- 1Department of Crop Production Ecology, Swedish University of Agricultural Sciences, Uppsala, Sweden
- 2International Center for Tropical Agriculture (CIAT), Cali, Colombia
- 3Department of Botany, Jomo Kenyatta University of Agriculture and Technology, Nairobi, Kenya
- 4Department of Agriculture, Cape Peninsula University of Technology, Wellington, South Africa
- 5Department of Soil and Environment, Swedish University of Agricultural Sciences, Uppsala, Sweden
- 6Department of Environmental Science, Bindura University of Science Education, Bindura, Zimbabwe
- 7Department of Plant, Soil and Microbial Sciences, Michigan State University, East Lansing, MI, United States
In Sub-Saharan Africa (SSA), many smallholder communities continuously grapple with soil-based poverty as the coarse-textured (sandy) soils, on which they eke out a living, have low water and nutrient retention capacities. The new sub-surface water retention technology (SWRT), which is based on the subsurface installation of impermeable water-retaining membranes of linear and low-density polyethylene, reduces the amount of water and nutrients lost through deep percolation especially on coarse-textured soils. In this study, we used stochastic simulations on the diffusion of SWRT on coarse-textured soils in eight different Eastern and Southern African countries. Using results from previous research on SWRT we estimated increases in maize grain yields and biomass accumulation. Results from the most promising diffusion scenarios suggested that with 20 years of widespread adoption of SWRT regional maize production could increase by 15 and 50 million tons per season. Carbon sequestration could reach 15 tons around 22 years after implementation (YAI) for the best diffusion scenario because of increased biomass production following SWRT adoption. The increased grain yield and carbon sequestration are limited by the initial state of SWRT adoption, which we expect will be based on the extent of awareness and promotional campaigns conducted by governments and development practitioners. While our results suggest synergistic reductions in the rate of water and nutrient loss from crop root zones, SWRT is characterized by high initial financial and labor investment costs, which without effective financial support, would be prohibitive to uptake by the generally resource-limited smallholder farmers working on sandy soils. Moreover, as SWRT is new, there is a need for more dissemination efforts to increase awareness on the technologies amongst extension workers, decision-makers, and agricultural investors that can promote and incentivize the adoption of this technology in areas where agricultural productivity is constrained by coarse-textured soils.
Introduction
Agricultural production in Sub-Saharan Africa (SSA) is constrained by factors related to soil quality, some of which are attributable to highly permeable coarse-textured soils. Coarse-textured soils (Arenosols) cover an estimated 21.5% of continental Africa (Dewitte et al., 2013), and are widely spread in the southern part of the continent (Hartemink and Huting, 2005, 2008). The soil organic carbon (SOC) content of these soils is generally low and rarely exceeds 10 g kg−1 (Hartemink and Huting, 2008; Singh et al., 2011). To exacerbate the challenge, these soils are generally in regions that are characterized by arid and semi-arid climates, where rainfall is erratic. While irrigation and water harvesting are among key technologies recommended for crop production on coarse-textured soils (Zougmore et al., 2018), the expansion of irrigation technologies is constrained by the scarcity of water, lack of on-farm irrigation techniques and equipment and lack of technical know-how among most smallholder communities. A combination of these factors explain why, despite the importance of irrigation under arid and semi-arid climatic conditions, only 4% of the cultivated area in Africa is equipped with irrigation infrastructure (Svendsen et al., 2009).
Coarse-textured soils, which are characterized by high water and nutrient percolation rates, represent a major resource loss pathway, which negatively affects limited-resource-endowed farmers that eke out a living through crop production on these soils. Technological options that increase water and nutrient retention will have far-reaching agroecological and economic implications by potentially increasing crop production and yield stability while reducing costs associated with the inefficient use of fertilizers, and rainfall and/or irrigation water (Ismail and Ozawa, 2007).
Technological options that decelerate the deep percolation of water and nutrients by changing soil composition and structure have different merits and limitations. For example:
1. Addition of organic matter (e.g., compost, livestock manure, and leaf litter) increases water retention and hence the productivity of sandy soils (Rawls et al., 2003). Yet, high tropical temperatures, low soil clay content, and poor soil aggregation make soil organic matter vulnerable to microbial breakdown resulting in short-term benefits (Vanveen and Kuikman, 1990).
2. Biochar application improves water retention capacity of sandy soils (Glab et al., 2016). However, uncertainty of production gains at realistic application rates, competing uses of limited biomass resources, and transportation costs of large biomass volumes, lack of technical know-how and potentially negative environmental impacts associated with biochar production have contributed to limited adoption of biochar use (Gwenzi et al., 2015).
3. Treating sandy soils with clay potentially improves their water retention capacity (Ismail and Ozawa, 2007). Yet, overlaying or incorporation of clay/termitaria soil on large areas is costly (McKissock et al., 2000), labor intensive, and gives inconsistent yield benefits (Garba et al., 2011) because of heterogeneity in the chemical properties of termitaria soil.
4. Soil conditioners or superabsorbent material that absorb water and swell up to several times their weight improve soil aggregation, reduce water infiltration and saturated hydraulic conductivity rates (Al-Omran and Al-Harbi, 1997; Wenbo et al., 2013). Large-scale adoption of soil conditioners is currently limited by high costs associated with required annual new applications. Under tropical conditions, the rates can be very high due to the state of soil degradation, thus; insufficient longevity; and inconsistency of effects on soil properties (Falatah et al., 1996).
5. Water barriers such as pond sediments (Gupta and Aggarwal, 1980) and asphalt (Erickson et al., 1968), and large sheets of polyethylene (Garrity et al., 1992) have also been tested and abandoned due to amplified vulnerability to either drought or flooding events.
A relatively new subsurface water retention technology (SWRT) represents an in-field water harnessing and a self-draining design useful for optimizing soil water contents in plant root zones promoting long-term and sustainable food production on coarse-textured soil. SWRT is a zero-maintenance technology based on engineered impermeable water micro-reservoirs located below and/or adjacent to plant root zones (Kavdir et al., 2014). Precisely engineered SWRT membranes reduce the amounts of water and nutrients lost through deep percolation especially on coarse-textured soils, resulting in optimal soil water nutrients, and oxygen contents in root zones of most field-grown plants (Guber et al., 2015). Dual layers of spatially arranged water-saving membranes (see Figure S1, from Kavdir et al., 2014) retain much of the water added to the soil surface by rainfall or irrigation for prolonged periods. Both the shape and layout of the membranes have a huge impact on the amounts of retained water and nutrients. For instance, in a modeling study on SWRT membranes, Guber et al. (2015) showed that the 2:1 aspect ratio of U-shaped SWRT membranes retained and maintained the most optimal soil water, nutrients and oxygen in plant root zones, thus meeting crop water demands for 5–7 days longer than sandy soils without water retaining membranes. Once installed, the SWRT can last over 50 years, while the return on investment is between 2 and 5 years, depending on market prices of the selected crop (Kavdir et al., 2014). Therefore, we expect the use of SWRT to increase agricultural productivity on low output marginal lands, and soils abandoned due to poor physical characteristics.
