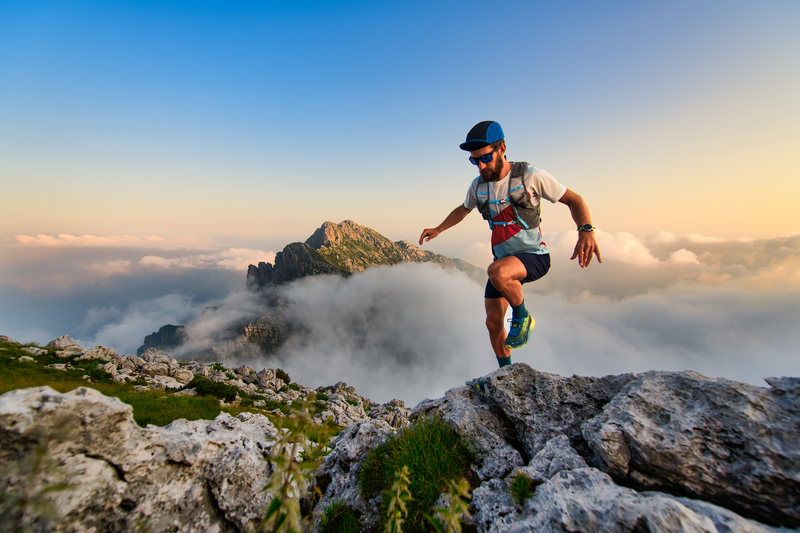
94% of researchers rate our articles as excellent or good
Learn more about the work of our research integrity team to safeguard the quality of each article we publish.
Find out more
PERSPECTIVE article
Front. Virtual Real. , 23 January 2025
Sec. Technologies for VR
Volume 5 - 2024 | https://doi.org/10.3389/frvir.2024.1442774
This article is part of the Research Topic A Metaverse for the Good: Design, Application and Understanding View all 15 articles
In recent years, extended reality (XR) has gained interest as a platform for human communication, with the emergence of the “Metaverse” promising to reshape social interactions. At the same time, concerns about harmful behavior and criminal activities in virtual environments have increased. This paper explores the potential of technology to support social harmony within XR, focusing specifically on audio aspects. We introduce the concept of acoustic coherence and discuss why it is crucial for smooth interaction. We further explain the challenges of speech communication in XR, including noise and reverberation, and review sound processing methods to enhance the auditory experience. We also comment on the potential of using virtual reality as a tool for the development and evaluation of audio algorithms aimed at enhancing communication. Finally, we present the results of a pilot study comparing several audio enhancement techniques inside a virtual environment.
The idea of XR as a platform for human communication has become increasingly popular in recent years. It has led to the emergence of the concept of the “Metaverse,” which is speculated to become the leading medium of communication in the future (Dzardanova et al., 2022; Dwivedi et al., 2022). The primary aim of such virtual worlds is to enable individuals from different locations to interact within a shared audiovisual environment. In parallel to the widespread appreciation for the technological potential and the promise of fostering social connections, there is also a common concern that the Metaverse could facilitate toxic behavior and pose threats of criminal activity (Gómez-Quintero et al., 2024). In response to this, there has been a growing interest in studying ways in which technology could promote social harmony and inclusive behavior within virtual worlds. In this brief paper, we take a closer look at this issue from the perspective of audio technology. We identify challenges related to speech communication in XR and discuss sound processing methods that could improve the overall auditory experience in XR. We support this discussion with a pilot study featuring virtual reality, spatial sound and selected audio enhancement methods, and report the preliminary results obtained from a user feedback survey.
Figure 1A illustrates a common VR meeting scenario where four users, depicted as avatars, gather around a virtual table to engage in a conversation. Because the users are physically located in different spaces, the microphones of their respective headsets, apart from speech, capture additional environment-specific disturbances e.g., noise, background sounds, wind or movement disturbances, and reverberation. Additionally, microphone positioning may not be optimal for capturing voice with high quality (See Figure 1C). The final mixture of sounds delivered to the user originates from several distinct environments. This results in a virtual scene where the acoustic elements fail to form a coherent and plausible soundscape. Coherence in XR refers to the degree to which the virtual environment behaves in a reasonable or predictable way (Collins et al., 2017; Skarbez et al., 2020; Slater, 2009). In this paper, we adopt this concept and use the term acoustic incoherence to describe a lack of a plausible auditory illusion in mixed reality–a problem that has already been discussed by several authors (Neidhardt et al., 2022; Fantini et al., 2023; Popp and Murphy, 2024). Acoustic incoherence may arise from the evident contrast between the acoustics of individual sound sources or from the discrepancies between perceived sounds and their visual representations within the virtual environment. In augmented reality (AR) scenarios (Figure 1B), where remotely recorded sounds must seamlessly integrate with the user’s real soundscape, such incoherence might be even more noticeable.
Figure 1. (A) VR conversation with users at remote locations; (B) AR conversation with users at remote locations; (C) Microphones of the Quest 2 VR Headset; (D) Diagram depicting audio processing pathway for augmented reality conversations. (E) Proposed demo: user interface to change audio settings; (F) Proposed demo: schematic signal flow.
