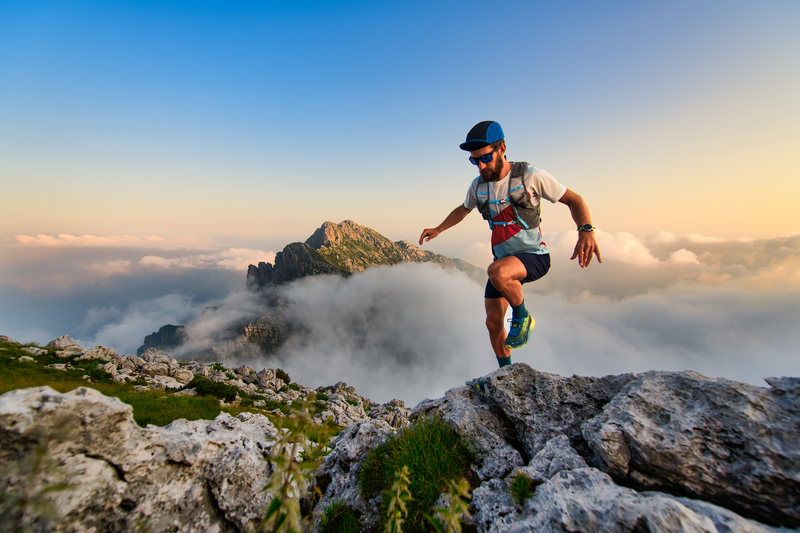
95% of researchers rate our articles as excellent or good
Learn more about the work of our research integrity team to safeguard the quality of each article we publish.
Find out more
SYSTEMATIC REVIEW article
Front. Water , 12 March 2025
Sec. Water Resource Management
Volume 7 - 2025 | https://doi.org/10.3389/frwa.2025.1537868
Climate change is increasingly affecting the water cycle, with droughts and floods posing significant challenges for agriculture, hydropower production, and urban water resource management due to growing variability in the factors influencing the water cycle. Reinforcement learning (RL) has demonstrated promising potential in optimization and planning tasks, as it trains models on historical data or through simulations, allowing them to generate new data by interacting with the simulator. This systematic literature review examines the application of reinforcement learning (RL) in water resource management across various domains. A total of 40 articles were analyzed, revealing that RL is a viable approach for this field due to its capability to learn and optimize sequential decision-making processes. The results show that RL agents are primarily trained in simulated environments rather than directly on historical data. Among the algorithms, deep Q-networks are the most commonly employed. Future research should address the challenges of bridging the gap between simulation and real-world applications and focus on improving the explainability of the decision-making process. Future studies need to address the challenges of bridging the gap between simulation and real-world applications. Furthermore, future research should focus on the explainability behind the decision-making process of the agent, which is important due to the safety-critical nature of the application.
• Climate change affects water systems, and RL provides solutions for resource management.
• This research uses a systematic literature review to explore RL in water resource applications.
• RL models are mainly trained in simulated environments.
• Model-based RL could enhance planning by predicting future states in water resource management.
Water resource management involves managing and regulating the use of scarce water resources, such as managing reservoir water levels amid uncertain inflows. Climate change significantly affects water resources and the water cycle (Ciampittiello et al., 2024). Rising evapotranspiration, more frequent droughts and floods, shifts in the timing of spring floods due to snowmelt (Ciampittiello et al., 2024; IEA, 2021), and changing precipitation patterns are among the key consequences (Kahaduwa and Rajapakse, 2021). These changes in the water cycle are expected to impact multiple sectors, including hydropower (IEA, 2021; IPCC, 2023), agriculture (IPCC, 2023), urban water resource management, and overall water flow dynamics (IPCC, 2023; Li et al., 2023).
In wastewater treatment, climate change is expected to have an effect due to increased uncertainty in precipitation patterns, resulting in reservoirs becoming overburdened when a large amount of rain occurs, resulting in untreated water discharge, but also increased energy use in the treatment of the larger volume of water (Li et al., 2023). Similarly, urban drainage systems are also expected to be at risk of failure to manage increased bodies of water with increased precipitation, resulting in disruption in transportation systems, flood damage, and increased risk to people’s health and safety (Kourtis and Tsihrintzis, 2021). Due to the rising uncertainty in the water cycle caused by climate change, effective water resource management is essential.
In the field of machine learning (ML), there are three paradigms: supervised learning, unsupervised learning, and reinforcement learning (RL) (Bishop, 2006). In datasets that consist of a corresponding target for each input, the task falls under the supervised learning paradigm (Bishop, 2006). Deep learning (DL) is a set of supervised learning methods capable of learning a representation from raw data required to solve a prediction task (LeCun et al., 2015). In unsupervised learning, the target is missing for each input. Instead, unsupervised learning can be used to find groupings in the input data, such as cluster analysis (Bishop, 2006). RL is the third paradigm in ML, where an agent, acting as the decision-maker, learns which actions to take in an environment by selecting those that yield the highest rewards from the environment (Sutton and Barto, 2018; Bishop, 2006).
By allowing the agent to interact and train within the environment, it learns a policy, which is a strategy that tells the agent which decision to make given the current circumstances in the environment (Sutton and Barto, 2018). RL, combined with the representation learning capacity of DL, had a breakthrough in video games, namely the Atari games, in which the agent learned to reach human-level performance (Mnih et al., 2015; Mnih et al., 2013). Another breakthrough with RL combined with DL won over the world champion in the board game Go (Silver et al., 2016). Other examples of RL applications are in transportation (Haydari and Yılmaz, 2020), autonomous driving (Kiran et al., 2021), and power and energy systems (Cao et al., 2020).
Previous literature reviews explored the application of ML and DL in hydrological processes (Croll et al., 2023; Sit et al., 2020; Ahmed et al., 2024; Tripathy and Mishra, 2023; Krechowicz et al., 2022; Villeneuve et al., 2023; Bernardes et al., 2022; Zhu et al., 2022; Ortiz-Lopez et al., 2022; Mohammed et al., 2022); however, few have focused on the application of RL across multiple domains of water resource management. Croll et al. (2023) investigated the potential applications of RL in wastewater treatment. Ortiz-Lopez et al. (2022) and Zhu et al. (2022) have analyzed how ML can be used for water quality prediction. Mohammed et al. (2022) reviewed how ML can be used to predict water levels in watersheds. Multiple reviews perform a wider comparison by investigating how ML has been applied in multiple topics in hydrology and water resource management (Tripathy and Mishra, 2023; Sit et al., 2020; Ahmed et al., 2024). Previous literature reviews have addressed ML applications in hydropower planning (Bernardes et al., 2022; Krechowicz et al., 2022).
Water resource management is a sequential decision-making task and shares common challenges, such as uncertainty in reservoir inflow, managing weather conditions, and planning water levels. By allowing an RL agent to train on various climate scenarios to manage water, the possibilities for efficient water management using ML and RL in unpredictable and changing weather conditions caused by climate change need to be further explored. Therefore, a thorough investigation of how RL has been applied in water resource management allows for a comparison of how the aforementioned challenges are treated.
Although previous review articles have explored the potential of ML in topics related to water resource management, a review specifically focused on the application of RL in this field is still lacking. Water resource management can be classified as a sequential decision task, where previous decisions will affect future decisions. Therefore, careful consideration must be taken before making such decisions. Moreover, RL algorithms are designed in various ways, affecting the training and convergence of policies and resulting in different sequential decision-making. Therefore, it is important to assess the current status of algorithm selection and how the agents are trained in relation to water resource management. The article aims to examine how RL has been applied across various domains of water resource management, with objectives such as minimizing the energy use of water pumps and managing water level constraints. This systematic review aims to answer the following research questions (RQs):
• RQ1: What are the most common RL algorithms applied in water resource management and in each specific domain?
• RQ2: What is the most common method for training an agent across various domains?
The research contributions of this study are twofold. First, it provides a comprehensive analysis of how RL has been applied across various domains of water resource management, examining the choice of algorithms, training and evaluation methods, and the handling of constraints such as water level management. Second, it offers a comparative review of approaches across these domains to identify gaps and potential areas for improvement. The findings of this study provide insights into how RL can be utilized for water resource management in the presence of uncertainties across multiple variables affecting the water cycle. This study provides a thorough analysis of how RL is applied in water resource management by examining, for example, the choice of algorithm and how the agent is trained, which are important topics not addressed in previously published articles. This research is valuable for various stakeholders, including urban planners, energy companies, and agricultural enterprises seeking more efficient water resource management. Furthermore, the study contributes to one of the United Nations’ Sustainable Development Goals, namely the sixth goal, the clean water and sanitation goal, by supporting efforts to ensure access to clean water, improve sanitation, and optimize the efficient use of water resources (United Nations, 2022).
Chapter 2 introduces the general theory of RL, describes a sample of algorithms commonly found in this systematic literature review (SLR), and outlines general approaches for training RL models and selecting hyperparameters. Chapter 3 describes the methodology and details how the systematic review was conducted. Chapter 4 presents the results and analysis, where the selected articles are investigated and discussed. Chapter 5 discusses the results presented in Chapter 4, and Chapter 6 provides the conclusions and suggests directions for future research.
This section presents a general theory of RL, common algorithms found in the SLR, training the agents, and challenges with hyperparameter selection and how it affects the performance of the algorithms.
RL is learning what to do given the state of nature in a defined environment (Sutton and Barto, 2018). Following the notation and definitions presented by Sutton and Barto (2018), an agent is a decision-maker who interacts with the environment. Through the interaction, the agent will receive rewards from the environment, which the agent aims to maximize. At time point , the agent will receive a description of the environment, which is called the state and possible states of the environment create the state space . Given , the agent decides upon an action , which is the action space, to interact with the environment and receive the reward (Sutton and Barto, 2018).
To provide an example related to water resource management. In urban drainage systems (further discussed in section 4.4), RL is a method used to manage water levels in the systems. The states can be water levels in a water tank and water inflows; the action could be how to run the pumps; and finally, the reward can be related to the energy used when running the pumps, which should be as small as possible.
A finite Markov Decision Process (MDP) is one where the sets of states, actions, and rewards are finite. The objective of the agent is to maximize the expected discounted reward presented in Equation 1,
where is the discount factor and the number of steps from until the end of an episode. A policy provides a mapping from the state to the probabilities of each possible action that the agent can perform in the given state. The value function of a state provides the expected return when the agent begins in a state and then proceeds to follow the policy. In Equation 2, let the observed state be denoted as . Then, the value function is defined as follows:
A policy is better than or equal if the value function for all states. An optimal policy is a policy that has a better value function than all other found policies in the MDP (Sutton and Barto, 2018).
RL algorithms can further be classified into categories depending on how the policy is derived: value-based and policy-based learning. Value-based RL algorithms find the value function for a given state and then derive the policy by selecting the action that returns the highest value of the value function. Value-based RL algorithms only work for discrete action spaces because they select the action that provides the highest return of the value function. In contrast, policy-based learning learns a parameterized policy using, for example, a neural network that predicts which action to select given the state. Furthermore, policy-based methods work for both continuous and discrete action spaces (Plaat, 2022).
In single-objective RL, the reward signal is a scalar value, whereas, in multi-objective RL, the reward is a vector which provides a signal on each objective given the action made by the agent in a state (Hayes et al., 2022). Furthermore, in multi-objective RL, there are multiple optimal value functions, whereas there is only a single optimal value function as defined in Equation 2, which can be used to identify the optimal policy in single-objective RL. A utility function provides a mapping of and is the user’s preference regarding each objective and is central in determining whether a solution is optimal or not. An example of a utility function is a linear utility function where the weighted sum of all objectives equals one (Hayes et al., 2022).
The action value function, defined in Equation 3, is the expected return when an agent has observed a state , selects an action and then proceeds to follow the policy (Sutton and Barto, 2018). The Q-learning algorithm utilizes the action value function.
which defines the expected return from taking an action in any given state and then proceeds to follow the policy (Sutton and Barto, 2018). The Q-learning algorithm aims to find the optimal action-value function in each state by updating in Equation 4
in each observed state. To ensure that the action value functions are all updated, a method called epsilon greedy is used, which balances the challenge of exploration or exploitation (i.e., use the learned policy to receive the most expected reward or attempt other actions that may result in more reward). The epsilon greedy strategy states that in each observed state, pick a random action with probability or exploit the policy with probability (Sutton and Barto, 2018). Based on the updating rule in Equation 4, an optimal policy can be derived by choosing the action in each state that maximizes (Sutton and Barto, 2018).
The success of DL (LeCun et al., 2015) has enabled complicated control tasks in RL research with large action and state spaces to be solved (Mnih et al., 2015; Mnih et al., 2013). Mnih et al. (2013) introduced Deep Q-networks (DQN), which combine Q-learning with DL and have shown impressive results in Atari games. In DQN, DL is used to parameterize the action value function, which predicts the Q-value for each action in each state. The network is trained by minimizing the loss function in Equation 5:
by sampling minibatches from a replay buffer, which stores previous environmental transitions. is the action in the minibatch. In Equation 6, , is the target and is defined as calculated as follows:
A challenge in minimizing the objective function presented in Equation 5 is the moving target value . Mnih et al. (2015) used a separate neural network, called a target network, to predict the target in which the weights of the target network are infrequently updated.
Continuous improvements have been made with DQN to improve performance and training stability. Dueling networks predict a state value function and the advantage in a state, which improves policy evaluation in states where actions are similarly valued (Wang et al., 2016). Van Hasselt et al. (2016) introduced double DQN (DDQN) to address challenges with overestimation of the Q-value under certain conditions by introducing an action selection and action evaluation network to minimize the overestimation that occurs in the max operator in Equation 6. Originally, uniform sampling from the replay buffer, in which each previous transition has an equal probability of being included in the minibatch, was utilized for DQN (Mnih et al., 2013; Mnih et al., 2015). Prioritized sampling, which samples states in which most training can be achieved (Schaul, 2015). Finally, Hessel et al. (2018) found that combining techniques that improve DQN outperformed DQN or the single improvements (such as dueling networks or DDQN) on many common benchmark environments used in RL research.
Proximal policy optimization (PPO) was introduced to combat multiple challenges, such as implementing algorithms to combat challenges with hyperparameters and training stability (Schulman et al., 2017). PPO uses a clipped objective function to minimize the risk of moving too far away from a good policy in the previous iteration of training the actor-network. One loss function in PPO utilizes a clipped loss defined in Equation 7:
where is the ratio between the old policy in the previous iteration and the new updated policy, is a truncated version of generalized advantage estimation. In addition to the clipped loss, Schulman et al. (2017) proposed an additional loss using KL-divergence [see Bishop (2006) for a definition of KL-divergence] for training the agent, as defined in Equation 8. The penalty based on the KL-divergence uses the same ratio as in Equation 7 but adds an additional term to prevent deviation from the old policy and is defined as follows:
Deep deterministic policy gradient (DDPG) aimed to address the challenges that DQN has in tasks with continuous action spaces. DQN requires the action space to be discrete, and one method is to discretize the action space. However, this may result in a large space for action, resulting in difficulties in exploration and, thus, the training of the agent (Lillicrap, 2015). DDPG utilizes an actor-network, which is the parameterized policy, and a critic network, which estimates the action value function. Similarly to the original DQN presented in Sec 2.5, the target networks are infrequently updated. However, Lillicrap (2015) introduced a soft update where are the weights in the online network, and are the weights in the target network.
The loss functions in DDPG minimize the squared loss for the critic network in Equation 9 and for the policy utilizing the sampled policy gradient in Equation 10. The critic loss is defined as
where are the weights of the critic network. The sampled policy gradient is defined as
Training the behavior of RL agents can be achieved through trial-and-error interaction with the environment (Sutton and Barto, 2018). Based on the response from the environment, the agent will adapt and learn a better policy based on the feedback. However, depending on the task, directly interacting with the environment could be expensive and result in dangerous outcomes (Salvato et al., 2021). Learning the policy can be achieved in multiple ways. For example, creating a simulation of the environment and letting the agent interact with the simulation is one alternative, and then transferring the learned policy into the real environment. Transfer learning involves learning policies in a set of source environments, which are later used to learn an optimal policy in the target environment (Zhu et al., 2023).
Kober et al. (2013) addressed the challenges of using RL in robotics and stated that allowing the agent to train in the real environment can be beneficial if the task is difficult to simulate correctly. However, real environment training is often more costly due to the need for human supervision to reset the elements of the environment when the robot finishes or fails to solve the task. Furthermore, potential damages to the hardware can be very costly if the robot fails to solve the task. Simulation training solves these problems and can easily generate samples to train the agent to solve the task.