The overall objective of this study was to model scenarios of adoption and diffusion of SWRT and thereafter to estimate subsequent increases in maize grain yields, crop biomass accumulation and, consequently, soil carbon sequestration. Based on potential benefits, we discuss the trade-offs and synergies associated with local environmental, socio-economic conditions and policy implications following the diffusion and adoption of SWRT in eight East and Southern African countries. Potential impacts of adopting the SWRT were determined by combining data on (i) the area under coarse-textured soils (Arenosols) in the eight East and Southern African countries (Hengl et al., 2015); and (ii) yields obtained from fields where SWRT membranes were installed in other water-limited regions. We selected Maize (Zea mays L.) because of its importance as a major food crop in SSA.
Materials and Methods
The Extent of Arenosols in the Study Regions
Data on Arenosols in six Southern African countries (Angola, Botswana, Mozambique, Namibia, South Africa, and Zimbabwe) and two East African countries (Kenya and Tanzania) were obtained from the Africa SoilGrids database (Hengl et al., 2015) with emphasis on the topsoil (0–5 cm) sand fraction (see Figure 1). Arenosols under forest, deserts or dunes or alkaline soils were excluded (see Table S1 adapted from Hartemink and Huting, 2005; Supplementary Material). Data on the total area of Arenosols, under crop production, in the eight countries were integrated into a stochastic logistic model to account for the randomness in the diffusion and adoption patterns of SWRT.
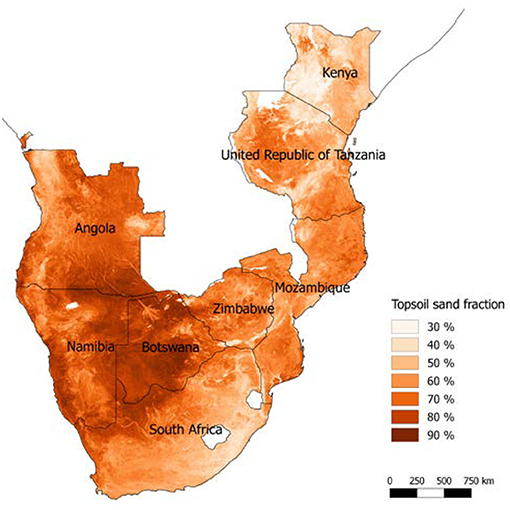
Figure 1. The topsoil (0–5 cm) sand fractions in six Southern African and two East African countries (Hengl et al., 2015).
Stochastic Logistic Function and Assumptions on SWRT Diffusion and Adoption
The simple logistic growth function of Verhulst (Bacaër, 2011), which has previously been applied in economics and sociology, to estimate technology adoption and diffusion (Rogers, 2003; Gordon et al., 2017) was used. In this approach, adopters are grouped in four different groups: early adopters, early majority, a late majority or laggards. In our case, adoption was characterized as the coarse-textured soil with SWRT installed. This implies that the proxy for rate of adoption is an increase in the area of coarse-textured soils with SWRT membranes.
The percentage area of coarse-textured soils with SWRT at any given time depends on parameters such as the initial state, the maximum adoption and the rate of diffusion. Yet, the rate of technology diffusion is a dynamic process, which depends on factors such as learning through information (e.g., extension services and government programs), acquisition of individual or collective experience and access to financial resources for initial investment. These factors introduce uncertainty in determining the extent of expansion of SWRT over a given period. To capture this uncertainty, we used a stochastic logistic model to introduce a random effect to a logistic deterministic function. In the mid-twentieth century, the notion of sigmoid curves using the percentage area planted with new maize hybrid was introduced (Grilichies, 1957). Later, the stochastic behavior of the adoption of new technologies was added (Hierbert, 1974) and a review of models of the adoption behavior of individual firms (Feder et al., 1982). The stochastic simulation algorithm, with the Gillespie procedure in the R package Gillespie's Stochastic Simulation Analysis (GSSA) (Pineda-Krch, 2008), was applied for estimating the area with SWRT using the following equation:
Where A(t) is the area (%) with SWRT at time t, No is the initial state of coarse-textured soils (in percentage) under SWRT at the time t0, K is the total coarse-textured soils (100%) in the target region, r is the rate of diffusion and g(t) ε is the error caused by the stochasticity of the adoption behavior. The outcome can vary significantly depending on parameters associated with the rate of technology adoption, the state-change matrix and the propensity function (Pineda-Krch, 2008).
For the implementation of GSSA, we first assumed that the rate of technology diffusion (r) varies with new SWRT adopters as well as adopters who, for some reasons, might withdraw from using fields with SWRT for crop production. Nine realizations (scenarios) were simulated, where diffusion rate per season is one to five new adopters per year without withdrawal (S1-0, S2-0, S3-0, S4-0, and S5-0) and two to five new adopters with one adopter withdrawing from using fields with SWRT for maize production (S2-1, S3-1, S4-1, and S5-1). Even though simulations with and without withdrawal end up with the same diffusion rate, which controls the shape of the curve, the corresponding simulation outcomes differed in terms of volatility associated with different realizations from the stochastic model (Wilkinson, 2006). Before estimating the effects of the technology on grain yield and carbon sequestration, we compared the simulation outcomes with and without withdrawal of use of fields with installed SWRT membranes for crop production. Second, we tested the effects of four propensity functions (0.1, 0.05, 0.03, and 0.02) for each of the different adoption rates without withdrawals of the acreage in coarse-textured soils with SWRT and crop production. We retained the best and conservative propensity level of 0.03, which has also been found to be the average of coefficient of innovation in a meta-analysis on 213 applications (Sultan et al., 1990). Third, we emphasized the influence of initial state parameter that requires initial investments in terms of installation of SWRT before early adopters can take over the technology. The three steps provided us possible projection pathways with one propensity level, five adoption rates and six initial states.
