- 1Faculty Geography Resource Sciences, Sichuan Normal University, Chengdu, China
- 2Key Laboratory of Watershed Geographic Sciences, Nanjing Institute of Geography and Limnology, Chinese Academy of Sciences, Nanjing, China
- 3University of Chinese Academy of Sciences, Nanjing (UCASNJ), Nanjing, China
- 4University of Chinese Academy of Sciences, Beijing, China
Lake water level is an important variable to indicate lake hydrological balances and climate change impacts. Benefiting from the launch of the laser altimeters ICESat and ICESat-2, higher spatial-resolution elevation measurements have opened new possibilities for monitoring lake levels globally over the past two decades. However, uncertainties on the combined use of two-generation satellite laser measurements have not yet been investigated specifically. This study aimed to summarize the important technique notes on water level data processing by integrating the ICESat and ICESat-2 altimetry measurements. We mainly focused on the effect of geoid height, water masks for extracting altimetry footprints, and the 9-year data gap between the two generations of satellites on water level change estimates. We compared the influences of the above three factors in different situations by selecting typical lakes worldwide as study cases. The results showed that: (1) In the combination of ICESat and ICESat-2 products, geoid heights need to be recalculated for each footprint based on its longitude and latitude in order to replace the geoid values of the original products when calculating orthometric heights. It is necessary because the default geoids in both generations of products (ICESat and ICESat-2) exhibit a systematic deviation; (2) To balance the accuracy and efficiency, the small water mask in the low-level year is recommended to extract the potential footprints in comparison with the laborious processing of time-varying water masks; (3) The 9-year data gap between ICESat and ICESat-2 observations may cause inevitable overestimations or underestimations of the long-term change rate of lake levels with a non-linear trajectory, yet it has few effects on lakes with (near) linear trending or fluctuating changes.
1 Introduction
Lakes, as the key component of the hydrosphere, participate in the global hydrological cycle. Climate change and human activities significantly affect lake formation, development, expansion, and shrinkage. Monitoring the long-term lake variations is important to understand the climatic and anthropogenic impacts on water resources. Global lakes have changed rapidly in the past decades, according to growing evidence (Pham-Duc et al., 2020). However, long-term in situ lake-level data are usually unavailable due to the sparse gauge observations. Remote sensing techniques have demonstrated great potential for monitoring lake-level changes and understanding the driving mechanisms (Song et al., 2015b; Jiang et al., 2019; Mi et al., 2019; Yao et al., 2019; Luo et al., 2021; Xu N. et al., 2022).
In the past few decades, numerous studies have investigated lake water level changes on a regional or global scale via satellite altimeters (Song et al., 2015a, 2023; Cretaux et al., 2016; Jiang et al., 2017; Lei et al., 2017; Qiao et al., 2019; Shu et al., 2020; Chen et al., 2021). Multi-source radar altimetry missions provide lake elevation measurements with a temporal coverage of more than 20 years, such as TOPEX/POSEIDON (1992–2005), ERS-1/2 (1991–2000/1995–2011), Envisat (2002–2012), Jason-1/2/3 (2001–2013/2008-/2016-), CryoSat-2 (2010-), SARAL (2013-), and Sentinel-3 (2016-) (Schwatke et al., 2015; Shen et al., 2020; Feng et al., 2023). Radar altimeters are suitable for monitoring large lakes due to their coarse footprints and large inter-track spacing gaps. In contrast, the Ice, Cloud, and Land Elevation Satellite (ICESat) data have been widely applied to monitor global lakes at a finer scale by benefiting from its small footprint diameter of ~70 m. However, the investigation time period is impeded by the short temporal coverage of ICESat data (2003–2009). Thus, it is rather necessary to seek an approach to synthesizing multiple altimetry datasets for monitoring global lake dynamics. ICESat-2, launched in September 2018, is the follow-on mission of ICESat. Compared to radar altimetry, this satellite has the characteristics of multi-beam and high-resolution photon-counting observation, and its laser footprints are ~17 m and separated by ~0.7 m. Thus, combining the observations of two laser altimetry satellites, ICESat and ICESat-2, can reveal the temporal and spatial change characteristics of global lake levels over the past two decades (Feng et al., 2022; Luo et al., 2022).
However, the accuracy of the synthesized altimetry data from different instrument measurements and potential elevation bias is the major concern for monitoring lake changes. There have been several global-scale lake-level datasets synthesizing multi-source altimeter observations, such as the River and Lake Database (Berry et al., 2005), the Global Reservoir and Lake Monitor (G-REALM) (Birkett et al., 2011), HYDROWEB (Cretaux et al., 2011), and the Database for Inland Waters (DAHITI) (Schwatke et al., 2015). These datasets have been successfully applied to tracking the hydrological dynamics of lakes on regional or global scales (Dubey et al., 2015; Song et al., 2015a; Tan et al., 2017; Luo et al., 2019; Schwatke et al., 2020; Liang et al., 2023). These data products all emphasized the importance of data integration for multi-source radar altimetry measurements (e.g., correction of inconsistent geoid heights). For laser altimetry data processing, a few previous studies have explored the influences of lake water masks on extracting satellite altimetry potential footprints. For example, Xu et al. (2020) analyze the effects of using different buffers to obtain water masks and conclude that the buffer width set at ~100 m for the narrow lake can obtain the highest altimetry accuracy. However, the method to join the two-generation satellite laser measurements has not yet been investigated comprehensively. The inundation extents of most lakes exhibit strong intra-annual and inter-annual variations, and accurately extracting lake elevation points is a critical prerequisite for obtaining reliable water levels. After thoroughly considering the key processes involved in altimetry data processing, three crucial aspects were identified for the joint processing of ICESat and ICESat-2 data: the geoid height, the water mask used to extract altimetry points, and the 9-year data gap between the two satellite missions. Through experimentation, the best method for extracting water levels was selected among these three key aspects. Other potential influencing factors, such as the efficient number of altimetry footprints or the water level data sampling interval, were excluded after initial testing, as their impacts on water level change rates exist in local-scale lakes or can be reduced by the setting of statistical thresholds. Therefore, this study aims to summarize the three important technique notes on water level data processing by integrating the ICESat and ICESat-2 altimetry measurements.
2 Study data
2.1 Lake water level data
2.1.1 Lake water level time series from laser altimetry
The ICESat laser altimeter was launched in January 2003, and its small footprints allow for measuring elevations effectively at a fine scale. The Release-34 GLAH14 product (February 2003–October 2009) of the National Snow and Ice Data Center (NSIDC) is applied in this study. ICESat-2 ATL13, a dataset for inland water surface height, was applied to monitor lake water level changes in 2018–2020. The version-5 datasets of ICESat-2 ATL13 are available at NSIDC.
2.1.2 Radar altimetry dataset for cross-evaluating lake water levels
In this study, the lakes with the HYDROWEB water level data over the period of 2003–2020 were selected for cross-evaluation on the synthesized ICESat/ICESat-2 measurements. HYDROWEB, initiated by LEGOS (Cretaux et al., 2011), is a website that provides water level time series of global lakes, reservoirs, and rivers based on multiple altimetry missions, such as TOPEX/Poseidon, ENVISAT, and Sentinel-3B. In this study, potential instrument biases between different products (ICESat/ICESat-2 data and the validation data) and geoid differences were removed by using the validation data as a reference.
2.1.3 In situ data for lake water level validation
In this study, the available in situ data were applied to validate the water levels of ICESat/ICESat-2. In situ data for Dauphin Lake and Lake Erie were collected from the website of the Government of Canada and the TIDES and CURRENTS websites of the National Oceanic and Atmospheric Administration, respectively. In situ water level data for Qinghai Lake were obtained from Xu F. et al. (2022). The in situ data in this study have been organized to closely match a monthly temporal resolution.
2.2 Lake masks for extracting altimetry footprints
Three types of commonly used water mask data were applied to compare the effects of different water masks on extracting potential altimetry footprints, including the historically maximum water extent, the stationary water body data product (e.g., the HydroLAKES dataset), and time-varying dynamic water inundation masks.
The Global Surface Water (GSW) dataset, which applies Landsat imageries from March 1984 to the present to identify changes in the surface water area, was used to obtain the first type of water mask (Pekel et al., 2016). This study employed surface water occurrences from GSW to map the maximum water extents (all locations ever mapped as water during the observation period) of lakes from 1984 to 2020.
We chose the HydroLAKES dataset, which includes 1,427,688 lakes (>0.1 km2), to obtain the small water mask of lakes at low water levels. Lake polygons (including all attributes) of HydroLAKES are available in a shapefile format (Messager et al., 2016). One of the primary sources of HydroLAKES is the Shuttle Radar Topography Mission (SRTM) Water Body Data, which provided the extent of the water inundation in February 2000. As there are many lakes that have been expanding in the past 20 years (Luo et al., 2022), their water extents from HydroLAKES data can be used as small water masks for conservatively extracting on-lake footprints.
The monthly water frequency data produced by the Global Land Analysis and Discovery Laboratory (Pickens et al., 2020) were used to derive dynamic lake water masks. The water mask corresponding to the ICESat/ICESat-2 measurements data in the same month was chosen to filter on-lake altimetry footprints.
3 Methods
Lake water levels can be obtained from on-lake footprint elevations by an overlay analysis of the lake water mask and altimetry tracks. The averaging elevations of footprints are calculated based on ellipsoid and geoid heights. After obtaining the orthometric height of each observation date, the lake-level change rate is calculated via the linear fitting of the water level time series during the observation periods (e.g., 2003–2009 and 2018–present of ICESat/ICESat-2). Therefore, we focused on investigating the influences of three key data-processing procedures on estimating lake water levels based on ICESat/ICESat-2 observations, including the selection of geoid models, different water masks for filtering footprints, and the 9-year data gap (2010–2018) of water level time series (Figure 1).
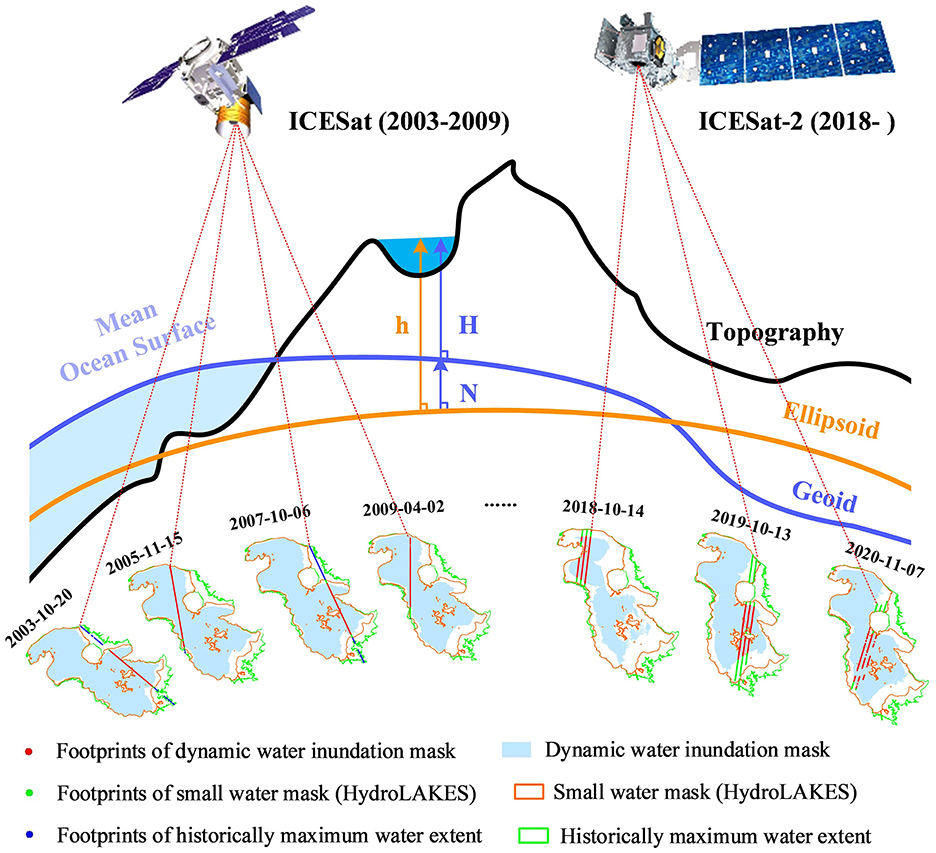
Figure 1. Framework of lake-level calculations, including geoid, different water masks, and the 9-year gap between ICESat/ICESat-2.
