BRIEF RESEARCH REPORT
Published on 05 Dec 2019
Quantitative Visualization of Hypoxia and Proliferation Gradients Within Histological Tissue Sections
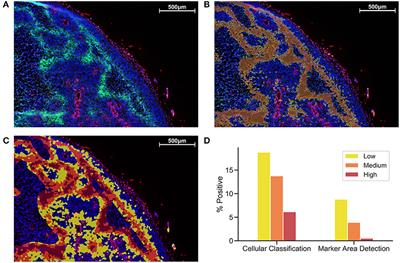
doi 10.3389/fbioe.2019.00397
- 10,220 views
- 37 citations
30k
Total downloads
273k
Total views and downloads
Select the journal/section where you want your idea to be submitted:
BRIEF RESEARCH REPORT
Published on 05 Dec 2019
ORIGINAL RESEARCH
Published on 01 Nov 2019
ORIGINAL RESEARCH
Published on 01 Oct 2019
ORIGINAL RESEARCH
Published on 01 Oct 2019
REVIEW
Published on 01 Oct 2019
ORIGINAL RESEARCH
Published on 23 Aug 2019
METHODS
Published on 16 Jul 2019
ORIGINAL RESEARCH
Published on 05 Jul 2019
ORIGINAL RESEARCH
Published on 21 Jun 2019
ORIGINAL RESEARCH
Published on 15 May 2019
ORIGINAL RESEARCH
Published on 02 Apr 2019
ORIGINAL RESEARCH
Published on 27 Mar 2019
Frontiers in Genetics
Frontiers in Medicine