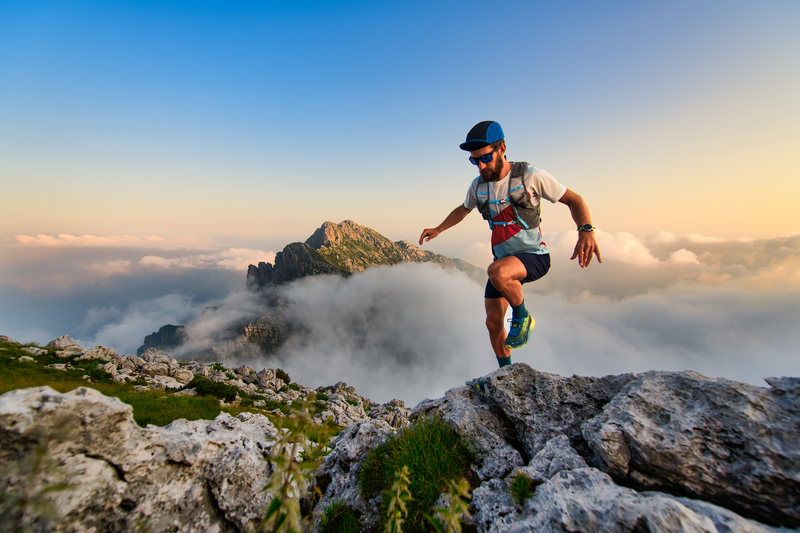
95% of researchers rate our articles as excellent or good
Learn more about the work of our research integrity team to safeguard the quality of each article we publish.
Find out more
ORIGINAL RESEARCH article
Front. Virtual Real. , 02 April 2025
Sec. Virtual Reality and Human Behaviour
Volume 6 - 2025 | https://doi.org/10.3389/frvir.2025.1497237
This article is part of the Research Topic Impact of Virtual Reality on Sensory Neuroscience: Delving into Body Ownership and Agency View all articles
Concepts of spatial navigation rest on the idea of landmarks, which are immobile features or objects in the environment. However, behaviorally relevant objects or fellow humans are often mobile. This raises the question of how the presence of human agents influences spatial exploration and knowledge acquisition. Here, we investigate exploration and performance in subsequent spatial tasks within a virtual environment containing numerous human avatars. In the exploration phase, agents had a locally limited effect on navigation. They prompted participants to revisit locations with agents during their initial exploration without significantly altering overall exploration patterns or the extent of the area covered. However, agents and buildings competed for visual attention. When spatial recall was tested, pointing accuracy toward buildings improved when participants directed their attention to the buildings and nearby agents. In contrast, pointing accuracy for agents showed weaker performance and did not benefit from visual attention directed toward the adjacent building. Contextual agents and incongruent agent-environment pairings further enhanced pointing accuracy, revealing that violations of expectations by agents can significantly shape navigational knowledge acquisition. Overall, agents influenced spatial exploration by directing attention locally, with the interaction between agent salience and environmental features playing a key role in shaping navigational knowledge acquisition.
Spatial navigation is essential for goal-oriented movement and active environmental interaction (Epstein et al., 2017; Ito et al., 2015). In humans, regular engagement in spatial navigation, whether studied through navigation done in the context of professional activities (Griesbauer et al., 2022; Maguire et al., 2006; Woollett and Maguire, 2011), targeted training (Choi et al., 2012), or virtual environments (West et al., 2017), is related to the enhancement of cognitive functions, particularly memory and spatial awareness. This intricate link between spatial navigation and cognitive processes highlights the crucial role of spatial navigation in developing and organizing spatial knowledge.
The transformation of navigational experiences into spatial knowledge begins with recognizing key environmental elements, followed by their gradual integration into a cohesive reference system (Ekstrom and Isham, 2017). When individuals explore new surroundings freely, they can identify elements that aid their orientation and harness them as building blocks for knowledge construction, such as remembering shops when visiting a new town. This process, known as landmark identification, is essential for maintaining positional awareness and planning future pathways (Janzen et al., 2006). Spatial knowledge encompasses recognizing and recalling critical elements of the landscape, understanding their spatial relationships, and the routes connecting them.
Despite extensive research on the role of static landmarks in spatial cognition (Malanchini et al., 2020), the dynamic human aspects influencing spatial exploration and knowledge acquisition have yet to be fully explored. Often, humans are viewed primarily as modifiers of navigational paths rather than as active contributors to spatial knowledge acquisition (Bicanski and Burgess, 2020; Ekstrom and Isham, 2017). This perspective overlooks the significant role other individuals play in real-world spatial cognition. Encounters with others can impact pedestrian dynamics (Dalton et al., 2019), influence visual exploration (Gert et al., 2020), provide vital information about the safety and usability of spaces (Bajorunaite et al., 2022), affect the recall of locations (Kuehn et al., 2018), and prompt a parallel social mapping of the environment (Schafer and Schiller, 2018). Thus, incorporating other humans into navigation research is essential for a deeper understanding of spatial cognition.
However, studying the influence of fellow humans on concrete spatial knowledge faces the difficulties of maintaining a navigation scenario of real-world scale in controlled environments. When available, researchers often conduct retrospective studies using real-world data, such as mobile phone and GPS data, which can characterize human mobility patterns but lack the controlled variables necessary for isolating specific factors mediating the results (Pappalardo et al., 2015; Schläpfer et al., 2021). Moreover, these studies do not link exploration patterns to the future acquisition of spatial knowledge, hindering a comprehensive understanding of cognitive processes derived from navigation patterns. When moving to the other side of the spectrum, laboratory setups tend to be bound to small-scale environments and simplified tasks that lack the challenge of spatial scales of the real world (Wiener et al., 2020). Participants are asked to use humans as anchors inside a maze-like virtual reality (VR) or as landmarks in visual flow experiments (Kuehn et al., 2018). Dalton et al. (2019) point out that VR technologies in wayfinding research often exclude the presence of others, thereby underestimating their importance. They suggest that the mere presence of others (i.e., weak social cues), even without active interaction, can help infer the importance of a space within its environment, and actively call for more studies to be done in this realm of research. Hence, it is acknowledged that examining how the mere presence of others might impact navigation and knowledge acquisition when humans are not primary targets is essential but remains unstudied.
With this work, we raise the question of whether spatially relevant information can be extracted from observing other humans in the space around us. It is important to note that humans do not inherently serve as fixed landmarks in natural navigation, primarily due to their mobility, preventing them from forming intrinsic associations with specific places. However, rather than considering other humans as reliable reference points, we explore the notion that their relevance within a given context can be harnessed as a source of information to enhance spatial knowledge. Essentially, it is not the presence of others per se that aids in spatial cognition but rather how they interact with the elements in their surroundings that could be relevant for spatial exploration and knowledge acquisition. This prompts us to investigate what minimal change in a human agent’s interaction with the environment can elicit participants’ different behavioral responses. We seek to understand whether these human elements act as distractions, potentially diminishing the saliency of the surrounding stimuli, or if, conversely, they contribute to improved performance by anchoring individuals to a more vivid mental representation of specific locations.
To address this complexity, we investigated how spatial knowledge acquisition developed in a controlled one-square-kilometer VR environment with human agents. The study incorporated human agents at two levels: one in which the agent interacted with the environment by holding an object relevant to the context, such as a toolbox in front of a hardware store (i.e., Contextual agent), and another in which the agent simply stood without interacting with any objects around it (i.e., Acontextual agent). These agents were placed in front of public buildings, such as stores, basketball courts, restaurants, or residential buildings. For our first group of participants, all contextual agents were placed in front of public buildings that matched their object interaction. For the second group, we disrupted this congruency to study the sole influence of the type of agent and their building on the context in which they are situated, under the hypothesis that having context-congruent agents will enhance the participant’s ability to recall. Specifically, we propose three hypotheses: 1) the Visit Hypothesis, which states that contextual agents will influence participants’ exploration patterns by drawing them back to previously visited locations; 2) the Dwell Hypothesis, which posits that participants will allocate more visual attention to contextually congruent agents compared to acontextual ones; and 3) the Performance Hypothesis, which suggests that this increased engagement will lead to improved performance in spatial knowledge tasks, such as pointing accuracy. The aim of our study is to explore the role of these human agents and their congruency with context in spatial exploration and the development of spatial knowledge.
We examined the impact of human agents on spatial navigation and knowledge acquisition in a virtual city named Westbrook, consisting of 236 buildings. We identified 26 public buildings (e.g., shops, basketball court) and 26 residential buildings as task-relevant, marking them with street art. Additionally, there were 180 buildings without graffiti and four large buildings on the city’s outskirts, which could serve as global landmarks given their dimensions. We designed two categories of human agents: contextual agents, who performed context-relevant actions (e.g., holding a toolbox in front of a hardware store), and acontextual agents, who held a resting position without interacting with objects. In the first experiment, contextual agents were placed in public areas, displaying actions congruent with the buildings, while acontextual agents were positioned in front of residential buildings without interaction. In the second experiment, both agent types were split evenly across public and residential areas, disrupting the congruent pairs. Participants in both experiments completed five 30-min exploration sessions, totaling 150 min. Additionally, to provide a baseline for comparison of the exploration strategies, we used the control group from Schmidt et al. (2023) who explored the same VR city (Westbrook) with the same session lengths and numbers but no agents present. We investigated the exploration phase by analyzing participants’ navigational coverage of the city, their walking strategies, agent-induced bias in their exploration, and their visual behavior during exploration. Finally, we tested their spatial knowledge acquisition in a separate session using VR pointing tasks.
To establish comparability in spatial orientation abilities between the participants of the experiments (experiment 1, experiment 2, and control), participants completed the FRS (Fragebogen Räumlicher Strategien) questionnaire, and their scores were contrasted. There were no significant differences between the groups at baseline on any of the three subscales (global, survey, and cardinal),
During the VR city experiment, we tracked participants’ exploration, including walking behavior, navigational coverage, decision-point strategies, and visual behavior. This comprehensive analysis revealed their navigational strategies and engagement, highlighting the agents’ impact on their exploration.
We quantified participants’ walking behavior in the virtual city using a primal city graph (Neal, 2013) to analyze participants’ free exploration patterns. In this graph, decision points (i.e., intersections of walkable paths) were represented as nodes and paths connecting them as edges. Participants’ navigational coordinates (see Figure 1a) were assigned to the nearest graph element (see Figure 1b), defining their exploration as movements from one graph element to another. Out of 159 nodes, participants visited between 45 and 113 unique nodes during each 30-min session (M = 87.06, SD = 16.49). We calculated the coverage ratio by dividing the number of nodes visited at least once by the total number of nodes.
Figure 1. Free exploration of Westbrook (a) Presents a heatmap that visualizes the spatial navigation data of all participants across the exploration phase. It compiles movement across all sessions, with participant locations discretized to the nearest centimeter and averaged on a second-by-second basis after removing the initial 2 s of each recording. We fitted a heatmap grid approximating a 1-cm resolution and created a color scale ranging from 0 to 10 visits, highlighting areas of varied exploration intensity (b) Shows Westbrook from above, overlaid with a graph structure we used to formalize navigational data as decision units (c) Quantifies exploration through a map coverage ratio, calculated by dividing the number of unique nodes visited by the total nodes in the city (d) Compiles these ratios on a cumulative basis, reflecting the participants’ expanding discovery of the city over time.
To account for repeated measures, we implemented a linear mixed-effects model (LMM) that considered intrasubject variability and generated individual intercepts for each participant. This model predicted the individual session coverage ratio as a function of the session, the experimental group (control versus city with agents), and their interaction. Using the first session as a baseline, we observed significant cumulative increases in navigational coverage with each subsequent session. Starting from an individual coverage of roughly half the city (as shown by the mean of the blue curves in Figures 1c, d), the LMM analysis explained a substantial portion of the variance in navigational coverage, with marginal
The effect of the experiment group (agents versus control) on the coverage ratio was not statistically significant (
In order to estimate if the participants were differentially accumulating unique decision points in the city as the sessions progressed, we calculated a cumulative coverage ratio, accumulating the number of uniquely visited nodes as the sessions advanced. A LMM with participants as random effects was used to predict the cumulative coverage ratio as a function of session, experiment group, and their interaction (
To examine participants’ walking strategies, we analyzed whether they tended to choose paths they had previously visited more frequently (conservative) or if they favored less-traveled routes (explorative). We defined discrete navigational decisions as movements from one node to another and quantified them using a strategy matrix (see Figures 2a,b). A linear mixed-effects model was fitted to the data to examine differences in decision numbers based on session, strategy (conservative vs explorative), and experiment (control vs city with agents), with random intercepts for participants to account for the nested data structure. The model’s fixed effects (
Figure 2. Exploration strategy quantification (a) This panel illustrates the translation of continuous movement into quantifiable behavioral units. Movement coordinates, denoted by beige dots, are assigned to the closest node or edge centroid. Nodes, represented by blue circles, are positioned at decision points and are interconnected by black lines (edges). A conservative decision occurs when participants return to a frequently visited node, whereas an explorative decision is made when a less frequented node is chosen (b) This panel introduces a strategy matrix designed to analyze navigational choices. The matrix evaluates the path chosen by a participant (displayed on the y-axis) against all other possible paths at a decision point (x-axis). For instance, upon exiting node 157 (as seen on Panel A), the participant’s choice of node 156 (with two previous visits) is compared against nodes 155 and 158, which had 5 and 0 visits, respectively. The matrix is structured such that rows indicate visits to the selected node (in this case, 156) and columns to the unselected nodes (in this case, 155 and 158). Entries in the matrix at positions [2,0] and [2,5] increment by one, reflecting the selection of node 156 over alternatives. This matrix visually encodes decision-making patterns: counts above the diagonal suggest conservative decisions, and those below indicate exploratory actions (c) This panel synthesizes decision-making trends by displaying the average sum of decisions, categorized by strategy, by all participants across our two experiments and the control group in a single session.
In order to test our Visit Hypothesis, we evaluated the impact of agents on participants’ exploratory behavior; we analyzed decision points where participants could choose between a path with an agent and one without, assuming that agents might elicit visits. Data from these points were compared with a control group from Schmidt et al. (2023), who explored the same VR city (Westbrook) without agents (see Figure 3a). We used identical decision points in both scenarios. We applied a linear mixed-effects model to assess visit counts, accounting for session and experiment type (control vs city with agents) with random intercepts for participants (
Figure 3. Agent-induced bias in exploration patterns (a) The figure compares decision-making in virtual environments with and without agent presence. It contrasts our experimental data against a control from Schmidt et al. (2021). Panel A shows the average visit count to agent locations in our experiment (black) and the visits to those same points in the control (grey) (b) The probability of participants opting for paths where agents are located, classifying decisions as conservative when going towards the agent was the more familiar path, and exploratory when choosing the agent path was the less frequented route. We only show the first two sessions as the difference disappears afterward.
According to our Dwell Hypothesis, we expected participants to allocate more visual attention to contextually meaningful agents within the environment. To characterize what participants focused on in the city, we quantified their visual behavior by summing the cumulative time spent gazing at each object, termed dwell time. We hypothesized that participants would have higher dwell times for contextual agents and public buildings compared to acontextual agents and residential buildings, assuming contextual agents congruent with their surroundings would attract the most attention.
The data revealed distinct patterns in the attention participants allocated to different types of objects in the city. Notably, general residential houses across the city were observed for a shorter duration compared to our experimental buildings, with participants spending on average approximately 6.68 s (M = 6.68, SD = 6.69) gazing at these buildings. This is in contrast to the longer viewing times for our public task buildings (M = 11.82, SD = 8.48) and residential task buildings (M = 11.66, SD = 8.29), which attracted more sustained attention from participants. Furthermore, global landmarks within the city garnered significantly longer dwell times, with participants spending an average of 16.27 s (M = 16.27, SD = 10.69) focusing on these prominent features, nearly three times the average dwell time of general buildings. In terms of agents, contextual agents were observed for an average of 3.63 s (M = 3.63, SD = 3.74), while acontextual agents attracted slightly less attention, with an average dwell time of 2.66 s (M = 2.66, SD = 2.78). These findings indicate that our experimental manipulations effectively captured participants’ gaze in the expected order for both buildings and agents.
