasumba, A., Wairegi, L., Baret, P. V., Deraeck, J., Mukasa, D., et al. (2013). “Coffee/banana intercropping as an opportunity for smallholder coffee farmers in Uganda, Rwanda and Burundi,” in Banana Systems in the Humid Highlands of Sub-Saharan Africa, eds G. Blomme, P. van Asten, and B. Vanlauwe (Wallingford; Boston: CAB International), 144–149. doi: 10.1079/9781780642314.0144
Kansiime, M. K., van Asten, P., and Sneyers, K. (2018). Farm diversity and resource use efficiency: targeting agricultural policy interventions in East Africa farming systems. NJAS - Wageningen J. Life Sci. 85, 32–41. doi: 10.1016/j.njas.2017.12.001
Karamura, E., Frison, E., Karamura, D. A., and Sharrock, S. (1998). “Banana production systems in eastern and southern Africa,” in Bananas and Food Security, eds. C. Picq, E. Fouré, and E. A. Frison (Montpellier, France: International Network for the Improvement of Banana and Plantain), 401–412.
Karamura, E. B., Jogo, W., Rietveld, A., Ochola, D., Staver, C., and Tinzaara, W. (2013). Effectiveness of agroecological intensification practices in managing pests in smallholder banana systems in East and Central Africa. Acta Hortic. 986, 119–126. doi: 10.17660/ActaHortic.2013.986.10
Katungi, E., Smale, M., and Tushemereirwe, W. (2006). “Management of banana plantations,” in A Baseline Assessment of Banana Production and Management Practices in Uganda - Uganda Country Report, eds. W. Tushemereirwe, F. Bagamba, E. Katungi, E. Kikulwe, D. Karamura, S. Edmeades, et al. (Kampala, Uganda: Makerere University), 50–60.
Kongkijthavorn, S. (2017). Towards sustainable banana production in Central Uganda : Assessing four alternative banana cropping systems (dissertation). Farming Systems Ecology Group, Wageningen University: Netherlands.
Kuivanen, K. S., Alvarez, S., Michalscheck, M., Adjei-Nsiah, S., Descheemaeker, K., Mellon-Bedi, S., et al. (2016). Characterising the diversity of smallholder farming systems and their constraints and opportunities for innovation: a case study from the Northern Region, Ghana. NJAS - Wageningen J. Life Sci. 78, 153–166. doi: 10.1016/j.njas.2016.04.003
Le Gal, P., Dugué, P., Faure, G., and Novak, S. (2011). How does research address the design of innovative agricultural production systems at the farm level? A review. Agric. Syst. 104, 714–728. doi: 10.1016/j.agsy.2011.07.007
Meya, A. I., Ndakidemi, P. A., Mtei, K. M., Swennen, R., and Merckx, R. (2020). Optimizing soil fertility management strategies to enhance banana production in volcanic soils of the Northern Highlands, Tanzania. Agronomy 10, 289–309. doi: 10.3390/agronomy10020289
Mpiira, S., Staver, C., Kagezi, G. H., Wesiga, J., Nakyeyune, C., Ssebulime, G., et al. (2013). “The use of trees and shrubs to improve banana productivity and production in Central Uganda: An analysis of the current situation,” in Banana systems in the humid highlands of Sub-Saharan Africa, eds. G. Blomme, P. van Asten, and B. Vanlauwe (Wallingford; Boston, USA: CAB International), 150–157. doi: 10.1079/9781780642314.0150
Murtagh, F., and Legendre, P. (2014). Ward's hierarchical clustering method: clustering criterion and agglomerative algorithm. J. Classif. 31, 274–295. doi: 10.1007/s00357-014-9161-z
Němečková, A., Christelová, P., CíŽková, J., Nyine, M., Van den houwe, I., Svačina, R., et al. (2018). Molecular and cytogenetic study of East African highland banana. Front. Plant Sci. 9:1371. doi: 10.3389/fpls.2018.01371
Ocimati, W., Karamura, D., Rutikanga, A., Sivirihauma, C., Ndungo, V., Ntamwira, J., et al. (2013). “Agronomic practices for musa across different agro-ecological zones in burundi, eastern democratic republic of congo and rwanda,” in Banana Systems in the Humid Highlands of Sub-Saharan Africa, eds G. Blomme, P. van Asten, and B. Vanlauwe (Montpellier, France: CAB International), 175–190. doi: 10.1079/9781780642314.0175
Otte, M. J., and Chilonda, P. (2002). Cattle and Small Ruminant Production Systems in Sub-Saharan Africa - A Systemic Review. Rome, Italy: Food and Agriculture Organization of the United Nations.
Schroth, G. (1995). Tree root characteristics as criteria for species selection and systems design in agroforestry. Agrofor. Syst. 30, 125–143. doi: 10.1007/BF00708917
Sileshi, G., Akinnifesi, F. K., Ajayi, O. C., Chakeredza, S., Kaonga, M., and Matakala, P. W. (2007). Contributions of agroforestry to ecosystem services in the miombo eco-region of eastern and southern Africa. African J. Environ. Sci. Technol. 1, 68–80. Available online at: https://www.ajol.info/index.php/ajest/article/view/135037
Ssebulime, G., Kagezi, G., Nyombi, K., Mpiira, S., Kucel, P., Byabagambi, S., et al. (2017). Farmers' knowledge of the banana (Musa spp.) agroforestry systems in Kiboga district, central Uganda. Afr. J. Food Agric. Nutr. Dev. 17, 12556–12572. doi: 10.18697/ajfand.80.16395
Ssebulime, G., Nyombi, K., Kagezi, G., Mpiira, S., Byabagambi, S., Tushemereirwe, W., et al. (2019). Assessing the potential of trees to sustain soil fertility in the banana agroforestry system in Uganda. Agroecol. Sustain. Food Syst. 43, 662–677. doi: 10.1080/21683565.2019.1566190
Stoorvogel, J. J., and Smaling, E. M. A. (1990). Assessment of Soil Nutrient Depletion in Sub-Saharan Africa: 1983–2000. Wageningen, Netherlands: The Winand Staring Centre.
Tinzaara, W., Kiggundu, A., Gold, C. S., Tushemereirwe, W. K., and Karamura, E. B. (2009). Management options for highland banana pests and diseases in East and Central Africa. Outlooks Pest Manag. 20, 204–209. doi: 10.1564/20oct04
Tinzaara, W., Stoian, D., Ocimati, W., Kikulwe, E., Otieno, G., and Blomme, G. (2018). “Challenges and opportunities for smallholders in banana value chains,” in Achieving Sustainable Cultivation of Bananas, eds. G. H. J. Kema and A. Drenth (Burleigh Dodds Science), 86–111. doi: 10.19103/AS.2017.0020.10
Tittonell, P., Muriuki, A., Shepherd, K. D., Mugendi, D., Kaizzi, K. C., Okeyo, J., et al. (2010). The diversity of rural livelihoods and their influence on soil fertility in agricultural systems of East Africa – a typology of smallholder farms. Agric. Syst. 103, 83–97. doi: 10.1016/j.agsy.2009.10.001
Tittonell, P., van Wijk, M. T., Rufino, M. C., Vrugt, J. A., and Giller, K. E. (2007a). Analysing trade-offs in resource and labour allocation by smallholder farmers using inverse modelling techniques: a case-study from Kakamega district, western Kenya. Agric. Syst. 95, 76–95. doi: 10.1016/j.agsy.2007.04.002
Tittonell, P., Zingore, S., van Wijk, M. T., Corbeels, M., and Giller, K. E. (2007b). Nutrient use efficiencies and crop responses to N, P and manure applications in zimbabwean soils: exploring management strategies across soil fertility gradients. F. Crop. Res. 100, 348–368. doi: 10.1016/j.fcr.2006.09.003
USDA (2018). USDA Food Composition Table. Available online at: https://ndb.nal.usda.gov/ndb/search/list (accessed October 1, 2018).