Beyond acoustic incoherence, even in an ideal scenario where all audio sources are perfectly adapted to each user’s environment, group conversations in XR can still lead to the so-called “cocktail party effect,” where multiple people speak at the same time (Cherry, 1953). This situation is particularly challenging because it involves both energetic and informational masking and requires a high level of selective auditory attention (Brungart et al., 2006; Oberfeld and Kloeckner-Nowotny, 2016). Below, we explain why difficult acoustic conditions are a potential bottleneck for interactions in XR.
Human interaction heavily relies on auditory perception. Various studies illustrate how factors such as noise, reverberation, and the inaccuracy of binaural cues can impair our ability to understand speech, localize sound sources in space, or concentrate on specific sounds (Puglisi et al., 2021; Good and Gilkey, 1996; Bronkhorst, 2000). Even when the linguistic message is comprehended, reduced audio quality escalates the effort required for listening, resulting in measurable physiological changes in the body, typically associated with psycho-social stressors (Francis and Love, 2020).
The studies mentioned above focus on measuring how sound degradation affects an individual’s auditory perception and ability to understand speech. While good hearing and speech comprehension are usually prerequisites for communication, passive listening is not the same as active communication. In recent years, there has been growing interest in measuring how acoustic conditions influence communication between groups of people. New study paradigms aim to quantify conversation quality by analyzing changes in speech production, turn-taking time, or movement synchronization between participants (McKellin et al., 2007; Hadley and Ward, 2021; Beechey et al., 2020; Petersen et al., 2022; Petersen, 2024; Sørensen et al., 2021; Hadley et al., 2019). Generally, factors that degrade speech intelligibility or increase listening effort also impact conversation dynamics. Noisy conditions, in particular, force conversation partners to adapt their behavioral strategies to overcome communication challenges.
The influence of adverse acoustic conditions has also been discussed from a social perspective. In Jones et al. (1981) the authors review the existing evidence and propose three main social effects of noise: (a) social interaction is disrupted by the masking of sounds; (b) the weighting of interpersonal judgments is changed; and (c) noisy settings are perceived as aversive, which governs the utility of social engagement. Moreover, multiple studies report the negative effects of noise exposure on the social interactions in the work environment or classroom (Singh et al., 1982; Cohen and Spacapan, 1978).
Communication difficulties and their psycho-social consequences have also been well studied in the hearing-impaired population - a social group inherently exposed to degraded auditory information (Podury et al., 2023; Monzani et al., 2008). People with hearing difficulties are more at risk of social isolation and depression. They perceive the interactions as less successful, and more frustrating and effortful (Aliakbaryhosseinabadi et al., 2023; Beechey et al., 2020).
The studies mentioned above represent well-established research on the perceptual effects of low signal-to-noise ratios, which are typical in complex, real-world acoustic environments and often studied in the context of hearing impairment. However, sound can influence the overall experience in VR in other ways, such as impacting immersion (Geronazzo et al., 2019; Kim and Lee, 2022) and presence (Rogers et al., 2018; Kern and Ellermeier, 2020). For a recent scoping review, see Bosman et al. (2024). Additionally, a few studies have investigated the auditory consequences of audio-visual incongruities unique to XR, such as their effects on distance perception (Gil-Carvajal et al., 2016), localization (Roßkopf et al., 2023), and speech recognition (Siddig et al., 2019). It is important to note that these aspects, even if they do not directly affect auditory perception, may still be important for successful communication.
In summary, although the influence of audio on social interaction in XR still requires more investigation (Bosman et al., 2024), the existing literature suggests that human interactions are likely to be less harmonious in environments with poor acoustics.
Binaural technology is the foundation for most audio experiences in virtual and augmented reality. Through a rigorous preparation of the signal in the left and right audio channels, an auditory illusion of sound being placed at a specific location inside a defined environment can be created. In an XR meeting scenario, the voice captured by the headset on one user’s end becomes a virtual audio source for the other users.
Figure 1D illustrates a possible audio processing pipeline applied to such a recording before reaching the listener’s ears: Initially, the sound emitted by User 1 in Environment 1 –
The depicted pipeline specifically addresses augmented reality, where the target acoustic space is defined by the user’s actual location. Consequently, the properties of the target environment must be estimated through acoustic characterization. In the graph, the user’s own voice recording serves as the source of this information. However, alternative methods exist for estimating room acoustic properties, such as estimating plausible reverberation from images (Chen et al., 2022). In VR setups, this step is typically unnecessary since the reverberation can be simulated from scratch using geometric methods based on polygon meshes in the scene (Välimäki et al., 2016).
The sub-tasks depicted in Figure 1D have been the focus of research in audio and acoustics, leading to diverse approaches utilized in a broad range of audio processing devices. Notably, recent advancements in artificial intelligence are beginning to change the landscape of audio technology. Besides numerous deep learning solutions for individual signal processing tasks, there is a trend of replacing entire conventional audio processing pipelines with end-to-end neural approaches. Table 1 presents a non-exhaustive comparison between traditional signal-processing-based solutions and their deep-learning-based counterparts used for solving the challenges of audio in XR.
Table 1. Traditional and deep-learning-based approaches for the sub-tasks of virtual acoustic simulation.
In previous sections, we outlined the challenges of interactions in virtual reality (VR) that stem from the insufficient quality of acoustic signals, and we discussed the state-of-the-art audio technologies that can help to mitigate these challenges. In this section, we want to draw the reader’s attention to the fact that VR not only benefits from advancements in audio technology but can also serve as a powerful tool for researchers and engineers developing new audio algorithms, particularly in the domain of speech enhancement.
Although most of the speech enhancement algorithms aim at facilitating smooth communication, they are seldom assessed in actual communication settings. Instead, novel algorithms, especially in rapidly evolving fields, are typically evaluated using objective metrics or basic passive listening tests. There are practical reasons for this gap: Setting up an interactive communication scenario in the lab is time-consuming, requires multiple participants, and poses significant challenges in ensuring reproducibility or scaling the study paradigms. VR offers the possibility to simulate real-life environments with highly controlled audio content, visual input and head movements, providing a great platform for detailed testing of speech enhancement algorithms. Additionally, VR can automatically generate large volumes of realistic data samples, which can significantly augment the training and evaluation datasets needed for developing deep learning models.
The idea to use VR to study human interaction, originating from experimental psychology (Pan and Hamilton, 2018), is gaining interest in hearing research (Mehra et al., 2020; Keidser et al., 2020; Hohmann et al., 2020) and musicology (Van Kerrebroeck et al., 2021). In our opinion, VR is likely to become a core technology in developing audio algorithms aimed at enhancing human communication, particularly for assistive listening devices such as consumer earbuds, hands-free communication headsets, hearing aids, and head-mounted displays for XR.
We designed a qualitative pilot experiment to validate the virtual conversation scenes with spatial audio, varying acoustic conditions, and speech enhancement. In this prototype study, users are asked to evaluate aspects of the proposed virtual environment and rate several different speech enhancement algorithms. Below, we describe the technical system, the method for collecting user feedback, and the obtained results.
Using Unity game development software, we created an interactive environment simulating a typical meeting in VR, where four people engage in a conversation. The user is virtually placed at the same table as four virtual characters and has the opportunity to observe and listen to their conversation, while also being able to modify audio processing via the user interface (See Figure 1E). The user interface allows switching between the following audio options:
• COHERENT: original recording of the avatars conversation.
• INCOHERENT: noisy, reverberant audio without signal enhancement.
• CONVENTIONAL AUDIO PROCESSING: spectral noise gating (Sudheer Kumar et al., 2023).
• DEEP LEARNING METHOD FROM 2020: Facebook Denoiser (Defossez et al., 2020).
• DEEP LEARNING METHOD FROM 2023: DeepFilterNet (Schröter et al., 2023).
The signal flow of our VR application is depicted in Figure 1F: Each virtual character was assigned to an audio source extracted from a DiPCo dataset containing close microphone recordings of real conversations (Van Segbroeck et al., 2019). The clean audio sources were corrupted with various noises and reverberations to mimic a real scenario (i.e., each participant located in a different noisy space). The broadband SNR was set to −5 dB for all sources. We used ACE database for room impulse responses (Eaton et al., 2015), AVAD-VR for anechoic recordings of instruments (Thery and Katz, 2019), and Freesound (Fonseca et al., 2021) for other background sounds. Next, the audio was processed by one (or none) of the signal enhancement methods listed in the user interface. The enhanced audio was spatialized based on the relative position between the source and the listener and placed in a simulated acoustic environment i.e., a shoebox room corresponding to the visual scene. We used the Steam Audio spatializer plugin as the binaural synthesis engine. Apart from spatialization, the signal was processed offline. The offline signal enhancement did not affect the binaural reproduction, thereby not limiting the user’s ability to move their head and explore the virtual scene. Nevertheless, it simulated an idealized future scenario where speech enhancement algorithms could process sound in real time without compromising performance. A real VR or AR conversation would require implementing a fully real-time version of these algorithms, which is challenging and still an active field of research (Westhausen et al., 2024). This interactive demonstration was developed as a standalone Meta Quest 2 application.
Using the application described above, we conducted a within-subject experiment with five different acoustic conditions. We invited 11 young participants (age 31–42). Apart from self-reported healthy hearing, there were no other inclusion criteria. All participants volunteered to take part in the study. They were informed in detail about the content of the experiment, agreed to have their survey responses used in the analysis presented here, and were free to withdraw from the demonstration and analysis at any time. No personal information was collected, and only anonymized data was used.
Participants were asked to put on the Quest 2 VR headset coupled with Sennheiser Epos Adapt 360 over-ear headphones. First, they were given an introduction, which consisted of several guided VR scenes. The scenes demonstrated the main audio features in VR and gave examples of acoustically coherent and incoherent scenes in virtual reality. Additionally, a hearing loss simulation by Cuevas-Rodríguez et al. (2019) was presented to raise awareness about auditory perception of individuals with hearing impairments.
After this introduction, the participants were virtually placed in the VR meeting scene (described in detail in the previous subsection), where they could adjust the audio enhancement settings via the user interface. Participants were instructed to consider aspects like speech intelligibility and sound quality. They were encouraged to experiment with audio settings until they formed a clear opinion about each audio processing option. After completing this phase, participants removed the headset and completed an online questionnaire about their experience in the virtual scene. The questionnaire consisted of 7 Likert scale type questions. Likert scales are broadly used in social sciences for the collection of attitudes and opinions (Likert, 1932). They typically consist of 5 options ranging from “strongly disagree” to “strongly agree” (or a similar 5-point symmetrical range of responses with neutral response in the middle) and have been previously used for evaluating soundscapes (Mitchell et al., 2020).
The following questions were presented in the survey:
1. The listening scenario, i.e., audio material, source positions, loudness levels felt realistic (strongly disagree - strongly agree).
2. It was difficult to follow the conversation in the acoustically incoherent scene (strongly disagree - strongly agree).
3. I would have difficulty interacting with people in acoustic conditions similar to the acoustically incoherent scene (strongly disagree - strongly agree).
4. The experiment has raised my awareness about perception of sound (strongly disagree - strongly agree).
5. The experiment has raised my awareness about audio technology (strongly disagree - strongly agree).
6. Rate how much each audio processing method helped you understand speech (did not help at all - helped a lot).
7. Rate the audio quality provided by each audio processing method (very poor - very good).
To analyze the responses we used the Mann–Whitney U test. The collected data is ordinal, meaning the response categories have a natural order but potentially unequal intervals between them. This non-parametric test compares the distributions of two independent groups and does not assume normality, making it suitable for analyzing Likert scale data that may not follow a normal distribution.
A video with the full demonstration including the introduction is available online1.
The survey results are shown in Figure 2. In Question 1, most participants agreed that the selected listening scenario was realistic, supporting the expectation that virtual reality can create ecologically valid acoustic scenarios, as proposed by Hohmann et al. (2020) and Mehra et al. (2020).
Figure 2. Results for 7 survey questions performed by the users after participating in the study. Upper panel: Pie charts showing the results of 5 out of 7 questions. Bottom panel: Stacked bar charts representing the results of the remaining 2 survey questions. Statistical significance was computed with the Mann–Whitney U test. The symbol ns stands for
In Question 2 and 3, participants agreed that the incoherent acoustic scene represented conditions in which it would be difficult to follow the conversation and interact with others. In additional interviews, they reported that the acoustic coherence had a crucial impact on the willingness to take part in the VR meeting and the ability to associate individual voices with the avatars. The result of Question 2 confirms the well-known finding that speech intelligibility is impaired by noise or reverberation (Bradley, 1986; Bronkhorst, 2000). The results for Question 3 suggest that not only speech comprehension, but also interpersonal interactions, are negatively affected by poor acoustics, which is in agreement with the findings of McKellin et al. (2007) and Hadley et al. (2019) who studied real conversations in noisy settings.
Questions 4 and 5 assessed the potential educational impact of participating in the experiment. The results indicate that for most of them, the experiment increased awareness of sound perception and audio technology, although not all participants experienced this benefit.
Questions 6 and 7 rated three speech enhancement methods. While it was evident that all methods improved the auditory experience to some degree, participants reported distinct preferences when evaluating the methods. The survey questions were formulated to gather ratings reflecting speech intelligibility (Question 6) and perceived sound quality (Question 7) for each algorithm. The DNN-based solution by Schröter et al. (2023) was regarded as the most beneficial for understanding speech and yielding the highest signal quality. The second-best option in both categories was the DNN-based method by Defossez et al. (2020). Finally, the conventional speech enhancement technique (Sudheer Kumar et al., 2023) was rated as the least helpful, providing only marginal improvement in speech perception and exhibiting poor audio quality. These results suggest that while traditional signal processing may be insufficient for improving communication in XR, recent deep learning approaches show significant potential.
The benefit of DNN-based techniques over traditional methods has been reported in multiple studies (Xu et al., 2013; Zheng et al., 2023). This benefit is generally attributed to the fact that DNNs do not rely on the unrealistic assumptions about the statistical properties of speech and noise that constrain the performance of traditional methods. However, it should be noted that end-to-end deep learning approaches, such as those used in this pilot study, remain computationally expensive and may be challenging to implement in real-time on mobile devices.
Only a few studies in the context of hearing aid signal enhancement have compared various speech enhancement techniques in complex acoustic environments (Gusó et al., 2023; Westhausen et al., 2024; Hendrikse et al., 2020). Furthermore, most evaluations rely on objective metrics, which have been criticized for providing only a limited view of how humans perceive sound (Torcoli et al., 2021; López-Espejo et al., 2023). To our knowledge, there is no prior research comparing traditional and DNN-based speech enhancement methods subjectively in audio-visual VR.
Although the method by Defossez et al. (2020) received slightly higher ratings than Sudheer Kumar et al. (2023), this difference was not statistically significant in either aspect (see Figure 2, bottom panel). Informal listening reports indicate that while the DNN is better at removing noise, it distorts the signal in a way that makes listening to the conversation unpleasant. This suggests that perceptually distinct signals can result in similar effective speech enhancement and comparable assessed quality. Hence, despite the benefit of SNR, we might expect similar communication benefit. Further studies are needed to quantify how different types of distortions interact with binaural XR environments and if such differences between methods influence social interactions.
Collecting participant opinions is an efficient way to probe perception. In this pilot study, we used this approach to explore new research ideas. However, to validate these findings, future studies will require quantitative measures of communication success.
In this article, we presented our perspective on how contemporary audio technologies enhance social interaction in extended reality (XR). Our main goal was to conceptually link technical topics in XR and audio technology with concepts from the psychology of human interaction, particularly speech communication and hearing science.