As shown in Figure 1, the orthometric height based on the geoid (H) is calculated by subtracting the geoid height relative to the ellipsoid system (N) from the ellipsoid height (h). The geoid is the shape that the ocean surface would take under the Earth's gravity without considering other effects such as winds and tides. This surface is recombined with the mean ocean surface and extends into the continent's interior (Gauss, 1828). The EGM, published by the National Geospatial-Intelligence Agency (NGA), is a series of geopotential models of the Earth. The EGM reference frames include EGM84, EGM96, EGM2008, and EGM2020 (Barnes et al., 2015). Both EGM96 and EGM2008 are the commonly used geoid reference systems. Therefore, the geoids of these two reference systems are adopted and compared in this study.
The procedure for obtaining the long-term lake water levels based on ICESat/ICESat-2 is summarized as follows. First, water masks were used to extract potential lake footprints from the laser altimeter. Second, the normalized median absolute deviation (NMAD) method was applied to remove the errors from the clouds, lake surface waves, snow, or saturated reflected signals (Hoehle and Hoehle, 2009). Data processing using the NMAD method includes the following steps: (1) calculating the median of all water levels within 1 day, (2) computing the absolute deviations of each factor from the median value, (3) determining the median of these absolute deviations (MAD), and (4) multiplying by a constant of 1.4826, and removing water levels that exceed the range ([median-MAD, median+MAD]). We excluded lake levels with fewer than five altimetry footprints on each observation date to reduce the height bias derived from insufficient measurement times. To further eliminate potential outliers in water levels, we tested the water level processing results of multiple lakes and chose a threshold of 0.3 m. Then, lakes with the water level standard deviation of each observation date larger than 0.3 m (depending on the uncertainty range relative to lake-level changes) were also removed. Third, we computed the lake water level on each observation date by averaging the remainder of the footprint elevations. Finally, the water level change rate based on the time series data was obtained via the robust fitting method (Holland and Welsch, 1977).
4 Results and analyses
4.1 The effect of geoid selection on estimating lake levels and change rates
Orthometric height is approximated by subtracting geoid height from ellipsoid height. An accurate geoid model is needed for converting ellipsoidal heights to orthometric heights. Therefore, the main function of the geoid is deemed to be a reference surface for the water level in geodesy, and the geoid heights at different locations of one lake may be quite different (Li and Gotze, 2001). This height difference is more obvious in large water bodies because large lakes span more spatial domains. Thus, we chose Lake Erie (25,768 km2), one of the Great Lakes in North America, as the research case to investigate the effect of geoid on lake water levels. Then, we considered four scenarios of the geoid reference, including no use of the geoid (1), default geoid heights contained in the NASA data product (2), recalculation of geoid by the EGM96 model (3), or EGM2008 model (4). The EGM2008/EGM96 geoid heights were recalculated based on the longitude and latitude of each footprint by using the NGA tool. To validate the integration of ICESat and ICESat-2 under various scenarios, we compared the time-series waveforms and water level change rates with those of in situ water level data.
The water level time series in the four scenarios show that the lake levels without considering geoid height are significantly disorganized (Figure 2A), and the seasonal signal of water level is not reasonable in both ICESat and ICESat-2 periods (Figure 2B). As shown in Figures 2A, C, there is an obvious systematic deviation between the ICESat and ICESat-2 water levels (based on the default EGM 2008 geoids provided by the ICESat and ICESat-2 products). Therefore, the water level change rate is obviously overestimated based on the original geoid of the product. From Figures 2D–F, the water levels based on the EGM2008 and EGM96 geoids have comparable change patterns and the same water level change rate, which is rather consistent with the in situ data. According to the user guidebook for ICESat and ICESat-2 raw products, although both of the datasets were processed with the EGM2008 geoids, there is a systematic bias when combining the two-time series (2003–2009 and 2018–present). It could be due to the inconsistent usage of different spherical harmonic coefficients of the EGM2008 model applied in ICESat and ICESat-2 products (not stated in the product manual). In previous studies, deviations in the water levels of some lakes on the Tibetan Plateau were also mentioned. Zhang et al. (2019) mentioned that for Qinghai Lake, ICESat aligns well with in situ measurements, and the lake level derived from HYDROWEB is slightly higher than both ICESat and station observations. In the case of Selin Co and Nam Co, the elevations from ICESat/ICESat-2 are slightly higher than those from HYDROWEB, possibly due to altimeter instrument biases and geoid variations. Therefore, it is important to obtain orthometric heights via a consistent geoid model, and it is necessary to recalculate geoids when obtaining lake water levels from ICESat/ICESat-2.
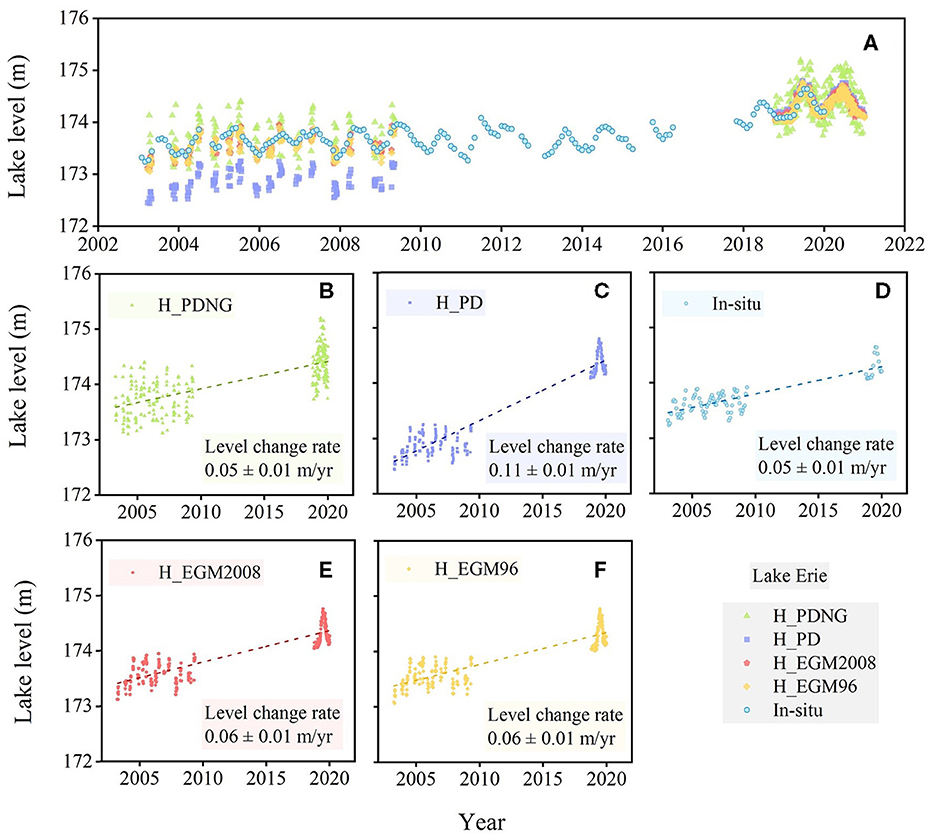
Figure 2. Time series of water levels under four different scenarios and comparison with in situ data (A). H_PDNG: no use of the geoid (B), H_PD: the water level based on the product-default geoid (C), the in situ water level (D), H_EGM2008: the water level based on recalculated geoid by EGM2008 (E), and H_EGM96: the water level based on recalculated geoid by EGM96 (F).
