- 1CIRAD, UMR TETIS, Montpellier, France
- 2TETIS, Univ Montpellier, AgroParisTech, CIRAD, CNRS, INRAE, Montpellier, France
- 3CIRAD, UPR AIDA, Dakar, Senegal
- 4AIDA, Univ Montpellier, CIRAD, Montpellier, France
- 5Centre de Suivi Ecologique, Dakar, Senegal
- 6Institut d'Economie Rurale BP 262, Bamako, Mali
- 7IRD, UMR ESPACE-DEV, Montpellier, France
- 8GEOMATICA, Saint-Louis, Senegal
- 9Laboratoire LEIDI, Univ Gaston Berger, Saint-Louis, Senegal
In the last decade, governments, international institutions, donors, the private sector, etc. have shown a renewed interest in agricultural issues in Sub-Saharan Africa (SSA). This interest came with a strong need for information in countries where the lack of reliable and timely basic information can be a problem. Thanks to its capacity to observe the Earth at local, regional, and global scales and from various vantage points, satellite remote sensing is a powerful tool to streamline the monitoring and improvement of the existing systems, and thus to support decision-making. However, the path from satellite images to public policy decisions is not straightforward, and today, only few operational information services are available in SSA countries (e.g., early warning systems for food security and desert locust plagues prevention, rangeland production forecasting). This paper aims to analyze the gap between the technical aspects of the remote sensing sciences and the pragmatic need for relevant data to address agricultural policies in Africa and produce operational recommendations. To achieve this goal, the authors (1) determine the information, and in particular the geoinformation, needed to develop, implement and evaluate agricultural public policies (2) illustrate the role of remote sensing as a public policy tool in SSA through an overview of the current off-the-shelf products and services derived from Earth Observation systems, and (3) propose an analysis of the existing gap between the remote sensing research community and the policy makers. Based on this review, the authors conclude that to benefit from this technological advancement and bridge the gap between technical analysts and policy makers, some key points are fundamental: capacity building, political will and institutional commitment, public-private partnership, and proofs of concept.
Introduction
Africa remains the world's only region that is a net importer of food products, but all studies show that the continent has sufficient resources in manpower, land, and water to be a breadbasket. While agriculture is central to Africa's economies, its performance in this sector has traditionally lagged compared to that of other regions. Weak productivity has been caused by a wide range of factors, including poverty (e.g., limited access to inputs and machines), population growth (e.g., a decrease in the fallow periods and cropland expansion to less fertile land), climate changes (i.e., changes in the weather pattern and an increase in extreme climatic events such as floods or heat waves), technological issues and a weakness of the institutional framework, the security environment or the health care situation. The structural part of the food insecurity situation in Sub-Saharan Africa (SSA) is still increasing (FAO, 2017).
In the last decade, governments, the OAU (Organization of African Unity), United Nations institutions, donors, the private sector, etc. have shown a renewed interest in agricultural issues. This concern is reflected in international initiatives, such as the AUDA (African Union Development Agency), private investments, and the conception of new agricultural policies to reform land and resource access, to build infrastructure and to develop services such as research, extension, credit and insurance. More than half of the seventeen Sustainable Development Goals for the 2015–2030 period are directly or indirectly connected with farming.
Consequently, there is a strong need for information about agricultural issues in SSA, which often lacks basic data due to the poor condition of the African national systems of statistics. These systems often suffer not only from weak financial and material resources and technical skill gaps but also from the complexity of the cropping systems and, possibly, from the mistrust of the farmers. The lack of reliable and timely basic information is a problem, and any approach that can streamline the monitoring and improvement of the existing systems is an important step.
One promising focus of research has centered on the potential of satellite technologies and the new sources of information that they offer for decision-making. For the last 30 years, a rich and detailed scientific literature on using remote sensing for agricultural practices and the environment has emerged, demonstrating a range of capabilities from land cover/use mapping (e.g., Gómez et al., 2016; Bégué et al., 2018) to vegetation growth monitoring (e.g., Atzberger, 2013). There are still few examples of these scientific and technical advances in the realm of the operational uses of Earth Observation (EO) data for agricultural public policy at the national or regional scale. The available examples include the control of subsidies (e.g., in Europe; Schmedtmann and Campagnolo, 2015), yield forecasting (e.g., in Europe and North African countries; López-Lozano and Baruth, 2019), agricultural compliance (e.g., in Brazil with the Green Ethanol protocol; Aguiar et al., 2011) or the soy moratorium (Rudorff et al., 2011), or early warning systems for food security [see Fritz et al. (2019) for a review].
The scientific studies linking land changes to agricultural public policies are also weak compared to those dedicated to environmental policies (e.g., Lein, 2012). Based on classifications of high resolution images (e.g., Landsat and SPOT), studies have shown the effect (or the absence of an effect) of agricultural policies on land cover and land use in Syria (Ibrahim et al., 2014), the Peruvian Amazon (Chavez et al., 2014), Cambodia (Kong et al., 2019), or in the Yucatan region in Mexico (Turner, 2010). Using MODIS NDVI time series, Lein (2012) showed how an agricultural tax free ordinance issued in 2006 changed the adoption of multiple cropping practices in China, while in Brazil, Arvor et al. (2011) developed satellite-derived indices to describe the agricultural dynamics in Amazonia between 2000 and 2007 in relation to the existing public policies.
Despite these interesting examples, there is a consensus on the global underutilization of remote sensing data for public policy, resulting in a real asymmetry in information, with private traders having access to better and more information than governments1 The development of large international initiatives in the domain of agriculture attests to the fact that there is an urgent need to further mobilize remote sensing data for public decision making. These initiatives concern the production of off-the-shelf global land products (e.g., GEOGLAM2 for the world (Becker-Reshef et al., 2019); Copernicus3 for Europe; and GMES & Africa4 for Africa) and the development of demonstration cases in pilot studies (e.g., customized agricultural information services to support the operations of multilateral development banks and international financing institutions with EO4SD5 (ESA, 2017); user-tailored geospatial services to inform resilient development in various regions of the world with SERVIR6 projects (USAID et al., 2019); demonstrations of the effectiveness of delivering information about biomass and surface water availability to pastoralists' mobile phones with the STAMP7 and MODHEM8 projects SNV, 2019). However, currently, these initiatives cannot totally fulfill the government needs for better agricultural and land management and planning.
Using the specific context of West Africa as an entry point, this paper aims to analyze the gap between the technical aspects of the remote sensing sciences and the pragmatic need for relevant data to address agricultural policies in Sub-Saharan Africa, and to produce operational recommendations. To achieve this goal, the authors (1) determine the information, and in particular the geoinformation, needs to develop, implement, and evaluate agricultural public policies, (2) clarify the role of remote sensing as a public policy tool through a brief review of the existing remote sensing products and services in the Sub-Saharan African context, and (3) propose an analysis of the existing gap between the remote sensing research community and the policy makers.
Agricultural Public Policy Information
Challenges for African Agriculture
To achieve the Sustainable Development Goals and produce more food using limited resources via innovative practices, while preserving the food quality and the environment as much as possible, the development of both productive and sustainable farming became a priority in Africa (Shimeles et al., 2018). To cope with this situation, African agriculture needs to produce more food to meet the demand tied to population growth (the sub-Saharan population is projected to double by 2050; UN, 2019a) combined with rapid urbanization (from 42% urban population today to 59% by mid-century; UN, 2019b). In addition, the African agricultural system will have to increase the competitiveness of its agricultural exports to contribute more efficiently to the global trade system and thus to the continental economic growth. The final challenges that African agriculture will have to face are to retain the economically active population in rural areas (Losch and Freguin-Gresh, 2011; Conceição et al., 2016), particularly by creating employment for young people and containing regional and international migration and by reducing rural poverty by boosting wealth creation, especially as it relates to the incomes of small farmers. These challenging goals must be addressed in a sustainable way that secures the productive capacity of the natural resources for present and future needs (Goyal and Nash, 2017), no matter how complex and diverse the African agriculture types are (Collier and Dercon, 2014).
After the shrinking of international aid during the'80 and '90s and the 2008 food crisis, a consensus now exists: a major investment in agriculture pays off both in terms of food security and national economic development. African nations must devise coherent public resources, strategies and policies such as infrastructures, energy, land regulations, agronomic research, the availability of credit, mechanisms to fight price volatility and manage risks, the reinforcement of agricultural organizations, and training (Jayne et al., 2014). Renewed interest in agriculture and agriculture policies has been strong (Pingali, 2010). Initiatives to design strategies for the development of African agriculture have been multiplied, in the regional and continental level. The most significant initiative is the Comprehensive African Agriculture Development Program (CAADP) which aims to increase public investment in agriculture by a minimum of 10% of national budgets, and to raise agricultural productivity by at least 6% (Zimmermann et al., 2009; AUDA, 2010; KolalavalIi et al., 2010). Many countries have applied these general orientations in national programs by developing investment plans, agricultural public policies, and partnerships.
Agricultural Public Policy: Information Needs
An agricultural policy is a set of laws, regulatory measures, structural arrangements, and financial and human means implemented by a government to enable agricultural production (Ribier, 2008). Governments usually implement agricultural policy with the goal of increasing food and raw material production (Pisani and Chatellier, 2010). However, in recent years, new agricultural goals have been set: raising the standard of living through rural population employment, food and nutrition security, poverty reduction, climate resilience and water, soil, and biodiversity conservation (Felix, 2006).
Policy making process is a matter of ongoing debate. Nowadays, the debate focuses on the best way to modernize production systems, green revolution (Woodhouse, 2010; Horlings and Marsden, 2011) vs. agroecology (Öborn et al., 2017), or between both paths? With which actors, large-scale commercial agro-industries specialized in crops and animal production (Woodhouse, 2010) vs. family farming (HLPE, 2013)? So designing public agricultural policies is a difficult task, mainly to reconcile various objectives (Howlett, 2011). Surely, there is neither a simple solution to Africa's underproduction of foodstuffs, nor a single answer, that will bring food security to everyone. Agricultural policies must be adapted to diversity, and information is needed to enable this adaptation.
According to knowledge-based decision-making (Hale et al., 2002), accurate, timely, and comprehensive information is required for guiding the conception, implementation, monitoring, and evaluation of these agricultural policies (Wilson and Gapsiso, 2018). Examples of information needs for agriculture-related public policies have been gathered and listed in Table 1.
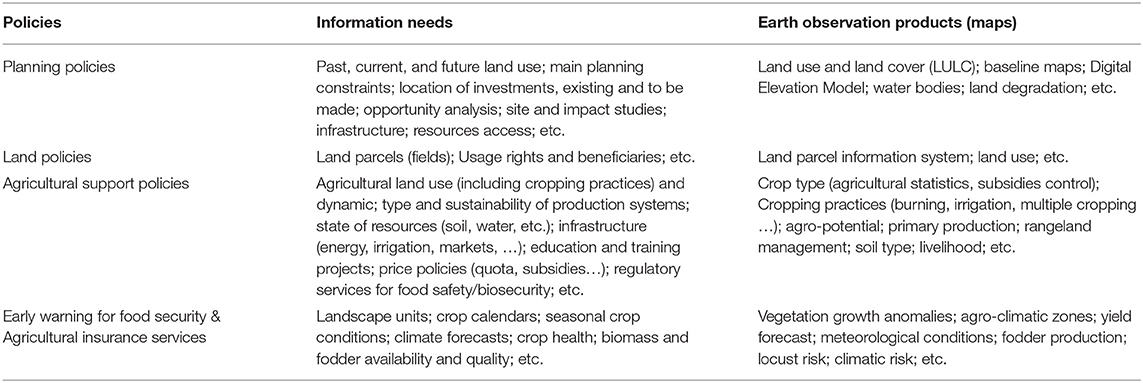
Table 1. What information and what EO products, for what policy? [adapted from Bégué et al. (2016)].
Until 90s, data had been usually provided by the agricultural censuses (every 10 years for some countries) and surveys. In the two last decades, the situation of the African national systems of agricultural statistics has been strongly criticized (Leif et al., 2007; CILSS, 2009). In most cases, the systems created in the sixties became inoperative, generally due to financing difficulties, and their contribution to the national systems of statistics and the global system organized by the FAO (FAOSTAT, CountrySTAT) is now marginal. In 2010, the FAO adopted a strategy to improve rural and agricultural statistics (World Bank et al., 2010). The goal is now to develop more efficient tools, which are integrated into national statistics systems (GSARS, 2017). Digital technologies, such as Earth Observation, mobile devices, and web services, offer new possibilities in this regard.
Geoinformation to Support Agricultural Policies
Thanks to its capacity to observe the Earth at local, regional, and global scales and with different thematic focuses, remote sensing is an essential technology for providing geoinformation in support to land and agricultural policies. The use of remote sensing for mapping land characteristics has been studied for ~40 years, resulting in the use of a large number of land surface and climatic variables and products for application demonstrations. The main EO variables and products identified in Table 1 to support agricultural public policies can be categorized as follows:
- The baseline maps, to ensure spatial consistency within heterogeneous datasets;
- The land use and land cover maps produced at high spatial resolutions and updated on a regular basis, to monitor the land changes (evaluation, prospective), support the statistical sampling protocols (agricultural census), or manage the agricultural risks and opportunities;
- The biogeophysical maps of crop (phenology, biomass, growth anomalies, etc.) and environmental conditions (soil moisture, rainfall, topography, etc.) provided throughout the year for near real-time monitoring, early warning and decision-making.
Even though the combination of these three categories of products provides relevant elements to support and evaluate public policies through baseline setting, targeting, monitoring and evaluation, it is not sufficient. Most of the time, EO data must be completed by inquiries, ancillary data, and local expertise in order to derive products in accordance with the real needs of each policy.
Remote Sensing Data and Products for Agricultural Applications
In this section, we present a non-exhaustive overview of the existing products and agriculture-related services based on Earth-Observation data in Africa, in the challenging context of smallholder agriculture in global South.
Overview of the EO Products Available for Agricultural Monitoring
Baseline Maps
Metric and sub-metric resolution images are used to produce baseline maps that provide geographically localized information and offer a shared vision for a territory. To date, there are no baseline maps at the global scale, but projects to produce these maps at national scales are multiplying throughout the world.