In the context of learning policy in a simulator, sim-to-real transfer can be interpreted as a form of transfer learning in which the policy learned in the simulation is applied and tuned in a real-world environment. However, a challenge in training a simulation remains, and that is called the reality gap. If the simulator incorrectly describes the real world, the agent may learn how to interact with the environment; however, when the policy is applied in a real-world setting, then it may fail the task due to incorrect representation of the real-world environment inside the simulator. The issue with the RL agent managing to solve the given task in the simulator but not in the real-world environment is called the reality gap (Salvato et al., 2021). A presentation of methods to perform sim-to-real transfer and methods for minimizing the reality gap is out of the scope of this study; however, the methods are discussed in Salvato et al. (2021) and Zhao et al. (2020).
In contrast to creating a simulator of the real-world environment, offline RL (ORL) utilizes previous interactions with the environment, i.e., states, actions, rewards, and state transitions. Given the static dataset, the goal in ORL is to utilize the dataset to identify a better policy compared to the one observed (Levine et al., 2020). However, there are challenges in offline RL. When an agent is trained in simulation through trial and error, it allows the agent to explore states and actions, potentially identifying high-reward states. In ORL, however, if no high-reward states and actions are observed, then it may be challenging for the agent to identify those states and actions on its own (Levine et al., 2020). Another challenge in ORL is the distributional shift in which the agent is trained under one distribution but evaluated under a different distribution due to the exploitation policy, resulting in the agent acting in states not previously observed in the static dataset (Levine et al., 2020).
Previous studies have shown that the selection of hyperparameters (HPs) plays an important role in the performance of the RL agents. In ML, a distinction is made between parameters and HPs. A parameterized model estimates the parameters with data by minimizing an objective function. HPs are model configurations manually adjusted prior to executing the algorithms to train the model and affect the performance of the final model (Bischl et al., 2023). Adjusting HPs manually is a challenging task due to the vast number of combinations of HPs resulting in long computation times. Hyperparameter optimization (HPO) algorithms are useful in the search of HPs. Previous studies have highlighted that the selection of HPs has shown to be an important factor in the performance and training stability of the agent (Henderson et al., 2018; Engstrom et al., 2019; Andrychowicz et al., 2021). Factors such as random starting values and initialization of underlying neural networks (Henderson et al., 2018), code implementation (Engstrom et al., 2019), network architecture, advantage estimation, optimizers, and normalization techniques (Andrychowicz et al., 2021) affect the final performance of the agent. As addressed by Eimer et al. (2023), the selection process of HPs is important, and how the selection process is performed is important in the final evaluation of the agents. Utilizing HPO to find a set of HPs may result in the development of a fair benchmark but also ensures reproducibility of the results.
SLR is a form of review in which a systematic approach is used to gather and analyze a large amount of published research according to pre-defined research questions and criteria (Carrera-Rivera et al., 2022; Jesson et al., 2011). An SLR includes a clear description of how articles are collected, included or excluded, and analyzed by following a strict protocol (Carrera-Rivera et al., 2022). An SLR provides well-defined criteria on which articles are included or excluded from the SLR and proper documentation of the search process in databases by presenting the search string used, the date on which the search was performed, the name of the database, and the number of articles in each database (Jesson et al., 2011).
Jesson et al. (2011) describe the SLR process in six phases. (i) The first phase begins by performing a wide search in databases to discover existing knowledge gaps, what is already known, and how much relevant research is available. Furthermore, in this phase, the research plan is determined, which consists of defining the research question of the SLR, defining the inclusion and exclusion criteria, and identifying useful keywords to be used to search for suitable research articles. The identified keywords for water resource management are for example hydropower, irrigation, flood and water basin. The complete search strings are defined in Table 1. The initial search was performed in the databases Scopus and Web of Science to assess the number of publications relevant to the topic of this SLR. Furthermore, an additional search for previous review articles, previously presented in section 1.1, was performed to assess the relevance of performing an SLR on this topic.
(ii) The second phase involves performing a narrow search using the identified keywords and inclusion and exclusion criteria to filter the database and identify research articles relevant to the given research question. An initial screening of abstracts and titles is performed to reduce the number of articles in the search query before thoroughly analyzing each article. The initial search results using the search strings presented in Table 1 resulted in 678 articles (349 from Scopus and 329 from Web of Science). After screening titles and abstracts and removing duplicates, the sample size was reduced to 159 articles. The search was performed on 2024-05-07 in both Scopus and Web of Science.
(iii) The third phase consists of a quality assessment of each article included in the sample and a decision on whether the article should be included or not in the final sample based on the defined inclusion and exclusion criteria. In the sample of 159 articles, only 40 articles were found to be relevant according to the inclusion and exclusion criteria defined in Table 2.
(iv) The fourth phase is the data extraction phase, where important information from each article included in the sample is extracted and documented. The information extracted from each article includes the names of the authors, year of publication, research purpose, methodology, conclusion, how the authors have defined the reward function, action space, and state space, the authors’ choice of the algorithm in their application and how the agents are trained.
(v) This phase is called synthesis, which involves drawing conclusions about all articles and compiling knowledge into a single article. This phase is important for drawing conclusions about what is currently known and what remains to be studied. (vi) The sixth phase is the writing phase, in which all the information from phase (v) is combined and presented. The workflow of the SLR process and the results are presented in Figure 1.
Figure 1. Each phase of the SLR. indicates the number of articles remaining in the sample after each phase.
Table 3 presents an overview of all the optimization objectives by describing the purpose of the reward function in a study presented in this SLR.
Table 3. A summary of the purpose of the reward function and optimization objective in water resource management included in the SLR.
The number of publications in this sample steadily increases over the years, as presented in Figure 2. This suggests an increasing trend in the number of publications on RL application in water resource management and where there is an increasing research interest in how RL may be applied in water resource management.
Figure 2. Number of published articles each year in the sample (n = 40). 2024 was removed due to a search conducted in the second quarter of 2024.
Figure 3 presents the total occurrences of algorithms in the sample analyzed in the SLR. Less frequently occurring algorithms (occurring only once in the sample) are combined into another category. The first RQ, which poses the question of which algorithm is the most common, is DQN, followed by PPO, and finally DDPG and Q-learning. In the other category, algorithms such as multiagent DDPG, Q-learning, SARSA, or REINFORCE are occurring, albeit less frequently.
Figure 3. Frequency of RL algorithms in the sample. Infrequently occurring (occurring only once) algorithms are grouped together as Other.
Continuing the analysis of choice of algorithms, Figure 4 presents the choice of algorithms decomposed into various domains established in the SLR. Across all domains except for stormwater systems, PPO and DQN are utilized. In contrasts, DDPG only occurs in stormwater systems.
Figure 4. Frequency of RL-algorithm in various domains. Infrequent occurring (occurring only once) algorithms are grouped together as Other.
The trend in choice of algorithms is visualized in Figure 5. The use of DQN has steadily increased over time whereas the frequency of DDPG, Q-learning and PPO has remained steady.
Figure 5. Frequency of RL-algorithm in various domains over time. 2024 was removed due to a search conducted in the second quarter of 2024.
To further analyze the choice of algorithm based on whether it is value-based, policy-based, or actor-critic, Figure 6 presents the distribution of the methods among the different domains specified in this SLR. The most common choice is the value-based category, as supported by Figure 4, where DQN is the dominating choice in many of the domains.
Figure 6. Frequency of type of RL algorithm in various domains. Missing indicates that the algorithm can be classified as none of the categories. Both indicate that the chosen article has used both Value-based and Policy-based types in their study.
To address the second RQ, which poses which method is the most common to train an agent, Figure 7 presents the occurrences of training the agent in an offline, simulation, or mixed setting (e.g., training offline and evaluating in simulation or training in simulation and evaluating in a real-world setting). Across all domains, creating a simulation of the environment and training the RL agent is the most common choice, with the exception of hydropower, where offline training is more common.
Figure 8 presents the distribution of study regions in the sample. Although multiple studies are trained in simulation, local weather conditions are included. Therefore, the case study was conducted in the same country where the weather conditions were set. In many cases, the study nation or case study is not specified in the given study.
Zeng et al. (2023) applied DQN combined with dueling networks, DDQN, and prioritized experience replay for managing dispatch needs for the hydropower plant, such as water dispatch and power generation dispatch. Jiang et al. (2023) investigated how RL can be used to maximize profits in a multi-energy production system by using DQN to improve the scheduling of hydropower production when solar and wind power production forecasts are included in the model. Xu et al. (2020) investigated the potential of DQN in planning cascaded hydropower production. The authors mention two challenges: uncertainty in reservoir inflow forecast and large action spaces. The authors implement an aggregation desegregation model to reduce the action space, which allows for the discretization of the action space.
Wu et al. (2024) investigated multi-objective RL to train the agent to manage power generation, ecological aspects, and water supply benefits to nearby neighborhoods. The authors created multiple weight combinations for each objective in the reward function and found that RL found a policy that improved all objectives compared to baselines. Mitjana et al. (2022) investigated how power production in multiple reservoirs can be optimized where uncertainty in the expected reservoir inflow causes difficulties in managing constraints, such as water levels. The constraints are handled through chance constraints and backoffs, which are extended in the REINFORCE algorithm. The results show that although the amount of electricity production is smaller compared to the baseline, the water level constraints are much better handled using chance constraints and backoffs.
Riemer-Sorensen and Rosenlund (2020) used soft actor-critic to improve the weekly scheduling of hydropower production to maximize profits and reduce spillage water. The authors utilize historical weekly data. The authors utilize two different data sets: the first one, in which there is a clear correlation between reservoir inflow and electricity prices, and the second one, which provides a realistic representation of electricity prices and inflows.
DQN has been applied in irrigation applications with various objectives such as maximizing profits (Zhao et al., 2023), optimal water use for maintaining plant health (Maszuhn et al., 2023), or balancing multiple objectives (e.g., profits, water use and price of water) (Tao et al., 2023). Commonly, the actions of the DQN control how much water should be added based on different characteristics in the soil, such as ground moisture surrounding the crop. Chen et al. (2021) incorporated weather forecasts as a state together with DQN to plan how much irrigation should be provided. The agent is rewarded by how efficiently the agent provides irrigation conditioned on the expected rainfall and how it is expected to affect the ground moisture. If excessive irrigation is applied, the agent is penalized.
Maszuhn et al. (2023) investigated how weather forecasts can be included in the state space to plan for how irrigation should be added to maintain a certain level of ground moisture. However, a challenge still remains of how time delays affect the properties of the soil. Sun et al. (2017) applied SARSA for irrigation control to maximize profits from crop yields. However, the authors argue that training with a simulation environment reflecting the irrigation may be difficult to integrate with training the agent. Therefore, the authors train a neural network on a simulation environment to predict state transition, which is later used to train the agent.
Kelly et al. (2024) used PPO to manage profits from crop yields. A weather simulation model is used to create new weather conditions on which the agent is later tested to assess the performance of the learned policy. The authors found that the trained agent performed well in states that were observed during training. However, testing it on new states resulted in worse performance than the baseline, suggesting overfitting. Ding et al. (2022) also investigated PPO to minimize water use in irrigation to improve crop health. The authors implemented a safety mechanism to prevent the agent from taking actions that could harm the crop.
Huong et al. (2018) used the Markov-Decision process (MDP) to improve water and energy use in irrigation, where the objective is to decide upon the amount of water to achieve a certain level of ground moisture, which the authors define as the OK range. The authors compared their method with a baseline that provides water until the ground moisture is at the upper bound of the OK range. They found that the MDP outperformed the baseline in terms of energy and water use. A similar investigation was performed by Campoverde et al. (2021), in which the authors applied MDP in managing ground moisture surrounding the crop by modeling the MDP with three discrete actions in which the agent chose between providing no irrigation, little irrigation, and a lot of irrigation.
In larger water distribution networks (WDS), water pumps is the major component resulting in high energy use. Hu et al. (2023b) proposed that PPO manage water demands and water level constraints while minimizing the water pumps’ energy use. Additional perturbations are added to the water demand to reflect realistic daily variations. Zaman et al. (2023) proposed using DQN to schedule the use of water pumps to reduce the total energy use while simultaneously managing water levels in the WDS. Xu et al. (2021) investigated how PPO training can be improved using knowledge-assist (KA), which predicts the maximum state value from historical data. The reward structure is modified to include KA to better assess when the algorithm converges to its final policy, alleviating training instability in environments. Candelieri et al. (2019a) utilized Q-learning to manage the energy cost of a pump in the WDS. The authors designed the action space to be binary, in which each pump is either on or off, and it was found that Q-learning could manage the scheduling of pumps, even for larger action spaces (i.e., increasing the number of pumps to schedule), but also to manage the uncertainty in water demand.
Hu et al. (2023a) investigated how multi-agent RL can be used to reduce energy use and minimize water loss in WDS. The authors trained MADDPG in simulation to schedule all pumps and valves in the network. The authors found that MADDPG outperformed the established baseline method used in WDS and that the computation times for MADDPG are substantially smaller compared to baselines.
Hung and Yang (2021) addressed the challenge of meeting water demands in a non-stationary environment where water availability may change the water storage. The authors used Q-learning to distribute water and meet water demands. Moreover, Donâncio and Vercouter (2022) investigated how offline data of historical state-action transitions may be utilized to improve the exploration of DQN to manage water levels and improve pump selection, which is the most efficient given the state. Given any state, the authors use k-nearest neighbors, a supervised learning algorithm, to identify the states that are most similar to the current observed state and then see which action was made in similar states.
Wang et al. (2021) investigated how PPO can be applied in stormwater systems to manage flooding. In a simulation, PPO performed well. However, a challenge with DRL is that any applications of DRL utilize deep neural networks, which make it difficult to interpret and understand the underlying logic. Tian et al. (2024) proposed a method for better understanding why a decision is made in urban drainage systems, how the inputs affect the decision, and finally, interpreting the consequences of the given action made by the agent. To understand how decisions are affected by the inputs, the authors added small perturbations to the inputs and found that PPO was more sensitive to the perturbations compared to DQN. The authors train a surrogate tree-based model on a stored state-action dataset to interpret how a decision is made based on the given state. In urban drainage systems, ensuring safety and managing constraints are important. Tian et al. (2022b) address the safety constraints by implementing a method they call voting. They initially train multiple agents such as DQN, DDQN, and PPO using clipping loss, KL-loss, and A2C. The voting is then performed by selecting the agent whose actions minimize the expected risk, given the current state, which the authors define as the average water level in the whole system. The authors argue that voting is the same as adding constraints to the optimization problem but avoids the additional computational cost that may come with adding constraints. Instead, an action is picked from one of the trained agents.
Saliba et al. (2020) examined how incorrect estimates of states affect the performance of the agent in managing flooding in stormwater systems using DDPG. Noise is added to the states to simulate incorrect forecasts of future states. DDPG managed to deal with flooding even though incorrect forecasts of precipitation or incorrect estimates of water levels were included as states. Bowes et al. (2021) trained three agents with different reward functions to assess how the agent can manage flooding in the system. The first agent addressed water level constraints and flooding, and the second agent, in addition to water levels and flooding, also managed total suspended solids from nearby ponds in the systems. The third agent aimed to find a balance between the two previous agents’ reward functions. All three agents were trained with DDPG, and the authors found that the agents converged to different policies, resulting in different performance. Bowes et al. (2021) compared DDPG with model predictive control (MPC) and rule-based control, which stipulates certain decisions, such as water levels and predicted total precipitation. The DDPG agent was trained offline and compared to the MPC and rule-based agent in a simulation. It was found that both the DDPG and rule-based agents performed better than MPC, with the additional benefit of substantial reduction in computational cost, suggesting that RL is feasible for real-time control of stormwater systems.
Tian et al. (2023a) investigated how state selection affects the model performance in managing flooding in drainage systems. Using PPO, the authors found that how the state space is defined affects the performance of the agent in managing flooding. Tian et al. (2022a) addressed how computational times of training an agent in simulation can be reduced. The authors utilize the Koopman emulator to learn the dynamics of urban drainage systems and train the RL agent based on the learned emulator. The authors use PPO and DQN, both of which are trained on the Koopman emulator, and then the policies are compared to agents trained in a correct simulation. The authors found that the agent trained with the Koopman emulator worked well but with the additional benefit of a substantial reduction in computation cost. Tian et al. (2023b) applied DQN and PPO to manage drainage system water levels. The authors investigated how constraints can be included in the model to ensure safety in the final RL policy by adding a penalty to the reward signal and applying a method called constrained policy optimization (see Tessler et al., 2018). The authors found that the trained RL agent provided safe action to manage water levels; however, the design of suitable safety constraints for different drainage systems is not established.