We further analyzed how each experimental factor influenced visual attention. On average, participants spent more time looking at contextual agents (M = 3.39, SD = 3.49) compared to acontextual agents (M = 2.73, SD = 2.91, see Figure 4a). Contextual agents also seemed to distract participants from focusing on the area behind them, as buildings with contextual agents had lower dwell times (M = 12.42, SD = 8.13, see Figure 4e) compared to those with acontextual agents (M = 13.28, SD = 9.27) see Figure 4b. These results underscore the adversarial relationship between agents and building attention, where increased focus on contextual agents corresponded with decreased attention to the buildings behind them.
Figure 4. Dwell times across sessions: This figure presents the dwell time, defined as the cumulative sum of fixations on specific objects during the exploration phase, measured in seconds. The bar graph shows the interplay of two factors, building and agent category (a) Illustrates the average dwell times for four distinct building and agent type combinations. Each bar represents a unique combination: public buildings with contextual agents, public buildings with acontextual agents, residential buildings with contextual agents, and residential buildings with acontextual agents. The height of each bar indicates the average dwell time, reflecting the relative visual attention each combination received. To the right, we examine one factor at a time for dwell time on the agent on the top (b–d), and on buildings on the bottom (e–g).
Regarding building type, dwell time on the agent was not significantly affected by the surrounding area, as agents in public areas (M = 3.07, SD = 3.07) and residential areas (M = 3.03, SD = 3.37, see Figure 4c) received similar attention. However, participants spent more time gazing at residential buildings (M = 13.39, SD = 9.01) compared to public buildings (M = 12.32, SD = 8.42, see Figure 4f). These results imply that incongruent agents divert focus from surroundings, as indicated by the anti-correlation in dwell times between agents and buildings.
Examining congruency (see Figure 4d), participants looked at incongruent agents (M = 3.23, SD = 3.48, see Figure 4d) for longer periods compared to congruent agents (M = 2.60, SD = 2.44). This pattern was opposite for building gazing time, with participants spending more time looking at buildings and surroundings when the agent matched the context in which it was placed (M = 12.92, SD = 7.65) compared to when the agent did not match the surroundings (M = 12.83, SD = 9.15, see Figure 4g). These results imply that when faced with incongruent agents, participants redirect their focus away from the surroundings, as evidenced by the anti-correlation in dwell times between agents and buildings in the congruency factor.
To account for the high inter-individual variability and the nested structure of the data, we employed a linear mixed-effects model to predict dwell time on agents, with subjects as a random effect. The fixed effects included the context (residential vs public), agent type level (acontextual vs contextual), the congruency of the agent with their surroundings (not congruent vs congruent), and the interaction between agent type and context. The results (
We fitted an analogous linear mixed-effects model for the dwell time on buildings (
We assessed participants’ spatial knowledge acquisition using pointing tasks. In the Pointing to Buildings task, participants were given screenshots of target buildings, while in the Pointing to Agents task, they received screenshots of agents against a grey background. Participants were asked to point toward the target. Accuracy was measured by calculating the angular difference between their pointing direction and the direction to the center of the target building or agent. This angular error served as the performance indicator, with a perfect score yielding zero degrees of error and higher values indicating greater inaccuracy.
According to our Performance Hypothesis, participants would have more accurate pointing for public buildings, especially when targets had active contextually congruent agent-building pairs. We applied a linear mixed-effects model to predict pointing errors, incorporating fixed and random effects. The random effects accounted for the test location of the pointing task (28 distinct locations with repeated measures). The fixed effects included the type of building, type of agent, congruency pairs, and the interaction between agent and building (
After the linear mixed-effects analysis, we examined the estimated marginal means (EMM) to clarify how different factors influenced pointing accuracy. Public buildings (
Figure 5. Analysis of pointing accuracy across experimental conditions. This figure illustrates the absolute pointing angular error, a measure of spatial recall accuracy whose lower values indicate better performance, with data points representing the mean error rates and error bars indicating standard errors (a) pointing to buildings: This plot delineates participants’ pointing accuracy to buildings paired with contextual and acontextual agents (b) Pointing to Agents: Here, participants’ accuracy in pointing to agents is compared, with a focus on the agent’s activity level and the type of building (c) Aggregated Task Performance: This plot displays overall performance across both building and agent pointing tasks.
According to our Performance Hypothesis, participants would demonstrate lower pointing errors for the locations of contextual agents, particularly those positioned in front of public buildings. To test this hypothesis, we employed a linear mixed-effects model similar to the one used for analyzing pointing-to-building performance. The model included crossed random effects for subjects and the starting locations of the pointing tasks, covering 28 distinct locations. The analysis revealed (
Consistent with the Performance Hypothesis, participants exhibited lower pointing errors for contextual agents (
We expected participants to be less precise when pointing to agent stimuli compared to building stimuli. To investigate this, we first assessed whether participants exhibited significantly lower precision when pointing to agents. This was achieved by fitting a two-crossed random effects model (i.e., ID and pointing location) and predicting pointing error with the type of stimuli (agent vs building) as the sole predictor. The analysis revealed (
To assess whether the time spent looking at both agents and buildings would significantly predict participants’ performance, we incorporated the dwell time in seconds each participant spent gazing at the agents and buildings as fixed effects in the pointing-to-building and pointing-to-agent tasks (see Figure 6). For the pointing-to-building task, the results showed (
Figure 6. Accuracy prediction models: The estimates for the five effects and one interaction are displayed using one standard error as error bars. The linear mixed models were computed employing a structure of two crossed-random effects, which accounted for the pointing place (i.e., the 28 pointing locations) and the incorporation of repeated measures. The red line denotes no difference amongst the levels of the factor. Instances marked by three asterisks indicate significance levels falling below 0.001, while two asterisks indicate significance below 0.01. The upper panel represents the same results as the first two lines of the lower panel but zoomed in.
Table 1. Bootstrap Confidence Intervals for Model Estimates. The table presents the 95% confidence intervals obtained from bootstrap resampling (1,000 iterations).
Comparing the results from the two tasks, it becomes evident that there is an inverse relationship between the ability to locate agents and buildings, as both compete for attention. Contextual agents improved the recall of their locations, yet the presence of public buildings appeared to detract from the ability to remember the agent. Therefore, while agents may serve as useful proxies for recalling target locations, their own positions are not necessarily better remembered in relation to their surroundings.
This study explored the impact of human agents on spatial navigation and knowledge acquisition within a controlled virtual reality city environment. Our findings suggest that while the presence of agents influenced spatial exploration, this effect was relatively minor, as the overall exploration strategy remained largely unchanged. Nonetheless, agents increased the likelihood of participants revisiting the buildings where they were located upon first exploration of the environment. In these spaces, participants paid more visual attention to agents that did not match their surroundings, indicating a context-dependent perception of these agents. During exploration, agents and buildings competed for attention, shaping how spatial information was processed. When testing spatial knowledge, pointing accuracy for buildings benefited from both the buildings and the agents, suggesting a synergistic effect where agents enhanced the salience of building locations. However, this benefit did not extend to pointing accuracy for agents, where accuracy did not improve when the agent was located at a more salient building. Additionally, contextual incongruence played a crucial role in enhancing pointing accuracy, highlighting non-linear effects that further underscore the complexity of how the presence of others can shape salience. Overall, it can be concluded that the presence of agents and their integration into the environment significantly shape how spatial information is remembered and, to a lesser extent, how the space is explored.
A key limitation of this study is that the agents, including those classified as “contextual,” remained static, which does not reflect the dynamic interactions typical of real-world scenarios. Additionally, the fixed positions of the agents throughout the study may have obscured more subtle changes in participant behavior that could occur with moving or varying agent locations. This lack of movement may limit the generalizability of our findings to real-life situations where the presence and behavior of others are more variable. However, we maintained this static feature to ensure consistency between the two agent categories, allowing us to investigate how even minimal actions, such as holding an object, can influence participant behavior and performance. Through this design, we identified a minimal level of experimental manipulation that exerts a differential impact by human agents without introducing the inherent eye movement biases that a moving group might create.