4.2 The effect of water mask on estimating lake levels and change rates
Lake levels are calculated generally by averaging on-lake footprints filtered by water masks. The water mask selection is a key step that affects altimetry accuracy, especially for lakes with remarkable changes in water inundation extent. Due to limitations such as the scarcity of hydrological monitoring stations, remote locations, and constraints related to data politics, obtaining long-term and large-scale in situ data on water levels is challenging, particularly for small lakes. Radar altimetry satellites, with footprints ranging from 0.3 to 8 km, are well-suited for long-term water level monitoring of large lakes. However, their coverage is limited to medium- and small-sized lakes. Small lakes are difficult to monitor with in situ data or radar altimetry satellite datasets, but reference data are needed for lake water level verification. It only makes sense to discuss using different time periods of lake inundation extents as masks for extracting potential water levels when the lake area changes are significant. Therefore, we chose a large lake (Lake Urmia in Central Asia) with obvious inundation area changes as the study case, to compare the effect of different water masks on the lake-level estimates. Lake Urmia is an endorheic salty lake in Iran, and its area was ~4,600 km2 in 2000. Persistent drought and groundwater pumping cause the continuous shrinkage of the lake. Thus, we set three different types of water masks historically maximum water extent, a small water mask (HydroLAKES data, representing the status of ~2000), and a monthly dynamic inundation mask. The historically maximum water extent may obtain as many observation opportunities as possible and add the observation times for some lakes. The small water mask (e.g., HydroLAKES data) has the advantages of easy access and a large number of lakes. The conservative water mask makes it easy to eliminate the outliers of water level data in the following process. The dynamic water masks can track the expansion or shrinkage of the lake and avoid including the “polluted” footprints of the lakeshore. There is a trade-off between the temporal-spatial resolution of the water level and the efficiency of data processing in the mask choice. Therefore, it is important to compare the water level time series and water level change rate under the three mask scenarios. In this study, we calculated the orthometric heights from ICESat/ICESat-2 data for each type of mask based on the recalculated geoid (EGM2008) and the WGS84. We validated the integration of ICESat and ICESat-2 data under different water masks by comparing the absolute difference in water levels and change rate with validation data. The water level data obtained from three different masks have all been processed to remove outliers using the NAMD method. During the satellite mission periods (2003–2009 and 2018–2020), the historically maximum water extent, small water mask, and dynamic water inundation mask for Lake Urmia derived observations 110 times (717,701 footprints), 109 times (710,340 footprints), and 106 times (584,666 footprints), respectively (Table 1). The comparison of the water level change rate shows that the result from maximum water extent (−0.17 ± 0.01 m/year in Figure 3B) is the most deviated from the reference value (−0.14 ± 0.01 m/year in Figure 3E). The results from the small water mask and dynamic water inundation mask are roughly consistent (Figure 3).

Table 1. Statistics of lake-level monitoring results from three water masks in the period of 2003–2009 and 2018–2020 in Lake Urmia.
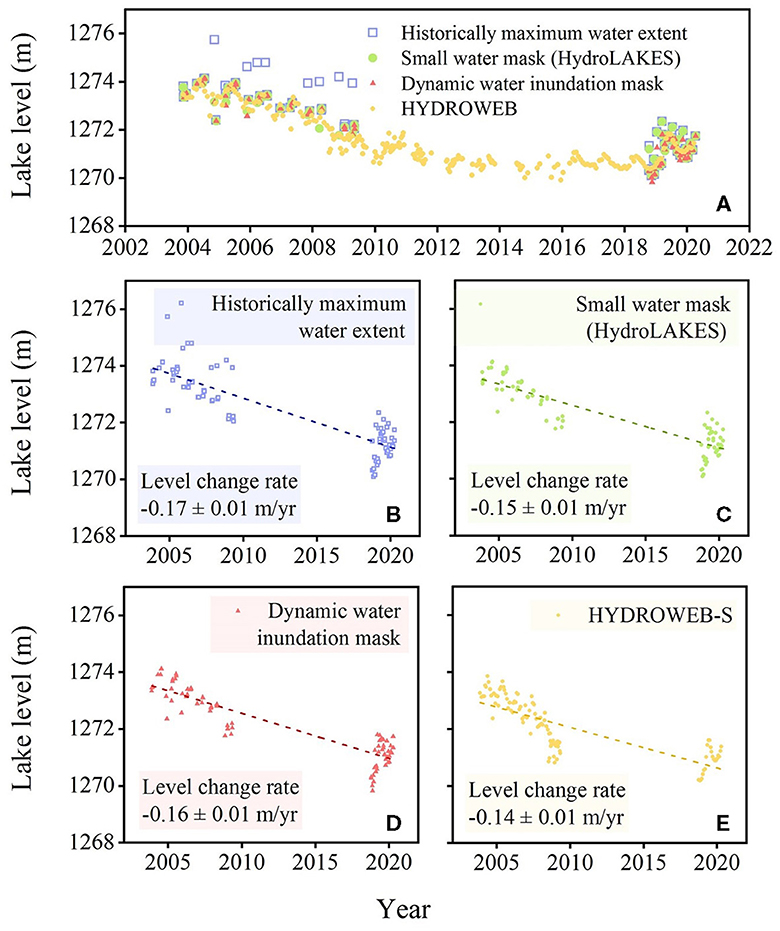
Figure 3. (A–E) Time series of water levels under three different water masks. HYDROWEB-S (e) means the sparse HYDROWEB based water level time series. The intermediate period is removed, and the monitoring period is consistent with ICESat/ICESat-2.