Zhang et al. (2023) compared centralized and decentralized multi-agent RL (MARL) algorithms for managing flooding in drainage systems whilst simultaneously reducing energy use. A challenge is the communication among the agents when managing flooding, which the authors aimed to address. They found that decentralized systems could better manage flooding and that MARL shows promise in real-time control of drainage systems.
Ren et al. (2021c) utilized Hidden Markov Models (HMM) to guide the exploration of the DDPG agent in canal management to manage energy use in controlling gates and water level constraints. Due to the large action space, efficient exploring and training are difficult. The authors, therefore, utilize HMM and historical data to generate the reward and guide the learning process.
Ren et al. (2021a) used DQN for a multi-objective task to optimize canal management in regard to multiple objectives such as water levels, and the velocity of water, i.e., the time it takes to deliver water from the beginning of the canal to the end of the canal and to prevent frequent changes in gate opening. Multi-objective RL poses a challenge, which is how much each objective should receive in the reward function, and to alleviate that challenge, Ren et al. (2021a) utilized a reward network that predicts the reward of the actions made in a given state. Shahverdi et al. (2022) used double Q-learning for irrigation canals to manage water demands while simultaneously managing water levels in the canal. The authors’ inutility in establishing a state-space model of the canal based on historical data allows for a simulation of the dynamics to be performed. Based on the simulation, the Double Q-learning agent is trained to learn a policy with satisfactory results.
Ren et al. (2021b) applied hierarchical RL to address challenges with large action spaces in the management of large-scale canals. The authors divide the task into two levels: policy learning and action learning. The policy learning learns an abstract state of the canals, and the action learning is applied to RL algorithms aimed at managing water levels in the individual pools.
Seo et al. (2021) investigated PPO and DQN in wastewater treatment. The challenge in this article is the uncertainty in reservoir inflow in order to manage energy use in regard to water pumps and water level constraints. The authors develop two types of models: a predictive model and an RL model. The predictive model is used to forecast the water inflow, and based on the forecast, the agents are learning a policy to manage. The authors found that water constraints, i.e., water levels, are violated during testing, and they concluded that a contributing factor to poor performance is an incorrect forecast of future states. Filipe et al. (2019) also investigated how PPO can be used for wastewater treatment to control water levels and reduce the energy use of pumps in the network. The authors used weather forecasts to plan and identify a better policy that reduced energy use when the forecasts were incorporated into the state space.
Bertoni et al. (2017) used fitted Q-iteration [see Ernst et al. (2005) for details of the algorithm] for reservoir management to manage the reservoir such that water demand constraints downstream are met. External factors, such as reservoir inflow, are assumed to come from some probability distribution, which the authors include in their FQI model. The challenge is that the reservoir inflow is stochastic and not stationary due to climate change. To address this, the authors assessed how changes in the parameterization of the probability distribution of reservoir inflow affect the model performance. FQI managed to meet the water demand when reservoir inflows were normal or higher than normal but failed to meet the demand. The authors argue that smaller inflows and lack of storage result in the failure to meet the demand.
Moreira et al. (2022) investigated the potential of PPO in real-time control of pumps in tidal power to optimize the production. The authors trained PPO in simulation and found that PPO performed well compared to state-of-the-art models used in tidal power optimization, with the added benefit of the model not requiring a forecast to optimize the production but rather can perform the optimization in real time.
In sequential decision-making, understanding the logic of how a decision is made by the agent is important for transparency and reliability. Tian et al. (2024) explored how to explain the logic of the agent when a decision is made in any given state by utilizing tree models and more. Explaining the complicated decision process provides insights into the decision-making process and helps better understand if and why the agent fails under certain circumstances. In sensitive and safety-critical applications such as water resource management, understanding why the systems fail to manage constraints, e.g., water levels, is important to further improve the agent and the final policy. Improvement can later be made by, for example, designing other reward functions or collecting more data in states that are rarely observed to improve the training of the agents.
DQN is the most frequently occurring choice of algorithm in this SLR. DQN requires the action space to be discrete, and the action space is often designed by discretizing a continuous variable. The results often show promising performance. However, what are the effects of discretizing a continuous variable? What is the effect of border cases, for example, managing river discharges that are almost equal but are placed in two different categories due to the discretization? A key point of interest is comparing agents with discrete action spaces to those with continuous action spaces, focusing on cumulative rewards, constraint handling, and computational times to assess the impact of discretization on performance.
Figure 4 shows the frequency of algorithms in the various domains. In hydropower, PPO and DDPG have not yet been explored as an approach for resource management, highlighting a future research direction. Similarly, Q-learning only occurs in the water distribution network category, suggesting a suitable baseline method in all domains when using value-based RL algorithms.
Although multiple studies only use a single type of RL algorithm, either policy-based or value-based, and compare them against a simpler baseline, only a few studies have benchmarked policy-based against value-based RL algorithms (e.g., Tian et al., 2022b; Tian et al., 2024; Seo et al., 2021). Often, the results show no significant difference in the overall performance of the models. However, the RL agents may converge to different policies, thus resulting in different behaviors. Therefore, future research should encourage the implementation of both value-based and policy-based RL algorithms to assess the potential of utilizing RL for sequential decision-making in water resource management.
The most common way to train an RL agent is to utilize a simulator, which allows the agent to explore through trial and error which actions are the best to take in a given state. However, as addressed in section 2.6, the reality gap is a challenge of training in simulations where the simulation is not a fully correct description of the environment in which the agent will later interact post-training. Moreover, when training agents in a simulated environment, it is important to address the challenges associated with this process and to outline the measures implemented to minimize the reality gap. Similarly, the articles using historical data for training the agents, i.e., ORL, need to address challenges with overfitting to a training set and to carefully assess the performance on a large data set with a wide variety of observed states. As discussed in section 2.7, the distributional shift poses a challenge in which the policy will be evaluated under a different distribution. Therefore, a large and varied test set is required to test the final learned policy. As highlighted by Kelly et al. (2024), the overfitting issue appears to occur even in simulation, suggesting that a test set and validation set may be required when trained in simulation to assess errors made by the agent in new unseen states.
Figure 5 presents the frequency of training methods in each domain. In hydropower, offline learning is the most frequent method of training the agent, whereas in other domains, utilizing a simulation environment to train the agent is more common. The future research direction is to establish an open-source simulation environment for hydropower or similar exploration of offline methods in irrigation, stormwater systems, or urban drainage systems to assess the potential of RL in sequential decision-making. Although several studies using simulation as a method for training the agent have attempted to deal with the reality gap by adding uncertainty to states by adding perturbations, there is an existing research gap in a more thorough analysis of established methods in managing reality gaps.
In Figure 2, the number of articles included after the initial screening process is 40, due to either being out of scope or authors not properly defining the action space, state space, or the reward function. To encourage replicability and enhance the understanding of the implementation, thorough documentation of how the RL task is defined is crucial. Therefore, it is essential to include comprehensive documentation of all components of the modeling process. Moreover, the selection of hyperparameters (HPs) is vital, as discussed in section 2.7, for both overall performance and training stability. Utilizing algorithms to identify a suitable selection of HPs is significant for two reasons: performance and reproducibility. Multiple studies in this systematic literature review (SLR) do not disclose how HPs are selected, highlighting the importance of transparency in HP choices. In addition to potentially improving the performance of the RL algorithm, a clear explanation of HP selection or utilizing Hyperparameter Optimization (HPO) facilitates the reproduction of results more effectively.
The reward function controls the learned behavior of the agent by providing feedback on the action made in a given state. Often, prior knowledge is required to design the reward signal to solve the given task in water resource management. In the studies included in the SLR, the reward signal was manually designed, potentially missing out on important information for training the agent. Preference-based RL (Wirth et al., 2017) allows domain experts and stakeholders to assess the actions made by agents, resulting in a preferable policy that can manage various water resource management tasks without the need to manually design the reward signal.
Finally, utilizing RL for sequential decision-making requires continuous model evaluation to assess performance and ensure constraints are not violated. In critical infrastructure such as water distribution networks or hydropower, minimizing risk is an important ethical consideration that requires attention and is therefore important in future research.
This research reveals that most RL models are currently trained in simulation environments. While simulations provide a controlled setting for model development, there exists a notable research gap in transitioning from simulation to real-world applications. Addressing this gap is crucial to ensure that RL models can be effectively applied in practice, particularly in complex water resource systems. Offline RL, an approach that learns from pre-collected datasets rather than through direct interaction, is another area that warrants further investigation. Given the challenges of gathering real-time data in water management systems, offline RL could present a promising alternative, facilitating more efficient model training.
In terms of constraint handling, this review identifies that the most common method is to integrate constraints directly into the reward signal. However, there is a need to develop multi-objective reward functions that can more effectively capture the complexities of water resource management. Future research should focus on automating reward function design, as the current method relies heavily on trial and error, which can be time-consuming and suboptimal. Another significant research gap lies in addressing the selection of HPs, which directly affects model performance. Numerous studies fail to address or optimize HPs adequately, making it challenging to evaluate the reproducibility of the results. To address this issue, we recommend employing HPO algorithms. This would help ensure (i) convergence to an optimal solution and (ii) enhanced reproducibility of research findings.
Furthermore, scientific investigations have placed limited focus on planning models, particularly model-based RL. Most approaches emphasize real-time decision-making rather than leveraging models that can predict future states of the system. Assessing the use of model-based RL in future research could provide a pathway to more effective and well-informed decision-making in water resource management.
Finally, designing the reward function may require prior knowledge of the domain in which RL is applied. Preference-based RL is a future research direction in which the preferences of domain experts and stakeholders can be incorporated into the training process of the RL agents, potentially leading to a safer policy suitable for water resource management.
LK: Conceptualization, Formal analysis, Investigation, Methodology, Visualization, Writing – original draft. VM: Conceptualization, Supervision, Writing – review & editing. MA: Supervision, Writing – review & editing. MW: Conceptualization, Project administration, Supervision, Writing – review & editing.
The author(s) declare financial support was received for the research, authorship, and/or publication of this article. The authors express their gratitude to the company Tekniska Verken i Linköping AB for their funding and to Professor Bahram Moshfegh for the acquisition of the funding.
The authors declare that the research was conducted in the absence of any commercial or financial relationships that could be construed as a potential conflict of interest.
The author(s) declare that Gen AI was used in the creation of this manuscript. Copilot was utilized for checking grammar in the final manuscript.
All claims expressed in this article are solely those of the authors and do not necessarily represent those of their affiliated organizations, or those of the publisher, the editors and the reviewers. Any product that may be evaluated in this article, or claim that may be made by its manufacturer, is not guaranteed or endorsed by the publisher.
Ahmed, A. A., Sayed, S., Abdoulhalik, A., Moutari, S., and Oyedele, L. (2024). Applications of machine learning to water resources management: a review of present status and future opportunities. J. Clean. Prod. 441:140715. doi: 10.1016/j.jclepro.2024.140715
Andrychowicz, M., Raichuk, A., Stańczyk, P., Orsini, M., Girgin, S., Marinier, R., et al. (2021). "What matters for on-policy deep actor-critic methods? A large-scale study." International conference on learning representations.
Bernardes, J., Santos, M., Abreu, T., Prado, L., Miranda, D., Julio, R., et al. (2022). Hydropower operation optimization using machine learning: a systematic review. AI 3, 78–99. doi: 10.3390/ai3010006
Bertoni, F., Giuliani, M., and Castelletti, A. (2017). "Scenario-based fitted Q-iteration for adaptive control of water reservoir systems under uncertainty." IFAC-Papers online.
Bischl, B., Binder, M., Lang, M., Pielok, T., Richter, J., Coors, S., et al. (2023). Hyperparameter optimization: foundations, algorithms, best practices, and open challenges. Wiley Interdiscipl. Rev. 13:e1484. doi: 10.1002/widm.1484
Bishop, C. M. (2006). Pattern recognition and machine learning (information science and statistics). New York, NY: Springer-Verlag.
Bowes, B. D., Tavakoli, A., Wang, C., Heydarian, A., Behl, M., Beling, P. A., et al. (2021). Flood mitigation in coastal urban catchments using real-time stormwater infrastructure control and reinforcement learning. J. Hydroinf. 23, 529–547. doi: 10.2166/hydro.2020.080
Bowes, B. D., Wang, C., Ercan, M. B., Culver, T. B., Beling, P. A., and Goodall, J. L. (2022). Reinforcement learning-based real-time control of coastal urban stormwater systems to mitigate flooding and improve water quality. Environ. Sci. Water Res. Technol. 8, 2065–2086. doi: 10.1039/d1ew00582k
Campoverde, L. M. S., Tropea, M., and De Rango, F. (2021). "An IoT-based smart irrigation management system using reinforcement learning modeled through a Markov decision process." Proceedings of the 2021 ieee/acm 25th international symposium on distributed simulation and real time applications (ds-rt 2021).
Candelieri, A., Galuzzi, B. G., Giordani, I., Perego, R., and Archetti, F. (2019a). "Business information Systems for the Cost/energy Management of Water Distribution Networks: a critical appraisal of alternative optimization strategies." Business information systems workshops (bis 2018).
Candelieri, A., Perego, R., and Archetti, F. (2019b). Intelligent pump scheduling optimization in water distribution networks : Learning and intelligent optimization, LION, 12.
Cao, D., Weihao, H., Zhao, J., Zhang, G., Zhang, B., Liu, Z., et al. (2020). Reinforcement learning and its applications in modern power and energy systems: a review. J. Mod. Power Syst. Clean Energy 8, 1029–1042. doi: 10.35833/MPCE.2020.000552
Carrera-Rivera, A., Ochoa, W., Larrinaga, F., and Lasa, G. (2022). How-to conduct a systematic literature review: a quick guide for computer science research. MethodsX 9:101895. doi: 10.1016/j.mex.2022.101895
Chen, M. T., Cui, Y. L., Wang, X. N., Xie, H. W., Liu, F. P., Luo, T. Y., et al. (2021). A reinforcement learning approach to irrigation decision-making for rice using weather forecasts. Agric. Water Manag. 250:106838. doi: 10.1016/j.agwat.2021.106838
Ciampittiello, M., Marchetto, A., and Boggero, A. (2024). Water resources management under climate change: a review. Sustain. For. 16:3590. doi: 10.3390/su16093590
Croll, H. C., Ikuma, K., Ong, S. K., and Sarkar, S. (2023). Reinforcement learning applied to wastewater treatment process control optimization: approaches, challenges, and path forward. Crit. Rev. Environ. Sci. Technol. 53, 1775–1794. doi: 10.1080/10643389.2023.2183699
Ding, X. Z., Du, W., and Ieee Comp, S. O. C. (2022). "DRLIC: deep reinforcement learning for irrigation control." 2022 21st ACM/IEEE international conference on information processing in sensor networks (IPSN 2022).
Donâncio, H., and Vercouter, L. (2022). "Safety through intrinsically motivated imitation learning." ALA 2022—Adaptive and Learning Agents Workshop at AAMAS 2022.
Eimer, T., Lindauer, M., and Raileanu, R. (2023). "Hyperparameters in reinforcement learning and how to tune them." International conference on machine learning.
Engstrom, L., Ilyas, A., Santurkar, S., Tsipras, D., Janoos, F., Rudolph, L., et al. (2019). "Implementation matters in deep rl: a case study on ppo and trpo." International conference on learning representations.
Ernst, D., Geurts, P., and Wehenkel, L. (2005). Tree-based batch mode reinforcement learning. J. Mach. Learn. Res. 6, 503–556.
Filipe, J., Bessa, R. J., Reis, M., Alves, R., and Póvoa, P. (2019). Data-driven predictive energy optimization in a wastewater pumping station. Appl. Energy 252:113423. doi: 10.1016/j.apenergy.2019.113423
Haydari, A., and Yılmaz, Y. (2020). Deep reinforcement learning for intelligent transportation systems: a survey. IEEE Trans. Intell. Transp. Syst. 23, 11–32. doi: 10.1109/TITS.2020.3008612
Hayes, C. F., Rădulescu, R., Bargiacchi, E., Källström, J., Macfarlane, M., Reymond, M., et al. (2022). A practical guide to multi-objective reinforcement learning and planning. Auton. Agent. Multi-Agent Syst. 36:26. doi: 10.1007/s10458-022-09552-y
Henderson, P., Islam, R., Bachman, P., Pineau, J., Precup, D., and Meger, D. (2018). "Deep reinforcement learning that matters." Proceedings of the AAAI conference on artificial intelligence.