It is necessary to note that people’s interactions with agents can differ based on the agent’s group size, and our study focused solely on single agents. We excluded groups because previous research shows that individuals are more inclined to approach single agents (Bonsch et al., 2018), and crowded spaces may deter pedestrians, causing navigation avoidance (Dickinson et al., 2019; Li et al., 2019). Our experiment, with 56 agents per square kilometer, likely stayed below the discomfort threshold. Therefore, our findings suggest that lower agent densities can be utilized to study navigation behaviors without inducing avoidance, providing insights into optimal crowd levels for effective spatial navigation.
The virtual environment, though extensive, cannot fully replicate the complexity and nuances of real-world navigation and interactions, particularly concerning general movement flow. In real-world environments, a multitude of factors contribute to how individuals navigate and perceive spaces, including moving objects, varying light conditions, and the presence of other moving entities. While controlled and consistent, the static nature of our virtual environment lacks these dynamic elements that typically influence human behavior. This limitation means that our study did not capture certain interactive and responsive behaviors, which are naturally triggered by a dynamic environment. However, we deliberately opted against including dynamic environmental elements for two primary reasons: first, to optimize the sample rate, as additional movement could burden the system and reduce the reliability of data capture; second, to avoid introducing confounding variables that might draw visual attention and skew our eye-tracking analysis.
Lastly, it is worth considering whether the presence of agents in our study, particularly in incongruent conditions, influenced participants’ sense of being within the virtual environment and their perceived reality of the environment, as described by (Slater, 2009). Variations in agent-context alignment may have unintentionally modulated these aspects of presence. Since this was not the focus of our study, we did not assess these potential effects; however, future research should explore whether such factors influence spatial learning.
To understand participants’ broader navigational behavior, we analyzed their spatial coverage and decision-making strategies, contrasting participants who explored the city with (our experiments) and without agents [control group from (Schmidt et al., 2023)]. We developed a behaviorally-based method to quantify the exploration trade-off through a primal graph that captures decision-level spatial navigation dynamics in the virtual city environment. The results demonstrated that as participants became more familiar with the environment, they progressively explored more of the city with each session. Notably, participants showed consistent patterns in both the total area covered and the balance between exploratory and conservative behavior, regardless of whether agents were present. Our findings align with previous research on exploration-exploitation dynamics. For instance, Choi et al. (2012) observed that participants switched from long, unpredictable search movements (Levy flights) when uncertain to shorter, deliberate paths (Brownian walks) as they gained confidence. Similarly (Dickinson et al., 2019; Li et al., 2019), demonstrated that various optimal foraging strategies, from ballistic to Brownian motion, emerge based on the experience with the environment. In concordance with these free exploration studies, our study found that participants’ strategies evolved in response to their growing familiarity with the VR city, balancing exploration and exploitation with agents not interfering in this process.
By analyzing participants’ navigational decisions, specifically their tendencies between revisiting known locations and exploring new paths, we identified an enhanced likelihood of revisiting behavior unique to the first session, where participants were significantly more likely to move in the direction of agents. These results provide partial support for the Visit Hypothesis, indicating that while agents initially biased participants’ revisitation behavior, their influence diminished over time. Specifically, in the first session, participants were more likely to move in the direction of agents, suggesting that agents can momentarily shape navigation choices. However, this effect did not persist across sessions, as broader spatial exploration strategies remained stable regardless of agent presence. This highlights that agents primarily exert a localized influence on movement patterns rather than systematically altering long-term exploration behavior. Globally, participants maintained a consistent exploration rate, characterized by a mix of exploratory and conservative decisions, across all sessions. This consistency reinforces the idea that agents exert a localized impact on navigation, affecting behavior at specific decision points without altering the overall approach to exploring the environment.
When participants explored areas with agents, they preferred visually engaging with contextual agents over acontextual ones despite all agents being static. This aligns with theories indicating that stimuli with higher informational density demand more cognitive processing, making them more salient (Henderson 2007; Summerfield and Egner 2009; Wolfe 2020). Such stimuli are perceived as more salient particularly when they contain elements relevant to the task at hand or the environment’s narrative (Harel et al., 2014; Miller et al., 2014). Previous studies have found that, whether consciously or not, people map their surroundings in terms of future action planning (Bach et al., 2014; Bonner and Epstein, 2017; Ohm et al., 2014). This tendency extends to situations involving other people, where the presence of a person in a position to interact with objects can lead observers to adopt that person’s spatial perspective (Tversky and Hard, 2009). Thus, one could argue that contextual agents holding objects and displaying varied body positions garner longer dwell times because they hint at navigational affordances; that is, they prime the participants for possible actionable routes. Consequently, contextual agents would have higher informational value for participants navigating a city since they hint at contextual elements that could be acted upon.
Furthermore, our analysis revealed that the longest gaze times were directed toward contextual agents that mismatched their surroundings. This finding can be understood through the framework of spatial schema, which are structured bodies of prior knowledge used to navigate and interpret environments (Farzanfar et al., 2023). When these agents clashed with their background, it likely elicited an expectancy violation (Burgoon, 2015), prompting participants to engage in a longer visual search to reconcile this disparity. Interestingly, this heightened attention to incongruent agents in residential settings could also reflect an innate tendency to monitor unexpected behaviors in familiar environments, similar to how individuals remain vigilant in their own neighborhoods. Together, these results support the notion that agents are perceived contextually, and participants showed signs of trying to integrate them into the space they inhabit. These findings align with the Dwell Hypothesis, confirming that participants allocated more visual attention to elements with higher contextual relevance.
Regarding building gazing, our participants spent more time inspecting residential buildings than public buildings. This finding contradicts previous research, which generally suggests that people tend to spend longer looking at public buildings like restaurants, stores, and landmarks due to their visual complexity and social relevance (Rounds et al., 2020). Eye-tracking research has consistently found that functional and visually salient landmarks, such as public buildings, are more likely to be used for navigation and remembered longer Farran et al., 2016; Walter et al., 2022. However, our participants’ preference for residential buildings suggests a potential contextual influence. In our study, all task-relevant buildings had graffiti, which may have caused the extended gaze time. Additionally, all task-relevant residential buildings had an agent in front of them, which could have garnered more viewing time. Finally, it might be the case that since the residential buildings were more similar to each other, participants required more time to tell them apart. While one gaze at a doughnut shop can already provide a clear memory cue, a gaze at a standard residential house might need more time to gather a cue that would help differentiate it from neighboring buildings.
Our study provides significant insights into the role of human agents in spatial knowledge acquisition, particularly regarding pointing accuracy. The consistent finding across both pointing-to-agent and pointing-to-building tasks is that contextual agents significantly enhance performance. This aligns with previous research indicating that social targets and interactive cues improve navigational accuracy and spatial encoding. For instance, Kuehn et al. (2018) demonstrated that participants exhibited reduced positional errors when navigating with a person as a target, suggesting that social targets facilitate spatial encoding by enhancing the processing of both body-based and environment-based cues. Similarly, Gunalp et al. (2019) found that including an avatar in spatial perspective-taking tasks improved performance compared to abstract directional cues, underscoring the importance of social and interactive aspects in aiding mental simulation processes required for spatial tasks. More specifically, the pronounced effect of contextual agents on spatial knowledge acquisition may be attributed not only to increased visual engagement but also to the cognitive implications of perceived action. Previous studies have shown that even the suggestion of action in still images can enhance spatial processing (Tversky and Hard, 2009). In our study, when those actions were incongruent with the surroundings, performance was further enhanced, likely because reconciling the mismatch strengthened the memory cues for the location. This suggests that by implying potential actions, contextual agents encourage participants to process spatial information from multiple perspectives, thereby enhancing their overall spatial understanding and memory. These findings provide strong support for the Performance Hypothesis, demonstrating that agents embedded in a meaningful narrative context enhance spatial recall and improve accuracy in pointing tasks.
A notable finding from our study was the overall higher precision in pointing to buildings compared to agents. This indicates that while human agents can enhance spatial recall, participants generally exhibited greater accuracy when recalling traditionally static buildings. This could be attributed to buildings’ more stable and distinctive features, which provide consistent spatial cues. In contrast, agents, being potential sources of movement, may introduce variability in spatial memory. The differential impact of agents on pointing accuracy to buildings and agents highlights the importance of contextual relevance in spatial knowledge acquisition. Contextual agents, particularly those performing contextually incongruent actions, appear to be strong spatial anchors for their surroundings but are not remembered as landmarks. This aligns with the idea that while faces naturally attract attention (Gert et al., 2020), their mobility reduces their usefulness as fixed spatial references. Instead, their presence may shape how participants engage with and encode the surrounding environment, particularly static landmarks.
These findings have significant implications for the design of spatial navigation systems. Incorporating human contextually relevant cues such as contextual agents can enhance spatial recall and bias navigation efficiency. This aligns with the broader literature on wayfinding, which emphasizes the importance of functional landmarks and visual salience (Franke and Schweikart, 2017; Ohm et al., 2014). By leveraging contextual agents and ensuring their actions are contextually relevant, navigation systems can provide more effective spatial cues, aiding users in more accurately recalling and navigating environments. Additionally, intentionally contrasting agents with the environment could improve the memory of a location as it might contradict expectations. In summary, our study underscores the critical role of human agents in shaping spatial knowledge acquisition. Contextual agents enhance pointing accuracy and spatial recall, mainly when they are incongruent. These findings contribute to a deeper understanding of how human elements within an environment can be effective navigational aids, providing valuable insights for developing more intuitive and effective spatial navigation systems.
This study explored the impact of human agents on spatial navigation and knowledge acquisition within a virtual city. Our findings show evidence that human agents, particularly those portraying actions, locally influence navigational behavior and enhance spatial recall. Contextual agents drew more visual attention and served as effective spatial cues, improving participants’ ability to recall specific locations. Interestingly, agents that were incongruent with their surroundings further enhanced spatial memory, suggesting that expectancy violations prompt spatial knowledge acquisition. These results underscore the importance of incorporating human elements into virtual environments to better understand their role in spatial cognition. The insights gained from this study have potential applications in designing more intuitive spatial navigation systems and enhancing training programs for environments where human interaction is a critical component.
We recruited 70 participants, distributing them equally across two experiments (35 per experiment). Participants were required to have normal or corrected-to-normal vision and to attend five 30-min sessions, plus one final 60-min test session in a VR environment. Sessions were scheduled at intervals ranging from 4 hours to 3 days. However, attrition occurred due to sickness or missed appointments, resulting in 10 participants being unable to complete the required schedule and 15 participants being excluded due to technical failures, like interruption of files. Additionally, three participants withdrew due to motion sickness. The final sample included 21 participants (12 females,
The virtual environment, known as Westbrook see Figure 7b, was developed using Unity LTS 2019.4.27f1, as described by Schmidt et al. (2023). The virtual city covers an area of approximately 1 km2 and is inspired by the layout of the Swiss city Baulmes. It features 236 buildings, including 26 public buildings (i.e., shops, basketball courts), 26 residential buildings marked with graffiti, and 180 regular buildings devoid of graffiti. Additionally, four large buildings are strategically placed at the city’s periphery. The buildings with graffiti were designated as task buildings in both our experiment and the experiment by Schmidt et al. (2023) and are distributed roughly equally throughout the city. Mesh colliders were applied to all objects to facilitate the tracking of participants’ visual behavior through 3D eye vector projection onto the environment. In Unity, a collider is an invisible component that defines the physical boundaries of an object, allowing the physics engine to detect interactions with it. One can imagine it as an invisible skin covering the surface of objects, enabling precise collision detection without affecting their visual representation. This approach ensured that gaze data could be accurately mapped onto the complex surfaces of the virtual world, allowing for precise analysis of visual attention.
Figure 7. Overview of the virtual reality environment (a) Experimental setup, highlighting the use of a swivel chair for lateral navigation and a joystick on the VIVE controller for forward movement (b) First-person immersive view available to participants (c) Aerial view of the virtual city.
Navigation within the city is confined to visible paths and streets defined by a custom navigation mesh, with restricted access to areas blocked by fences or other physical barriers. Conventional orientation cues such as street names, house numbers, and solar positioning were deliberately excluded to enhance the navigational challenge. Participants controlled their translational movement up to a maximum speed of 5 m/h using a joystick, while rotational movements were facilitated by physical rotation on a swivel chair.
The inclusion of 56 human agents from the Adobe Mixamo collection (Mixamo, 2008) was designed to explore the impact of human elements on spatial learning. Agents were divided into two groups: 28 (14 males and 14 females) in the contextual agent group and an equal number in the acontextual agent group. Contextual agents were equipped with items and body postures that underscored the significance of specific buildings—such as a sandwich at a sandwich shop or a toolbox at a tool store (see Figure 8a). Conversely, acontextual agents, matched in skin tone, hair color, and gender with the contextual group, were depicted in a relaxed standing pose see Figure 8b), enhancing environmental realism without engaging in explicit, building-specific activities. All agents were static and non-responsive to the participants.
In the first experiment, contextual agents were designed to be positioned in public buildings to reflect the thematic context of their surroundings. Specifically, contextual agents were positioned in front of the 26 street art-marked public buildings and two larger buildings. For instance, a construction worker wielding a shovel was situated at a construction site among 26 public buildings, along with two thematic global landmarks (i.e., a church-goer with a bible at a church and another construction worker at a castle under construction). Conversely, acontextual agents were stationed in neutral stances within the 26 residential areas and at two additional larger buildings (i.e., a silo and a windmill).
In the second experiment, we changed the setup by redistributing contextual and acontextual agents across public and residential areas, intentionally breaking the congruency established in the first experiment. This shuffle placed half of the contextual agents in non-matching public buildings and the other half in residential settings. Similarly, acontextual agents were also re positioned, with half now found in public spaces and the remaining half in residential areas. This reconfiguration aimed to probe the effects of agent-building incongruency on spatial learning, challenging the direct association between agents and specific buildings.
The experiments were divided into two main phases: the exploration and task assessment phases. The exploration phase consisted of five sessions, each lasting 30 min, resulting in 150 min of free exploration (see Figure 9). The task assessment phase was the sixth session, during which participants performed a pointing task. Sessions were spaced with intervals ranging from a minimum of 4 hours to a maximum of 3 days.
Figure 9. Experimental design and procedure: Panel A illustrates our 2 × 2 experimental design, featuring acontextual/contextual agent types and public/residential buildings, which were assigned differently in the two experiments. Panel B outlines the exploration phase procedure, where participants first filled out the FRS questionnaire and then completed five 30-min sessions of unguided exploration within the virtual city of Westbrook. Panel C describes the assessment phase during a sixth session, where participants completed pointing tasks within the VR city. (A) Experimental Design. (B) Exploration phase. (C) Assesment phase.
In the initial session, participants received a comprehensive overview of the experiment and provided written informed consent. They then completed the “Fragebogen Räumlicher Strategien” (FRS) questionnaire to assess their spatial orientation abilities (Münzer and Hölscher, 2011). Subsequently, participants were familiarized with the VR environment in a neutral VR room. In this room, they practiced controlling their lateral movements by rotating in a swivel chair and regulating their forward movement using the joystick on their VIVE controller. The exploration phase within the actual city began once participants confirmed their understanding of the movement mechanics.
During the exploration phase, participants were instructed to freely explore the virtual city for 30 min, imagining they were getting acquainted with a real city they would be tested on by the end of the experiment. In contrast with Schmidt et al. (2023), we did not instruct them to look for street art specifically marked houses, but rather to just try to pay attention to the city’s layout. The sessions were divided into three 10-min segments, and a 9-point eye-tracking calibration and validation were performed at the start of each segment to ensure measurement accuracy, maintaining a visual tracking error of less than one degree.
Participants performed a pointing task within the VR environment in the assessment session from a first-person perspective. Within the task, participants were teleported to 28 predetermined locations inside Westbrook. At each location, they were presented with a target at the center of their visual field and instructed to point directly at the center of the target. In experiment 1, the targets were solely buildings (i.e., pointing-to-building). In contrast, in experiment 2, we included a test that displayed only the agent against a gray background (i.e., pointing-to-agent). To account for the sequence effect, the task order was strategically randomized, with one-half of the participants starting with the pointing-to-agent task followed by the pointing-to-building task and the other completing the tasks in reverse order. Finally, all participants completed three self-assessment questionnaires that inquired about their perception of each agent, their social tendencies in real life, and the realism level of the VR. The questionnaire data has not been analyzed in this work.
