ORIGINAL RESEARCH
Published on 22 Mar 2023
Personalized diversification of complementary recommendations with user preference in online grocery
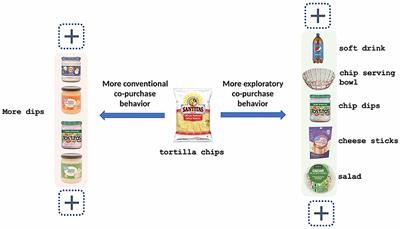
doi 10.3389/fdata.2023.974072
- 2,016 views
2,787
Total downloads
15k
Total views and downloads
You will be redirected to our submission process.
ORIGINAL RESEARCH
Published on 22 Mar 2023
ORIGINAL RESEARCH
Published on 26 Sep 2022
ORIGINAL RESEARCH
Published on 18 Jul 2022
ORIGINAL RESEARCH
Published on 09 Jun 2022
ORIGINAL RESEARCH
Published on 25 Apr 2022