In West Africa, Benin9 has recently joined the circle of those countries (Senegal, Mali and Burkina Faso10 with an up-to-date and homogeneous national topographic database and the tools to exploit them. The maps were produced at a scale between 1:50 000 and 1:200 000, ranging from mosaics of satellite high resolution orthoimages (e.g., RapidEye and SPOT6 images) to aerial photographs. These projects, funded by the European Union, were implemented with the national geographic institutes of the countries. More seldomly, country baseline maps are produced annually, such as in South Africa where the South African National Space Agency (SANSA) has provided a country-wide true color composite image11 made of a mosaic of SPOT images each year, since 2006. The mosaic is packaged and distributed to government departments, universities, and research institutes.
Land Cover and Land Use Products
At the global and regional scales (i.e., the continental to subcontinental scale), no less than twenty land cover/land use maps are referred in the literature, and advancements are continuously made owing to new services, such as free access to high spatial and temporal resolution data and increased computational capabilities (Tsendbazar et al., 2018).
Although these land cover maps have been largely used for global analysis, several studies highlighted large discrepancies between them, both in area estimation and the location of the main land cover classes, and concluded that there is generally no consensus concerning the cropland classes in Africa (Fritz et al., 2010; Lambert et al., 2016; Tsendbazar et al., 2018). The reasons for the discrepancies between products are mainly related to the specificities of smallholder agriculture in the African context (see section The African agriculture challenges for remote sensing). Waldner et al. (2015) evaluated the available cropland datasets at the global scale and concluded that Africa (mainly West African and South African countries) is one of the highest priority areas for the improvement of cropland mapping.
At the national scale, land cover maps have been produced in several African countries, mainly in the context of North-South partnership projects or national land cover programs. For instance, in the framework of the Africover and the Global Land Cover Network projects overseen by the Food and Agriculture Organization (FAO), more than fifteen countries, mainly in East Africa, have been mapped through visual interpretation of Landsat images (Latham et al., 2014). Recently, the Sen2-Agri project has developed a platform to produce monthly dynamic cropland masks and cultivated crop type maps at a 10 m resolution twice during the agricultural season based on Sentinel-2 and Landsat-8 data (Defourny et al., 2019). Finally, at the subnational scale, many isolated land cover projects were conducted based on the need for research projects or thematic expertise (e.g., the AfricaRISING program in southern Mali12 However, these maps are generally not accurate enough for the operational requirements of a large-scale project, such as land use plans and the computation of agricultural statistics. Land use maps at a relatively high spatial resolution can be produced on request for large areas, often as a part of a project, such as the production of the Burkina Faso land cover database (BDOT13 at a 1/100 000 scale developed by the Geographic Institute of Burkina Faso (IGB).
Biogeophysical Products
For agricultural applications, information about the crop growing conditions is essential to detect and manage potential vegetation stress (or drought). Hence, international programs have developed off-the-shelf global biogeophysical products based on satellite imagery. These products are mainly provided by public scientific organizations (NASA's MODIS Land Team, the JRC, the EUMETSAT, the ESA, etc.) and by the European COPERNICUS initiative. The COPERNICUS Global Land Surface Services offer biogeophysical products at a global scale that are free, validated, documented, and near real-time, and their provision is sustainable through the Copernicus14 web portal or EUMETCast channel. The global biogeophysical products are categorized based on the land cover, vegetation status (vegetation indices, productivity, leaf area index, fraction of absorbed photosynthetically active radiation, and fires), water cycle (rainfall, evapotranspiration, soil moisture, and water bodies), energy budget (albedo, reflectance, and radiation), and topography indices from digital elevation models. The spatial resolutions of these products range from 20 m to 200 km, while the temporal resolutions range from 15 min to annual, depending on the biogeophysical variables.
The African Agriculture Challenges for Remote Sensing
Despite the widely acknowledge potential of remote sensing for agricultural monitoring, and the existing EO-derived products, the complexity and variety of African agriculture types (Collier and Dercon, 2014), the complex interactions between weather and geography where diverse rainfall patterns may exist even in nearby areas (Becker-Reshef et al., 2020), and the lack of ancillary data challenge the application of remote sensing approaches that are primarily designed for the global North.
High Spatial and Temporal Heterogeneities of the Cropping Systems
The soil quality, climate, population density, infrastructure, markets, financial instruments, governance, etc. shape the different forms of agriculture that coexist among a large range of models from domestic food crops to cash crops and from household agriculture to agro-industry (Mellor, 2014). However, except Southern Africa, Maghreb, Egypt and few coastal West African countries, small-scale farming is the prevalent form of agriculture in Africa (Dixon et al., 2001; Samberg et al., 2016). Small-scale agriculture is characterized by small to very small plot sizes with high inter- and intra-plot variability, making it difficult to produce agricultural land use maps (Arias and Inglada, 2015; Fritz et al., 2015). The spatial heterogeneity of agricultural lands is often enhanced by the presence of trees within plots (e.g., West African parklands) and/or intercropping (Félix et al., 2018). The crop-fallow rotation is also widely used in African small-scale agriculture as mean to restore soil fertility, but until recently was poorly detected by remote sensing (Tong et al., 2020).
Most of decision support products for agriculture rely on the processing of satellite image time series, as they allow to account for land surface phenology which is the cornerstone for the discrimination of the different land use type (e.g., crop vs. non-crop, crop groups). In Africa, land surface phenology can be difficult to account for, as: (i) in humid tropical zones, changes in land surface phenology is not related to climate, but to cropping practices; (ii) in dry tropical zones, where the rainy season is short, crops and natural vegetation have a quasi-synchronized vegetative development; (iii) mixed cropping systems may have intercropping with a very short cycle, “blurring” the signal of the main crop.
Unfavorable Weather Conditions
The high cloud cover during rainy season is a major constraint to the rainfed crop monitoring that dominate in African cropping systems. Recent studies (Whitcraft et al., 2015a,b) highlighted that a revisit time period of 1–3 days in August is required to obtain 8-day images composite with 70% agricultural land in clear sky conditions in sub-Saharan Africa. This frequency increases to 3–6 days in July and September. In addition to the low spatial resolution satellites (daily time frequency), the ESA's Sentinel-2 constellation with a 5-day revisit period offers a revisit frequency closed to that required to cover the entire growing season.
A Lack of Ancillary Data, With a Limited Accessibility
Processing and analysis of satellite images required ancillary data. For instance, atmospheric information from ground station are needed to calibrate correction model during the preprocessing phase of satellite images. Besides, information related to topography, soil type, climate, or agricultural statistics are very useful for image processing and evaluation of resulting products. However, since the 1980's weather systems and agricultural statistics systems of several African countries underwent an ongoing decrease of quality, due to a lack of resources and institutional coordination (World Bank et al., 2010). This results in the paucity of ground databases, which is critical for developing and assessing the accuracy of remote sensing based indicators and methods (Becker-Reshef et al., 2020).
Existing high-resolution satellite archives are heterogeneous. Landsat repositories are not completed. SPOT repositories are highly heterogeneous depending on region and time periods considered. Hence, until the launch of the Sentinel-2 constellations (2015 and 2017), mainly images acquired during the dry season are available.
Examples of Current Earth Observation-Based Services in Africa
Despite the challenges posed by the African agricultural systems, services focusing on agricultural policy information have been developed based on EO-derived products. These information services produce regular, standardized information that is easy to access and use. They comply with precise specifications, according to the needs of the end users. Among the few services implemented in Africa, three thematic domains are presented below as examples.
Some of the off-the-shelf products are routinely used in the early warning systems for food security [see Fritz et al. (2019) for a review]. For West Africa, a complete system to monitor rainfall, crop water requirement satisfaction and prospective yields, and the progress of the vegetative development and its seasonal and interannual variations has been developed by the AGRHYMET Regional Center from ground and satellite data (Traore et al., 2014). These services rely, in particular, on the former SPOT-VEGETATION and PROBA-V NDVI time series to detect vegetation growth anomalies that can potentially lead to pastoral or crop production shortages and satellite-derived rainfall data. However, despite the very large improvement in data availability, processing methods, and the stream of dissemination, these systems still suffer from insufficient spatial input data (especially accurate cropland maps, crop calendars, and meteorological data) and methods for better yield predictions (Fritz et al., 2019). However, things are moving forward, and in East Africa, thanks to work with national ministries in Tanzania, Uganda and Kenya, the GEOGLAM Crop Monitor for Early Warning (CM4EW) has recently been adapted for national contexts (Becker-Reshef et al., 2020).
NDVI and precipitation products are also largely used for crop and pasture yield forecasting and estimation. Many African countries have developed their own forecasting systems based on different datasets and models and produce monthly or seasonal bulletins. In South Africa, the official crop forecast for summer and winter crops is released monthly by the Crop Estimates Committee. Remote sensing data are used for crop condition monitoring on a broad scale, but despite some progress, crop acreage assessments using remote sensing data has never reached an operational stage in South Africa, mainly due to resource constraints (Bernardi et al., 2016). In Senegal, the annual biomass is estimated on an operational basis to monitor fodder availability in the national pastoral rangelands. This method is based on an empirical relationship between the satellite-derived seasonal integrated NDVI and in situ measured biomass at the end of the growing season (Diouf et al., 2015). In Mali and Burkina Faso, the Garbal information service, developed by the Netherlands Space Office (NSO), offers information on biomass availability and quality and surface water availability, which is developed from satellite images, herd concentration data and market prices for livestock and staple grains, to pastoralists over simple mobile phones (with a modest fee to the subscribers per request). This first experience is real progress, even if some technical (e.g., better estimates of fodder biomass in the dry season are still needed) or economic (e.g., the generated income is insufficient to cover the operating costs, which could be reduced through upscaling) adjustments should be made to ensure complete success (SNV, 2019).
The FAO Desert Locust Information Service (DLIS) is the key monitoring and early warning tool in preventing desert locust plagues from devastating farmers' fields in Africa and Asia. The DLIS operates an early warning system that monitors weather (rainfall and temperature derived from models, satellite images or ground stations), ecological conditions (vegetation detection and vegetation period length and density), and locust infestations in the potentially affected area on a daily basis (FAO, 2015). The DLIS produces monthly situation summaries and forecasts for each country and sends warnings and alerts about potential invasions or other significant developments to affected countries and donors.
Apart from a few regional successes, the general scarcity of information services over the African continent (as in most regions of the world) is explained by the relatively high difficulty in designing decision support products compared to that of using off-the-shelf products, and by the difficulty in implementing research products over large areas as they often include complementary data and methods adapted to the local conditions (e.g., the environment, climate and agricultural practices).
The Gap Between Technical Analyst and Policy-Maker
A Longstanding Observation and a Situation That Is Not Changing
In 2002, Harris analyzed the gap between Earth observation and science policy in the UK, and his conclusions are still globally valid nearly 20 years on. There is still a discrepancy between the success of remote sensing in providing inputs to environmental science models, operational meteorology, and the control of agricultural subsidies, on the one hand, and the lack of consistent and operational civilian end-user systems, on the other, despite the numerous number of international projects and initiatives in this domain. According to Harris (2002), the major limitation has been the lack of a mature marketplace.
In a recent policy brief, Tonneau et al. (2019) come to the same conclusion. The gap between EO and science policy is reinforced in African countries where the technology resources strategy, largely driven by institutions of the Global North, aims to share data and products that only partially meet African demand. The data processing methods are often inadequate for the African agricultural systems, which are more diversified and much less documented than agricultural systems in industrialized countries. The environmental conditions, the diversity of agricultural systems, the scarcity of free images at very high spatial resolutions, the paucity of ground databases, and the challenges of partnerships are just some of the transfer and adaptation constraints.
There are also other reasons that compromise the quality of the services available. Many of these challenges have been reported during the UN Information for Meeting Africa's Agricultural Transformation and Food Security Goals conference (IMAAFS, 2014). Remote sensing projects for national and regional public institutions have been supported by international donors, in only a few countries. These projects have equipped centers, trained managers and technicians and have launched applications for agricultural development, but their limited duration has not always ensured the maintenance of the equipment, the preservation of human resources and the upgrading or transfer of skills. In some extreme cases, the work is carried out by experts from the North with no interaction with and thus no transfer to the local partners. These projects are more driven by technological aspects than by developmental needs.
Furthermore, geospatial information in Africa is hampered by the dispersal of activities, mainly in the form of one-off projects, which are often in competition for funding uncoordinated planning, design, and implementation of development projects due to donor preferences. Closed focus areas or sectors, and coordination gaps between the national and regional levels, often result in duplication of efforts and resources and avoidable cost increases (UNECA, 2017).
Finally, local capacity is insufficient. The geospatial domain is very dynamic, and the data offer and technologies change very quickly. Organizations and people are not always able to keep up with them, because of cost constraints in purchasing or updating equipment, or because of insufficient networking and training. Technicians and scientists who were trained abroad on novel methods and high-tech equipment are sometimes assigned to unrelated tasks and cannot use or transfer the knowledge gained (UNECA, 2017).
Saah et al. (2019) summarize these observations: However, technical, capacity, and institutional challenges inhibit the creation of consistent and relevant land cover maps for use in developing regions. Many developing regions lack coordinated capacity, infrastructure, and technologies to produce a robust land cover monitoring system that meets land management needs. Local capacity may be replaced by external consultants or methods which lack long-term sustainability.
Establishing an Environment Favorable to Operational Sustainability
The challenge here is to develop user services that meet the priority needs of stakeholders of the agricultural sector. Most authors insist on the minimum conditions necessary to maintain an environment favorable to the development of these services. It is an ecosystem defined as a dynamically stable network of interconnected firms and institutions within bounded geographical space (Auerswald and Lokesh, 2017). This ecosystem is made up of three main elements: (1) access to images, data and tools; (2) training and capacity building; (3) national/regional strategies.
All satellite images (recent and historical), adapted processing procedures and computing capacities required must be easily accessible. The satellite images and derived products, which are often financed by public funds, need to be pooled in order to capitalize on existing resources and to foster innovation. Another need is to update and make available “basic” geographic data such as topographic maps, land use maps and cadastral maps. Some work is often necessary to improve these maps, which are “often out of date and in analog form. Cadastral maps or databases are mostly unavailable; coherent and standardized geographic names are not available yet; and in most cases geodetic controls are not yet unified and adjusted and are not in sufficient density for information management in Africa” (UNECA, 2017). This raises the question of whether or not these services should incur a fee: do they constitute a public service or should users participate financially? In any case, the pooling of resources helps to reduce costs. Pooling of resources is also recommended for georeferenced updated field data, that are indispensable for developing sustainable services based on remote sensing. Simple and free mobile phone data collection systems (e.g., the SMS system developed by Act Against Hunger for pastoral monitoring15, or crowd sourcing (e.g., Geowiki16, have enabled rapid progress in field data collection, but an Open Data policy should facilitate the cross-sector sharing of the publicly funded data.
Building a critical mass of technicians, professionals, and researchers will enable African countries to control and adapt technologies, and to propose services in line with their socioeconomic and geographical environments. This implies first investing in initial and vocational training and, second, increasing the number and quality of courses. This capacity building will also benefit consultancies and non-governmental organizations, as well as national public institutes.
Coordination by public institutions or regulatory bodies imparts coherence to this ecosystem. The public authorities need to ensure upfront investment to this end. National and/or regional geospatial strategies are required to encourage the pooling of data and of human and material investments, and to thereby avoid redundant investments. As in the case of Senegal with its Plan National Géomatique (PNG), these strategies can coordinate action, define a regulatory framework for the production and use of geographical data and information and, finally, ensure the technical and economic sustainability of services.
Interaction With the End-Users
Without denying the importance of a favorable environment for the development of operational and sustainable services, the lack of interaction with the end-users is the main difficulty. The co-development of information services within the framework of community of practices is required. To this end, a set of activities involving research (to remove a certain number of methodological bottlenecks), support for intermediate operators, and support for end-users (institutions responsible for the development and implementation of land management policies, support structures of the agricultural sector, agricultural producers) can be proposed.
A community of practices (Lave and Wenger, 1991) is formed when professionals come together and organize themselves, either in person or virtually, in a learning process to (i) share information and experiences, (ii) produce knowledge, (iii) interact and cooperate in order to solve together the problems that confront them in their professional activities. The knowledge exchanged is usually generated from experience and constantly evolves with practice. The challenge is to formalize, codify and describe them. The use of information and communications technologies (ICTs) facilitates the effective sharing of knowledge by overcoming time and distance constraints.
An information service consists in making available a set of information, here spatial, that is directly useful for end-users, allowing them to make decisions in the most rational and informed way possible.
An information service links data to needs in a process that is co-constructed between researchers, consultancies and end-users. This service is based on a chain of activities involving research functions, technical, methodological, and financial assistance functions for intermediate operators, and user support functions. This chain of activities covers not only the expression of needs but also the actual implementation stage of the service.
To do so, a community of practices brings together technical centers, service providers and users, with the goal of transforming needs into a formalized and shared demand, which is set out in detailed specifications. First, the community of practices will try to align the views of the various stakeholders. Indeed, end-users are often unaware of the full potential of remote sensing, while operators often have insufficient knowledge of the conditions available to end-users for the implementation of services. Thus, the community of practices will transform needs into a formalized and shared demand with a clear perception of the relationship between the environment/needs and supply. From this first analysis, specifications for the development of operational services will be drafted. These specifications will then form the basis for a call for projects intended to identify the local teams that may be capable of delivering or developing them. Depending on what exists, financing can facilitate the provision of services that are already operational (local deployment, training) or the creation of applications or services that respond to needs that have been expressed but have not yet been addressed.
Figure 1 lays out the process of building information services based on spatial data by using a co-development chain. The identified needs are translated into specifications. To develop the service, existing thematic applications, and products are adapted or new ones developed, using generic products, themselves obtained through image processing. Co-construction involves successive adjustments and iterations which can modify the specifications if they are found to be impossible or too expensive to meet.
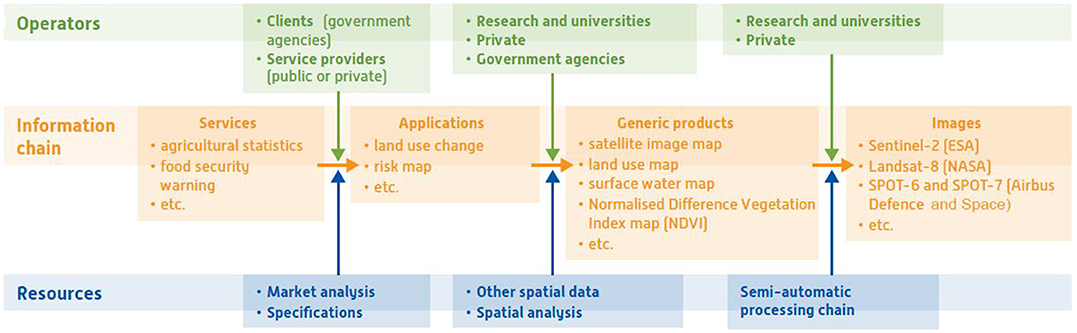
Figure 1. The co-construction of geospatial information services based on EO data (source: Tonneau et al., 2019).
Conclusions and Recommendations
The sociological, environmental, and economic challenges are enormous for the planet and, in particular, for the African continent, which still has to deal with poverty, poor access to the tools of production, increasing population, local conflicts, etc. The human potential and the natural resources of this continent are great, and any help in informing public policies is welcome as a first step for sustainable land management.
Thanks to its objectivity and repeatability, Earth Observation is an essential tool for producing measurable findings that can help decision-making and inform public policies. Over the last 15 years, spatial information has been used in many initiatives to help monitor and implement the UN Sustainable Development Goals. However, a satellite image is not a map, let alone a dashboard, and there are substantial technical and knowledge gaps between satellite images and their derived products; the latter implies the use of and mixes physical, computer, thematic and social sciences (e.g., Montfort et al., 2020).
Today, access to data is facilitated by data repositories and many freely available datasets. Data processing capabilities can be resolved by cloud-based platform such as Google Earth Engine. The arrival of very promising tools and approaches, such as machine learning or deep learning algorithms applied to satellite imagery, in conjunction with relatively high frequency and resolution data, should boost the Earth observation market in the upcoming decade, provided that massive training databases are available, which is not yet the case for many countries in Africa and in other regions of the world.
To benefit from this technological advancement and bridge the gap between technical analysts and policy makers, some key points are fundamental: capacity building, political, will and institutional commitment, public-private partnership, and proofs of concept.
Capacity building should move away from traditional models focused exclusively on training technical specialists and focus on building institutional capacity and creating an enabling environment (UNECA, 2017). It is necessary to train computer scientists with a specialization in geomatics not only because the mastery of computer languages and systems communication takes an increasing amount of expertise but also to reinforce the geomatics skills of specialists (agronomists, hydrologists, geographers, etc.). This capacity building cannot be a singular event because it will always be necessary to train and educate people to be able to replace staff who leave. Capacities do not develop in a vacuum. Therefore, to be useful, they must be anchored in a broader developmental objective, a national developmental strategy, or an initiative under a particular theme such as geospatial information management. This strategy must be implemented at the organizational level (i.e., policies, procedures, and frameworks) to succeed. It can rely on regional centers of excellence, such as RECTAS or AGRHYMET in West Africa, RCMRD in East and South Africa, and on the West African Economic and Monetary Union (UEMOA) support.
At the global level, in 2011, the United Nations launched the Geospatial Information Management Initiative (UN-GGIM) to establish a formal mechanism for member states to review and coordinate their geospatial information management activities globally. The African branch of this initiative, the African Action Plan on Global Spatial Information Management 2016–2030, is a first step that clearly establishes the basis for what this plan should be (UNECA, 2017), but it will never replace the national political will and decision, such as the PNG (Plan National Géomatique17 in Senegal.
The sustainability and success of geospatial information management in Africa requires effective funding. It is necessary to ensure the effective participation of the private sector in the production and management of spatial data through task outsourcing and public-private partnerships. In addition, private structures are probably better able to hire new geomatics graduates.
For all this to succeed, the link between the decision-making level and geomatics expertise must be strengthened. This includes raising awareness about the benefits of space information technology applications and proofs of concept associated with the economic evaluations. These proofs of concept must emerge from participatory approaches to better define and prioritize the actionable information needed and identify the most relevant information dissemination channels according to the different issues. From the authors' experience, one priority could be to produce an annual agricultural land use map for each African country at a scale between 1:50 000 and 1:100 000 based on remote sensing data, local knowledge and expertise, and ground data.
Author Contributions
AB, LL, and J-PT conceived the paper, conducted the literature review and drafted the plan of the paper. All the authors contributed to the writing and to the reviewing.
Funding
This work was supported by CIRAD, the French Agricultural Research and International Cooperation Organization); IRD, the French Research Institute for Development; CNES, the French Spatial Agency; the AFD, the French Development Agency (GeoForAgri project).
Conflict of Interest
The authors declare that the research was conducted in the absence of any commercial or financial relationships that could be construed as a potential conflict of interest.
Acknowledgments
Thanks to all the participants of the Dakar and Abidjan meetings in October 2018, and to the persons that were personally interviewed, for helping us to collect material about the use, and the ways to strengthen this use, of satellite imagery in support of public agricultural and natural resource management policies in West Africa.
Footnotes
1. ^https://www.eo4idi.eu/news/eo4sd-world-bank-agricultural-productivity-agricultural-water-management-and-sustainable-land.
3. ^https://www.copernicus.eu/.
4. ^http://gmes4africa.blogspot.com/.
6. ^https://servirglobal.net/.
7. ^http://www.snv.org/project/stamp-building-success.
8. ^http://www.snv.org/project/mobile-data-moving-herd-management-and-better-incomes-modhem.
10. ^https://www.ignfi.fr/en/portfolio-item/cartographie-burkina-faso/).
11. ^https://www.sansa.org.za/products-services/earth-observation/.
12. ^https://africa-rising.net/2015/09/16/maps-mali/).
13. ^https://www.ignfi.fr/fr/portfolio-item/occupation-des-terres-burkina-fao/).
14. ^https://www.copernicus.eu/fr.
15. ^https://www.actioncontrelafaim.org/a-la-une/un-systeme-d-information-satellite-pour-lutter-contre-la-faim-au-sahel/).
References
Aguiar, D. A., Rudorff, B. F. T., Silva, W. F., Adami, M., and Mello, M. P. (2011). Remote sensing images in support of environmental protocol: monitoring the sugarcane harvest in sao paulo state, Brazil. Remote Sens. 3, 2682–2703. doi: 10.3390/rs3122682
Arias, M., and Inglada, J. (2015). “Benchmarking for L4 crop type product, version 1.1,” in Sentinel-2 for Agriculture Design Justification File, ed UCL (Louvain: ESA Sentinel-2 for Agriculture project), 20–21.
Arvor, D., Jonathan, M., Simões, M., Dubreuil, V., and Durieux, L. (2011). Classification of MODIS EVI time series for crop mapping in the state of Mato Grosso, Brazil. Int. J. Remote Sens. 32, 7847–7871. doi: 10.1080/01431161.2010.531783
Atzberger, C. (2013). Advances in remote sensing of agriculture: context description, existing operational monitoring systems and major information needs. Remote Sens. 5, 949–981. doi: 10.3390/rs5020949
AUDA (2010). Accelerating CAADP Country Implementation. A Guide for Implementors. Midrand: African Union Development Agency (AUDA).
Auerswald, P. E., and Lokesh, D. (2017). “Economic Ecosystems,” in The New Oxford Handbook of Economic Geography, eds G. L. Clark, M. P. Feldman, M. S. Gertler, and D. Wójcik (New York, NY: Oxford University Press), 245–268.
Becker-Reshef, I., Barker, B., Humber, M., Puricelli, E., Sanchez, A., Sahajpal, R., et al. (2019). The GEOGLAM crop monitor for AMIS: assessing crop conditions in the context of global markets. Glob. Food Sec. 23, 173–181. doi: 10.1016/j.gfs.2019.04.010
Becker-Reshef, I., Justice, C., Barker, B., Humber, M., Rembold, F., Bonifacio, R., et al. (2020). Strengthening agricultural decisions in countries at risk of food insecurity: the GEOGLAM crop monitor for early warning. Remote Sens. Environ. 237:11553. doi: 10.1016/j.rse.2019.111553
Bégué, A., Arvor, D., Bellon, B., Betbeder, J., de Abelleyra, D., Ferraz, R. P. D., et al. (2018). Remote sensing and cropping practices: a review. Remote Sens. 10:99. doi: 10.3390/rs10010099
Bégué, A., Charrier, B., Torre, C., Lo Seen, D., Tonneau, J.-P., Leroux, L., et al. (2016). “Observation spatiale pour l'agriculture en Afrique: Potentiels et défis,” in Notes Techniques, ed AFD (Paris: AFD), 15–25.
Bernardi, M., Deline, J., Durand, W., and Zhang, N. (2016). Crop Yield Forecasting: Methodological and Institutional Aspects. Rome: FAO.
Chavez, A. B., Broadbent, E. N., and Zambrano, A. M. A. (2014). Smallholder policy adoption and land cover change in the southeastern Peruvian Amazon: a twenty-year perspective. Appl. Geogr. 53, 223–233. doi: 10.1016/j.apgeog.2014.06.017.
CILSS (2009). “Des statistiques agricoles fiables pour une meilleure gestion de la sécurité alimentaire au Sahel et en Afrique de l'ouest,” in 24ème Journée du CILSS, ed CILSS (Ouagadougou, BF: Comité inter-Etats de lutte contre la sécheresse au Sahel), 1–20.
Collier, P., and Dercon, S. (2014). African agriculture in 50 years: smallholders in a rapidly changing world. World Dev. 63, 92–101. doi: 10.1016/j.worlddev.2013.10.001
Conceição, P., Levine, S., Lipton, M., and Warren-Rodriguez, A. (2016). Toward a food secure future: ensuring food security for sustainable human devlopment in Sub-Saharan Africa. Food Policy 60, 1–9. doi: 10.1016/j.foodpol.2016.02.003
Defourny, P., Bontemps, S., Bellemans, N., Cara, C., Dedieu, G., Guzzonato, E., et al. (2019). Near real-time agriculture monitoring at national scale at parcel resolution: performance assessment of the Sen2-Agri automated system in various cropping systems around the world. Remote Sens. Environ. 221, 551–568. doi: 10.1016/J.RSE.2018.11.007
Diouf, A. A., Brandt, M., Verger, A., El Jarroudi, M., Djaby, B., Fensholt, R., et al. (2015). Fodder biomass monitoring in sahelian rangelands using phenological metrics from FAPAR time series. Remote Sens. 7, 9122–9148. doi: 10.3390/rs70709122
Dixon, J. A., Gibbon, D. P., and Gulliver, A. (2001). Systèmes de Production Agricole et Pauvreté. Rome et Washington, DC: FAO et Banque mondiale.
ESA (2017). “Agriculture and Rural Development|Service Portfolio,” in EO4SD – Earth Observation for Sustainable Devlopment (Frascati: European Space Agency).
FAO (2015). “FAO Desert Locust Information Service (DLIS) helps countries to control Desert Locust,” in FCC-EMPRES Information Sheets (Rome: FAO).
FAO (2017). Regional Overview of Food Security and Nutrition in Africa 2017. The Food Security and Nutrition–Conflict Nexus: Building Resilience for Food Security, Nutrition and Peace. Accra: Food and Agriculture Organization of the United Nations.
Felix, A. (2006). Éléments pour une refonte des politiques agricoles en Afrique sub-saharienne. Afrique Contemporaine 217, 159–172. doi: 10.3917/afco.217.0159
Félix, G. F., Diedhiou, I., Le Garff, M., Timmermann, C., Clermont-Dauphin, C., Cournac, L., et al. (2018). Use and management of biodiversity by smallholder farmers in semi-arid West Africa. Glob. Food Sec. 18, 76–85. doi: 10.1016/J.GFS.2018.08.005
Fritz, S., See, L., Bayas, J. C. L., Waldner, F., Jacques, D., Becker-Reshef, I., et al. (2019). A comparison of global agricultural monitoring systems and current gaps. Agric. Syst. 168, 258–272. doi: 10.1016/J.AGSY.2018.05.010
Fritz, S., See, L., McCallum, I., You, L., Bun, A., Moltchanova, E., et al. (2015). Mapping global cropland and field size. Glob. Change Biol. 21, 1980–1992. doi: 10.1111/gcb.12838
Fritz, S., See, L., and Rembold, F. (2010). Comparison of global and regional land cover maps with statistical information for the agricultural domain in Africa. Int. J. Remote Sens. 31, 2237–2256. doi: 10.1080/01431160902946598
Gómez, C., White, J. C., and Wulder, M. A. (2016). Optical remotely sensed time series data for land cover classification: a review. J. Photogr. Remote Sens. 116, 55–72. doi: 10.1016/j.isprsjprs.2016.03.008
Goyal, A., and Nash, J. D. (2017). Reaping Richer Returns: Public Spending Priorities for African Agriculture Productivity Growth. Washington, DC: World Bank Group.
GSARS (2017). Handbook on Remote Sens. for Agricultural Statistics. Rome: Global Strategy to improve Agricultural and Rural Statistics.
Hale, J. L., Householder, B. J., and Greene, K. L. (2002). “The theory of reasoned action,” in The Handbook of Persuasion, eds J. P. Dillard and M. Pfau (Thousand Oaks, CA: Sage), 259–286.
Harris, R. (2002). Earth observation and UK science policy. Space Policy 18, 205–213. doi: 10.1016/s0265-9646(02)00020-6
HLPE (2013). Investing in Smallholder Agriculture for Food Security. A report by the High Level Panel of Experts on Food Security and Nutrition of the Committee on World Food Security. Rome: High Level Panel of Experts.
Horlings, L. G., and Marsden, T. K. (2011). Towards the real green revolution? Exploring the conceptual dimensions of a new ecological modernisation of agriculture that could 'feed the world'. Glob. Environ. Change 21, 441–452. doi: 10.16/j.gloenvcha.2011.01.004
Ibrahim, W. Y., Batzli, S., and Menzel, W. P. (2014). Agricultural policy effects on land cover and land use over 30 years in tartous, syria, as seen in landsat imagery. J. Appl. Remote Sens. 8:083506. doi: 10.1117/1.JRS.8.083506
IMAAFS (2014). “Key conference findings and conclusions,” in Information for Meeting Africa's Agricultural transformation and Food Security goals (IMAAFS). eds H. Josserand and F. Rembold (Addis Ababa: JRC), 1–17.
Jayne, T. S., Chamberlin, J., and Headey, D. D. (2014). Land pressures, the evolution of farming systems, and development strategies in Africa: a synthesis. Food Policy 48, 1–17. doi: 10.1016/j.foodpol.2014.05.014
KolalavalIi, S., Flaherty, K., Al-Hassan, R., and Owusu Baah, K. (2010). “Do Comprehensive Africa Agriculture Development Program (CAADP) Processes Make a Difference to Country Commitments to Develop Agriculture? The Case of Ghana,” in IPFRI Discussion Paper, ed IFPRI (IFPRI: Washington, DC), 1–37.
Kong, R., Diepart, J.-C., Castella, J.-C., Lestrelin, G., Tivet, F., Belmain, E., et al. (2019). Understanding the drivers of deforestation and agricultural transformations in the Northwestern uplands of Cambodia. Appl. Geogr. 102, 84–98. doi: 10.1016/j.apgeog.2018.12.006
Lambert, M.-J., Waldner, F., and Defourny, P. (2016). Cropland mapping over Sahelian and Sudanian agrosystems: a knowledge-based approach using PROBA-V time series at 100m. Remote Sens. 8:232. doi: 10.3390/rs8030232
Latham, J., Cumani, R., Rosati, I., and Bloise, M. (2014). FAO Global Land Cover (GLC-SHARE) Beta-Release 1.0 Database. FAO.
Lave, J., and Wenger, E. (1991). Situated Learning: Legitimate Peripheral Participation. Cambridge: Cambridge University Press.
Leif, E. C., Bezanson, K., Lele, U., Davies, M., Perez del Castillo, C., and Awori, T. (2007). FAO: The Challenge of Renewal. an Independent External Evaluation of the Food and Agriculture Organization. Rome: FAO.
Lein, J. K. (2012). Environmental Sensing: Analytical Techniques for Earth Observation. New York, NY: Springer.
López-Lozano, R., and Baruth, B. (2019). An evaluation framework to build a cost-efficient crop monitoring system. Experiences from the extension of the European crop monitoring system. Agric. Syst. 168, 231–246. doi: 10.1016/j.agsy.2018.04.002
Losch, B., and Freguin-Gresh, S. (2011). “Les défis de la transition en Afrique sub-saharienne : comment accompagner la transformation structurelle du continent dans la mondialisation?,” in Transition Démographique et Emploi en Afrique Subsaharienne : Comment Remettre l'emploi au Coeur Des Politiques De Développement, eds R. Beaujeu, R. Kolie, J.-F. Sempere, and C. Uhder (Paris: AFD), 34–40.
Mellor, J. W. (2014). High rural population density in Africa: what are the growth requirements and who participates? Food Policy 48, 66–75. doi: 10.1016/j.foodpol.2014.03.002
Montfort, F., Bégué, A., Leroux, L., Blanc, L., Gond, V., Cambule, A., et al. (2020). From land productivity trends to land degradation, a question of perception – Demonstration in Mozambique. Land Degradation and Development.