GSSA is a versatile and extensible framework for stochastic simulation and provides a simple interface to Monte Carlo implementations of the stochastic simulation algorithm (Pineda-Krch, 2008). The dynamic or kinetic Monte Carlo method is useful in the optimization, numerical integration and generation of samples from a probability (Rui-mel, 2015). The utility of the Monte Carlo method has resulted in it being used in many disciplines to determine possible outcomes associated with random effects, over time (Battaile and Srolovitz, 2002; Fang and Giles, 2019; Rausch et al., 2019; Togashi, 2019; Tracheva and Ukhinov, 2019). The step size of the explicit tau-leaping (or τ-leaping) was used to improve the clarity of the illustrations and to reduce the time of calculations (Gillespie, 2001; Cao et al., 2006).
Estimation of Maize Production Increase and Soil Organic Carbon Sequestration
For the estimation of maize production and SOC sequestration, we targeted the implication of having farmers on 20–50% of the total sandy soils area adopting SWRT compared to no adoption. The time after which that range of adoption occurs depended on the scenario and the initial states. Using an increase of the area with SWRT, the production levels of maize grain were calculated through a linear function based on each respective country's coarse-textured areas and percentage increases of maize grain and biomass production obtained in other previous studies. Specifically, we used mean yield increases in grain and biomass from a 5-year crop rotation of maize-soybean/potato/vegetable, which were correspondingly 67% and 91% with and without irrigation compared to a control without SWRT (Unpublished data, Smucker et al., 2018). As we could not get maize yields under coarse-textured soils for the respective countries, we used figures of national grain yields per country by averaging the yields obtained in 2012, 2013, and 2014 in the respective countries1 Yield levels of each country are in Table S1.
Estimations of carbon sequestration were based on experimental data from SWRT (e.g., harvest index of maize and increase in total biomass), published values and some assumptions (Bolinder et al., 1999; Zhang et al., 2014). For example, we assumed that if all the maize fodder is left and incorporated in the field, between 20 and 30% would be converted into soil organic matter (Zhang et al., 2014) the rest being consumed by microbes, soil fauna and emitted as carbon dioxide. The total biomass input was obtained by summing the aboveground biomass and belowground biomass and we assumed that about 30% of the this biomass would be incorporated into the various soil carbon pools. SOC was estimated to be ~58% of the total biomass input (Cai et al., 2017; Komainda et al., 2018). We then deducted 3% of carbon of the available SOC as mineralized each year. We calculated carbon sequestration using the above assumption and following the adoption and diffusion pattern in combination with the increases (%) for different options (Irrigation + SWRT) and local production levels.
Results
Patterns of Adoption and Diffusion
The comparisons of stochastic modeling of the adoption with and without withdrawal showed a rather good relationship with a coefficient of determination R2 = 0.99 (Figure 2). However, it was also shown that the volatility caused by different realizations with stochastic model resulted in marginal differences where adoptions with withdrawals could increase slowly (Figure 2B) and quicker (Figures 2C,D). With the same propensity level the use of different adoption rates and initial states in the stochastic simulations resulted in significantly different pathways on the area with SWRT membranes (Figure 3).
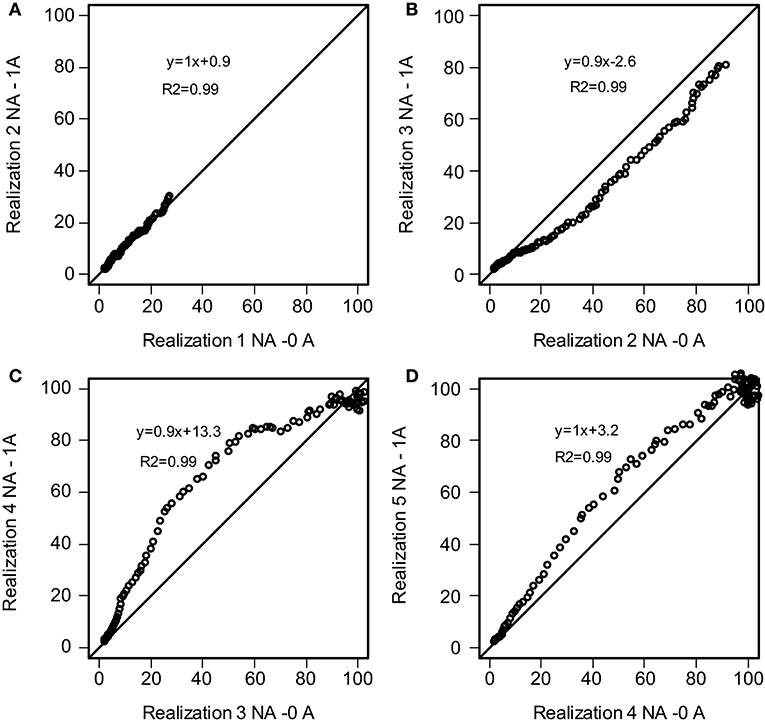
Figure 2. Linear relationships of two scenario parameters (with and without withdrawal of adopters) in stochastic simulations based on a logistic function to calculate the new area with SWRT over time. (A) y-axis represents output with two new adopters and one withdrawal against one new adopter in x-axis, (B) y-axis represents output with three new adopters and one withdrawal against two new adopter in x-axis, (C) y-axis represents output with four new adopters and one withdrawal against three new adopter in x-axis, and (D) y-axis represents output with five new adopters and one withdrawal against four new adopter in x-axis. The straight line represents a perfect relationship (1:1).
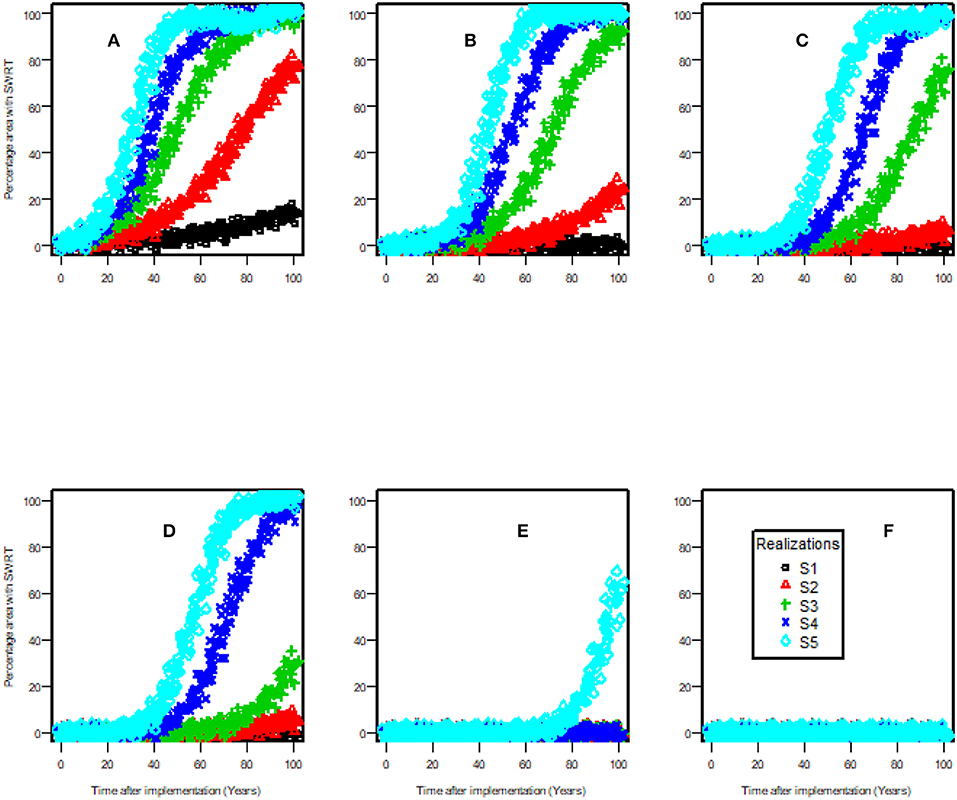
Figure 3. Percentage of area projections with SWRT in stochastic simulations of five SWRT diffusion rates for the different numbers of new adopters per year (S1 = 1, S2 = 2, S3 = 3, S4 = 4, and S5 = 5) with six initial values (N0) in percentage area [(A) N0 = 1, (B) N0 = 0.1, (C) N0 = 0.05, (D) N0 = 0.01, (E) N0 = 0.005, and (F) N0 = 0.001].
By changing the initial states, the number of years after implementation (YAI) required to reach a certain adoption level of SWRT on the coarse-textured soils changed greatly (Figure 3). The scenarios with the fastest diffusion rate (i.e., S4 and S5) suggested slightly similar adoption levels and the only ones reaching the plateaux before 100 years after technology penetration, at least with the four highest initial states (1, 0.1, 0.05, and 0.01) corresponding to Figures 3A–D, respectively. The initial states below 0.01 did not allow significant adoption level for any of the adoption rates (Figures 3E,F), except for S5 (Figure 3F). The modest diffusion rate (S1) was below 20% of adoption level in all the simulations. Scenarios S2 and S3, with a medium rate of diffusion and adoption, reached 40% of adoption level only for high initial states 1, 0.1, and 0.05 and S3 could only reach the plateau for the highest initial state. The estimated time required for the diffusion to reach 50% of the coarse-textured soils was lower for higher initial states and adoption rate (Figure 4).
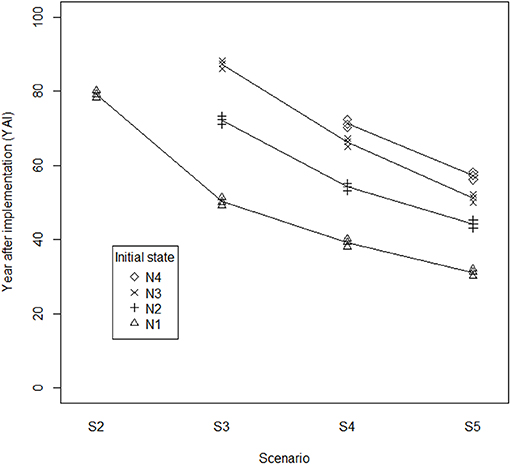
Figure 4. Estimated number of years required for SWRT to cover 50% of the total area of coarse-textured soils for each scenario (S2, S3, S4, and S5) at different initial state levels (N1 = 1, N2 = 0.1, N3 = 0.05 and N4 = 0.01). Scenario S1 remained below 50% adoption regardless of the initial state.
Estimated Maize Production Increase and Carbon Sequestration
Regardless on when the 20–50% of adoption happened in the different projections, the expected potential grain yield, and biomass increases varied between 15 and 38 million tons per season (Figure 5) when SWRT is installed but without irrigation (the dark gray). SWRT with irrigation would then mean an additional increase equivalent to the ca. 5–12 million tons per season (light gray area). With the most optimistic projections (S5 and S4) and an initial state of 1%, adoption level of 20% would reach after about 30 years of implementation. As the initial state decreases, the required years of implementation to reach a state where 20% of the total coarse-textured soils area has SWRT will increase. Increases in grain yield in each country showed that Angola and Mozambique would benefit the most from SWRT adoption while maize gain yield increases would be lowest in Kenya and Tanzania (Figure S2).
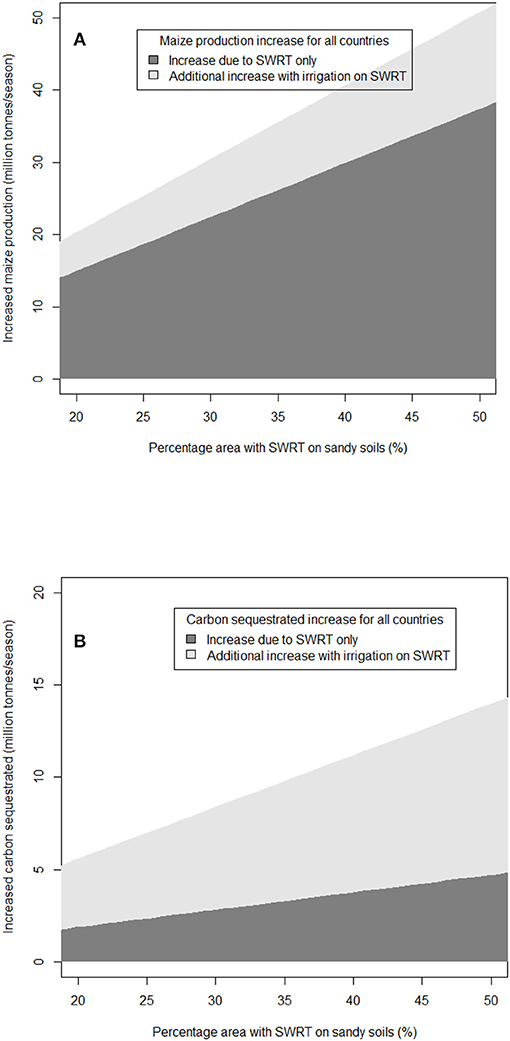
Figure 5. Estimates of maize production increases (A) and carbon sequestration (B) for 20 to 50% of new land under the adoption of SWRT for all the eight countries. The dark gray area represents the added value of SWRT without irrigation while the light gray area is the added value brought about by irrigation after SWRT adoption. Increases of grain yield were based on experimental results, local maize production yields levels for 2012, 2013, and 2014 (Food and Agriculture Organization, 2018) with the existing coarse-textured soils in the countries.