Furthermore, we compared the number of footprints and water levels from the three masks on the same observation date in Lake Urmia. The results under three different mask scenarios show that footprints derived from historically maximum water extent are nearly twice the number from small water masks and dynamic water inundation masks (Table 2; Figure 4). Specifically, the water level from the maximum water extent (1,274.61 m) is far from the reference value (1,272.69 m) under the same geographical reference system (see), while the water level results acquired in October 2005 based on the HydroLAKES lake mask (1,272.79 m) and dynamic mask (1,272.57 m) were basically consistent. The absolute difference between the water level results of three water masks and the reference value (HYDROWEB) is 1.92, 0.10, and 0.12 m, respectively.

Table 2. Statistics of lake-level monitoring results from three water masks on the same observation date in Lake Urmia.
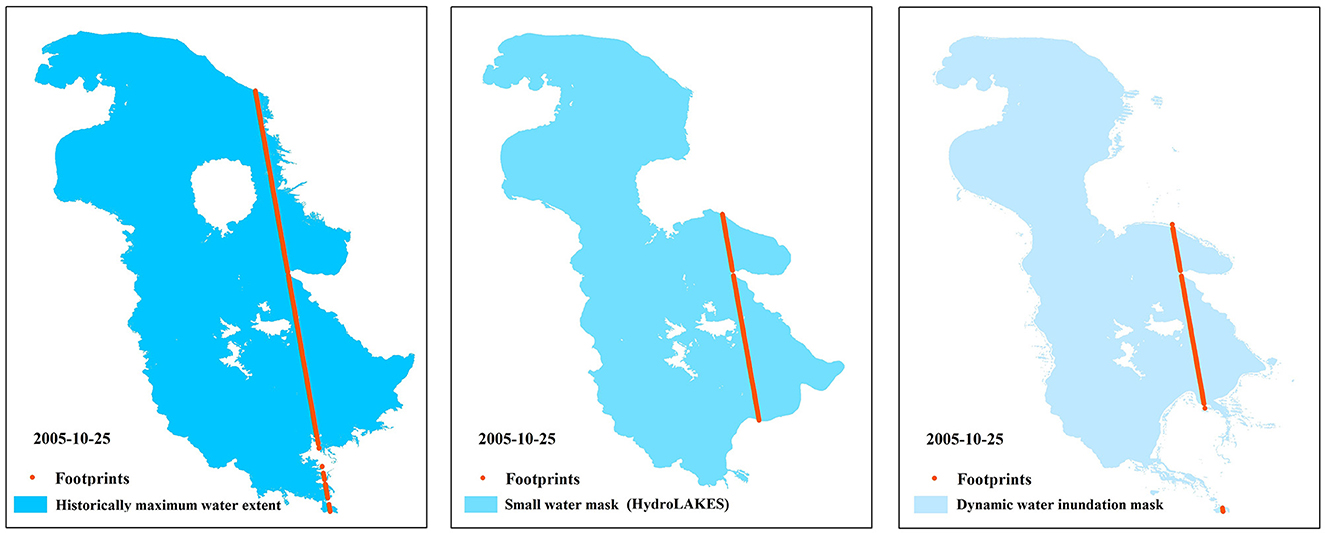
Figure 4. Altimetry footprints of Lake Urmia by three different water masks based on the observations carried out on October 25, 2005.
4.3 The effect of the 9-year data gap on estimating lake levels and change rates
The advantages of ICESat and ICESat-2 mainly lie in their capability to monitor small and medium-sized lakes with high accuracy. Combining the two generations of laser altimetry satellites can achieve the two-decade monitoring of ~7,000 lakes over 10 km2 globally (Luo et al., 2022). However, there is a 9-year data gap between the two satellites from 2010 to 2018, which inevitably induces some uncertainties in estimating the water level change rates. Therefore, to quantify the influence of the 9-year data gap on the water level change rate, we selected lakes with different time-series patterns for comparison. Considering that different lakes are experiencing varying impacts due to the data gap, we selected lakes with relatively stable water levels, seasonal fluctuations, and drastic inter-annual or decadal trending changes. Correspondingly, Dauphin Lake, Garabogazkol Lake, Hulun Lake, Qinghai Lake, and Migriggyangzham Co were selected as the study cases for investigating the effect of the 9-year data gap on estimating the water level change rate. We applied the HydroLAKES dataset, a small water mask, to extract the footprints and finally obtained the water level time series referred to as EGM2008 and WGS84. We assessed the influence of the 9-year data gap on deriving water level time series through the integration of ICESat and ICESat-2 data by comparing water level change rates with validation data. The results indicate that the correlation between the validation data and ICESat/ICESat-2 data for the five lakes is consistently above 0.99. The average absolute error between these five datasets and the validation data is 0.01 m/year, which is lower than the typical calculation error for water level change rates. The specific comparison results are shown in Figure 5. For the relatively stable water level pattern in 2010–2018, such as Dauphin Lake, or the water level pattern in Garabogazkol Lake with seasonal fluctuation, the 9-year data gap had a limited effect on the change rate estimation. For the water level pattern with large fluctuations in 2010–2018 such as Hulun Lake, the data gap led to an obvious underestimation of the results. For the water level pattern with small fluctuations in 2010–2018, such as Qinghai Lake, the data gap caused an overestimation of the water level change rate. For the water level pattern with a near-linear increase in 2010-2018, such as Migriggyangzham Co, there is little effect on the change rate estimation.
