ORIGINAL RESEARCH
Published on 06 Dec 2024
Inversion and analysis of transparency changes in the eastern coastal waters of China from 2003 to 2023 by an improved QAA-based method
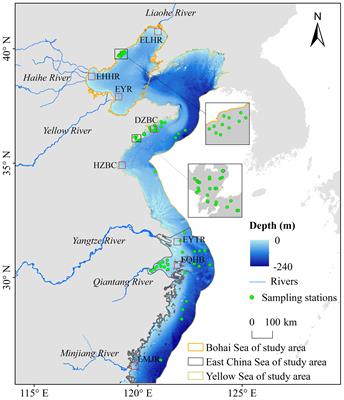
doi 10.3389/fmars.2024.1503177
- 428 views
381
Total downloads
3,389
Total views and downloads
You will be redirected to our submission process.
ORIGINAL RESEARCH
Published on 06 Dec 2024
ORIGINAL RESEARCH
Published on 25 Nov 2024
ORIGINAL RESEARCH
Published on 10 Oct 2024
Manuscripts can be submitted to this Research Topic via the main journal or any other participating journal.
You will be redirected to our submission process.