The estimated range of sequestrated carbon would be between 5 and 14 million tons of carbon with SWRT and irrigation (Figure 5).
Discussion
The Importance of the Initial State for the Adoption Outcome
Simulation of the adoption and diffusion of SWRT revealed the importance of the initial state as a trigger to adoption. In spite of higher propensity level or adoption rate, it will take many YAI to see significant adoption, if the initial state is low and in some cases the adoption will never happen. The importance of initial states in technology or new product penetration of the market have been stressed in several other studies (Bass, 1969; Meade and Islam, 2006). In fact, a certain level of engagement and knowledge is required before a new technology or product can be accepted and could influence consumer behavior (Rogers, 2003). This implies that, in the context of our study, farmers will only adopt SWRT if there are initial awareness raising and piloting efforts by either governments or development practitioners.
Increases in Grain Yield
The modeling conducted through the current study suggests that at the regional level, the adoption of SWRT could lead to an increase of 50 million tons in maize grain yields (Figure 5). These increases in maize yields are higher than the regional production levels for the year 2017, which were around 34 million tons2 However, the numbers obtained from the modeling conducted in the current study are under the assumptions of good diffusion and proper use of the technology. In order to reach the 20–50% area with SWRT adoption, the initial state, the propensity and the diffusion rates (or the imitation coefficient) require initial investments. The yield increases associated with SWRT imply that this long-term (>50 years) technology can provide a solution to soil-based poverty by enabling communities to overcome productivity challenges associated with coarse-textured soils. In the case of lower initial states, the diffusion rates above S1 (which was always below 20% of the area with SWRT) would still result in production level increases. However, although the yield increase would not be significant at the regional level, benefits would accure to the individual farmer level as the return on investment period is short, a maximum of four seasons for grain crops (Smucker and Basso, 2014).
Increases in Carbon Sequestration
For carbon sequestration, up to 15 million tons of carbon would be accumulated over 20 years of implementation on the typically low organic matter coarse-textured soils and this would enable countries to advance toward meeting their emission reduction targets under the Paris Climate Agreement. However, it is important to note that coarse-textured soils generally have very low C storage potential due to little or no capacity to bind, and thus protect, added carbon (Elliott et al., 1991). Several studies (Manlay et al., 2002; Venkatapen et al., 2004) have shown that, under tropical conditions, with high organic inputs, the C contents in soils under annual crops could increase to match levels attained under native or perennial conditions. The formation of stable soil aggregates (that physically protect stored organic matter) is influenced by several factors such as mineralogy, texture, land use management as well as the quality and quantity of organic matter inputs, which all interact in rather complex ways even in coarse textured soil (Feller and Beare, 1997). Yet, past soil carbon saturation (Stewart et al., 2007), soils still accumulate carbon in the form of particulate organic matter or polymeric decomposition products (Puget et al., 1995; Carter et al., 2003). The additional non-protected organic matter is highly susceptible to decomposition but can persist for long periods of time due to physical or chemical associations with mineral soils (Berhe and Kleber, 2013) or its quality (Ruffo and Bollero, 2003).
It is also important to take note that for tropical agriculture, the level of C-sequestration from crop residues may be lower because farmers generally transfer maize stover to livestock pens where they use it as supplementary feed during the period when grazing is lean. The resultant livestock dung may then be transferred back to maize fields, as a soil amendment product, through a highly labor intensive process (Rawls et al., 2003). This implies that increases in biomass production from the use of SWRT would benefit the integrated crop-livestock systems that are common in the tropics.
Despite the initial labor intense membrane installations, SWRT results in long-term in-situ carbon inputs that have positive feedback on soil health and water retention, which in turn improves biomass accumulation on marginal soils (Manzoni and Katul, 2014). Concurrently, an increase in the organic matter would improve long-term agronomic yield stability for communities working on coarse-textured soils and thus potentially alleviates food insecurity problems faced in SSA (Vico and Porporato, 2015). This is in agreement with the proposition that better management of water, soils and crops would close the soil-based yield gap observed in SSA (Rockstrom et al., 2010). Kavdir et al. (2014) and Smucker et al. (2016) reported substantial savings of water (ca. 40%) and nutrients (ca. 44%), which would translate into huge economic savings at the regional and global level (Smucker and Basso, 2014). These findings suggest that SWRT will support the implementation of sustainable agriculture, based on high resource-use efficiency and higher economic returns on investments on otherwise low productive soils. While the present study explored the potential of maize production, economic returns from high-value crops will probably be even greater. A potential downside of SWRT is a reduction in the rate of groundwater recharge as water is held in the topsoil where it is also vulnerable to be lost through evaporation if additional measures (e.g., mulching and cover crops) are not put in place (Zheng et al., 2018). The SWRT can also face a potential pitfall of getting damaged by deep plowing operations, if not installed deep enough for best soil water content controls in plant root zone. Furthermore, SWRT can result in surface level waterlogging, which may result in anaerobic conditions in the root zone, which are not favorable for maize growth.
Synergies and Trade-Offs
For the diffusion of a technology to be successful, there is a need to overcome non-technical barriers. The main barrier to adoption would be the high initial financial and labor investment costs of at least USD $0.05 per square meter. The exact price depends upon polymer thickness and costing by the polymer extruding sources. Policies aimed at reducing the cost of the technology, through loans, tax reductions or exemptions or subsidies could incentivize the adoption of SWRT. Creative public-private partnerships whereby the government guarantees the farmer's initial investment and they pay the debt through returns from higher-yielding fields could contribute toward reducing barriers to adoption. Since these mechanisms are not yet present, this could limit the adoption rate and influence the assumptions of reaching the expected increases in grain yield and carbon sequestration.
