ORIGINAL RESEARCH
Published on 22 Jul 2024
Sparseness-constrained nonnegative tensor factorization for detecting topics at different time scales
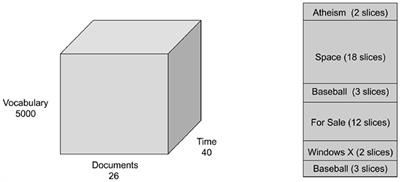
doi 10.3389/fams.2024.1287074
- 525 views
473
Total downloads
2,534
Total views and downloads
Select the journal/section where you want your idea to be submitted:
ORIGINAL RESEARCH
Published on 22 Jul 2024
ORIGINAL RESEARCH
Published on 17 Apr 2024
Frontiers in Big Data
Frontiers in Signal Processing
Manuscripts can be submitted to this Research Topic via the main journal or any other participating journal.
Select the journal/section where you want your idea to be submitted: