- 1Department of Food Science and Technology, University of Georgia, Athens, GA, United States
- 2Egg Safety and Quality Research Unit, U.S. National Poultry Research Center, Agricultural Research Service, United States Department of Agriculture, Athens, GA, United States
Predictive models offer food scientists, farmers, and processors tools to help identify variables that lead to an increase in the food safety risk of a product. Foodborne pathogens, such as Listeria spp., pose a major problem for the pastured poultry industry. Currently, there is a lack of understanding of what farm practices lead to higher prevalence of Listeria spp. This study constructed random forest (RF) models to predict the prevalence of Listeria spp. in pastured poultry farming environments and the final broiler product based on major farm practices and variables. Feces, soil, and whole carcass rinse samples were collected from 11 farms in the southeastern United States and evaluated for Listeria spp. presence. The preharvest sample RF model identified the time of year and age of the broiler flock at time of sampling as factors of increased probability of Listeria spp. presence in feces and soil samples. The final product RF model identified brood feed and the presence of chlorine in processing rinse water as the two most important variables associated with an increased likelihood of Listeria spp. presence. Both the preharvest RF model and final sample RF model performed well on a held-out test set, with area under the receiver operating characteristic curve values of 0.876 and 0.887, respectively. The presented models showed the usefulness of RF models in a food safety context. Both RF models will help pastured poultry farmers and processors guide control strategies to manage Listeria contamination in pastured poultry farms and products.
Introduction
Listeria spp. are gram-positive, ubiquitous organisms that have been found in a variety of environments, including agricultural and farming, food processing, and retail environments (Martín et al., 2014; Sasaki et al., 2014; Ahmed et al., 2015). Listeria spp. are hardy organisms that have shown the ability to establish a niche once introduced to an environment, allowing for persistence within that environment (Carpentier and Cerf, 2011). Listeria monocytogenes is a foodborne pathogen that belongs to the Listeria genus that causes listeriosis in humans. The pathogen has been shown to be a significant foodborne risk in the meat and poultry industry, especially with ready-to-eat (RTE) products (Frye et al., 2002; Olsen et al., 2005; Zhu et al., 2005; Gottlieb et al., 2006; Dev Kumar et al., 2016). From 2010–2016, there were 18 multistate foodborne illness outbreaks attributed to L. monocytogenes, resulting in 324 illnesses and 65 deaths (Centers for Disease Control Prevention, 2018).
Although much is known about the impact and prevalence of Salmonella spp. and Campylobacter spp. throughout the poultry supply chain, relatively little is known about the prevalence of Listeria spp. (Rothrock et al., 2017). Studies have shown that the presence of Listeria spp. in the environment often indicates an environment that supports and increases the likelihood of L. monocytogenes presence and survival (Ivanek et al., 2009). Additionally, studies have shown that the occurrence of L. monocytogenes in food products is mainly contributed to cross contamination from processing and retail environments, emphasizing the importance of eliminating the transfer of the organism into a processing facility from the outside environment (Lianou and Sofos, 2007; Ferreira et al., 2014). Listeria can colonize the intestines of poultry and spread into the litter and environment through poultry feces (Njagi et al., 2004; Dhama et al., 2013). This marks a potential entry pathway into a poultry processing plant, if not controlled for. Therefore, it is important for poultry producers and processors to understand what factors are most important in controlling for Listeria spp. prevalence in the environment and postharvest product of pastured poultry farms.
In the recent years, pastured poultry and other similar alternative poultry products have increased in interest in the United States (Hilimire, 2012). Pastured poultry farms are characterized by poultry flocks that are reared in open-air, movable pens (Siemon et al., 2007). Pens are often rotated to fresh pasture to encourage forage intake by the birds (Salatin, 1993). Flock sizes vary, but often contain < 3,000 birds (Elkhoraibi et al., 2017). While broilers reared on these types of farms can cost up to 200% more than traditionally raised broilers, US consumers have shown a willingness to pay more for organic chicken meat (Van Loo et al., 2011; O'Bryan et al., 2017). Compared to conventional broiler farms, research on the food safety of pastured poultry farms is very limited. An understanding on the impact of different types of pastured poultry farm practices on Listeria spp. prevalence in the environment and final broiler product is important to poultry farmers and producers.
The current study evaluated preharvest environmental and processed broiler samples collected from pastured poultry farms from 2014 to 2017 and constructed random forest (RF) models to predict Listeria spp. prevalence based on various farm practice variables, like feed type, egg source, and broiler breed. The presented models and information will be useful for poultry farmers, processors, and risk managers to minimize the risk of Listeria spp. contamination.
Materials and Methods
Farm Sampling Design
A longitudinal study was conducted on 43 flocks of broilers across 11 pastured poultry farms in the southeastern United States from March 2014 to November 2017. All 11 farms reared their broiler flocks in movable pens with temporary fences. A brief description of the size and scale of each farm is contained in Table 1. Data were collected for 40 major farm practice variables (Table 2) over a flock's lifecycle and all samples were evaluated for the presence of Listeria spp.
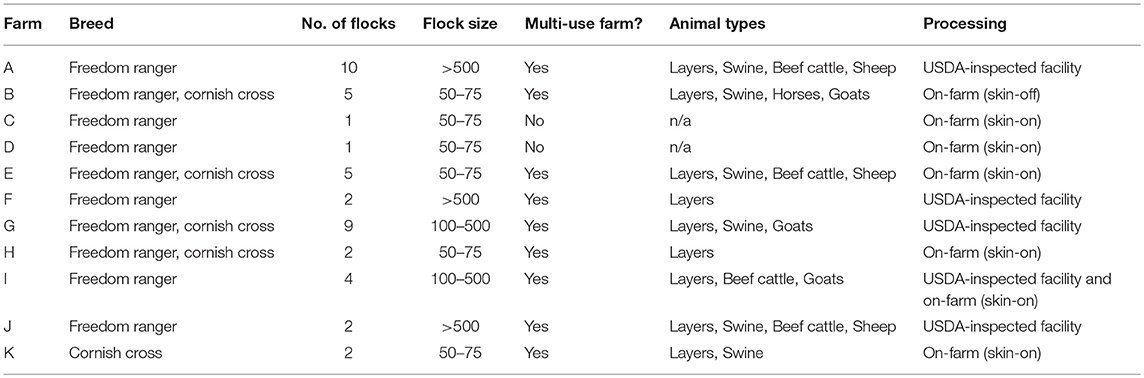
Table 1. Comparison of the 11 all-natural, antibiotic-free, pastured broiler farms included in this study.
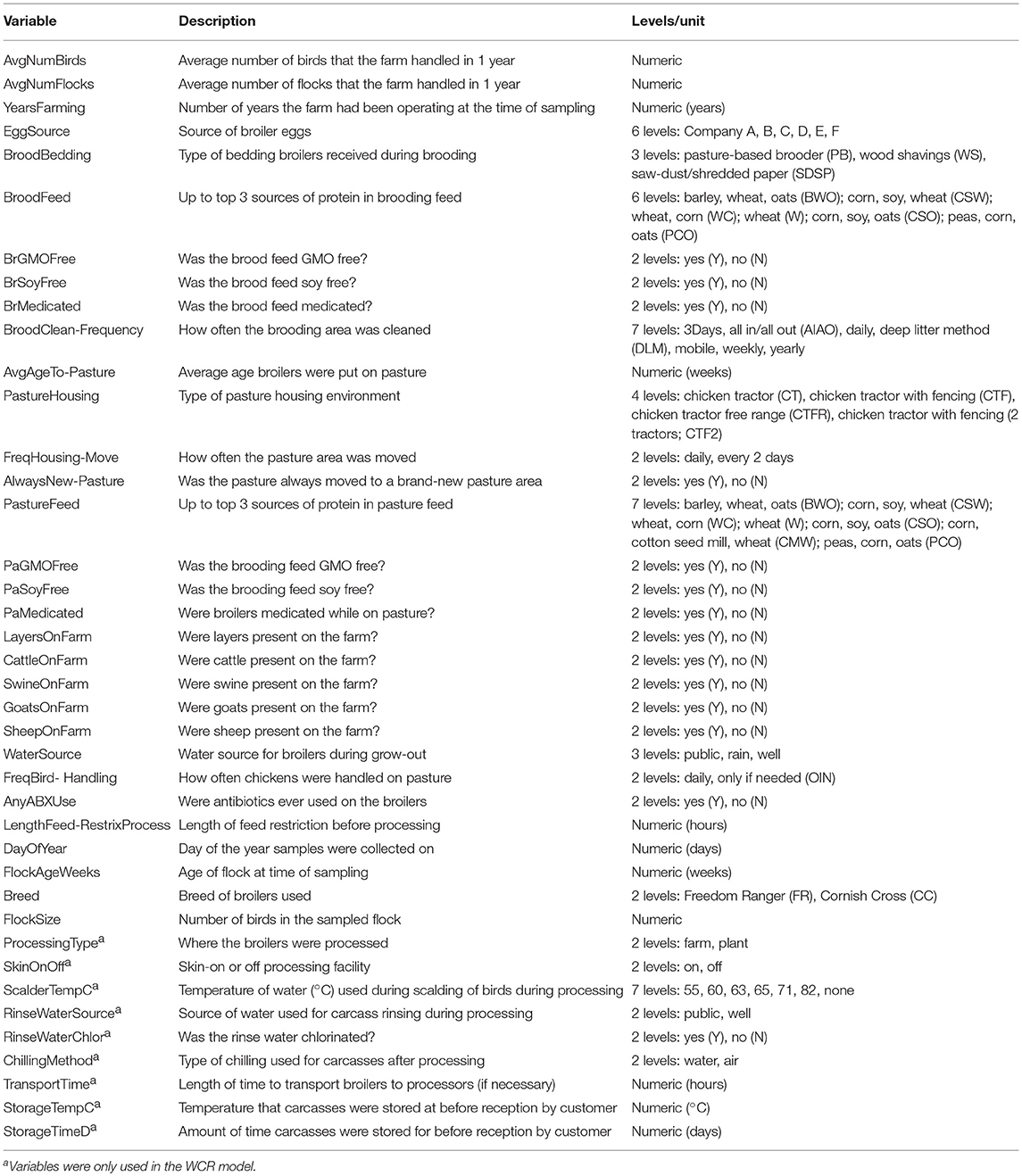
Table 2. Predictors used in the preharvest (feces, soil) and final product whole carcass rinse (WCR) random forest models.
Sample Collection
The following samples were collected along the farm-to-fork continuum for each flock to analyze for the presence of Listeria spp.: (i) feces, (ii) pasture soil, and (iii) whole carcass rinse (WCR) directly after processing from each farm. If a farm was multi-use and contained other types of animals, environmental samples were collected from the area of residence of the other animals as well (Table 1).
Preharvest samples (feces and soil) were taken 3 times throughout a flock's lifecycle: (i) within a few days of being placed on the pasture, (ii) halfway through their time on the pasture, and (iii) on the day in which the flock was processed. Processing samples were only taken when the flock had reached the processing point. In all, there were 1,867 samples from 43 flocks of birds.
On each sampling day, feces, and soil samples were collected from the area in which the flock was residing. Fecal samples were collected from fresh fecal droppings at the sampling site. Soil samples were collected from the surface of the pasture (0–7 cm). Each sample consisted of at least 25 g. Sterile scoops and gloved hands were used to collect each type of environmental sample, with all equipment being changed after each collected sample. During sampling, the pasture area was divided into 5 areas. In each area 5 subsamples were taken and pooled into one sample to account for low expected pathogen population size (Semenov et al., 2008; Bergholz et al., 2011).
To assess the prevalence of Listeria spp. on the final broiler product, 25 carcasses were sampled after processing, packaging, and cold storage of the carcasses according to the practices followed by each farm (Table 3). This step monitors prevalence at the point closest to the carcass being available at the consumer level. Each carcass was placed in an individual sterile sample bag. Carcasses were rinsed with 100 mL of 10 mM phosphate-buffered saline (PBS) and the bags were vigorously shaken. Whole carcass rinses from 5 carcasses were combined into 1 pooled sample, creating 5 pooled samples (n = 25) in total. Carcasses were then returned to the processor to be packed, stored, and distributed in the appropriate fashion for that farm.
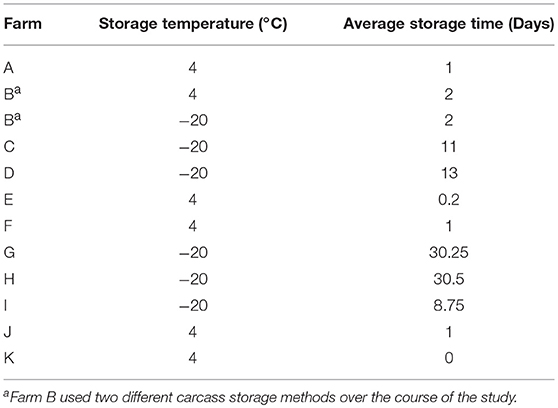
Table 3. Broiler cold storage procedures before making the product available for consumers for each farm.
All fecal, soil, and WCR samples were transferred to a microbiological lab on ice and processed within 2 h of collection. Once to the lab, no further preparation was performed with the WCR samples.
At each farm, the respective farmers managed all of the flocks on farm, and the processing was performed by the farmers or the processing facility workers, not the researchers or the technicians. The preharvest samples were not collected directly from live birds and all postharvest samples were taken post mortem, therefore, no ethics review process was required for the current study.
Listeria spp. Enrichment and Isolation
Listeria spp. enrichment and isolation followed a modified version of the USDA-FSIS MLG 8.10 method (United States Department of Agriculture-Food Safety Inspection Service, 2017). To prepare the feces and soil samples for pre-enrichment, 3 g (feces or soil) was added to a filtered stomacher bag (Seward Laboratory Systems, Inc., Davie, FL) and diluted 1:3 buffered peptone water (BPW; Acumedia, Lansing, MI). Samples were then homogenized for 60 s. All sample bags were then incubated at 35°C overnight. This step acted as a pre-enrichment. Following pre-enrichment, two subsequent primary enrichments were carried out first in University of Vermont Modified Listeria Enrichment Broth (UVM; Remel, Lenexa, KS) incubated at 30°C for 24 h and then into Fraser Broth (FB; Oxoid, Basingstoke, UK) incubated at 30°C for 24. For both, tubes were incubated at 30°C for 24 h. Following primary enrichment, one loopful (~10 μL) of the FB was streaked onto Listeria selective agar (LSA; Oxoid, Basingstoke, UK), and plates were incubated at 30°C overnight. If present, 3 presumptive Listeria colonies were picked from each plate and kept for further analysis.
Listeria spp. Characterization by PCR
Speciation of Listeria was carried out using the procedures described by Huang et al. (2007) and Locatelli et al. (2017). Briefly, two multiplex PCR reactions were conducted, and samples were types as L. innocua, L. welshimeri, L. monocytogenes, L. grayi, or L. ivanovii. If a sample was typed as L. monocytogenes, it underwent further testing to determine the serovar, using the methods described by Doumith et al. (2004). In short, one L. monocytogenes isolate was thoroughly mixed with 25 μL PCR media containing: 1X EconoTaq PLUS 2X Master Mix (Lucigen Corporation, Middleton), 1 μM of each lmo0737, ORF2819, and ORF2110 reverse and forward primers, 1.5 μM of lmo1118 reverse and forward primers, 0.2 μM of prs reverse and forward primers and qs water. Following completion of PCR, products were mixed with 3 μL of BlueJuice™ loading buffer (Invitrogen, Carlsbad, CA) and separated on a 2% E-gel® with SYBR-safe™ (Invitrogen, Carlsbad, CA) along with 12 μL of E-Gel™ 1 kb Plus DNA Ladder (Invitrogen, Carlsbad, CA).
Statistical Analyses
All statistical analyses were performed in R (Version 3.4.0; R Foundation for Statistical Computing, Vienna, Austria). The Fisher's exact test was used to compare Listeria spp. prevalence among different sample types. P < 0.05 were considered statistically significant. The caret package was used for model training and analysis (Kuhn, 2008).
The random forest (RF) model is an ensemble, tree-based, machine learning (ML) model that was introduced by Breiman (2001). This method utilizes a large number of unpruned classification and regression trees (Breiman, 1984) and two sources of randomization during construction (Prasad et al., 2006; Philibert et al., 2011). During construction of each tree, data are randomly chosen using the non-parametric bootstrap sampling method (Efron and Tibshirani, 1994), and trees are constructed on those data. These observations represent the in-bag sample. Trees are then built using the CART method, with a number of randomly selected variables (mtry) chosen at each split to determine the best split according to the Gini index (Breiman, 1984). Each tree's performance is then evaluated on the leftover out-of-bag samples. This step helps to rank the importance of each predictor through the calculation of mean decrease accuracy (Philibert et al., 2011). Finally, all trees are aggregated into a final RF model. For classification problems, the model passes new data through each tree in the forest and counts the number of “votes” for each classification result (Breiman, 2001). The result with the most “votes” represents the final prediction.
Random forest models were built for both the preharvest (feces and soil) and WCR sample data to predict the presence or absence of Listeria spp. based on the predictors presented in Table 2. For the WCR model, all 40 predictors were used, but for the preharvest samples, only non-processing specific predictors were used, since these samples were collected before any processing occurred. In all, there were 1,637 preharvest samples and 230 WCR samples. Each data set was first split into training and testing sets using stratified random sampling to preserve the overall class distribution. The training set contained 80% of observations and the test set contained the remaining 20%. Models were trained on the training data, while the test set was held out to resemble an independent, “real-world” data set to evaluate the performance of the final model.
To choose an appropriate value for mtry, RF models were trained by the training set using various values for mtry and 10-fold cross-validation. From these results, the mtry value with the highest receiver operating characteristic (ROC) statistic was chosen and the model was retrained by the training set with the appropriate mtry value to construct the final RF model. For the preharvest model, an mtry of 53 was used, and for the WCR model, an mtry of 35 was used. Variable importance was determined in each model using the mean decrease in Gini as described by Breiman (2001) and Breiman (2002). Variables were ranked by relative importance on a scale of 0 to 100, where a score of 100 represents the most important variable. Partial dependency plots (PDP) were constructed for the 2 most important variables in each model using the pdp package (Greenwell, 2017).
For the preharvest and WCR data sets, there were far more negative observations than positive observations. To correct for this class imbalance, the synthetic minority over-sampling technique (SMOTE) was used (Chawla et al., 2002). The SMOTE method utilizes a mixture of over-sampling the minority class (positives) and under-sampling the majority class (negatives) to attempt to achieve higher classifier performance. This method was used within the cross-validation step and was only applied to the training set.
After the final models were constructed, each model's performance was evaluated on the held-out test set. Each model was used to predict Listeria spp. prevalence given the predictor data and model predictions were compared to observed values. Models were evaluated using area under the ROC curves (AUC) (Bradley, 1997), sensitivity, and specificity.
Results
The prevalence of Listeria spp. in all samples collected in the current study was 17.4% (Table 4). Out of the 1,867 total samples, only 37 (2.0%) of the samples were positive for L. monocytogenes. Among sample types, there was no significant difference in the presence of Listeria spp. (p = 0.11) or in the prevalence of L. monocytogenes (p = 0.58). The WCR samples had the highest prevalence of both Listeria spp. and L. monocytogenes (22.2 and 2.6%, respectively). The total prevalence of Listeria spp. in preharvest samples was 16.7%.
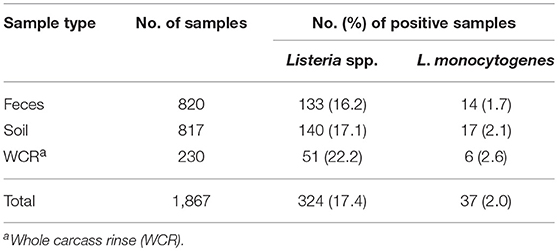
Table 4. Effect of sample type on prevalence of Listeria spp. and Listeria monocytogenes in pastured poultry farm samples.
Farm-by-farm Listeria spp. prevalence results are illustrated in Table 5. For preharvest samples, each farm had at least one Listeria spp. positive sample with prevalence values ranging from 6.7 to 60%. Farm D had the highest percentage of positive samples, with 18 of the 30 samples positive. Of these 18 samples, 17 were positive for L. monocytogenes. For WCR samples, Listeria spp. prevalence values ranged from 0 to 80%, with Farm D having the highest percentage of Listeria spp. positive WCR samples. There were 3 farms that did not have any positive WCR samples.