The long-term nature of this technology makes it an attractive solution for reducing soil-based poverty. Whereas, in the current study we show mostly potential benefits of the technology, testing the technology under SSA conditions will allow the exploration of other technical challenges and environmental impacts under local conditions. Another non-technical challenge is on identifying appropriate mechanisms to promote scaling of the technology (Boldt et al., 2012). Scaling up SWRT may be achieved by integrating it with other water harvest technologies currently in use in the SSA region such as tied contours, basin planting and ridging; resulting in a synergistic effect.
Conclusion
Our study highlighted the potential benefits of SWRT in terms of maize grain production and biomass production leading to carbon sequestration on coarse-textured soils in eight countries of East and Southern Africa. Our results suggest that the adoption of SWRT would result in gradual improvements of soil health and the mitigation of climate change through soil carbon sequestration on soils that typically have low carbon inputs and stocks. Nonetheless, to increase adoption rates there is clearly a need to increase awareness and pilot the technology under SSA conditions. We expect that combining SWRT with other commonly used technologies and promoting its participatory evaluation will increase awareness on the technology's potential benefits amongst local extension services and decision-makers.
Author Contributions
LN, NC, and AS initiated the idea to study the potential of SWRT in SSA and participated in the writing with contributions of ML, RS, SKa, IR, MR, MQ, SKu, FL, AJ, and GN.
Funding
The study was funded by The Swedish Research Council (Vetenskapsrådet), Grant number 2018-05790.
Conflict of Interest Statement
The authors declare that the research was conducted in the absence of any commercial or financial relationships that could be construed as a potential conflict of interest.
Acknowledgments
This work was implemented as part of the activities of SLU Global within the Theme on Climate adaptation for bio-based development and respective authors' institutions. The views expressed in this paper cannot be taken to reflect the official opinions of the organizations. We are also thankful to reviewers who improved the quality of the manuscript.
Supplementary Material
The Supplementary Material for this article can be found online at: https://www.frontiersin.org/articles/10.3389/fsufs.2019.00071/full#supplementary-material
Footnotes
References
Al-Omran, A. M., and Al-Harbi, A. R. (1997). “Improvement of sandy soils with soil conditioners,” in Handbook of Soil Conditioners: Substances That Enhance the Physical Properties of Soil, eds A. Wallace and R. E. Terry (New York, NY: Marcel Dekker), 363–384.
Bacaër, N. (2011). “Verhulst and the logistic equation (1838),” in A Short History of Mathematical Population Dynamics (London: Springer). doi: 10.1007/978-0-85729-115-8_6
Bass, F. M. (1969). A new product growth for model consumer durables. Manage. Sci. 15, 215–227. doi: 10.1287/mnsc.15.5.215
Battaile, C. C., and Srolovitz, D. J. (2002). Kinetic Monte Carlo simulation of chemical vapor deposition. Annu. Rev. Mater. Res. 32, 297–319. doi: 10.1146/annurev.matsci.32.012102.110247
Berhe, A. A., and Kleber, M. (2013). Erosion, deposition, and the persistence of soil organic matter: mechanistic considerations and problems with terminology. Earth Surf. Processes Landforms 38, 908–912. doi: 10.1002/esp.3408
Boldt, J. I., Nygaard, I., Hansen, U. E., and Traerup, S. (2012). Overcoming Barriers to the Transfer and Diffusion of Climate Technologies. Copenhagen: UNEP Riso Center.
Bolinder, M. A., Angers, D. A., Giroux, M., and Laverdiere, M. R. (1999). Estimating C inputs retained as soil organic matter from corn (Zea Mays L.). Plant Soil 215, 85–91. doi: 10.1023/A:1004765024519
Cai, Q., Zhang, Y. L., Sun, Z. X., Zheng, J. M., Bai, W., Zhang, Y., et al. (2017). Morphological plasticity of root growth under mild water stress increases water use efficiency without reducing yield in maize. Biogeosciences 14, 3851–3858. doi: 10.5194/bg-14-3851-2017
Cao, Y., Gillespie, D. T., and Petzold, L. R. (2006). Efficient step size selection for the tau-leaping simulation method. J. Chem. Phys. 124:11. doi: 10.1063/1.2159468
Carter, M. R., Angers, D. A., Gregorich, E. G., and Bolinder, M. A. (2003). Characterizing organic matter retention for surface soils in eastern Canada using density and particle size fractions. Can. J. Soil Sci. 83, 11–23. doi: 10.4141/S01-087
Dewitte, O., Jones, A., Spaargaren, O., Breuning-Madsen, H., Brossard, M., Dampha, A., et al. (2013). Harmonisation of the soil map of Africa at the continental scale. Geoderma 211, 138–153. doi: 10.1016/j.geoderma.2013.07.007
Elliott, E. T., Palm, C. A., Reuss, D. E., and Monz, C. A. (1991). Organic matter contained in soil aggregates from a tropical chronosequence: correction for sand and light fraction. Agric. Ecosyst. Environ. 34, 443–451. doi: 10.1016/0167-8809(91)90127-J
Erickson, A. E., Hansen, C. M., and Smucker, A. J. M. (1968). The influence of subsurface asphalt barriers OH the water properties and the productivity of sand soils. Trans. 9th Int. Congr. Soil Sci. Adelaide 1968 1, 331–337.
Falatah, A. M., Choudhary, M. I., and AL-Omran, A. M. (1996). Changes in some chemical properties of arid soils as affected by synthetic polymers. Arid Soil Res. Rehab. 10, 277–285. doi: 10.1080/15324989609381442
Fang, W., and Giles, M. B. (2019). Multilevel Monte Carlo method for ergodic SDEs without contractivity. J. Math. Anal. Appl. 476, 149–176. doi: 10.1016/j.jmaa.2018.12.032
Food and Agriculture Organization (2018). FAOSTAT Statistical Database. Rome: Food and Agriculture Organization of the United Nations.
Feder, G., Just, R. E., and Zilberman, D. (1982). Adoption of Agricultural Innovation in Developing Countries- A Survey. Washington, DC: World Bank.
