Published in
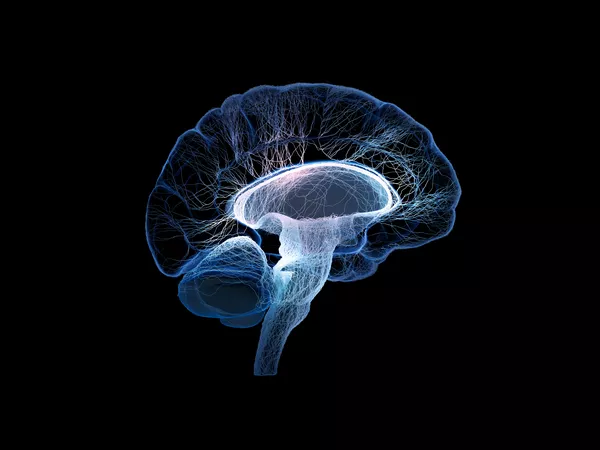
Frontiers in Neuroscience
Translational Neuroscience
754
Total views and downloads
Submit your idea
Select the journal/section where you want your idea to be submitted:
The Research Topic has been launched recently. Articles will be published on this page as soon as they become available.
Manuscripts can be submitted to this Research Topic via the main journal or any other participating journal.
Submit your idea
Select the journal/section where you want your idea to be submitted: