- Science News
- Featured news
- Finding magnetic eruptions in space, with an AI assistant
Finding magnetic eruptions in space, with an AI assistant
By Miles Hatfield |NASA Goddard Space Flight Center, Greenbelt, MD, USA
An alert pops up in your email: The latest spacecraft observations are ready. You now have 24 hours to scour 84 hours-worth of data, selecting the most promising split-second moments you can find. The data points you choose, depending on how you rank them, will download from the spacecraft in the highest possible resolution; researchers may spend months analyzing them. Everything else will be overwritten like it was never collected at all.
These are the stakes facing the Scientist in the Loop, one of the most important roles on the Magnetospheric Multiscale, or MMS, mission team. Seventy-three volunteers share the responsibility, working weeklong shifts at a time to ensure the very best data makes it to the ground. It takes a keen and meticulous eye, which is why it's always been left to a carefully-trained human – at least until now.
A paper published today describes the first artificial intelligence algorithm to lend the Scientist in the Loop a (virtual) hand.
“MMS is the first big NASA mission implementing machine learning into its mission operations,” said Matthew Argall, space physicist at the University of New Hampshire and lead author of the paper.
The algorithm performs a single task: detecting when the spacecraft crossed from Earth’s magnetic field to the Sun's, or vice versa. But it's just the first of many special-purpose algorithms that could change how MMS science is done.
MMS SITL Ground Loop: Automating the Burst Data Selection Process► Read original article► Download original article (pdf)
Bursting Earth’s Bubble
An invisible force field surrounds our planet, a giant bubble ballooning more than 40,000 miles into space. This is our magnetic field, and it serves us in several ways. It keeps things out, deflecting harmful cosmic rays that would otherwise strike Earth's surface, endangering life. But it also keeps things in, setting traffic patterns for the particles buzzing through near-Earth space. Electrons, tiny and light, turn tight pirouettes around Earth’s magnetic field lines; heavier ions plod along in slower, wider loops.
But Earth’s magnetic field is nothing compared to the Sun’s. Particles blown away from the Sun, known as the solar wind, carry our star’s magnetic field far past the orbit of Neptune. The particles within it trace out the Sun’s magnetic field lines, colliding with Earth’s magnetic bubble along the way. The collision sites form an invisible boundary that scientists call the magnetopause.
Credits: NASA Goddard/Mary Pat Hrybyk-Keith; NASA Goddard’s Conceptual Image Lab/Josh Masters/Joy Ng
By and large, the magnetopause holds strong – but not always. When conditions are right and magnetic fields align, the solar wind can puncture our magnetic bubble. The site of the breach is known as an electron diffusion region, or EDR, and finding them is the MMS mission’s primary goal.
Within an EDR, the Sun's and Earth's magnetic field lines fuse, cancel each other, and vanish. Electrons, energized and unbound, zip to and fro in a chaotic pandemonium.
“It’s like they lost their lane lines while somebody stomped on their accelerators,” said Barbara Giles, senior project scientist for MMS.
These particle bursts trigger a chain reaction that sparks the northern and southern lights – they can even endanger astronauts and spacecraft in their way. EDRs erupt throughout the universe, from the middle of solar flares to the rims of black holes. MMS searches for them closer to home, at the edge of Earth's magnetic field.
But catching one in the act is exceedingly difficult. EDRs appear without notice, extend as little as two miles across (within a 14 billion mile-wide search space), and last only tenths of a second. In five years of continuous search, MMS has measured just over 50. But each time it crosses the magnetopause, where our magnetic field meets the Sun's, it has another chance to see one.
Hunting for Magnetopause Crossings
So the Scientist in the Loop sifts through each orbit's data, hunting for magnetopause crossings. But they don’t necessarily stand out in the data – identifying them is more like pinpointing when a drizzle turns to rain. A single orbit's data may contain as few as two or as many as 100 magnetopause crossings, with false alarm look-a-likes peppered in between. To find them, the Scientist in the Loop simply has to put in the time.
“In the earliest days, it was basically a full-time job,” said Rick Wilder, space physicist at the Laboratory for Atmospheric and Space Physics in Boulder, Colorado. Since then, Wilder has helped optimize the Scientist in the Loop’s workflow and trained new recruits into seasoned experts. Today, an experienced Scientist in the Loop only needs a few hours a week. But it’s still a strain on researchers who volunteer on top of busy schedules. “Fatigue is always in the back of our mind,” Wilder said.