The exploration and task assessment sessions were conducted with a desktop computer with an Intel (R) Xeon (R) W-2133 CPU, 16 GB RAM, and a Nvidia RTX 2080 Ti graphics card. The VR environment was rendered with a HTC Vive Pro Eye head-mounted display (HMD) setup with a refresh rate of 90 Hz and horizontal and vertical field of view of 106° and 110°, respectively. We used four SteamVR Base Stations 2.0, an HTC VIVE body tracker 2.0, and Valve Index controllers to monitor participants’ positions within the environment. This combined setup achieved sub-millimeter precision in capturing the head, body, and eye positions, as well as rotation and orientation.
Participants performed pointing tasks in VR from a first-person perspective. In the pointing-to-building task, they were teleported to 28 unique locations within the city, each serving as a distinct reference point. The sequence of these locations was randomized for each participant to prevent order effects. To further minimize systematic biases, participants’ orientations after teleportation were also randomized, ensuring that they did not always begin facing the same direction. At each location, participants pointed repeatedly toward one of 56 potential targets, represented by static images of buildings, with agents positioned in front of them. These images were captured perpendicularly at a height of 1.80 m to ensure consistency in visual presentation.
The trials began with a visual and auditory cue: a 25 ms green circular loading bar at the screen’s center accompanied by a beep. The target image appeared at the upper center of the screen, with a green dashed laser beam providing a visual guide for pointing. Participants indicated their direction by pressing a trigger button on their controllers. Each trial was timed for 30 s, automatically concluding if a direction was not indicated within this period. Performance was assessed by measuring the angular difference between the participant’s pointing direction and the precise center vector of the target location.
In the initial experiment, participants completed 336 trials, pointing at 12 unique targets from each of the 28 reference locations. The reduction in the number of trials in the second experiment, where participants completed only 224 trials, pointing at eight distinct targets from each location, was necessitated by the additional pointing-to-agents task. In both experiments, the trials were balanced to ensure an even distribution of target types: 50% directed participants to public locations and 50% to residential areas.
This task was fundamentally equivalent to the pointing-to-building but specifically focused on agents as the target. The targets featured centered screenshots of individual agents set against a grey backdrop, captured perpendicularly at a height of 1.80 m. Participants undertook 224 trials and were instructed to point at eight unique agent targets from each of the 28 reference locations. The task mirrored the structure of the building task in terms of target placement, visual and auditory cues, and time constraints to maintain consistency in the testing conditions. Additionally, the sequence of these pointing locations was randomized for each participant, with the goal of avoiding order effects and preserving the integrity of the experimental data.
The “Fragebogen Räumlicher Strategien” [FRS; Münzer and Hölscher (2011)] questionnaire is a 7-point Likert scale that asks the participants to estimate their spatial orientation abilities in three areas of spatial knowledge in real-world scenarios. First, the global sub-scale consisted of 10 items
To analyze participant trajectories and their exploration decisions, we created a data-driven graph based on their actual trajectories while exploring the virtual city. Thus, this graph reflects only the paths and areas that participants walked through, constituted by the streets (edges) and crossings or decision points (nodes) that participants walked through. We first generated a heatmap of participants’ movement to transition from raw sequential coordinates to spatial data. This heatmap accounted for the number of times a participant stood at a specific cell within a defined 4 m × 4 m grid on top of the city map. This heatmap was then turned into a binary image, where cells visited at least once were assigned a value of one and cells without visits a value of zero. The resulting image clearly outlined the walkable paths and connections within the city. We filled isolated holes within the streets to ensure the algorithm generating the graph did not create extraneous nodes in these areas. We then generated a skeleton from the binary image, reducing the city’s representation to a one-pixel width while preserving its topography and connectivity. This skeleton was the foundation for identifying the graph’s nodes and edges. Using the external Python library “sknw,” we generated a NetworkX graph from the city skeleton image. Nodes were numbered sequentially, and edges were named based on the nodes they connected (see Figure 2a). For example, edge [76,60] connected nodes 76 and 60. Some manual adjustments, such as adding an edge, were necessary to perfect the graph. Each pixel in the skeleton was recognized as belonging to a node or an edge. This information was later used to plot the graph and calculate distances to identify the graph elements visited by participants during their sessions.
To analyze exploration strategies, we developed an algorithm that converts participants’ coordinates data into a sequence of visited nodes and edges. This process involved mapping the coordinates to the skeleton heatmap’s 4 m × 4 m cells and determining the closest graph element using the Euclidean distance formula. The algorithm tracked transitions between edges and nodes, recording each visited element. This record included the time of entry, graph element type (node/edge), and number of visits to the graph with a, well as the available paths from the node of origin and their respective number of previous visits to the possible elements available from that position. We adjusted the nodes’ radii to match the width of the corresponding streets. This adjustment ensured accurate detection of participant presence at nodes, preventing unnoticed transitions between edges. Overlapping node radii in areas with several short streets were resolved by assigning participant positions to the nearest node centroid.
We condensed participants’ decisions at nodes into a strategy matrix to analyze their exploration patterns. The strategy matrix was organized with the number of visits to the chosen node on the rows and the number of visits to the not-chosen nodes on the columns. Each decision was recorded in the row corresponding to the number of previous visits to the selected path. We added one to each column corresponding to the number of prior visits on the other available paths from that decision point that were not selected. For instance, if a participant was at a node that had three direct neighbors, visited zero, five, and two times, and chose to move in the direction of the node visited two times, we would add a count of one to the positions [0,2] and [5,2]. This represents that the participant moved to a node with two visits over the options that had been visited zero and five times (see Figure 2b). Decisions above the diagonal line in the strategy matrix were considered conservative, indicating that participants preferred nodes they had visited more frequently in the previous example, the one added at [0,2]. In contrast, decisions below the diagonal line were considered exploratory, as participants chose nodes with fewer visits compared to their neighboring nodes, in the example above the one added at [5,2]. Decisions exactly on the diagonal were neutral, as both the chosen and the adjacent nodes had the same number of previous visits. This method allowed us to quantify and compare participants’ exploratory and conservative tendencies as they navigated the virtual city, providing insight into their spatial decision-making processes as they gained experience inside Westbrook.
We applied a velocity-based algorithm that classified continuous eye movements into gazes and saccades and corrected the resulting gazes for the participants’ movement in the 3D environment, as developed by (Nolte et al., 2024). In preparation for this algorithm, we preprocessed the data by excluding portions detected as invalid (e.g., blinks). In cases where more than one collider hit was detected within the same sample, we retained the closest hit to the participant, except for background colliders, such as leaves or fences, in which case we kept the second closest hit. As a last step, we dropped duplicated samples and applied a 5-point median filter to the gaze coordinates. The algorithm calculates the median velocity of eye movements within a time window (in our case, a 10-s window). Samples exceeding this velocity threshold are identified as saccades, while those falling below the threshold are classified as gazes. To ensure accuracy, we applied outlier detection based on median absolute deviation to correct for gaze events with anomalous durations (i.e., exceeding three median absolute deviations). Dwell time was defined as the cumulative time a subject spent gazing at a specific object within the city across all sessions. We computed the dwell time for each subject-object pair during their entire exploration period within the Westbrook environment.
Given the hierarchical structure of our data, we employed Linear Mixed-Effects Models for our analysis. The modeling was done using R 4.3.2 with the lmer() function from the lme4 package. We used Restricted Maximum Likelihood for estimation and the nloptwrap optimizer (Bates et al., 2015). This approach allows us to handle the nested structure of our data, with random effects to account for within-subject variability. Fixed effects with two levels were effect-coded to ensure the betas reflect the differences between these levels, using the first level of each pair as the base for comparison.
To analyze participants’ free exploration patterns, we quantified their walking behavior through the virtual city using a primal city graph Neal (2013). The coverage ratio, defined as the proportion of unique nodes visited during each session, was modeled using a linear mixed-effects approach. The model included fixed effects for the session, experiment, and interactions, with planned contrast testing for each session against the first. Random intercepts for participants were included to account for repeated measures within subjects. The model formula for the individual session coverage ratio was (see Equation 1):
The same structure was used to test for the cumulative ratio of visited nodes, in which we kept track of how many unique decision points each participant had visited, accumulating them between sessions. The model formula for the cumulative coverage ratio was (see Equation 2):
To investigate the extent to which participants used conservative or exploratory walking strategies, we modeled the number of decisions at each node. A linear mixed-effects model (see Equation 3) was used with the session, strategy (conservative vs exploratory), and experiment as fixed effects and random intercepts for participants.
We quantified participants’ visual behavior by summing the cumulative time spent gazing at objects (dwell time) during the entire exploration phase. Separate models predicted dwell time on agents (see Equation 4) and buildings (see Equation 5), with fixed effects for agent type (acontextual vs contextual), context effect (residential vs public), the congruency of the agent with their surroundings (not congruent vs congruent), and their interactions, including random effects for participants with the following formulas:
We assessed spatial knowledge using pointing tasks, calculating the angular error as the performance indicator. Two separate linear mixed-effects models (see Equations 6, 7) predicted pointing error based on building type (residential vs public), agent type (acontextual vs contextual), the congruency of the agents with their surroundings, dwell time on agents, dwell time on buildings, and the interaction of agent and building type. Random effects for participants and pointing locations were included in each model:
To compare accuracy between pointing to buildings and pointing to agents, we used a model with the type of stimuli (agent vs building) as the fixed effect and participants and pointing locations as random effects (see Equation 8):
Following model fitting, we performed likelihood ratio tests to compare each model against a null model containing only the intercept, evaluating the added predictive power of our factors.
Figures, tables, and images will be published under a Creative Commons CC-BY licence and permission must be obtained for use of copyrighted material from other sources (including re-published/adapted/modified/partial figures and images from the internet). It is the responsibility of the authors to acquire the licenses, to follow any citation instructions requested by third-party rights holders, and cover any supplementary charges.
The raw data supporting the conclusions of this article will be made available by the authors, without undue reservation.
The studies involving humans were approved by University of Osnabrück Ethics Committee. The studies were conducted in accordance with the local legislation and institutional requirements. The participants provided their written informed consent to participate in this study.
TS: Conceptualization, Formal Analysis, Investigation, Writing – original draft, Writing – review and editing. MS: Formal Analysis, Investigation, Resources, Writing – review and editing. KG: Formal Analysis, Investigation, Resources, Writing – review and editing. VS: Conceptualization, Resources, Writing – review and editing. DN: Formal Analysis, Investigation, Resources, Writing – review and editing. SK: Conceptualization, Supervision, Writing – original draft, Writing – review and editing. GP: Conceptualization, Funding acquisition, Supervision, Writing – review and editing. PK: Conceptualization Funding acquisition, Supervision, Writing – original draft, Writing – review and editing.
The author(s) declare that financial support was received for the research and/or publication of this article. The University of Osnabrück supported this work in cooperation with the Deutscher Akademischer Austauschdienst (DAAD), Grant No. 57440921 and the Deutsche Forschungsgemeinschaft (DFG, German Research Foundation)—GRK 2340.
The authors express their gratitude to everyone who contributed to this project. They would like to thank Nora Maleki and Linus Tiemann for their help in developing the VR city and the Pointing Tasks required for these experiments, and Philipp Spaniol for his 3-dimensional art and implementation of differential loading of levels of details with the agents on this scene.
The authors declare that the research was conducted in the absence of any commercial or financial relationships that could be construed as a potential conflict of interest.
The author(s) declared that they were an editorial board member of Frontiers, at the time of submission. This had no impact on the peer review process and the final decision.
All claims expressed in this article are solely those of the authors and do not necessarily represent those of their affiliated organizations, or those of the publisher, the editors and the reviewers. Any product that may be evaluated in this article, or claim that may be made by its manufacturer, is not guaranteed or endorsed by the publisher.
Bach, P., Nicholson, T., and Hudson, M. (2014). The affordance-matching hypothesis: how objects guide action understanding and prediction. Front. Hum. Neurosci. 8, 254. doi:10.3389/fnhum.2014.00254
Bajorunaite, L., Brewster, S., and R. Williamson, J. (2022). ““Reality anchors”: bringing cues from reality into VR on public transport to alleviate safety and comfort concerns,” in CHI conference on human factors in computing systems extended abstracts (New Orleans LA USA: ACM), 1–6. doi:10.1145/3491101.3519696
Bates, D., Mächler, M., Bolker, B., and Walker, S. (2015). Fitting linear mixed-effects models using lme4. J. Stat. Softw. 67. doi:10.18637/jss.v067.i01
Bicanski, A., and Burgess, N. (2020). Neuronal vector coding in spatial cognition. Nat. Rev. Neurosci. 21, 453–470. doi:10.1038/s41583-020-0336-9
Bonner, M. F., and Epstein, R. A. (2017). Coding of navigational affordances in the human visual system. Proc. Natl. Acad. Sci. 114, 4793–4798. doi:10.1073/pnas.1618228114
Bonsch, A., Radke, S., Overath, H., Asche, L. M., Wendt, J., Vierjahn, T., et al. (2018). “Social VR: how personal space is affected by virtual agents’ emotions,” in 2018 IEEE conference on virtual reality and 3D user interfaces (VR) (Reutlingen: IEEE), 199–206. doi:10.1109/VR.2018.8446480
Burgoon, J. K. (2015). “Expectancy violations theory,” in The international encyclopedia of interpersonal communication. Editors C. R. Berger, M. E. Roloff, S. R. Wilson, J. P. Dillard, J. Caughlin, and D. Solomon 1 edn. (Wiley), 1–9. doi:10.1002/9781118540190.wbeic102
Choi, S. H., Jung, T. M., Lee, J. E., Lee, S.-K., Sohn, Y. H., and Lee, P. H. (2012). Volumetric analysis of the substantia innominata in patients with Parkinson’s disease according to cognitive status. Neurobiol. Aging 33, 1265–1272. doi:10.1016/j.neurobiolaging.2010.11.015
Dalton, R. C., Hölscher, C., and Montello, D. R. (2019). Wayfinding as a social activity. Front. Psychol. 10, 142. doi:10.3389/fpsyg.2019.00142
Dickinson, P., Gerling, K., Hicks, K., Murray, J., Shearer, J., and Greenwood, J. (2019). Virtual reality crowd simulation: effects of agent density on user experience and behaviour. Virtual Real. 23, 19–32. doi:10.1007/s10055-018-0365-0
Ekstrom, A. D., and Isham, E. A. (2017). Human spatial navigation: representations across dimensions and scales. Curr. Opin. Behav. Sci. 17, 84–89. doi:10.1016/j.cobeha.2017.06.005
Epstein, R. A., Patai, E. Z., Julian, J. B., and Spiers, H. J. (2017). The cognitive map in humans: spatial navigation and beyond. Nat. Neurosci. 20, 1504–1513. doi:10.1038/nn.4656
