ORIGINAL RESEARCH
Published on 11 Oct 2022
Hemodynamics-driven mathematical model of third heart sound generation
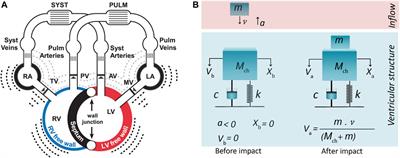
doi 10.3389/fphys.2022.847164
- 2,489 views
- 1 citation
2,480
Total downloads
11k
Total views and downloads
You will be redirected to our submission process.
ORIGINAL RESEARCH
Published on 11 Oct 2022
ORIGINAL RESEARCH
Published on 03 Jan 2022
ORIGINAL RESEARCH
Published on 11 Nov 2021
ORIGINAL RESEARCH
Published on 24 Nov 2020