Hessel, M., Modayil, J., Van Hasselt, H., Schaul, T., Ostrovski, G., Dabney, W., et al. (2018). "Rainbow: combining improvements in deep reinforcement learning." Proceedings of the AAAI conference on artificial intelligence.
Hu, S. Y., Gao, J. L., and Zhong, D. (2023a). Multi-agent reinforcement learning framework for real-time scheduling of pump and valve in water distribution networks. Water Supply 23, 2833–2846. doi: 10.2166/ws.2023.163
Hu, S. Y., Gao, J. L., Zhong, D., Wu, R., and Liu, L. M. (2023b). Real-time scheduling of pumps in water distribution systems based on exploration-enhanced deep reinforcement learning. Systems 11, 1–13. doi: 10.3390/systems11020056
Hung, F., and Yang, Y. C. E. (2021). Assessing adaptive irrigation impacts on water scarcity in nonstationary environments—a multi-agent reinforcement learning approach. Water Resour. Res. 57. doi: 10.1029/2020WR029262
Huong, T. T., Huu Thanh, N., Van, N. T., Tien Dat, N., Long, N. V., and Marshall, A. (2018). "Water and energy-efficient irrigation based on markov decision model for precision agriculture." 2018 IEEE 7th International conference on Communications and electronics, ICCE 2018.
IEA. (2021). Hydropower special market report. (IEA). Available online at: https://www.iea.org/reports/hydropower-special-market-report
IPCC (2023). “Water,” in Climate change 2022 – Impacts, adaptation and vulnerability: Working group II contribution to the sixth assessment report of the intergovernmental panel on climate change (Cambridge: Cambridge University Press), 551–712. Available at: https://www.cambridge.org/core/books/climate-change-2022-impacts-adaptation-and-vulnerability/water/7A49785973EC54E41371F6F36D471D9
Jesson, J., Matheson, L., and Lacey, F. M. (2011). “Doing your literature review: traditional and systematic techniques.” London: SAGE Publications Ltd.
Jiang, W., Liu, Y., Fang, G., and Ding, Z. (2023). Research on short-term optimal scheduling of hydro-wind-solar multi-energy power system based on deep reinforcement learning. J. Clean. Prod. 385:135704. doi: 10.1016/j.jclepro.2022.135704
Kahaduwa, A., and Rajapakse, L. (2021). Review of climate change impacts on reservoir hydrology and long-term basin-wide water resources management. Build. Res. Inform. 50, 515–526. doi: 10.1080/09613218.2021.1977908
Kelly, T. D., Foster, T., and Schultz, D. M. (2024). Assessing the value of deep reinforcement learning for irrigation scheduling. Smart Agric. Technol. 7:100403. doi: 10.1016/j.atech.2024.100403
Kiran, B. R., Sobh, I., Talpaert, V., Mannion, P., Al, A. A., Sallab, S. Y., et al. (2021). Deep reinforcement learning for autonomous driving: a survey. IEEE Trans. Intell. Transp. Syst. 23, 4909–4926. doi: 10.1109/TITS.2021.3054625
Kober, J., Andrew Bagnell, J., and Peters, J. (2013). Reinforcement learning in robotics: a survey. Int. J. Robot. Res. 32, 1238–1274. doi: 10.1177/0278364913495721
Kourtis, I. M., and Tsihrintzis, V. A. (2021). Adaptation of urban drainage networks to climate change: a review. Sci. Total Environ. 771:145431. doi: 10.1016/j.scitotenv.2021.145431
Krechowicz, A., Krechowicz, M., and Poczeta, K. (2022). Machine learning approaches to predict electricity production from renewable energy sources. Energies 15. doi: 10.3390/en15239146
LeCun, Y., Bengio, Y., and Hinton, G. (2015). Deep learning. Nature 521, 436–444. doi: 10.1038/nature14539
Levine, S., Kumar, A., Tucker, G., and Fu, J. (2020). Offline reinforcement learning: tutorial, review, and perspectives on open problems. arXiv. doi: 10.48550/arXiv.2005.01643
Li, J., Li, X., Liu, H., Gao, L., Wang, W., Wang, Z., et al. (2023). Climate change impacts on wastewater infrastructure: a systematic review and typological adaptation strategy. Water Res. 242:120282. doi: 10.1016/j.watres.2023.120282
Lillicrap, T. P. (2015). Continuous control with deep reinforcement learning. arXiv. doi: 10.48550/arXiv.1509.02971
Maszuhn, M., Aschwege, F. M. V., Boll-Westermann, S., and Pinski, J. (2023). Learning to irrigate—a model of the plant water balance : Lecture Notes in Networks and Systems.
Mitjana, F., Denault, M., and Demeester, K. (2022). Managing chance-constrained hydropower with reinforcement learning and backoffs. Adv. Water Resour. 169:104308. doi: 10.1016/j.advwatres.2022.104308
Mnih, V., Kavukcuoglu, K., Silver, D., Graves, A., Antonoglou, I., Wierstra, D., et al. (2013). Playing atari with deep reinforcement learning. arXiv. doi: 10.48550/arXiv.1312.5602
Mnih, V., Kavukcuoglu, K., Silver, D., Rusu, A. A., Veness, J., Bellemare, M. G., et al. (2015). Human-level control through deep reinforcement learning. Nature 518, 529–533. doi: 10.1038/nature14236
Mohammed, S. J., Zubaidi, S. L., Ortega-Martorell, S., Al-Ansari, N., Ethaib, S., and Hashim, K. (2022). Application of hybrid machine learning models and data pre-processing to predict water level of watersheds: recent trends and future perspective. Cogent Eng. 9:2143051. doi: 10.1080/23311916.2022.2143051
Moreira, T. M., de Faria, J. G., Vaz-de-Melo, P. O. S., Chaimowicz, L., and Medeiros-Ribeiro, G. (2022). Prediction-free, real-time flexible control of tidal lagoons through proximal policy optimisation: a case study for the Swansea lagoon. Ocean Eng. 247:110657. doi: 10.1016/j.oceaneng.2022.110657
Ortiz-Lopez, C., Bouchard, C., and Rodriguez, M. (2022). Machine learning models with potential application to predict source water quality for treatment purposes: a critical review. Environ. Technol. Rev. 11, 118–147. doi: 10.1080/21622515.2022.2118084
Ren, T., Niu, J., Cui, J., Ouyang, Z., and Liu, X. (2021a). An application of multi-objective reinforcement learning for efficient model-free control of canals deployed with IoT networks. J. Netw. Comput. Appl. 182:103049. doi: 10.1016/j.jnca.2021.103049
Ren, T., Niu, J. W., Liu, X. F., Wu, J. Y., Lei, X. H., and Zhang, Z. (2021b). An efficient model-free approach for controlling large-scale canals via hierarchical reinforcement learning. IEEE Trans. Industr. Inform. 17, 4367–4378. doi: 10.1109/TII.2020.3004857
Ren, T., Niu, J. W., Shu, L., Hancke, G. P., Wu, J. Y., Liu, X. F., et al. (2021c). Enabling efficient model-free control of large-scale canals by exploiting domain knowledge. IEEE Trans. Ind. Electron. 68, 8730–8742. doi: 10.1109/TIE.2020.3013778
Riemer-Sorensen, S., and Rosenlund, G. H., (2020). "Deep reinforcement learning for Long term hydropower production scheduling." 2020 International conference on SMART energy systems and technologies (SEST).