Öborn, I., Vanlauwe, B., Phillips, M., Thoas, R., Brooijmans, W., and Atta-Krah, K. (2017). Sustainable Intensification in Smallholder Agriculture. London: Routledge.
Pingali, P. (2010). “Agriculture renaissance: making “agriculture for development” work in the 21st century,” in Handbook of Agricultural Economics, eds R. Evenson and P. Pingali (Amsterdam: Elsevier), 3867–3894.
Pisani, E., and Chatellier, V. (2010). La faim dans le monde, le commerce et les politiques agricoles. Revue française d'économie XXV, 3–77. doi: 10.3917/rfe.101.0003
Rudorff, B. F. T., Adami, M., Aguiar Daniel Alves, Moreira, M. A., Mello, M. P., Fabiani, L., et al. (2011). The soy moratorium in the amazon biome monitored by remote sensing images. Remote Sens. 3, 185–202. doi: 10.3390/rs3010185
Saah, D., Tenneson, K., Matin, M., Uddin, K., Cutter, P., Poortinga, A., et al. (2019). Land cover mapping in data scarce environments: challenges and opportunities. Front. Environ. Sci. 7:150. doi: 10.3389/fenvs.2019.00150
Samberg, L. H., Gerber, J. S., Ramankutty, N., Herrero, M., and West, P. C. (2016). Subnational distribution of average farm size and smallholder contributions to global food production. Environ. Res. Lett. 11:124010. doi: 10.1088/1748-9326/11/12/124010
Schmedtmann, J., and Campagnolo, M. L. (2015). Reliable crop identification with satellite imagery in the context of common agriculture policy subsidy control. Remote Sens. 7, 9325–9346. doi: 10.3390/rs70709325
Shimeles, A., Verdier-Chouchane, A., and Boly, A. (2018). “Introduction: understanding the challenges of the agricultural sector in Sub-Saharan Africa,” in Building a Resilient and Sustainable Agriculture in Sub-Saharan Africa, eds A. Shimeles, A. Verdier-Chouchane, and A. Boly (Cham: Palgrave Macmillan), 1–12.
SNV (2019). “When satellites guide pastoralists in Sahel. Key lessons learned from STAMP project (2015-2018),” in: Technical Note. eds C. Le Côme, M. Cissé and J. Thiam (Bamako: Netherlands Development Organisation), 1–12.
Tong, X., Brandt, M., Hiernaux, P., Herrmann, S., Rasmussen, L. V., Rasmussen, K., et al. (2020). The forgotten land use class: mapping of fallow fields across the Sahel using Sentinel-2. Remote Sens. Environ. 239:111598. doi: 10.1016/j.rse.2019.111598
Tonneau, J.-P., Bégué, A., Leroux, L., Augusseau, X., Faure, J.-F., Mertens, B., et al. (2019). “Geospatial information for African agriculture: a key investment for agricultural policies,” in Perspective 51, eds P. Caron and C. Fovet-Rabot (Montpellier: CIRAD), 3.
Traore, S. B., Ali, A., Tinni, S. H., Samake, M., Garba, I., Maigari, I., et al. (2014). AGRHYMET: a drought monitoring and capacity building center in the West Africa Region. Weather Clim. Extremes 3, 22–30. doi: 10.1016/j.wace.2014.03.008
Tsendbazar, N.-E., Herold, M., de Bruin, S., Lesiv, M., Fritz, S., Van De Kerchove, R., et al. (2018). Developing and applying a multi-purpose land cover validation dataset for Africa. Remote Sens. Environ. 219, 298–309. doi: 10.1016/J.RSE.2018.10.025
Turner, II. B. L. (2010). Land change in the southern Yucata'n: case studies in land change science. Reg. Environ. Change 10, 169–174. doi: 10.1007/s10113-010-0129-1
UN (2019a). World Population Prospects 2019: Highlights. New-York: United Nations, Population Division of the Department of Economic and Social Affairs.
UN (2019b). World Urbanization Prospects 2018: Highlights. New-York, NY: United Nations, Department of Economic and Social Affairs, Population Division.
UNECA (2017). Geospatial Information for Sustainable Development in Africa - African Action Plan on Global Geospatial Information Management 2016-2030. Addis Ababa: United Nations Economic Commission for Africa, (UNECA).
USAID, NASA, RCMRD, ICIMOD, ADPC, and CILSS (2019). SERVIR Global, A Retrospective Report 2014-2018. Available online at: https://www.servirglobal.net/multimedia/annual-reports.
Waldner, F., Fritz, S., Di Gregorio, A., and Defourny, P. (2015). Mapping priorities to focus cropland mapping activities: fitness assessment of existing global, regional and national cropland maps. Remote Sens. of Environ. 7, 7959–7986. doi: 10.3390/rs70607959
Whitcraft, A. K., Becker-Reshef, I., Killough, B. D., and Justice, C. O. (2015a). Meeting earth observation requirements for global agricultural monitoring: an evaluation of the revisit capabilities of current and planned moderate resolution optical earth observing missions. Remote Sens. 7, 1482–1503. doi: 10.3390/rs70201482
Whitcraft, A. K., Vermote, E. F., Becker-Reshef, I., and Justice, C. O. (2015b). Cloud cover throughout the agricultural growing season: impacts on passive optical earth observations. Remote Sens. Environ. 156, 438–447. doi: 10.1016/j.rse.2014.10.009
Wilson, J., and Gapsiso, N. D. (2018). Information and communication technology and agricultural development in Africa: myth or reality? J. Agric. Econ. Environ. Soc. Sci. 4, 48–58.
Woodhouse, P. (2010). Beyond industrial agriculture? Some questions about farm size, productivity and sustainability. J. Agrarian Change 10, 437–453. doi: 10.1111/j.1471-0366.2010.00278.x
World Bank, FAO and UN (2010). Global Strategy to Improve Agricultural and Rural Statistics. Washington DC: World Bank, FAO, United Nations.
Keywords: satellite image, food security, land management, early warning, geospatial information, EO-based services
Citation: Bégué A, Leroux L, Soumaré M, Faure J-F, Diouf AA, Augusseau X, Touré L and Tonneau J-P (2020) Remote Sensing Products and Services in Support of Agricultural Public Policies in Africa: Overview and Challenges. Front. Sustain. Food Syst. 4:58. doi: 10.3389/fsufs.2020.00058
Received: 15 October 2019; Accepted: 09 April 2020;
Published: 14 May 2020.
Edited by:
Molly E. Brown, University of Maryland, United StatesReviewed by:
Melanie Kolb, National Autonomous University of Mexico, MexicoGregory Husak, University of California, Santa Barbara, United States
Copyright © 2020 Bégué, Leroux, Soumaré, Faure, Diouf, Augusseau, Touré and Tonneau. This is an open-access article distributed under the terms of the Creative Commons Attribution License (CC BY). The use, distribution or reproduction in other forums is permitted, provided the original author(s) and the copyright owner(s) are credited and that the original publication in this journal is cited, in accordance with accepted academic practice. No use, distribution or reproduction is permitted which does not comply with these terms.
*Correspondence: Agnès Bégué, YWduZXMuYmVndWUmI3gwMDA0MDtjaXJhZC5mcg==