van Asten, P. J. A., Fermont, A. M., and Taulya, G. (2011). Drought is a major yield loss factor for rainfed East African highland banana. Agric. Water Manag. 98, 541–552. doi: 10.1016/j.agwat.2010.10.005
Van Noordwijk, M., and Purnomosidhi, P. (1995). Root architecture in relation to tree-soil-crop interactions and shoot pruning in agroforestry. Agrofor. Syst. 30, 161–173. doi: 10.1007/BF00708919
Vanlauwe, B., van Asten, P., and Blomme, G., (Eds.). (2013). Agro-ecological intensification of agricultural systems in the african highlands. New York, NY: Routledge, 314. doi: 10.4324/9780203114742
Wairegi, L. W. I., and van Asten, P. J. A. (2010). The agronomic and economic benefits of fertilizer and mulch use in highland banana systems in Uganda. Agric. Syst. 103, 543–550. doi: 10.1016/j.agsy.2010.06.002
Wairegi, L. W. I., van Asten, P. J. A., Tenywa, M. M., and Bekunda, M. A. (2010). Abiotic constraints override biotic constraints in East African highland banana systems. F. Crop. Res. 117, 146–153. doi: 10.1016/j.fcr.2010.02.010
Wajja-Musukwe, T. N., Wilson, J., Sprent, J. I., Chin, K. O., Deans, J. D., and Okorio, J. (2008). Tree growth and management in ugandan agroforestry systems: effects of root pruning on tree growth and crop yield. Tree Physiol. 28, 233–242. doi: 10.1093/treephys/28.2.233
Wezel, A., Soboksa, G., McClelland, S., Delespesse, F., and Boissau, A. (2015). The blurred boundaries of ecological, sustainable, and agroecological intensification: a review. Agron. Sustain. Dev. 35, 1283–1295. doi: 10.1007/s13593-015-0333-y
World Agroforestry Centre (2018a). RELMA-ICRAF Useful Trees. Available online at: http://www.worldagroforestry.org/usefultrees/index.php# (accessed October 1, 2018).
World Agroforestry Centre (2018b). Species Profile. Available online at: http://www.worldagroforestry.org/treedb2/speciesprofile.php?Spid=17981 (accessed October 1, 2018).
World Agroforestry Centre (2018c). Tree Functional Attributes and Ecological Database. Available online at: http://worldagroforestry.org/output/tree-functional-and-ecological-databases (accessed October 1, 2018).
Keywords: agroecology, farm typology, FarmDESIGN, farm performance, farm optimization, nutritional yield, profitability, sustainability
Citation: Gambart C, Swennen R, Blomme G, Groot JCJ, Remans R and Ocimati W (2020) Impact and Opportunities of Agroecological Intensification Strategies on Farm Performance: A Case Study of Banana-Based Systems in Central and South-Western Uganda. Front. Sustain. Food Syst. 4:87. doi: 10.3389/fsufs.2020.00087
Received: 21 March 2020; Accepted: 18 May 2020;
Published: 23 June 2020.
Edited by:
Paulo Mazzafera, Campinas State University, BrazilReviewed by:
Nikolaos Vasileios Paranychianakis, Technical University of Crete, GreeceRakesh K. Upadhyay, United States Department of Agriculture (USDA), United States
Copyright © 2020 Gambart, Swennen, Blomme, Groot, Remans and Ocimati. This is an open-access article distributed under the terms of the Creative Commons Attribution License (CC BY). The use, distribution or reproduction in other forums is permitted, provided the original author(s) and the copyright owner(s) are credited and that the original publication in this journal is cited, in accordance with accepted academic practice. No use, distribution or reproduction is permitted which does not comply with these terms.
*Correspondence: Walter Ocimati, dy5vY2ltYXRpJiN4MDAwNDA7Y2dpYXIub3Jn