They had always planned to automate parts of the Scientist in the Loop’s role, but finding an algorithm to match human performance was a challenge. Scientists can see larger trends in data, something most algorithms struggle to do. “Part of what a scientist does is look at the progression in time of the data,” said Argall. “For instance, being able to identify that you’re in the magnetosphere at one point, and using that to influence how [you see] the data evolving.”
Argall and his collaborators built an algorithm that tries to emulate how humans read data. It takes the form of a neural network, a data-processing technique inspired by the brain. Unlike traditional algorithms, neural networks program themselves through trial and error. Argall showed the network examples of magnetopause crossings, then tested it on new cases. If it answered wrong – a non-crossing was chosen, or a true crossing was missed – he sent an error signal, triggering a cascade of adjustments before the next test. Like human Scientists in the Loop, the network learned to identify magnetopause crossings from experience.
But most neural networks process data in isolated snapshots, whereas scientists see measurements unfolding in time. The team approximated the scientist’s ability by using gates to store the data the network just saw as well as the data coming up next. As the network decides if it’s looking at magnetopause crossing or not, it can access surrounding data points to help. “The algorithm adds input data from the past and future to provide context for the decision it’s making at the present time,” Argall said.
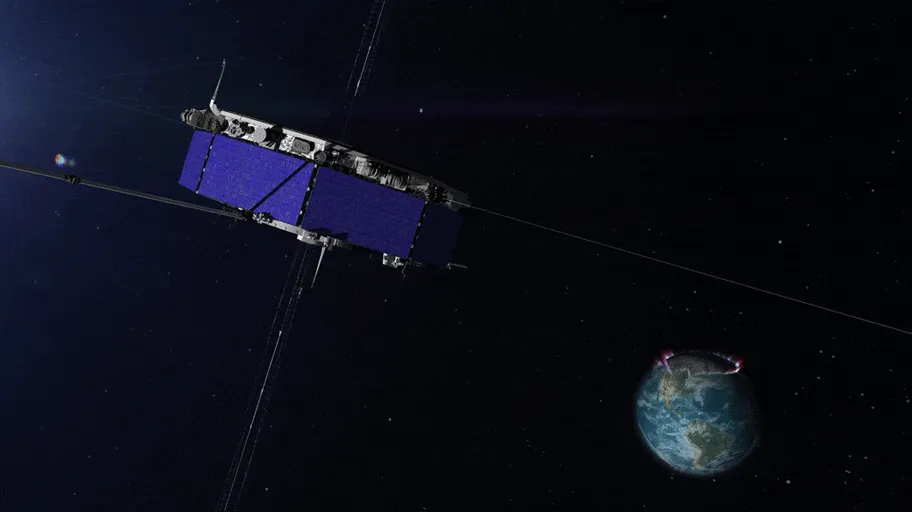
Animation showing the four MMS spacecraft in space.Credits: NASA's Goddard Space Flight Center Conceptual Image Lab/Walt Feimer/Genna Duberstein
It's the first algorithm of what may be many. The team imagines building several special-purpose detectors to work together in a hierarchy. (An assemblage of specialists, others have found, outperforms one jack-of-all trades algorithm.) At the lowest level, "region classifiers" look at the data to figure out where the spacecraft is in space. They pass their output to region-specific "event classifiers,” which look for the phenomena researchers want to find. With success over the next few years, MMS could automatically detect far more than magnetopause crossings.
“We could take requests, say for a certain signature in the data, and bring it down in real time," Giles said. "It becomes a system observatory in that sense – a community resource.”
That's still a ways off. The new algorithm currently matches human judgments about 70% of the time. (Even scientists don’t agree with each other 100% of the time.) Since October 2019, each week’s Scientist in the Loop has treated it like an assistant, double-checking their work and catching any mistakes.
“But I'm sure within a few more years, with these techniques that he's developing, he's going to make the Scientist in the Loop redundant,” Giles said. “We will know when that day comes, because all they will do is go in, check a box, and move on.”
With a trusty algorithmic assistant by their side, scientists could focus on those wiggles in the data they don't yet know how to label. We may be glimpsing a future where algorithms are less tools than collaborators, working alongside scientists as both learn from new data together.
Original article: MMS SITL Ground Loop: Automating the Burst Data Selection Process