Random forest models were constructed for preharvest and WCR samples. The variable importance plot containing the top 8 most important predictors as defined by the preharvest RF model is illustrated in Figure 1. The model predicted that day of year was by far the most important variable in predicting Listeria spp. preharvest prevalence of a pastured poultry farm with a relative importance score of 100, compared to 31.0 and 13.8 for flock age at time of sampling and the number of years that a farm has been operating, respectively. Partial dependency plots (PDPs) were constructed for the day of year and flock age variables (Figure 2). Generally, predicted probability of Listeria spp. isolation was highest during generally colder temperature days of the year (days 100–175 and 275–325) and lowest during generally warmer parts of the year (Figure 2A). There is some variability within the plot, but the variable's importance relative to other variables suggest its importance in the model. The RF model also predicted that probability of Listeria spp. preharvest isolation of pastured poultry farms is highest when the flock is between 5 and 10 weeks old and decreases substantially after 10 weeks (Figure 2B).
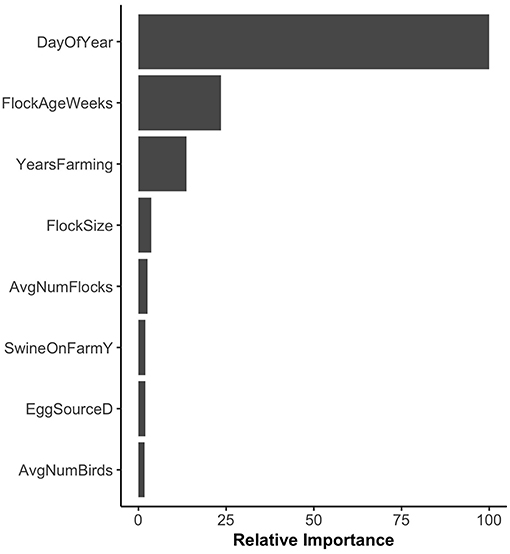
Figure 1. Variable importance plot for the preharvest sample random forest model. Relative importance is determined by average mean decrease in Gini and scaled from 0 to 100, where 100 is most important. SwineOnFarmY refers to farms that had swine present at the farm. EggSourceD refers to farms that received broiler eggs from Company D.
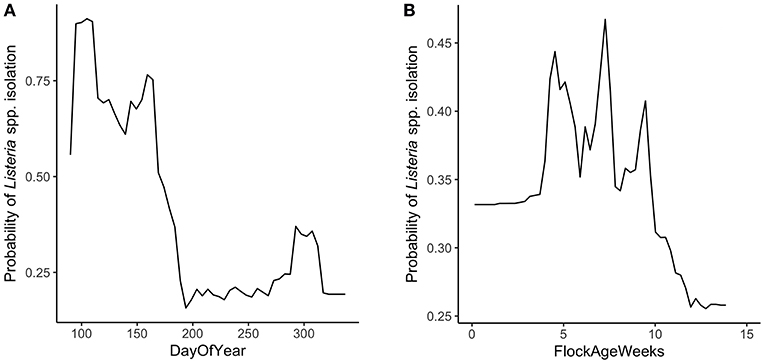
Figure 2. Partial dependency plots (PDPs) for the two most important predictors in the preharvest sample random forest model. (A) DayOfYear represents the day of the year that samples were collected, numerically from 1 to 365. (B) FlockAgeWeeks represents the age of the flock in weeks at the time of sampling.
The variable importance plot for the WCR RF model is shown in Figure 3. The model ranked brood feed whose top three ingredients were corn, soy, and wheat, as the most important predictor of Listeria spp. prevalence on the final broiler product from pastured poultry farms with a relative importance score of 100. The second most important indicator was chlorinated rinse water used during the processing of broilers with a relative importance score of 83.8. No other variable had a relative importance score of over 25. The model predicted that the marginal effect of corn/soy/wheat brood feed on the predicted Listeria spp. outcome was much higher than the other brood feeds (Figure 4A). Similarly, the marginal effect of chlorinated processing rinse water on the predicted final Listeria spp. prevalence outcome was much higher than the other values for that predictor (Figure 4B).
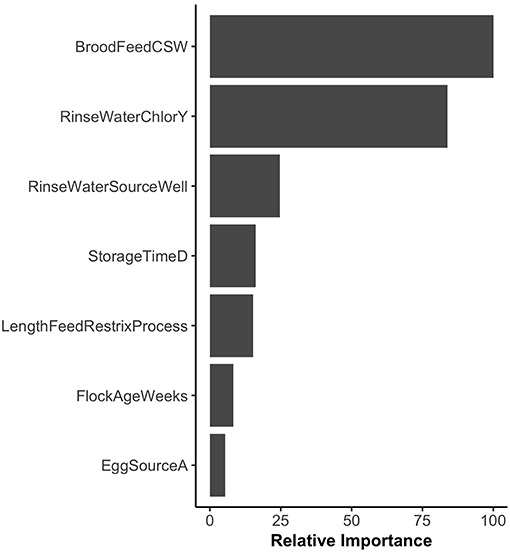
Figure 3. Variable importance plot for the whole carcass rinse (WCR) final product random forest model. Relative importance is determined by average mean decrease in Gini and scaled from 0 to 100, where 100 is most important. BroodFeedCSW refers to brood feed with top 3 sources of protein as corn, soy, and wheat. RinseWaterChlorY refers to farms that chlorinated rinse water used during broiler processing. RinseWaterSourceWell refers to farms that used well water as the source for rinse water used during processing. EggSourceA refers to farms that received broiler eggs from Company A.
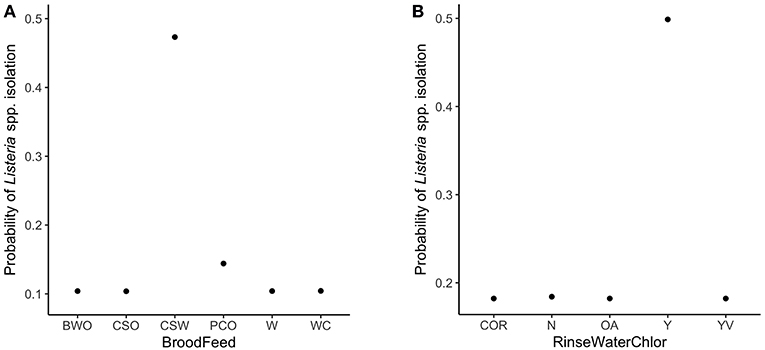
Figure 4. Partial dependency plots (PDPs) for the two most important predictors in the whole carcass rinse (WCR) final product random forest model. (A) Brood feed refers to up to the top 3 sources of protein in the brood feed. Ingredients include: barley (B), corn (C), oats (O), wheat (W), soy (S), cotton seed mill (M), peas (P). (B) RinseWaterChlor refers to whether the rinse water used during broiler processing was chlorinated or not. Levels for this variable include: yes (Y), no (N), organic acids used instead of chlorine (OA), yes with vinegar (YV), certified organic rinse used as wash (COR).