Feller, C., and Beare, M. H. (1997). Physical control of soil organic matter dynamics in the tropics. Geoderma 79, 69–116. doi: 10.1016/S0016-7061(97)00039-6
Garba, M., Cornelis, W. M., and Steppe, K. (2011). Effect of termite mound material on the physical properties of sandy soil and on the growth characteristics of tomato (Solanum lycopersicum L.) in semi-arid Niger. Plant Soil 338, 451–466. doi: 10.1007/s11104-010-0558-0
Garrity, D. P., Vejpas, C., and Herrera, W. T. (1992). “Percolation barriers increase and stabilize rainfed lowland rice yields on well-drained soil,” in International Workshop on Soil and Water Engineering for Paddy Field Management, eds V.N. Murphy and K. Koga (Bangkok: Asian Institution of Technology, 413–421.
Gillespie, D. T. (2001). Approximate accelerated stochastic simulation of chemically reacting systems. J. Chem. Phys. 115, 1716–1733. doi: 10.1063/1.1378322
Glab, T., Palmowska, J., Zaleski, T., and Gondek, K. (2016). Effect of biochar application on soil hydrological properties and physical quality of sandy soil. Geoderma 281, 11–20. doi: 10.1016/j.geoderma.2016.06.028
Gordon, M. B., Laguna, M. F., Goncalves, S., and Iglesias, J. R. (2017). Adoption of innovations with contrarian agents and repentance. Phys.Statist. Mech. Appl. 486, 192–205. doi: 10.1016/j.physa.2017.05.066
Grilichies, Z. (1957). Hybrid corn: an exploration in the economics of technological change. Econometrica 25, 501–522. doi: 10.2307/1905380
Guber, A. K., Smucker, A. J. M., Berhanu, S., and Miller, J. M. L. (2015). Subsurface water retention technology improves root zone water storage for corn production on coarse-textured soils. Vadose Zone J. 14:vzj2014.11.0166. doi: 10.2136/vzj2014.11.0166
Gupta, J. P., and Aggarwal, R. K. (1980). Use of an asphalt subsurface barrier for improving the productivity of desert sandy soils. J. Arid Environ. 3, 215–222. doi: 10.1016/S0140-1963(18)31649-5
Gwenzi, W., Chaukura, N., Mukome, F. N. D., Machado, S., and Nyamasoka, B. (2015). Biochar production and applications in sub-Saharan Africa: opportunities, constraints, risks and uncertainties. J. Environ. Manage. 150, 250–261. doi: 10.1016/j.jenvman.2014.11.027
Hartemink, A. E., and Huting, J. (2005). “Sandy soils in southern and eastern africa: extent, properties and management,” in Management of Tropical Sandy Soils for Sustainable Agriculture. A Holistic Approach for Sustainable Development of Problem Soils in the Tropics (Khon Kaen: FAO), 54–59. Avilable online at: http://www.fao.org/3/AG125E00.htm
Hartemink, A. E., and Huting, J. (2008). Land cover, extent, and properties of arenosols in Southern Africa. Arid Land Res. Manage. 22, 134–147. doi: 10.1080/15324980801957689
Hengl, T., Heuvelink, G. B. M., Kempen, B., Leenaars, J. G. B., Walsh, M. G., Shepherd, K. D., et al. (2015). Mapping soil properties of Africa at 250 m resolution: random forests significantly improve current predictions. PLoS ONE 10:e0125814. doi: 10.1371/journal.pone.0125814
Hierbert, D. (1974). Risk, learning and adoption of fertilizer responsive seed varieties. Am. J. Agric. Econ. 56, 764–768. doi: 10.2307/1239305
Ismail, S. M., and Ozawa, K. (2007). Improvement of crop yield, soil moisture distribution and water use efficiency in sandy soils by clay application. Appl. Clay Sci. 37, 81–89. doi: 10.1016/j.clay.2006.12.005
Kavdir, Y., Zhang, W., Basso, B., and Smucker, A. J. M. (2014). Development of a new long-term drought resilient soil water retention technology. J. Soil Water Conserv. 69, 154A−60A. doi: 10.2489/jswc.69.5.154A
Komainda, M., Taube, F., Kluss, C., and Herrmann, A. (2018). The effects of maize (Zea mays L.) hybrid and harvest date on above- and belowground biomass dynamics, forage yield and quality - a trade-off for carbon inputs? Eur. J. Agron. 92, 51–62. doi: 10.1016/j.eja.2017.10.003
Manlay, R. J., Chotte, J. L., Masse, D., Laurent, J. Y., and Feller, C (2002). Carbon, nitrogen and phosphorus allocation in agro-ecosystems of a West African savanna: III. Plant and soil components under continuous cultivation. Agric. Ecosyst. Environ. 88, 249–269. doi: 10.1016/S0167-8809(01)00220-1
Manzoni, S., and Katul, G. (2014). Invariant soil water potential at zero microbial respiration explained by hydrological discontinuity in dry soils. Geophys. Res. Lett. 41, 7151–7158. doi: 10.1002/2014GL061467
McKissock, I., Walker, E. L., Gilkes, R. J., and Carter, D. J. (2000). The influence of clay type on reduction of water repellency by applied clays: a review of some West Australian work. J. Hydrol. 231, 323–332. doi: 10.1016/S0022-1694(00)00204-3
Meade, N., and Islam, T. (2006). Modelling and forecasting the diffusion of innovation - a 25-year review. Int. J. Forecast. 22, 519–545. doi: 10.1016/j.ijforecast.2006.01.005
Pineda-Krch, M. (2008). GillespieSSA: implementing the stochastic simulation algorithm in R. J. Stat. Softw. 25, 1–18. doi: 10.18637/jss.v025.i12
Puget, P., Chenu, C., and Balesdent, J. (1995). Total and young organic matter distributions in aggregates of silty cultivated soils. Eur. J. Soil Sci. 46, 449–459. doi: 10.1111/j.1365-2389.1995.tb01341.x
Rausch, C., Nahangi, M., Haas, C., and Liang, W. H. (2019). Monte Carlo simulation for tolerance analysis in prefabrication and offsite construction. Autom. Construct. 103, 300–314. doi: 10.1016/j.autcon.2019.03.026
Rawls, W. J., Pachepsky, Y. A., Ritchie, J. C., Sobecki, T. M., and Bloodwortht, H. (2003). Effect of soil organic carbon on soil water retention. Geoderma 116, 61–76. doi: 10.1016/S0016-7061(03)00094-6
Rockstrom, J., Karlberg, L., Suhas Wani, P., Barron, J., Hatibu, N., Oweis, T., et al. (2010). Managing water in rainfed agriculture-the need for a paradigm shift. Agric. Water Manage. 97, 543–550. doi: 10.1016/j.agwat.2009.09.009
Ruffo, M. L., and Bollero, G. A. (2003). Modeling rye and hairy vetch residue decomposition as a function of degree days and decomposition days. Agron. J. 95, 900–907 doi: 10.2134/agronj2003.9000
Rui-mel, L. (2015). Properties of Monte Carlo and its application to risk management. Int. J. u e Serv. Sci. Technol. 8, 381–390. doi: 10.14257/ijunesst.2015.8.9.37
Singh, S. K., Pandey, C. B., Sidhu, G. S., Sarkar, D., and Sagar, R. (2011). Concentration and stock of carbon in the soils affected by land uses and climates in the western Himalaya, India. Catena 87, 78–89. doi: 10.1016/j.catena.2011.05.008
Smucker, A. J. M., and Basso, B. (2014). “Global potential for a new subsurface water retention technology- converting marginal soil into sustainable plant production,” in The Soil Underfoot: Infinite Possibilities for a Finite Resource, eds G. J. Churchman and E. R. Landa (New York, NY: CREC Press).
