METHODS
Published on 08 Mar 2024
A network-based method for associating genes with autism spectrum disorder
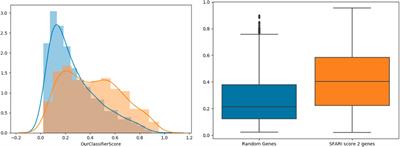
doi 10.3389/fbinf.2024.1295600
- 965 views
1,348
Total downloads
6,290
Total views and downloads
You will be redirected to our submission process.
METHODS
Published on 08 Mar 2024
MINI REVIEW
Published on 13 Jul 2023
Manuscripts can be submitted to this Research Topic via the main journal or any other participating journal.
You will be redirected to our submission process.