Farran, E. K., Formby, S., Daniyal, F., Holmes, T., and Van Herwegen, J. (2016). Route-learning strategies in typical and atypical development; eye tracking reveals atypical landmark selection in Williams syndrome. J. Intellect. Disabil. Res. 60, 933–944. doi:10.1111/jir.12331
Farzanfar, D., Spiers, H. J., Moscovitch, M., and Rosenbaum, R. S. (2023). From cognitive maps to spatial schemas. Nat. Rev. Neurosci. 24, 63–79. doi:10.1038/s41583-022-00655-9
Franke, C., and Schweikart, J. (2017). Mental representation of landmarks on maps: investigating cartographic visualization methods with eye tracking technology. Spatial Cognition and Comput. 17, 20–38. doi:10.1080/13875868.2016.1219912
Gert, A. L., Ehinger, B. V., Kietzmann, T. C., and Konig, P. (2020). “Faces strongly attract early fixations in naturally sampled real-world stimulus materials,” in ACM symposium on eye tracking research and applications (stuttgart Germany: acm), 1–5. doi:10.1145/3379156.3391377
Griesbauer, E.-M., Manley, E., Wiener, J. M., and Spiers, H. J. (2022). London taxi drivers: a review of neurocognitive studies and an exploration of how they build their cognitive map of London. Hippocampus 32, 3–20. doi:10.1002/hipo.23395
Gunalp, P., Moossaian, T., and Hegarty, M. (2019). Spatial perspective taking: effects of social, directional, and interactive cues. Mem. and Cognition 47, 1031–1043. doi:10.3758/s13421-019-00910-y
Harel, A., Kravitz, D. J., and Baker, C. I. (2014). Task context impacts visual object processing differentially across the cortex. Proc. Natl. Acad. Sci. 111, E962–E971. doi:10.1073/pnas.1312567111
Henderson, J. M. (2007). Regarding scenes. Curr. Dir. Psychol. Sci. 16, 219–222. doi:10.1111/j.1467-8721.2007.00507.x
Ito, H. T., Zhang, S.-J., Witter, M. P., Moser, E. I., and Moser, M.-B. (2015). A prefrontal–thalamo–hippocampal circuit for goal-directed spatial navigation. Nature 522, 50–55. doi:10.1038/nature14396
Janzen, G., Wagensveld, B., and Van Turennout, M. (2006). Neural representation of navigational relevance is rapidly induced and long lasting. Cereb. Cortex 17, 975–981. doi:10.1093/cercor/bhl008
Kuehn, E., Chen, X., Geise, P., Oltmer, J., and Wolbers, T. (2018). Social targets improve body-based and environment-based strategies during spatial navigation. Exp. Brain Res. 236, 755–764. doi:10.1007/s00221-018-5169-7
Li, H., Thrash, T., Hölscher, C., and Schinazi, V. R. (2019). The effect of crowdedness on human wayfinding and locomotion in a multi-level virtual shopping mall. J. Environ. Psychol. 65, 101320. doi:10.1016/j.jenvp.2019.101320
Maguire, E. A., Woollett, K., and Spiers, H. J. (2006). London taxi drivers and bus drivers: a structural MRI and neuropsychological analysis. Hippocampus 16, 1091–1101. doi:10.1002/hipo.20233
Malanchini, M., Rimfeld, K., Shakeshaft, N. G., McMillan, A., Schofield, K. L., Rodic, M., et al. (2020). Evidence for a unitary structure of spatial cognition beyond general intelligence. npj Sci. Learn. 5, 9. doi:10.1038/s41539-020-0067-8
Miller, A. M. P., Vedder, L. C., Law, L. M., and Smith, D. M. (2014). Cues, context, and long-term memory: the role of the retrosplenial cortex in spatial cognition. Front. Hum. Neurosci. 8, 586. doi:10.3389/fnhum.2014.00586
Münzer, S., and Hölscher, C. (2011). Entwicklung und Validierung eines Fragebogens zu räumlichen Strategien. Diagnostica 57, 111–125. doi:10.1026/0012-1924/a000040
Neal, Z. P. (2013). “The connected city: how networks are shaping the modern metropolis,” in Metropolis and modern life. 1st ed edn (New York, NY: Routledge).
Nolte, D., Vidal De Palol, M., Keshava, A., Madrid-Carvajal, J., Gert, A. L., Von Butler, E.-M., et al. (2024). Combining EEG and eye-tracking in virtual reality: obtaining fixation-onset event-related potentials and event-related spectral perturbations. Atten. Percept. and Psychophys. 87, 207–227. doi:10.3758/s13414-024-02917-3
Ohm, C., Müller, M., Ludwig, B., and Bienk, S. (2014). Where is the Landmark? Eye Track. Stud. Large-Scale Indoor Environ. Publisher: Universität Regensburg. doi:10.5283/EPUB.31436
Pappalardo, L., Simini, F., Rinzivillo, S., Pedreschi, D., Giannotti, F., and Barabási, A.-L. (2015). Returners and explorers dichotomy in human mobility. Nat. Commun. 6, 8166. doi:10.1038/ncomms9166
Rounds, J. D., Cruz-Garza, J. G., and Kalantari, S. (2020). Using posterior EEG theta band to assess the effects of architectural designs on landmark recognition in an urban setting. Front. Hum. Neurosci. 14, 584385. doi:10.3389/fnhum.2020.584385
Schafer, M., and Schiller, D. (2018). Navigating social space. Neuron 100, 476–489. doi:10.1016/j.neuron.2018.10.006
Schläpfer, M., Dong, L., O’Keeffe, K., Santi, P., Szell, M., Salat, H., et al. (2021). The universal visitation law of human mobility. Nature 593, 522–527. doi:10.1038/s41586-021-03480-9
Schmidt, V., König, S. U., Dilawar, R., Sánchez Pacheco, T., and König, P. (2023). Improved spatial knowledge acquisition through sensory augmentation. Brain Sci. 13, 720. doi:10.3390/brainsci13050720
Slater, M. (2009). Place illusion and plausibility can lead to realistic behaviour in immersive virtual environments. Philosophical Trans. R. Soc. B Biol. Sci. 364, 3549–3557. doi:10.1098/rstb.2009.0138
Summerfield, C., and Egner, T. (2009). Expectation (and attention) in visual cognition. Trends Cognitive Sci. 13, 403–409. doi:10.1016/j.tics.2009.06.003
Tversky, B., and Hard, B. M. (2009). Embodied and disembodied cognition: spatial perspective-taking. Cognition 110, 124–129. doi:10.1016/j.cognition.2008.10.008
Walter, J. L., Essmann, L., König, S. U., and König, P. (2022). Finding landmarks - an investigation of viewing behavior during spatial navigation in VR using a graph-theoretical analysis approach. PLOS Comput. Biol. 18, e1009485. doi:10.1371/journal.pcbi.1009485
West, G. L., Zendel, B. R., Konishi, K., Benady-Chorney, J., Bohbot, V. D., Peretz, I., et al. (2017). Playing Super Mario 64 increases hippocampal grey matter in older adults. PLOS ONE 12, e0187779. doi:10.1371/journal.pone.0187779
Wiener, J. M., Carroll, D., Moeller, S., Bibi, I., Ivanova, D., Allen, P., et al. (2020). A novel virtual-reality-based route-learning test suite: assessing the effects of cognitive aging on navigation. Behav. Res. Methods 52, 630–640. doi:10.3758/s13428-019-01264-8
Wolfe, J. M. (2020). Visual search: how do we find what we are looking for? Annu. Rev. Vis. Sci. 6, 539–562. doi:10.1146/annurev-vision-091718-015048
Keywords: spatial navigation, human agents, virtual reality, exploration-exploitation, social facilitation
Citation: Sánchez Pacheco T, Sarria Mosquera M, Gärtner K, Schmidt V, Nolte D, König SU, Pipa G and König P (2025) The impact of human agents on spatial navigation and knowledge acquisition in a virtual environment. Front. Virtual Real. 6:1497237. doi: 10.3389/frvir.2025.1497237
Received: 16 September 2024; Accepted: 06 March 2025;
Published: 02 April 2025.
Edited by:
Maria Pyasik, University of Udine, ItalyReviewed by:
Adriana Salatino, Royal Military Academy, BelgiumCopyright © 2025 Sánchez Pacheco, Sarria Mosquera, Gärtner, Schmidt, Nolte, König, Pipa and König. This is an open-access article distributed under the terms of the Creative Commons Attribution License (CC BY). The use, distribution or reproduction in other forums is permitted, provided the original author(s) and the copyright owner(s) are credited and that the original publication in this journal is cited, in accordance with accepted academic practice. No use, distribution or reproduction is permitted which does not comply with these terms.
*Correspondence: Tracy Sánchez Pacheco, dHJhY3kuc2FuY2hlei5wYWNoZWNvQHVuaS1vc25hYnJ1ZWNrLmRl
†These authors contributed equally and share senior authorship
Disclaimer: All claims expressed in this article are solely those of the authors and do not necessarily represent those of their affiliated organizations, or those of the publisher, the editors and the reviewers. Any product that may be evaluated in this article or claim that may be made by its manufacturer is not guaranteed or endorsed by the publisher.
Research integrity at Frontiers
Learn more about the work of our research integrity team to safeguard the quality of each article we publish.