Extensive research in psychology and auditory science shows that sound is fundamental to human communication ? However, ideal auditory input is often difficult or impossible to achieve. Whether it’s noisy environments encountered in daily life, partially inaudible sounds for individuals with hearing impairments, or remote meetings where sound undergoes multiple modifications before reaching users’ ears, imperfect sound can hinder effective communication (Balters et al., 2023; Beechey et al., 2020; Hadley et al., 2019; McKellin et al., 2007).
In this work, we focused on the challenges of achieving ideal audio signals in remote XR meeting scenarios. Beyond well-known issues like background noise and the “cocktail party” effect, XR presents additional challenges, such as incongruences between individual audio sources or between audio and visual virtual representations (Neidhardt et al., 2022). Distortions introduced by the signal processing pipeline, which involves multiple sub-tasks, may result in signals unfamiliar to our ears. Even with perfect hearing, the brain may struggle to adapt quickly to these synthetic sounds (Willmore and King, 2023).
We introduced the concept of “acoustic incoherence” to describe these new audio challenges posed by XR technology and emphasized the importance of coherent acoustic scenes for smooth interaction in XR. We briefly reviewed existing solutions to the sub-tasks of virtual acoustic simulation, mentioning deep-learning-based alternatives to conventional approaches, which are likely to dominate digital audio processing in the near future.
Although it is clear that sound influences interaction, few studies examine this influence in actual interactive settings (McKellin et al., 2007). Similarly, while the ultimate goal of speech enhancement is to improve communication, speech enhancement algorithms are rarely evaluated in interactive communication settings (Keidser et al., 2020). VR provides a valuable platform for creating realistic evaluation scenarios for hearing research and audio algorithm development. We believe that, in the long term, prioritizing communication benefits will transform speech enhancement algorithms into tools that enhance human interactions, enabling users to experience more harmonious social connections.
To support our perspective, we presented a pilot study conducted in virtual reality comparing classical and deep-learning-based approaches to speech enhancement. The results indicate that deep-learning-based methods hold potential for improving communication but also suggest that even de-noised signal retain distortions that can be detrimental in conversational scenarios. These initial results, which we plan to complement with a more formal study in the future, underscore the significance of speech enhancement in virtual reality interactions and demonstrate how VR can be used to evaluate novel audio processing algorithms.
In recent years, assistive and multimedia technologies have advanced significantly, with many low-level challenges, such as denoising, nearly resolved. Consequently, the focus is shifting towards a broader, more integrative view of how users interact with technology and each other (Pan and Hamilton, 2018; Billinghurst et al., 2024). Developing new algorithms increasingly requires expertise from human-centered fields such as psychology and sociology (Gregori et al., 2023). This paper examines existing audio technology through the lens of human interactions and presents an original perspective on communication in XR, supporting a multidisciplinary approach in research and technology.
The raw data supporting the conclusions of this article will be made available by the authors, without undue reservation.
No ethical approval was required for studies involving human participants. All participants were informed about the content of the demonstration and agreed to participate, as well as to have their responses to the final survey used for the analysis presented in the article. The surveys were completely anonymous, and participants were informed that they could withdraw from the demonstration and the analysis at any time. No personal data of the participants was processed; only fully anonymized data was collected and handled. The studies were conducted in accordance with national legislation and institutional requirements. Written informed consent to participate was not required from participants or their legal guardians/family members, in accordance with national legislation and institutional requirements, as no personal data of the participants was collected at any point. The participants consented to participate in the studies and agreed to complete the online form, being fully informed about the study, their rights, and the use of anonymized data to ensure confidentiality.
JL: Writing–original draft, Writing–review and editing. EG: Writing–review and editing. US: Writing–review and editing. AG: Writing–review and editing.
The author(s) declare that financial support was received for the research, authorship, and/or publication of this article. This project has received funding from the Horizon 2020 programme under grant agreement No. 101017884. This work reflects only the author’s view. Neither the European Commission nor the agency is responsible for any use that may be made of the information it contains.
The authors declare that the research was conducted in the absence of any commercial or financial relationships that could be construed as a potential conflict of interest.
All claims expressed in this article are solely those of the authors and do not necessarily represent those of their affiliated organizations, or those of the publisher, the editors and the reviewers. Any product that may be evaluated in this article, or claim that may be made by its manufacturer, is not guaranteed or endorsed by the publisher.
1Video available at https://www.youtube.com/watch?v=SaXMYn8b3eg&t=124s.