For both models, model performance was evaluated on a held-out test set. This test set was not used in training of the model, and is meant to resemble an independent, “real-world” data set. Confusion matrices for both models were generated to illustrate the comparison of model predictions and observed outcomes (Table 6). It is important to consider that in this context, false negatives are much more costly than false positives, as having a model that incorrectly misses positive contamination results can be more harmful than one that incorrectly predicts contamination. Thus, model sensitivity is of vast importance. The preharvest RF model had a sensitivity of 0.778 and a specificity of 0.846. Of the 326 model predictions, there were only 12 false negatives, but the model incorrectly predicted a positive result 42 times, indicating that the model appears to be fail-safe. To further illustrate these results, ROC curves and area under the ROC curve (AUC) were used (Figure 5). According to this statistic, the preharvest RF model performed exceptionally with an AUC of 0.876. The WCR model had a sensitivity of 0.800 and specificity of 0.886. Only 2 of the 45 test samples were false negatives. Additionally, the WCR RF model performed very well according to the ROC curve, with an AUC of 0.887 (Table 6).
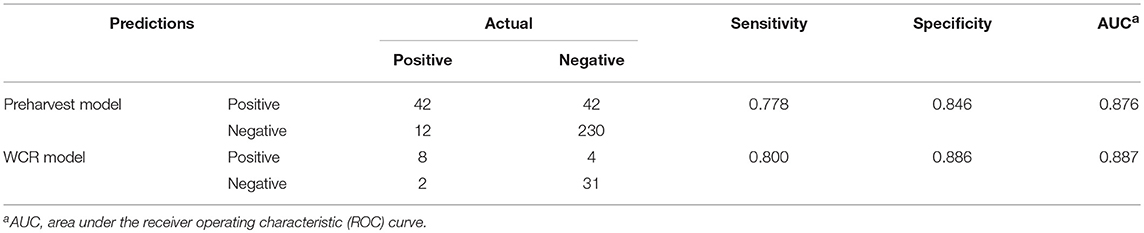
Table 6. Predictive performance of the preharvest and whole carcass rinse (WCR) random forest models.
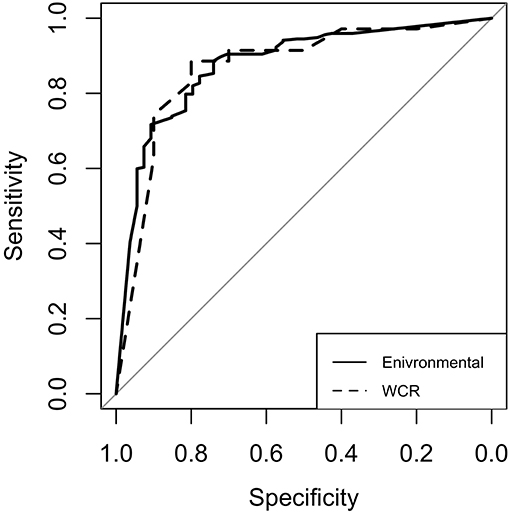
Figure 5. Receiver operating characteristic (ROC) curves for the environmental (solid line) and whole carcass rinse (dashed line) random forest models. The diagonal line represents the line of no discrimination, or the expectation of a random guess. Curves above this line are considered better than random guess. The area under each curve is referred to as AUC.
Discussion
Currently, there is a lack of understanding on the impact and prevalence of Listeria spp. in pastured poultry farming environments and products (Rothrock et al., 2017). The current study aimed to construct accurate predictive models that can be used to predict the presence or absence of Listeria spp. in the preharvest pastured poultry farm environment and in the final broiler product and examine the prevalence of the pathogen across 11 southeastern United States farms. Doing so would help identify major farm management variables (Table 2) that are associated with a higher risk of Listeria spp. presence, as predicted by the models.
The Risk of Listeria to Pastured Poultry Farmers
While there have been no known chicken-related listeriosis outbreaks in the United States, there have been several foodborne illness outbreaks due to Listeria monocytogenes contamination of deli turkey and other types of RTE meats (Olsen et al., 2005). This signals its potential significance in the chicken and RTE chicken product industry. It is important to control for the pathogen within a farming environment, because favorable Listeria spp. environments are indicative of conditions that increase the risk of Listeria monocytogenes (Ivanek et al., 2009). This means that an environment that is contaminated with Listeria could be a source of contamination for downstream processing areas (Ivanek et al., 2006). Furthermore, poultry can act as reservoirs for the organism (Njagi et al., 2004; Dhama et al., 2013). Therefore, it is important for farmers and processors to be aware of farm management practices that can lead to an increased risk of Listeria spp. contamination in the environment of pastured poultry farms and in the final broiler product.
Weather and Flock Age Are Likely to Impact the Presence of Listeria spp. in the Environment of Pastured Poultry Farms
The RF model generated from preharvest sample data identified the day of the year that the sample was collected as the most important variable in classifying a sample as positive or negative for the presence of Listeria spp. While specific weather data were not collected for each sampling day in this study, the RF model predicted that days at the early and late parts of the year were associated with a higher probability of Listeria spp. isolation in the environment (Figure 2A). We only sampled from day 90 to day 350 of the year that did not include the winter season, and thus, our model does not extrapolate the results for the days corresponding to this season. Days 100–175 showed exceptionally high predicted risk. These days of the year are associated with the spring season. It was previously found that there was a higher prevalence of L. monocytogenes in processing environments during spring and winter months (Guerini et al., 2007). It follows that if there is a higher probability of Listeria isolation in the environment during cold weather, there is a higher probability of Listeria being brought into a processing facility during these times.
The increased probability of Listeria isolation during cold weather months could be due to the fact that the pathogen is more resistant to lower temperatures. For example, Listeria are able to survive at temperatures as low as 1°C, whereas enteric foodborne pathogens are not (Doyle, 1989). Strawn et al. (2013) found that L. monocytogenes had a higher prevalence in above freezing, cooler temperatures in the environment of fruit and vegetable farms. Further data need to be collected to analyze the effect of temperature on Listeria prevalence in pastured poultry farms.
It is important to consider that the presented preharvest RF model may be region specific, as data were collected only from southeastern pastured poultry farms. It is most likely that the day of year variable is important due to weather patterns that are occurring during those times. Because weather patterns over the course of a year are region specific, this model might not be appropriate for all regions. Despite this, the presented RF model suggests that farmers and processors have a higher awareness of Listeria during colder, above freezing weather.
Flock age was the second most important variable in the preharvest RF model (Figure 1). Listeria spp. isolation from the environment was predicted to have the highest probability when a flock was between 5 and 10 weeks old. As a flock increases in age, changes occur in the flock's gut microbiota, and it has been found that Listeria spp. levels decrease in the intestine of poultry as the bird's intestinal microbiota develops (Milillo et al., 2012).
Farm Management Practices Can Impact Listeria spp. Prevalence in the Final Broiler Product
The presented final WCR RF model identified brood feed as the most important explanatory variable in predicting Listeria spp. presence in the final product (Figure 3). Flocks whose brood feed's 3 main sources of protein were corn, soy, and wheat had a significantly higher probability than the other 5 types of feed (Table 2). After hatching, the intestinal microbiota of a chicken develops rapidly (Lan et al., 2005; Chambers and Gong, 2011). As such, the type of feed that a chick receives early on its life can have a profound effect on its gut microbiota (Lan et al., 2005). This is an especially important point to consider for pastured poultry and organic farms that utilize feeds with very few additives. If present in the external environment, Listeria could contaminate a flock's food source, and be present throughout a bird's lifespan. It is important to note of the possibility that type of feed could just be important to our model. Rather, our model identified it as an important predictor of Listeria spp. presence, but not necessarily the cause of contamination.
The use of chlorinated rinse water during broiler processing was identified as the second most important variable in the final broiler product RF model (Figure 3). Studies have shown that gram-positive organisms, such as Listeria monocytogenes, are more resistant to chlorine than gram-negative bacteria (Virto et al., 2005). Thus, it is possible that chlorine is more effective at reducing gram-negative bacteria during rinsing of broilers during processing, and this might create a less competitive environment for Listeria to survive and grow during cold storage. Organic acids, on the other hand, have been shown to be more effective against gram-positive bacteria (Skrivanová et al., 2006). Further research needs to be conducted to see if the use of chlorinated rinse water during poultry processing leads to a more favorable environment for Listeria.
Our model did not identify major pastured poultry farm management practices such as type of processing unit and skin on/skin off processing as important predictors of Listeria spp. presence. While no studies have examined the effect of these practices on Listeria prevalence, there have been conflicting results on the effect of these variables on other foodborne pathogens. Trimble et al. (2013) found that Salmonella prevalence was significantly higher on carcasses processed on-farm compared to carcasses processed at a USDA-inspected facility, while there were no significant differences in Campylobacter prevalence. For traditionally-reared broilers, Berrang et al. (2001) found that skin-on and skin-off broiler parts had no significant difference in Campylobacter and Escherichia coli numbers. Currently, there are no data indicating the difference of foodborne pathogen prevalence in skin-on and skin-off broilers reared on pastured poultry farms.
Random Forest Model Performance
Machine learning models have been shown to have use in the food safety industry, with several studies being published on the performance of random forest models (Barco et al., 2012; Gu et al., 2015; Pang et al., 2017) and classification and regression tree models (Mokhtari et al., 2006; Ivanek et al., 2009; Strawn et al., 2013) in a food safety context. Benefits to using RF models is that they are robust to outliers and skewed data, provide variable importance rankings, and compute an unbiased out-of-bag error estimate (Rodriguez-Galiano et al., 2012).
Machine learning models are often tested on how they perform at classifying independent, new observations that were not used during training of the model. In many biological and research settings, it can be too costly to obtain a new testing set, so data sets are split into training and testing sets (Dupuy and Simon, 2007). Models are then evaluated by prediction performance on the testing set, and evaluated by metrics such as ROC curves and AUC (Bradley, 1997). In the current study, both RF models performed well with respect to AUC, with both scoring >0.85 (Table 6).
It is important to note that the sample size for the final product WCR model was small, which might have impacted the prediction performance estimation. It would be of great use to obtain a new, independent data set from other pastured poultry farms to confirm the usefulness of the generated RF model. The small sample size shouldn't have a large effect on the variable importance rankings, though (Figure 3). Strobl and Zeileis (2008) reported that importance measures from models trained on smaller data sets were not significantly different from those trained on larger data sets.
Conclusions
Random forest models were generated to classify pastured poultry preharvest and final product samples as positive or negative for Listeria spp. Our model identified time of year as a potential indicator of preharvest presence of Listeria spp. on pastured poultry farms. Additionally, corn/soy/wheat brood feed and rinse water chlorination were associated with a higher probability of Listeria spp. isolation on the final product WCR, as predicted by our model. Due to the variation in the types of pastured poultry farms sampled from Table 1, the information provided by our models could be representative of many different types of pastured poultry and similar organic type farms, although a greater geographic diversity of farms is needed to test this hypothesis. This study showed the use of RF models at predicting pathogen presence and should assist farmers and processors be aware of factors that are associated with a higher risk of Listeria contamination on pastured poultry farms.
Data Availability
The datasets generated for this study are available on request to the corresponding author.
Author Contributions
CG performed all statistical analyses and developed the predictive models. MR performed the data collection and organization. CG drafted the manuscript. MR and AM provided project oversight and assistance in manuscript preparation. All authors approved of the final manuscript draft.
Funding
These investigations were supported by the Agricultural Research Service, USDA CRIS Projects Genetic Analysis of Poultry-Associated Salmonella enterica to Identify and Characterize Properties and Markers Associated with Egg-Borne Transmission of Illness #6040-32000-007-00 and Molecular Approaches for the Characterization of Foodborne Pathogens in Poultry #6612-32000-059-00.
Conflict of Interest Statement
The authors declare that the research was conducted in the absence of any commercial or financial relationships that could be construed as a potential conflict of interest.
The handling editor declared a shared affiliation, though no other collaboration, with one of the authors, MR, at time of review.
Acknowledgments
We would like to thank Laura Lee Rutherford, Cheryl Gresham-Pearson, Tori McIntosh, and Aude Locatelli for assistance in sample acquisition, pathogen isolation, and Listeria speciation/subtyping.
References
Ahmed, O. M., Pangloli, P., Hwang, C.-A., Zivanovic, S., Wu, T., D'Souza, D., et al. (2015). The occurrence of Listeria monocytogenes in retail ready-to-eat meat and poultry products related to the levels of acetate and lactate in the products. Food Control 52, 43–48. doi: 10.1016/j.foodcont.2014.12.015
Barco, L., Mancin, M., Ruffa, M., Saccardin, C., Minorello, C., Zavagnin, P., et al. (2012). Application of the random forest method to analyse epidemiological and phenotypic characteristics of Salmonella and Salmonella Typhimurium strains. Zoonoses Public Health 59, 505–512. doi: 10.1111/j.1863-2378.2012.01487.x
Bergholz, P. W., Noar, J. D., and Buckley, D. H. (2011). Environmental patterns are imposed on the population structure of Escherichia coli after fecal deposition. Appl. Environ. Microbiol. 77, 211–219. doi: 10.1128/AEM.01880-10
Berrang, M. E., Ladely, S. R., and Buhr, R. J. (2001). Presence and level of Campylobacter, coliforms, Escherichia coli, and total aerobic bacteria recovered from broiler parts with and without skin. J. Food Prot. 64, 184–188. doi: 10.4315/0362-028X-64.2.184
Bradley, A. P. (1997). The use of the area under the ROC curve in the evaluation of machine learning algorithms. Pattern Recognit. 30, 1145–1159. doi: 10.1016/S0031-3203(96)00142-2
Carpentier, B., and Cerf, O. (2011). Persistence of Listeria monocytogenes in food industry equipment and premises. Int. J. Food Microbiol. 145, 1–8. doi: 10.1016/j.ijfoodmicro.2011.01.005
Centers for Disease Control Prevention (2018). Outbreak of Listeria Infections Linked to deli ham. Avaliable online at: https://www.cdc.gov/listeria/outbreaks/countryham-10-18/index.html (Accessed November 11, 2018).