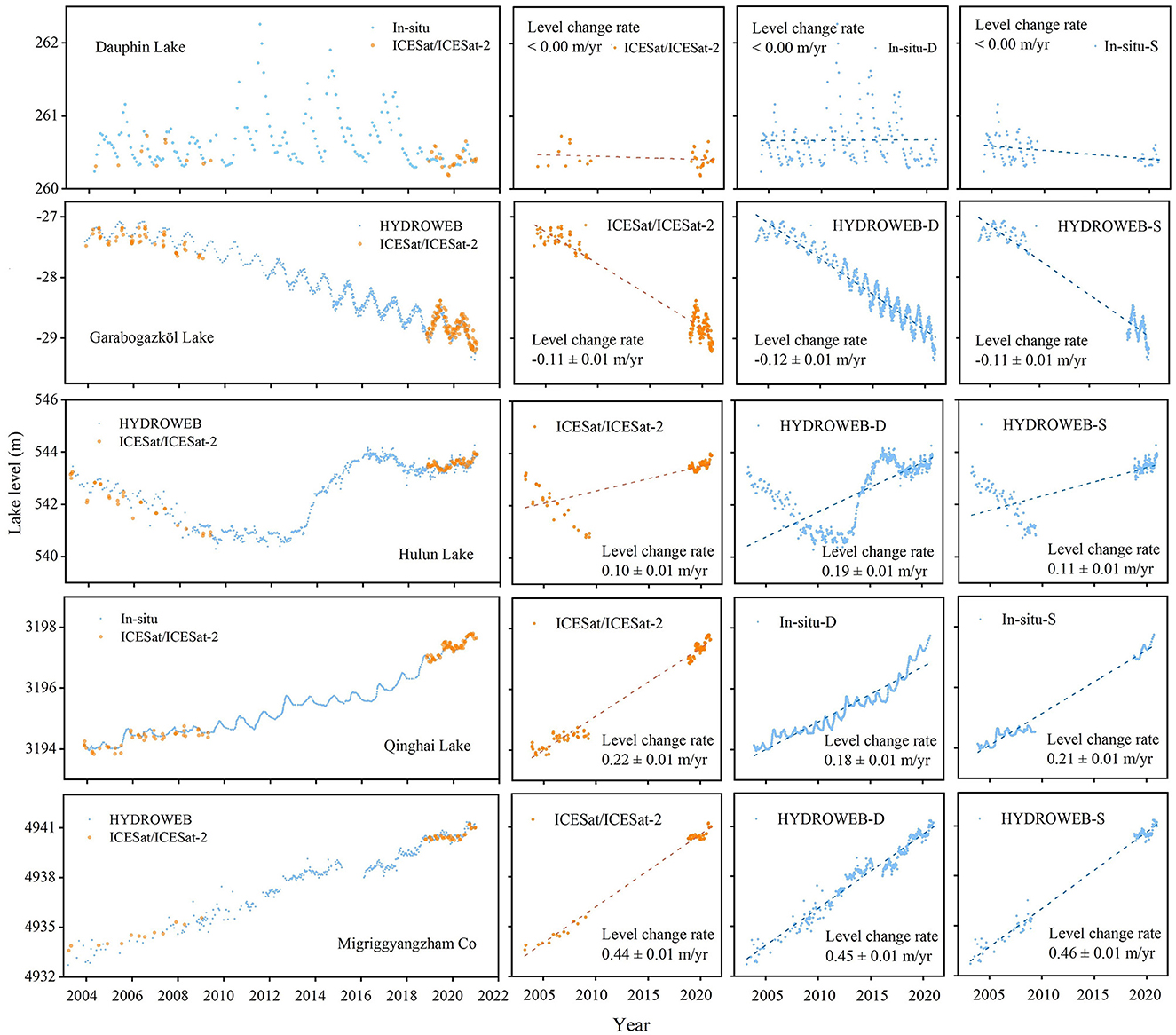
Figure 5. Effect of a 9-year data gap on the water level change rate from lakes with different water level change patterns. Dauphin Lake, Garabogazkol Lake, Hulun Lake, Qinghai Lake, and Migriggyangzham Co represent the stable, seasonal, large, small, and linear fluctuation patterns of water levels in 2003–2020, respectively.
5 Summary and concluding remarks
The development of satellite laser altimetry opens new possibilities for monitoring global lake levels at a finer scale. The joint use of ICESat and ICESat-2 laser altimetry data can facilitate the near two-decade detection of lake water level changes. In this study, we summarize three primary data-processing procedures (geoid height, water mask for extracting potential footprints, and the 9-year data gap between the two generations of laser altimetry satellites) impacting lake-level estimates when integrating the ICESat and ICESat-2 altimetry measurements. The major conclusions and suggestions are organized as follows.
Geodetic reference needs to be considered when constructing the time series of lake water levels. We suggest recalculating the geoid heights of ICESat and ICESat-2 altimetry data using the consistent geoid model (e.g., EGM2008 and EGM96) rather than the default geoid heights of product data. The comparison results of different water masks for extracting altimetry footprints showed that the maximum water extent would introduce larger errors, while the results based on the dynamic mask and the small water mask are basically comparable. However, the mapping of dynamic water masks is a laborious task, especially for the investigation of lakes at regional or global scales. The smaller water mask is recommended to extract the potential on-lake altimetry footprints when the lake is at lower water level. The lake mask should be selected according to the different hydrological characteristics of the study regions. For example, for the expanding lakes on the Tibetan Plateau, it is suitable to choose the water extent around the year 2000 to extract the on-lake footprints during the period 2003–2020. However, in Central Asia, where most lakes are shrinking, it would be better to obtain water extents derived from more recent satellite imagery.
The 9-year data gap between ICESat and ICESat-2 observations may result in an overestimation or underestimation of the water level change rate for lakes with non-linear expansion/contraction. However, it has a limited effect on lakes with (near-) linear change or seasonal fluctuation. Thus, it is very crucial to develop methods for filling the data gap. For example, we can establish the lake hypsometric curve by fitting the lake area and water level changes and reconstructing the missing water levels from the consecutive time series of lake areas. In addition, integration of the water levels from other altimetry satellites, such as CryoSat-2 (2010–present), is also an effective solution. However, this method is more suitable for large lakes due to the coarser footprints of CryoSat-2. In addition, overlaying lake shorelines on DEMs can be applied to extract lake levels for supplementing the data gap. It should be mentioned that there could be other possible data-processing procedures affecting the accuracy of lake level and change rate estimates when integrating ICESat and ICESat-2 data, for instance, the linear fitting method, data sampling intervals (e.g., the monthly or seasonal data composite), and methods of removing height outliers. All of these procedures should be considered cautiously for generating accurate lake-level estimates from the altimetry satellite observations.
Data availability statement
The data used in the study can be found in the https://doi.org/10.6084/m9.figshare.24456358.v1.
Author contributions
SL: Data curation, Methodology, Visualization, Writing – original draft. CS: Funding acquisition, Project administration, Supervision, Writing – review & editing.
Funding
The author(s) declare financial support was received for the research, authorship, and/or publication of this article. This study was supported by the National Key Research and Development Program of China (Grant Nos. 2022YFF0711603 and 2019YFA0607101), the Strategic Priority Research Program of the Chinese Academy of Sciences (Grant Nos. XDA23100102 and XDA28020503), and the National Natural Science Foundation of China (Grant Nos. 41971403 and 41801321).
Acknowledgments
We are grateful to the National Snow and Ice Data Center for providing ICESat/ICESat-2 data (https://nsidc.org/data/icesat, https://nsidc.org/data/atl13/versions/5), LEGOS for providing lake-level data (http://hydroweb.theia-land.fr/), the website Government of Canada for providing in situ data of Dauphin Lake (https://wateroffice.ec.gc.ca/mainmenu/historical_data_index_e.html), the website TIDES and CURRENTS for providing in situ data of Lake Erie (https://tidesandcurrents.noaa.gov/stations.html?type=Water+Levels), the Global Land Analysis and Discovery Laboratory for providing the monthly water frequency data (https://glad.umd.edu/dataset/global-surface-water-dynamics), Messager for providing the small water mask of the lakes (https://www.hydrosheds.org/page/hydrolakes), the Joint Research Center for providing the maximum water extent of the lakes (https://global-surface-water.appspot.com/), and the National Geospatial-Intelligence Agency for providing a tool of calculating geoid heights (https://earth-info.nga.mil/).
Conflict of interest
The authors declare that the research was conducted in the absence of any commercial or financial relationships that could be construed as a potential conflict of interest.
Publisher's note
All claims expressed in this article are solely those of the authors and do not necessarily represent those of their affiliated organizations, or those of the publisher, the editors and the reviewers. Any product that may be evaluated in this article, or claim that may be made by its manufacturer, is not guaranteed or endorsed by the publisher.
References
Barnes, D., Factor, J. K., Holmes, S. A., Ingalls, S., Presicci, M. R., Beale, J., et al. (2015). Earth Gravitational Model 2020. American Geophysical Union Fall meeting 2015 abstract id. G34A–03.
Berry, P. a. M., Garlick, J. D., Freeman, J. A., and Mathers, E. L. (2005). Global inland water monitoring from multi-mission altimetry. Geophys. Res. Lett. 32, 814. doi: 10.1029/2005GL022814
Birkett, C., Reynolds, C., Beckley, B., and Bradley, D. (2011). From research to operations: the USDA global reservoir and lake monitor. Coastal Altimetry 19–50.
Chen, T., Song, C., Ke, L., Wang, J., Liu, K., Wu, Q., et al. (2021). Estimating seasonal water budgets in global lakes by using multi-source remote sensing measurements. J. Hydrol. 593, 125781. doi: 10.1016/j.jhydrol.2020.125781
Cretaux, J. F., Abarca-Del-Rio, R., Berge-Nguyen, M., Arsen, A., Drolon, V., Clos, G., et al. (2016). Lake volume monitoring from space. Surv. Geophys. 37, 269–305. doi: 10.1007/s10712-016-9362-6
Cretaux, J. F., Jelinski, W., Calmant, S., Kouraev, A., Vuglinski, V., Berge-Nguyen, M., et al. (2011). SOLS: a lake database to monitor in the Near Real Time water level and storage variations from remote sensing data. Adv. Space Res. 47, 1497–1507. doi: 10.1016/j.asr.2011.01.004
Dubey, A. K., Gupta, P. K., Dutta, S., and Singh, R. P. (2015). An improved methodology to estimate river stage and discharge using Jason-2 satellite data. J. Hydrol. 529, 1776–1787. doi: 10.1016/j.jhydrol.2015.08.009
Feng, Y., Yang, L., Zhan, P., Luo, S., Chen, T., Liu, K., et al. (2023). Synthesis of the ICESat/ICESat-2 and CryoSat-2 observations to reconstruct time series of lake level. Int. J. Digital Earth 16, 183–209. doi: 10.1080/17538947.2023.2166134
Feng, Y., Zhang, H., Tao, S., Ao, Z., Song, C., Chave, J., et al. (2022). Decadal lake volume changes (2003–2020) and driving forces at a global scale. Remote Sens. 14, 1032. doi: 10.3390/rs14041032
Gauss, C. F. (1828). Bestimmung des Breitenunterschiedes zwischen den Sternwarten von Göttingen und Altona: durch Beobachtungen am Ramsdenschen Zenithsector. Bei Vandenhoeck und Ruprecht.
Hoehle, J., and Hoehle, M. (2009). Accuracy assessment of digital elevation models by means of robust statistical methods. ISPRS J. Photogram. Remote Sens. 64, 398–406. doi: 10.1016/j.isprsjprs.2009.02.003
Holland, P. W., and Welsch, R. E. (1977). Robust regression using iteratively re-weighted least-squares. Commun. Statis. A Theory Methods 6, 813–827. doi: 10.1080/03610927708827533
Jiang, L., Andersen, O. B., Nielsen, K., Zhang, G., and Bauer-Gottwein, P. (2019). Influence of local geoid variation on water surface elevation estimates derived from multi-mission altimetry for Lake Namco. Remote Sens. Environ. 221, 65–79. doi: 10.1016/j.rse.2018.11.004
Jiang, L., Nielsen, K., Andersen, O. B., and Bauer-Gottwein, P. (2017). Monitoring recent lake level variations on the Tibetan Plateau using CryoSat-2 SARIn mode data. J. Hydrol. 544, 109–124. doi: 10.1016/j.jhydrol.2016.11.024
Lei, Y., Yao, T., Yang, K., Sheng, Y., Kleinherenbrink, M., Yi, S., et al. (2017). Lake seasonality across the Tibetan Plateau and their varying relationship with regional mass changes and local hydrology. Geophys. Res. Lett. 44, 892–900. doi: 10.1002/2016GL072062
Li, X., and Gotze, H. J. (2001). Ellipsoid, geoid, gravity, geodesy, and geophysics. Geophysics 66, 1660–1668. doi: 10.1190/1.1487109
Liang, X., Song, C., Liu, K., Chen, T., and Fan, C. (2023). Reconstructing centennial-scale water level of large Pan-Arctic lakes using machine learning methods. J. Earth Sci. 34, 1218–1230. doi: 10.1007/s12583-022-1739-5
Luo, S., Song, C., Ke, L., Zhan, P., Fan, C., Liu, K., et al. (2022). Satellite laser altimetry reveals a net water mass gain in global lakes with spatial heterogeneity in the early 21st century. Geophys. Res. Lett. 49, 676. doi: 10.1029/2021GL096676
Luo, S., Song, C., Liu, K., Ke, L., and Ma, R. (2019). An effective low-cost remote sensing approach to reconstruct the long-term and dense time series of area and storage variations for large lakes. Sensors 19, 4247. doi: 10.3390/s19194247