Aliakbaryhosseinabadi, S., Keidser, G., May, T., Dau, T., Wendt, D., and Rotger-Griful, S. (2023). The effects of noise and simulated conductive hearing loss on physiological response measures during interactive conversations. J. Speech, Lang. Hear. Res. 66, 4009–4024. doi:10.1044/2023_jslhr-23-00063
Balters, S., Miller, J. G., Li, R., Hawthorne, G., and Reiss, A. L. (2023). Virtual (zoom) interactions alter conversational behavior and interbrain coherence. J. Neurosci. 43, 2568–2578. doi:10.1523/jneurosci.1401-22.2023
Beechey, T., Buchholz, J. M., and Keidser, G. (2020). Hearing impairment increases communication effort during conversations in noise. J. Speech, Lang. Hear. Res. 63, 305–320. doi:10.1044/2019_jslhr-19-00201
Billinghurst, M., Cesare Than 50 Years of Arr, P., Gonzalez-Franco, M., Isbister, K., Williamson, J., and Kitson, A. (2024). Social xr: the future of communication and collaboration. dag. semi. 23482. 13(11), 30. doi:10.4230/DagRep.13.11.167
Bosman, I. d. V., Buruk, O. â., Jørgensen, K., and Hamari, J. (2024). The effect of audio on the experience in virtual reality: a scoping review. Behav. & Inf. Technol. 43, 165–199. doi:10.1080/0144929x.2022.2158371
Bradley, J. S. (1986). Predictors of speech intelligibility in rooms. J. Acoust. Soc. Am. 80, 837–845. doi:10.1121/1.393907
Bronkhorst, A. W. (2000). The cocktail party phenomenon: a review of research on speech intelligibility in multiple-talker conditions. Acta acustica united acustica 86, 117–128. Available at https://www.ingentaconnect.com/content/dav/aaua/2000/00000086/00000001/art00016
Brungart, D. S., Chang, P. S., Simpson, B. D., and Wang, D. (2006). Isolating the energetic component of speech-on-speech masking with ideal time-frequency segregation. J. Acoust. Soc. Am. 120, 4007–4018. doi:10.1121/1.2363929
Chen, C., Gao, R., Calamia, P., and Grauman, K. (2022). “Visual acoustic matching,” in Proceedings of the IEEE/CVF conference on computer vision and pattern recognition, 18858–18868.
Cherry, E. C. (1953). Some experiments on the recognition of speech, with one and with two ears. J. Acoust. Soc. Am. 25, 975–979. doi:10.1121/1.1907229
Cohen, S., and Spacapan, S. (1978). The aftereffects of stress: an attentional interpretation. Environ. Psychol. nonverbal Behav. 3, 43–57. doi:10.1007/bf01114531
Collins, J., Regenbrecht, H., and Langlotz, T. (2017). Visual coherence in mixed reality: a systematic enquiry. Presence 26, 16–41. doi:10.1162/pres_a_00284
Cuevas Rodriguez, M. (2022). 3D binaural spatialisation for virtual reality and psychoacoustics. PhD diss. Málaga, Spain: Universidad de Málaga
Cuevas-Rodríguez, M., Picinali, L., González-Toledo, D., Garre, C., de la Rubia-Cuestas, E., Molina-Tanco, L., et al. (2019). 3d tune-in toolkit: an open-source library for real-time binaural spatialisation. PloS one 14–e0211899. doi:10.1371/journal.pone.0211899
Defossez, A., Synnaeve, G., and Adi, Y. (2020). Real time speech enhancement in the waveform domain. Proc. Interspeech 3291–3295. doi:10.21437/Interspeech.2020-2409
Dwivedi, Y. K., Hughes, L., Baabdullah, A. M., Ribeiro-Navarrete, S., Giannakis, M., Al-Debei, M. M., et al. (2022). Metaverse beyond the hype: multidisciplinary perspectives on emerging challenges, opportunities, and agenda for research, practice and policy. Int. J. Inf. Manag. 66, 102542. doi:10.1016/j.ijinfomgt.2022.102542
Dzardanova, E., Kasapakis, V., Gavalas, D., and Sylaiou, S. (2022). Virtual reality as a communication medium: a comparative study of forced compliance in virtual reality versus physical world. Virtual Real. 26, 737–757. doi:10.1007/s10055-021-00564-9
Eaton, J., Gaubitch, N. D., Moore, A. H., and Naylor, P. A. (2015). “The ace challengeacorpus description and performance evaluation,” in 2015 IEEE workshop on applications of signal processing to audio and acoustics (WASPAA) (IEEE), 1–5.
Fantini, D., Presti, G., Geronazzo, M., Bona, R., Privitera, A. G., and Avanzini, F. (2023). Co-immersion in audio augmented virtuality: the case study of a static and approximated late reverberation algorithm. IEEE Trans. Vis. Comput. Graph. 29, 4472–4482. doi:10.1109/tvcg.2023.3320213
Fonseca, E., Favory, X., Pons, J., Font, F., and Serra, X. (2021). Fsd50k: an open dataset of human-labeled sound events. IEEE/ACM Trans. Audio, Speech, Lang. Process. 30, 829–852. doi:10.1109/taslp.2021.3133208
Francis, A. L., and Love, J. (2020). Listening effort: are we measuring cognition or affect, or both? Wiley Interdiscip. Rev. Cognitive Sci. 11, e1514. doi:10.1002/wcs.1514
Gari, S. V. A., Robinson, P. W., and Calamia, P. T. (2022). “Room acoustic characterization for binaural rendering: from spatial room impulse responses to deep learning,” in International congress on acoustics.
Geronazzo, M., Rosenkvist, A., Eriksen, D. S., Markmann-Hansen, C. K., Køhlert, J., Valimaa, M., et al. (2019). Creating an audio story with interactive binaural rendering in virtual reality. Wirel. Commun. Mob. Comput. 2019, 1–14. doi:10.1155/2019/1463204
Gil-Carvajal, J. C., Cubick, J., Santurette, S., and Dau, T. (2016). Spatial hearing with incongruent visual or auditory room cues. Sci. Rep. 6, 37342. doi:10.1038/srep37342
Gómez-Quintero, J., Johnson, S. D., Borrion, H., and Lundrigan, S. (2024). A scoping study of crime facilitated by the metaverse. Futures 157, 103338. doi:10.1016/j.futures.2024.103338
Good, M. D., and Gilkey, R. H. (1996). Sound localization in noise: the effect of signal-to-noise ratio. J. Acoust. Soc. Am. 99, 1108–1117. doi:10.1121/1.415233
Gregori, A., Amici, F., Brilmayer, I., Ćwiek, A., Fritzsche, L., Fuchs, S., et al. (2023). “A roadmap for technological innovation in multimodal communication research,” in International conference on human-computer interaction (Springer), 402–438.
Gusó, E., Luberadzka, J., Baig, M., Sayin, U., and Serra, X. (2023). “An objective evaluation of hearing aids and dnn-based binaural speech enhancement in complex acoustic scenes,” in 2023 IEEE workshop on applications of signal processing to audio and acoustics (WASPAA) (IEEE), 1–5.
Hadley, L. V., Brimijoin, W. O., and Whitmer, W. M. (2019). Speech, movement, and gaze behaviours during dyadic conversation in noise. Sci. Rep. 9, 10451. doi:10.1038/s41598-019-46416-0
Hadley, L. V., and Ward, J. A. (2021). Synchrony as a measure of conversation difficulty: movement coherence increases with background noise level and complexity in dyads and triads. PLoS One 16, e0258247. doi:10.1371/journal.pone.0258247
Hendrikse, M. M., Grimm, G., and Hohmann, V. (2020). Evaluation of the influence of head movement on hearing aid algorithm performance using acoustic simulations. Trends Hear. 24, 2331216520916682. doi:10.1177/2331216520916682
Hohmann, V., Paluch, R., Krueger, M., Meis, M., and Grimm, G. (2020). The virtual reality lab: realization and application of virtual sound environments. Ear Hear. 41, 31S–38S. doi:10.1097/aud.0000000000000945
Hua, Y. (2002). Blind methods of system identification. Circuits, Syst. Signal Process. 21, 91–108. doi:10.1007/bf01211654
Huang, W. C., Markovic, D., Richard, A., Gebru, I. D., and Menon, A. (2022). End-to-end binaural speech synthesis. arXiv preprint arXiv:2207.03697. 1218, 1222. doi:10.21437/interspeech.2022-10603
Im, J., and Nam, J. (2024). Diffrent: a diffusion model for recording environment transfer of speech. arXiv Prepr. arXiv:2401.08102, 7425–7429. doi:10.1109/icassp48485.2024.10447818
Jones, D. M., Chapman, A. J., and Auburn, T. C. (1981). Noise in the environment: a social perspective. J. Environ. Psychol. 1, 43–59. doi:10.1016/s0272-4944(81)80017-5
Keidser, G., Naylor, G., Brungart, D. S., Caduff, A., Campos, J., Carlile, S., et al. (2020). The quest for ecological validity in hearing science: what it is, why it matters, and how to advance it. Ear Hear. 41, 5S–19S. doi:10.1097/aud.0000000000000944
Kendrick, P., Li, F. F., Cox, T. J., Zhang, Y., and Chambers, J. A. (2007). Blind estimation of reverberation parameters for non-diffuse rooms. Acta Acustica united Acustica 93, 760–770. Available at https://api.semanticscholar.org/CorpusID:115380484
Kern, A. C., and Ellermeier, W. (2020). Audio in vr: effects of a soundscape and movement-triggered step sounds on presence. Front. Robotics AI 7, 20. doi:10.3389/frobt.2020.00020
Kim, H., and Lee, I.-K. (2022). Studying the effects of congruence of auditory and visual stimuli on virtual reality experiences. IEEE Trans. Vis. Comput. Graph. 28, 2080–2090. doi:10.1109/tvcg.2022.3150514
Koo, J., Paik, S., and Lee, K. (2021). “Reverb conversion of mixed vocal tracks using an end-to-end convolutional deep neural network,” in ICASSP 2021-2021 IEEE international conference on acoustics, speech and signal processing (ICASSP) (IEEE), 81–85.
Krishnamoorthy, P., and Prasanna, S. M. (2009). Temporal and spectral processing methods for processing of degraded speech: a review. IETE Tech. Rev. 26, 137–148. doi:10.4103/0256-4602.49103
Li, Y., and Deng, L. (2021). “An overview of speech dereverberation,” in Proceedings of the 8th conference on sound and music technology: selected papers from CSMT (Springer), 134–146.
Likert, R. (1932). A technique for measurement of attitudes. Archives Psychol. 140, 5–55. Available at https://psycnet.apa.org/record/1933-01885-001
Lluís, F., Chatziioannou, V., and Hofmann, A. (2022). Points2sound: from mono to binaural audio using 3d point cloud scenes. EURASIP J. Audio, Speech, Music Process. 2022, 33–15. doi:10.1186/s13636-022-00265-4
López-Espejo, I., Edraki, A., Chan, W.-Y., Tan, Z.-H., and Jensen, J. (2023). On the deficiency of intelligibility metrics as proxies for subjective intelligibility. Speech Commun. 150, 9–22. doi:10.1016/j.specom.2023.04.001
Martin, I., Pastor, F., Fuentes-Hurtado, F., Belloch, J., Azpicueta-Ruiz, L., Naranjo, V., et al. (2023). “Predicting room impulse responses through encoder-decoder convolutional neural networks,” in 2023 IEEE 33rd international workshop on machine learning for signal processing (MLSP) (IEEE), 1–6.