Chambers, J. R., and Gong, J. (2011). The intestinal microbiota and its modulation for Salmonella control in chickens. Food Res. Int. 44, 3149–3159. doi: 10.1016/j.foodres.2011.08.017
Chawla, N. V., Bowyer, K. W., Hall, L. O., and Kegelmeyer, W. P. (2002). SMOTE: synthetic minority over-sampling technique. 16, 321–357. doi: 10.1613/jair.953
Dev Kumar, G., Williams, R. C., Sumner, S. S., and Eifert, J. D. (2016). Effect of ozone and ultraviolet light on Listeria monocytogenes populations in fresh and spent chill brines. Food Control 59, 172–177. doi: 10.1016/j.foodcont.2015.04.037
Dhama, K., Verma, A. K., Rajagunalan, S., Kumar, A., Tiwari, R., Chakraborty, S., et al. (2013). Listeria monocytogenes infection in poultry and its public health importance with special reference to food borne zoonoses. Pak. J. Biol. Sci. 16, 301–308. doi: 10.3923/pjbs.2013.301.308
Doumith, M., Buchrieser, C., Glaser, P., Jacquet, C., and Martin, P. (2004). Differentiation of the major Listeria monocytogenes serovars by multiplex PCR. J. Clin. Microbiol. 42, 3819–3822. doi: 10.1128/JCM.42.8.3819-3822.2004
Dupuy, A., and Simon, R. M. (2007). Critical review of published microarray studies for cancer outcome and guidelines on statistical analysis and reporting. J. Natl. Cancer Inst. 99, 147–157. doi: 10.1093/jnci/djk018
Efron, B., and Tibshirani, R. J. (1994). An Introduction to the Bootstrap. Boca Raton, FL: CRC press.
Elkhoraibi, C., Pitesky, M., Dailey, N., and Niemeier, D. (2017). Operational challenges and opportunities in pastured poultry operations in the United States. Poult. Sci. 96, 1648–1650. doi: 10.3382/ps/pew448
Ferreira, V., Wiedmann, M., Teixeira, P., and Stasiewicz, M. J. (2014). Listeria monocytogenes persistence in food-associated environments: epidemiology, strain characteristics, and implications for public health. J. Food Prot. 77, 150–170. doi: 10.4315/0362-028X.JFP-13-150
Frye, D. M., Zweig, R., Sturgeon, J., Tormey, M., LeCavalier, M., Lee, I., et al. (2002). An outbreak of febrile gastroenteritis associated with delicatessen meat contaminated with Listeria monocytogenes. Clini. Infect. Dis. 35, 943–949. doi: 10.1086/342582
Gottlieb, S. L., Newbern, E. C., Griffin, P. M., Graves, L. M., Hoekstra, R. M., Baker, N. L., et al. (2006). Multistate outbreak of listeriosis linked to turkey deli meat and subsequent changes in US regulatory policy. Clini. Infect. Dis. 42, 29–36. doi: 10.1086/498113
Greenwell, B. M. (2017). pdp: an R package for constructing partial dependence plots. R J. 9, 421–436. doi: 10.32614/RJ-2017-016
Gu, W., Vieira, A. R., Hoekstra, R. M., Griffin, P. M., and Cole, D. (2015). Use of random forest to estimate population attributable fractions from a case-control study of Salmonella enterica serotype Enteritidis infections. Epidemiol. Infect. 143, 2786–2794. doi: 10.1017/S095026881500014X
Guerini, M. N., Brichta-Harhay, D. M., Shackelford, S. D., Arthur, T. M., Bosilevac, J. M., Kalchayanand, N., et al. (2007). Listeria prevalence and Listeria monocytogenes serovar diversity at cull cow and bull processing plants in the United States. J. Food Prot. 70, 2578–2582. doi: 10.4315/0362-028X-70.11.2578
Hilimire, K. (2012). The grass is greener: farmers' experiences with pastured poultry. Renew. Agricul. Food Syst. 27, 173–179. doi: 10.1017/S1742170511000287
Huang, B., Eglezos, S., Heron, B. A., Smith, H., Graham, T., Bates, J., et al. (2007). Comparison of multiplex PCR with conventional biochemical methods for the identification of Listeria spp. isolates from food and clinical samples in Queensland, Australia. J. Food Protect. 70, 1874–1880. doi: 10.4315/0362-028X-70.8.1874
Ivanek, R., Gröhn, Y., Wells, M., Lembo, A., Sauders, B., and Wiedmann, M. (2009). Modeling of spatially referenced environmental and meteorological factors influencing the probability of Listeria species isolation from natural environments. Appl. Environ. Microbiol. 75, 5893–5909. doi: 10.1128/AEM.02757-08
Ivanek, R., Gröhn, Y. T., and Wiedmann, M. (2006). Listeria monocytogenes in multiple habitats and host populations: review of available data for mathematical modeling. Foodbourne Pathog. Dis. 3, 319–336. doi: 10.1089/fpd.2006.3.319
Kuhn, M. (2008). Building predictive models in R using the caret package. J. Stat. Softw. 28, 1–26. doi: 10.18637/jss.v028.i05
Lan, Y., Verstegen, M. W. A., Tamminga, S., and Williams, B. A. (2005). The role of the commensal gut microbial community in broiler chickens. World's Poultry Sci. J. 61, 95–104. doi: 10.1079/WPS200445
Lianou, A., and Sofos, J. N. (2007). A review of the incidence and transmission of Listeria monocytogenes in ready-to-eat products in retail and food service environments. J. Food Prot. 70, 2172–2198. doi: 10.4315/0362-028X-70.9.2172
Locatelli, A., Lewis, M. A., and Rothrock, M. J. Jr. (2017). The distribution of Listeria in pasture-raised broiler farm soils is potentially related to university of Vermont medium enrichment bias toward Listeria innocua over Listeria monocytogenes. Front. Vet. Sci. 4:227. doi: 10.3389/fvets.2017.00227
Martín, B., Perich, A., Gómez, D., Yangüela, J., Rodríguez, A., Garriga, M., et al. (2014). Diversity and distribution of Listeria monocytogenes in meat processing plants. Food Microbiol. 44, 119–127. doi: 10.1016/j.fm.2014.05.014
Milillo, S. R., Stout, J. C., Hanning, I. B., Clement, A., Fortes, E. D., Den Bakker, H. C., et al. (2012). Listeria monocytogenes and hemolytic Listeria innocua in poultry. Poult. Sci. 91, 2158–2163. doi: 10.3382/ps.2012-02292
Mokhtari, A., Frey, H. C., and Jaykus, L.-A. (2006). Application of classification and regression trees for sensitivity analysis of the Escherichia coli O157: H7 food safety process risk model. J. Food Prot. 69, 609–618. doi: 10.4315/0362-028X-69.3.609
Njagi, L. W., Mbuthia, P. G., Bebora, L. C., Nyaga, P. N., Minga, U., and Olsen, J. E. (2004). Carrier status for Listeria monocytogenes and other Listeria species in free range farm and market healthy indigenous chickens and ducks. East Afr. Med. J. 81, 529–533. doi: 10.4314/eamj.v81i10.9236