Smucker, A. J. M., Levene, B. C., and Ngouajio, M. (2018). Increasing vegetable production on transformed sand to retain twice the soil water holding capacity in plant root zone. J. Horticult. 5:246. doi: 10.4172/2376-0354.1000246
Smucker, A. J. M., Yang, Z., Guber, A. K., He, X. C., Lai, F. H., and Berhanu, S. (2016). A new revolutionary technology to feed billions by establishing sustainable agriculture on small and large landscapes including urban regions globally. Int. J. Dev. Res. 6, 9596–9602.
Stewart, C. E., Paustian, K., Conant, R. T., Plante, A. F., and Six, J. (2007). Soil carbon saturation: concept, evidence and evaluation. Biogeochemistry 86:19. doi: 10.1007/s10533-007-9140-0
Sultan, F., Farley, J. U., and Lehman, D. R. (1990). A meta-analysis of applications of diffusion models. J. Market. Res. 27, 70–77. doi: 10.1177/002224379002700107
Svendsen, M., Ewing, M., and Msangi, S. (2009). Measuring Irrigation Performance in Africa. Washington, DC: IFPRI.
Togashi, E. (2019). Risk analysis of energy efficiency investments in buildings using the Monte Carlo method. J. Build. Perform. Simul. 12, 504–522. doi: 10.1080/19401493.2018.1523949
Tracheva, N., and Ukhinov, S. (2019). A new Monte Carlo method for estimation of time asymptotic parameters of polarized radiation. Math. Comput. Simul. 161, 84–92. doi: 10.1016/j.matcom.2018.10.001
Vanveen, J. A., and Kuikman, P. J. (1990). Soil structural aspects of decomposition of organic-matter by microorganisms. Biogeochemistry 11, 213–233. doi: 10.1007/BF00004497
Venkatapen, C., Blanchart, E., Bernoux, M., and Burac, M (2004). Déterminants des stocks de carbone dans les sols et spatialisation à l'échelle de la Martinique. Les Cahiers PRAM 4, 35–36. Available online at: http://www.documentation.ird.fr/hor/fdi:010036828
Vico, G., and Porporato, A. (2015). Ecohydrology of agroecosystems: quantitative approaches towards sustainable irrigation. Bull. Math. Biol. 77, 298–318. doi: 10.1007/s11538-014-9988-9
Wenbo, B., Jiqing, S., and Huanzhong, Z. (2013). Repeated water absorbency of superabsorbent polymers in agricultural field application: a simulation study. Acta Agric. Scand. Section B Soil Plant Sci. 63, 433–441. doi: 10.1080/09064710.2013.797488
Wilkinson, D. J. (2006). “Introduction to biological modelling,” in Stochastic Modelling for Systems Biology, eds D. J. Wilkinson and H. Chapamn (Boca Raton, FL: Chapman & Hall/CRC), 1–18.
Zhang, P., Wei, T., Jia, Z. K., Han, Q. F., Ren, X. L., and Li, Y. P. (2014). Effects of straw incorporation on soil organic matter and soil water-stable aggregates content in semiarid regions of Northwest China. PLoS ONE 9:11. doi: 10.1371/journal.pone.0092839
Zheng, J., Fan, J., Zhang, F., Yan, S., Guo, J., Chen, D., et al. (2018). Mulching mode and planting density affect canopy interception loss of rainfall and water use efficiency of dryland maize on the Loess Plateau of China. J. Arid Land 10, 794–808. doi: 10.1007/s40333-018-0122-y
Keywords: technology adoption, water retention membranes, crop production increase, soil organic carbon, smallholder farmers, Sub-Sahara Africa
Citation: Nkurunziza L, Chirinda N, Lana M, Sommer R, Karanja S, Rao I, Romero Sanchez MA, Quintero M, Kuyah S, Lewu F, Joel A, Nyamadzawo G and Smucker A (2019) The Potential Benefits and Trade-Offs of Using Sub-surface Water Retention Technology on Coarse-Textured Soils: Impacts of Water and Nutrient Saving on Maize Production and Soil Carbon Sequestration. Front. Sustain. Food Syst. 3:71. doi: 10.3389/fsufs.2019.00071
Received: 23 January 2019; Accepted: 19 August 2019;
Published: 06 September 2019.
Edited by:
Robert Martin Rees, Scotland's Rural College, United KingdomReviewed by:
Justice Nyamangara, Marondera University of Agricultural Sciences and Technology (MUAST), ZimbabweKristal Jones, University of Maryland, College Park, United States
Copyright © 2019 Nkurunziza, Chirinda, Lana, Sommer, Karanja, Rao, Romero Sanchez, Quintero, Kuyah, Lewu, Joel, Nyamadzawo and Smucker. This is an open-access article distributed under the terms of the Creative Commons Attribution License (CC BY). The use, distribution or reproduction in other forums is permitted, provided the original author(s) and the copyright owner(s) are credited and that the original publication in this journal is cited, in accordance with accepted academic practice. No use, distribution or reproduction is permitted which does not comply with these terms.
*Correspondence: Libère Nkurunziza, bGliZXJlLm5rdXJ1bnppemEmI3gwMDA0MDtzbHUuc2U=
†Present address: Idupulapati Rao, Plant Polymer Research Unit, National Center for Agricultural Utilization Research, Agricultural Research Service, United States Department of Agriculture, Peoria, IL, United States