Luo, S., Song, C., Zhan, P., Liu, K., Chen, T., Li, W., et al. (2021). Refined estimation of lake water level and storage changes on the Tibetan Plateau from ICESat/ICESat-2. Catena 200, 105177. doi: 10.1016/j.catena.2021.105177
Messager, M. L., Lehner, B., Grill, G., Nedeva, I., and Schmitt, O. (2016). Estimating the volume and age of water stored in global lakes using a geo-statistical approach. Nat. Commun. 7, 13603. doi: 10.1038/ncomms13603
Mi, H., Fagherazzi, S., Qiao, G., Hong, Y., and Fichot, C. G. (2019). Climate change leads to a doubling of turbidity in a rapidly expanding Tibetan lake. Sci. Total Environ. 688, 952–959. doi: 10.1016/j.scitotenv.2019.06.339
Pekel, J.- F., Cottam, A., Gorelick, N., and Belward, A. S. (2016). High-resolution mapping of global surface water and its long-term changes. Nature 540, 418. doi: 10.1038/nature20584
Pham-Duc, B., Sylvestre, F., Papa, F., Frappart, F., Bouchez, C., Cretaux, J. F., et al. (2020). The Lake Chad hydrology under current climate change. Sci. Rep. 10, 5498. doi: 10.1038/s41598-020-62417-w
Pickens, A. H., Hansen, M. C., Hancher, M., Stehman, S. V., Tyukavina, A., Potapov, P., et al. (2020). Mapping and sampling to characterize global inland water dynamics from 1999 to 2018 with full Landsat time-series. Remote Sens. Environ. 243, 111792. doi: 10.1016/j.rse.2020.111792
Qiao, B., Zhu, L., Wang, J., Ju, J., Ma, Q., Huang, L., et al. (2019). Estimation of lake water storage and changes based on bathymetric data and altimetry data and the association with climate change in the central Tibetan Plateau. J. Hydrol. 578, 124052. doi: 10.1016/j.jhydrol.2019.124052
Schwatke, C., Dettmering, D., Bosch, W., and Seitz, F. (2015). DAHITI - an innovative approach for estimating water level time series over inland waters using multi-mission satellite altimetry. Hydrol. Earth Syst. Sci. 19, 4345–4364. doi: 10.5194/hess-19-4345-2015
Schwatke, C., Dettmering, D., and Seitz, F. (2020). Volume variations of small inland water bodies from a combination of satellite altimetry and optical imagery. Remote Sens. 12, 1606. doi: 10.3390/rs12101606
Shen, M., Duan, H., Cao, Z., Xue, K., Qi, T., Ma, J., et al. (2020). Sentinel-3 OLCI observations of water clarity in large lakes in eastern China: Implications for SDG 6, 3.2 evaluation. Remote Sens. Environ. 247, 111950. doi: 10.1016/j.rse.2020.111950
Shu, S., Liu, H., Beck, R. A., Frappart, F., Korhonen, J., Xu, M., et al. (2020). Analysis of Sentinel-3 SAR altimetry waveform retracking algorithms for deriving temporally consistent water levels over ice-covered lakes. Remote Sens. Environ. 239. 111643. doi: 10.1016/j.rse.2020.111643
Song, C., Huang, B., and Ke, L. (2015a). Heterogeneous change patterns of water level for inland lakes in High Mountain Asia derived from multi-mission satellite altimetry. Hydrol. Proc. 29, 2769–2781. doi: 10.1002/hyp.10399
Song, C., Ye, Q., Sheng, Y., and Gong, T. (2015b). Combined ICESat and cryoSat-2 altimetry for accessing water level dynamics of Tibetan lakes over 2003-2014. Water 7, 4685–4700. doi: 10.3390/w7094685
Song, L., Song, C., Luo, S., Chen, T., Liu, K., Zhang, Y., et al. (2023). Integrating ICESat-2 altimetry and machine learning to estimate the seasonal water level and storage variations of national-scale lakes in China. Remote Sens. Environ. 294, 113657. doi: 10.1016/j.rse.2023.113657
Tan, C., Ma, M., and Kuang, H. (2017). Spatial-temporal characteristics and climatic responses of water level fluctuations of global major lakes from 2002 to 2010. Remote Sens. 9, 20150. doi: 10.3390/rs9020150
Xu, F., Zhang, G., Yi, S., and Chen, W. (2022). Seasonal trends and cycles of lake-level variations over the Tibetan Plateau using multi-sensor altimetry data. J. Hydrol. 604, 127251. doi: 10.1016/j.jhydrol.2021.127251
Xu, N., Ma, Y., Wei, Z., Huang, C., Li, G., Zheng, H., et al. (2022). Satellite observed recent rising water levels of global lakes and reservoirs. Environ. Res. Lett. 17, 074013. doi: 10.1088/1748-9326/ac78f8
Xu, Y., Gu, S., Yin, Z., Lin, J., Zhao, J., Zhu, X., et al. (2020). Obtaining accurate water level measurements in lakes: analysis of changes in ICESat altimetry accuracy with buffer changes. IEEE Access 8, 81090–81100. doi: 10.1109/ACCESS.2020.2991228
Yao, F., Wang, J., Wang, C., and Cretaux, J.- F. (2019). Constructing long-term high-frequency time series of global lake and reservoir areas using Landsat imagery. Remote Sens. Environ. 232, 111210. doi: 10.1016/j.rse.2019.111210
Keywords: ICESat, ICESat-2, lake, satellite altimetry, water level
Citation: Luo S and Song C (2023) Uncertainties on the combined use of ICESat and ICESat-2 observations to monitor lake levels. Front. Water 5:1279444. doi: 10.3389/frwa.2023.1279444
Received: 18 August 2023; Accepted: 07 November 2023;
Published: 01 December 2023.
Edited by:
Jahangeer Jahangeer, University of Nebraska-Lincoln, United StatesReviewed by:
Mohamed Mwabumba, Nelson Mandela African Institution of Science and Technology, TanzaniaApoorv Verma, Indian Institute of Technology Roorkee, India
Copyright © 2023 Luo and Song. This is an open-access article distributed under the terms of the Creative Commons Attribution License (CC BY). The use, distribution or reproduction in other forums is permitted, provided the original author(s) and the copyright owner(s) are credited and that the original publication in this journal is cited, in accordance with accepted academic practice. No use, distribution or reproduction is permitted which does not comply with these terms.
*Correspondence: Chunqiao Song, Y3Fzb25nQG5pZ2xhcy5hYy5jbg==