Saliba, S. M., Bowes, B. D., Adams, S., Beling, P. A., and Goodall, J. L. (2020). Deep reinforcement learning with uncertain data for real-time Stormwater system control and flood mitigation. Water 12, 1–19. doi: 10.3390/w12113222
Salvato, E., Fenu, G., Medvet, E., and Pellegrino, F. A. (2021). Crossing the reality gap: a survey on Sim-to-real transferability of robot controllers in reinforcement learning. IEEE Access 9, 153171–153187. doi: 10.1109/ACCESS.2021.3126658
Schulman, J., Wolski, F., Dhariwal, P., Radford, A., and Klimov, O. (2017). Proximal policy optimization algorithms. arXiv. doi: 10.48550/arXiv.1707.06347
Seo, G., Yoon, S., Kim, M., Mun, C., and Hwang, E. (2021). Deep reinforcement learning-based smart joint control scheme for on/off pumping Systems in Wastewater Treatment Plants. IEEE Access 9, 95360–95371. doi: 10.1109/ACCESS.2021.3094466
Shahverdi, K., Alamiyan-Harandi, F., and Maestre, J. M. (2022). Double Q-PI architecture for smart model-free control of canals. Comput. Electron. Agric. 197:106940. doi: 10.1016/j.compag.2022.106940
Silver, D., Huang, A., Maddison, C. J., Guez, A., Sifre, L., van den Driessche, G., et al. (2016). Mastering the game of go with deep neural networks and tree search. Nature 529, 484–489. doi: 10.1038/nature16961
Sit, M., Demiray, B. Z., Xiang, Z., Ewing, G. J., Sermet, Y., and Demir, I. (2020). A comprehensive review of deep learning applications in hydrology and water resources. Water Sci. Technol. 82, 2635–2670. doi: 10.2166/wst.2020.369
Sun, L. J., Yang, Y. X., Hu, J., Porter, D., Marek, T., and Hillyer, C. (2017). "Reinforcement learning control for water-efficient agricultural irrigation." 2017 15th ieee international symposium on parallel and distributed processing with applications and 2017 16th ieee international conference on ubiquitous computing and communications (ISPA/IUCC 2017).
Sutton, R. S., and Barto, A. G. (2018). Reinforcement learning: an introduction. Cambridge, Massachusetts: MIT press.
Tao, R., Zhao, P., Wu, J., Martin, N., Harrison, M. T., Ferreira, C., et al. (2023). "Optimizing crop management with reinforcement learning and imitation learning." IJCAI International joint conference on artificial intelligence.
Tessler, C., Mankowitz, D. J., and Mannor, S. (2018). Reward constrained policy optimization. arXiv. doi: 10.48550/arXiv.1805.11074
Tian, W., Fu, G., Xin, K., Zhang, Z., and Liao, Z. (2024). Improving the interpretability of deep reinforcement learning in urban drainage system operation. Water Res. 249:120912. doi: 10.1016/j.watres.2023.120912
Tian, W., Liao, Z., Zhang, Z., Wu, H., and Xin, K. (2022a). Flooding and overflow mitigation using deep reinforcement learning based on Koopman operator of urban drainage systems. Water Resour. Res. 58. doi: 10.1029/2021WR030939
Tian, W., Liao, Z., Zhi, G., Zhang, Z., and Wang, X. (2022b). Combined sewer overflow and flooding mitigation through a reliable real-time control based on multi-reinforcement learning and model predictive control. Water Resour. Res. 58. doi: 10.1029/2021WR030703
Tian, W., Xin, K., Zhang, Z., Liao, Z., and Li, F. (2023a). State selection and cost estimation for deep reinforcement learning-based real-time control of urban drainage system. Water (Switzerland) 15. doi: 10.3390/w15081528
Tian, W., Xin, K., Zhang, Z., Zhao, M., Liao, Z., and Tao, T. (2023b). Flooding mitigation through safe & trustworthy reinforcement learning. J. Hydrol. 620:129435. doi: 10.1016/j.jhydrol.2023.129435
Tripathy, K. P., and Mishra, A. K. (2023). Deep learning in hydrology and water resources disciplines: concepts, methods, applications, and research directions. J. Hydrol. 628:130458. doi: 10.1016/j.jhydrol.2023.130458
United Nations. (2022). The sustainable development goals report 2022. Available online at: https://unstats.un.org/sdgs/report/2022/.
Van Hasselt, H., Guez, A., and Silver, D. (2016). "Deep reinforcement learning with double q-learning." Proceedings of the AAAI conference on artificial intelligence.
Villeneuve, Y., Séguin, S., and Chehri, A. (2023). AI-based scheduling models, optimization, and prediction for hydropower generation: opportunities, issues, and future directions. Energies 16. doi: 10.3390/en16083335
Wang, C., Bowes, B. D., Beling, P. A., and Goodall, J. L. (2021). "Reinforcement learning for flooding mitigation in complex Stormwater systems during large storms." IEEE eurocon 2021—19th international conference on smart technologies.
Wang, Z., Schaul, T., Hessel, M., Hasselt, H., Lanctot, M., and Freitas, N. (2016). "Dueling network architectures for deep reinforcement learning." International conference on machine learning.
Wirth, C., Akrour, R., Neumann, G., and Fürnkranz, J. (2017). A survey of preference-based reinforcement learning methods. J. Mach. Learn. Res. 18, 1–46.
Wu, R. X., Wang, R., Hao, J., Wu, Q., and Wang, P. (2024). Multiobjective multihydropower reservoir operation optimization with transformer-based deep reinforcement learning. J. Hydrol. 632:130904. doi: 10.1016/j.jhydrol.2024.130904
Xu, J., Wang, H., Rao, J., and Wang, J. (2021). Zone scheduling optimization of pumps in water distribution networks with deep reinforcement learning and knowledge-assisted learning. Soft. Comput. 25, 14757–14767. doi: 10.1007/s00500-021-06177-3
Xu, W., Zhang, X. L., Peng, A. B., and Liang, Y. (2020). Deep reinforcement learning for cascaded hydropower reservoirs considering inflow forecasts. Water Resour. Manag. 34, 3003–3018. doi: 10.1007/s11269-020-02600-w
Zaman, M., Tantawy, A., and Abdelwahed, S. (2023). "Optimizing Smart City water distribution systems using deep reinforcement learning." I2023 EEE 20th International conference on smart communities: Improving quality of life using AI, Robotics and IoT, HONET 2023.
Zeng, Y. X., Wen, X., Tan, Q. F., Liu, Y., and Chen, X. Y. (2023). Real-time load dispatch in hydropower plant based on D3QN-PER. J. Hydrol. 625:130019. doi: 10.1016/j.jhydrol.2023.130019
Zhang, Z., Tian, W., and Liao, Z. (2023). Towards coordinated and robust real-time control: a decentralized approach for combined sewer overflow and urban flooding reduction based on multi-agent reinforcement learning. Water Res. 229:119498. doi: 10.1016/j.watres.2022.119498
Zhao, H., Di, L., Guo, L., Li, L., Zhang, C., Yu, E., et al. (2023). "Optimizing irrigation scheduling using deep reinforcement learning." 2023 11th International conference on agro-Geoinformatics, agro-Geoinformatics 2023.
Zhao, W., Queralta, J. P., and Westerlund, T. (2020). "Sim-to-real transfer in deep reinforcement learning for robotics: a survey." 2020 IEEE symposium series on computational intelligence (SSCI).
Zhu, Z., Lin, K., Jain, A. K., and Zhou, J. (2023). Transfer learning in deep reinforcement learning: a survey. IEEE Trans. Pattern Anal. Mach. Intell. 45, 13344–13362. doi: 10.1109/TPAMI.2023.3292075
Keywords: reinforcement learning, machine learning, water resource management, systematic literature review, decision-making
Citation: Kåge L, Milić V, Andersson M and Wallén M (2025) Reinforcement learning applications in water resource management: a systematic literature review. Front. Water. 7:1537868. doi: 10.3389/frwa.2025.1537868
Received: 09 December 2024; Accepted: 17 February 2025;
Published: 12 March 2025.
Edited by:
Reza Kerachian, University of Tehran, IranReviewed by:
K. S. Kasiviswanathan, Indian Institute of Technology Roorkee, IndiaCopyright © 2025 Kåge, Milić, Andersson and Wallén. This is an open-access article distributed under the terms of the Creative Commons Attribution License (CC BY). The use, distribution or reproduction in other forums is permitted, provided the original author(s) and the copyright owner(s) are credited and that the original publication in this journal is cited, in accordance with accepted academic practice. No use, distribution or reproduction is permitted which does not comply with these terms.
*Correspondence: Linus Kåge, bGludXMua2FnZUBsaXUuc2U=
Disclaimer: All claims expressed in this article are solely those of the authors and do not necessarily represent those of their affiliated organizations, or those of the publisher, the editors and the reviewers. Any product that may be evaluated in this article or claim that may be made by its manufacturer is not guaranteed or endorsed by the publisher.
Research integrity at Frontiers
Learn more about the work of our research integrity team to safeguard the quality of each article we publish.