O'Bryan, C. A., Crandall, P., Jaroni, D., Ricke, S. C., and Gibson, K. E. (2017). Assessment of nitrogen and phosphorus loads present in environments impacted by alternative poultry processing operations utilized in pasture-raised poultry production. Renew. Agricult. Food Syst. 32, 33–42. doi: 10.1017/S1742170515000514
Olsen, S. J., Patrick, M., Hunter, S. B., Reddy, V., Kornstein, L., MacKenzie, W. R., et al. (2005). Multistate outbreak of Listeria monocytogenes infection linked to delicatessen turkey meat. Clini. Infect. Dis. 40, 962–967. doi: 10.1086/428575
Pang, H., McEgan, R., Mishra, A., Micallef, S. A., and Pradhan, A. K. (2017). Identifying and modeling meteorological risk factors associated with pre-harvest contamination of Listeria species in a mixed produce and dairy farm. Food Res. Int. 102, 355–363. doi: 10.1016/j.foodres.2017.09.029
Philibert, A., Desprez-Loustau, M.-L., Fabre, B., Frey, P., Halkett, F., Husson, C., et al. (2011). Predicting invasion success of forest pathogenic fungi from species traits. J. Appl. Ecol. 48, 1381–1390. doi: 10.1111/j.1365-2664.2011.02039.x
Prasad, A. M., Iverson, L. R., and Liaw, A. (2006). Newer classification and regression tree techniques: bagging and random forests for ecological prediction. Ecosystems. 9, 181–199. doi: 10.1007/s10021-005-0054-1
Rodriguez-Galiano, V. F., Ghimire, B., Rogan, J., Chica-Olmo, M., and Rigol-Sanchez, J. P. (2012). An assessment of the effectiveness of a random forest classifier for land-cover classification. ISPRS J. Photogramm. Remote Sens. 67, 93–104. doi: 10.1016/j.isprsjprs.2011.11.002
Rothrock, M. J Jr, Davis, M. L., Locatelli, A., Bodie, A., McIntosh, T. G., Donaldson, J. R., et al. (2017). Listeria occurrence in poultry flocks: detection and potential implications. Front. Vet. Sci. 4:125. doi: 10.3389/fvets.2017.00125
Sasaki, Y., Haruna, M., Murakami, M., Hayashida, M., Takahashi, N., Urushiyama, T., et al. (2014). Contamination of poultry products with Listeria monocytogenes at poultry processing plants. J. Vet. Med. Sci. 76, 129–132. doi: 10.1292/jvms.13-0267
Semenov, A. V., Franz, E., van Overbeek, L., Termorshuizen, A. J., and van Bruggen, A. H. C. (2008). Estimating the stability of Escherichia coli O157:H7 survival in manure-amended soils with different management histories. Environ. Microbiol. 10, 1450–1459. doi: 10.1111/j.1462-2920.2007.01558.x
Siemon, C. E., Bahnson, P. B., and Gebreyes, W. A. (2007). Comparative investigation of prevalence and antimicrobial resistance of Salmonella between pasture and conventionally reared poultry. Avian Dis. 51, 112–117. doi: 10.1637/0005-2086(2007)051[0112:CIOPAA]2.0.CO;2
Skrivanová, E., Marounek, M., Benda, V., and Brezina, P. (2006). Susceptibility of Escherichia coli, Salmonella sp. and Clostridium perfringens to organic acids and monolaurin. Vet. Med. 51, 81–88.
Strawn, L. K., Fortes, E. D., Bihn, E. A., Nightingale, K. K., Gröhn, Y. T., Worobo, R. W., et al. (2013). Landscape and meteorological factors affecting prevalence of three food-borne pathogens in fruit and vegetable farms. Appl. Environ. Microbiol. 79, 588–600. doi: 10.1128/AEM.02491-12
Strobl, C., and Zeileis, A. (2008). “Danger: high power!—exploring the statistical properties of a test for random forest variable importance,” in COMPSTAT 2008: Proceedings in Computational Statistics, ed P. Brito (Heidelberg: Physica Verlag). p. 59–66.
Trimble, L. M., Alali, W. Q., Gibson, K. E., Ricke, S. C., Crandall, P., Jaroni, D., et al. (2013). Salmonella and Campylobacter prevalence and concentration on pasture-raised broilers processed on-farm, in a Mobile Processing Unit, and at small USDA-inspected facilities. Food Control 34, 177–182. doi: 10.1016/j.foodcont.2013.04.024
United States Department of Agriculture-Food Safety Inspection Service (2017). Isolation and Identification of Listeria monocytogenes from Red Meat, Poultry, Ready-To-Eat Siluriformes (Fish) and Egg Products, and Environmental Samples. Avaliable online at: https://www.fsis.usda.gov/wps/wcm/connect/1710bee8-76b9-4e6c-92fc-fdc290dbfa92/mlg-8.pdf?MOD=AJPERES (Accessed August 6, 2018).
Van Loo, E. J., Caputo, V., Nayga, R. M. Jr., Meullenet, J.-F., and Ricke, S. C. (2011). Consumers' willingness to pay for organic chicken breast: evidence from choice experiment. Food Qual. Prefer. 22, 603–613. doi: 10.1016/j.foodqual.2011.02.003
Virto, R., Manas, P., Alvarez, I., Condon, S., and Raso, J. (2005). Membrane damage and microbial inactivation by chlorine in the absence and presence of a chlorine-demanding substrate. Appl. Environ. Microbiol. 71, 5022–5028. doi: 10.1128/AEM.71.9.5022-5028.2005
Keywords: Listeria spp., food safety, predictive microbiology, random forest, alternative poultry production, pastured poultry, machine learning
Citation: Golden CE, Rothrock MJ Jr and Mishra A (2019) Using Farm Practice Variables as Predictors of Listeria spp. Prevalence in Pastured Poultry Farms. Front. Sustain. Food Syst. 3:15. doi: 10.3389/fsufs.2019.00015
Received: 12 December 2018; Accepted: 28 February 2019;
Published: 22 March 2019.
Edited by:
Joshua B. Gurtler, Agricultural Research Service, United States Department of Agriculture, United StatesReviewed by:
Cangliang Shen, West Virginia University, United StatesGregory Ross Siragusa, Eurofins, United States
Copyright © 2019 Golden, Rothrock and Mishra. This is an open-access article distributed under the terms of the Creative Commons Attribution License (CC BY). The use, distribution or reproduction in other forums is permitted, provided the original author(s) and the copyright owner(s) are credited and that the original publication in this journal is cited, in accordance with accepted academic practice. No use, distribution or reproduction is permitted which does not comply with these terms.
*Correspondence: Abhinav Mishra, YW1pc2hyYUB1Z2EuZWR1