McKellin, W. H., Shahin, K., Hodgson, M., Jamieson, J., and Pichora-Fuller, K. (2007). Pragmatics of conversation and communication in noisy settings. J. Pragmat. 39, 2159–2184. doi:10.1016/j.pragma.2006.11.012
Mehra, R., Brimijoin, O., Robinson, P., and Lunner, T. (2020). Potential of augmented reality platforms to improve individual hearing aids and to support more ecologically valid research. Ear Hear. 41, 140S–146S. doi:10.1097/aud.0000000000000961
Mitchell, A., Oberman, T., Aletta, F., Erfanian, M., Kachlicka, M., Lionello, M., et al. (2020). The soundscape indices (ssid) protocol: a method for urban soundscape surveys—questionnaires with acoustical and contextual information. Appl. Sci. 10, 2397. doi:10.3390/app10072397
Monzani, D., Galeazzi, G. M., Genovese, E., Marrara, A., and Martini, A. (2008). Psychological profile and social behaviour of working adults with mild or moderate hearing loss. Acta Otorhinolaryngol. Ital. 28, 61–66. Available at https://pmc.ncbi.nlm.nih.gov/articles/PMC2644978/
Nakatani, T., Kinoshita, K., and Miyoshi, M. (2006). Harmonicity-based blind dereverberation for single-channel speech signals. IEEE Trans. Audio, Speech, Lang. Process. 15, 80–95. doi:10.1109/tasl.2006.872620
Neidhardt, A., Schneiderwind, C., and Klein, F. (2022). Perceptual matching of room acoustics for auditory augmented reality in small rooms-literature review and theoretical framework. Trends Hear. 26, 23312165221092919. doi:10.1177/23312165221092919
Oberfeld, D., and Kloeckner-Nowotny, F. (2016). Individual differences in selective attention predict speech identification at a cocktail party. Elife 5, e16747. doi:10.7554/elife.16747
Ochieng, P. (2023). Deep neural network techniques for monaural speech enhancement and separation: state of the art analysis. Artif. Intell. Rev. 56, 3651–3703. doi:10.1007/s10462-023-10612-2
Pan, X., and Hamilton, A. F. d. C. (2018). Why and how to use virtual reality to study human social interaction: the challenges of exploring a new research landscape. Br. J. Psychol. 109, 395–417. doi:10.1111/bjop.12290
Pascual, S., Bonafonte, A., and Serra, J. (2017). SEGAN: speech enhancement generative adversarial network. Proc. Interspeech 2017, 3642–3646. doi:10.21437/Interspeech.2017-1428
Peters, N., Choi, J., and Lei, H. (2012). “Matching artificial reverb settings to unknown room recordings: a recommendation system for reverb plugins,” in 133rd AES Convention, San Francisco, CA, USA, October 26–29, 2012.
Petersen, E. B. (2024). Investigating conversational dynamics in triads: effects of noise, hearing impairment, and hearing aids. Front. Psychol. 15, 1289637. doi:10.3389/fpsyg.2024.1289637
Petersen, E. B., MacDonald, E. N., and Josefine Munch Sørensen, A. (2022). The effects of hearing-aid amplification and noise on conversational dynamics between normal-hearing and hearing-impaired talkers. Trends Hear. 26, 23312165221103340. doi:10.1177/23312165221103340
Podury, A., Jiam, N. T., Kim, M., Donnenfield, J. I., and Dhand, A. (2023). Hearing and sociality: the implications of hearing loss on social life. Front. Neurosci. 17, 1245434. doi:10.3389/fnins.2023.1245434
Popp, C., and Murphy, D. T. (2024). Speech intelligibility versus congruency: user preferences of the acoustics of virtual reality game spaces. Virtual Worlds (MDPI) 3, 40–61. doi:10.3390/virtualworlds3010003
Puglisi, G. E., Warzybok, A., Astolfi, A., and Kollmeier, B. (2021). Effect of reverberation and noise type on speech intelligibility in real complex acoustic scenarios. Build. Environ. 204, 108137. doi:10.1016/j.buildenv.2021.108137
Rafaely, B., Tourbabin, V., Habets, E., Ben-Hur, Z., Lee, H., Gamper, H., et al. (2022). Spatial audio signal processing for binaural reproduction of recorded acoustic scenes–review and challenges. Acta Acust. 6, 47. doi:10.1051/aacus/2022040
Ratnam, R., Jones, D. L., Wheeler, B. C., OaBrien Jr, W. D., Lansing, C. R., and Feng, A. S. (2003). Blind estimation of reverberation time. J. Acoust. Soc. Am. 114, 2877–2892. doi:10.1121/1.1616578
Rogers, K., Ribeiro, G., Wehbe, R. R., Weber, M., and Nacke, L. E. (2018). “Vanishing importance: studying immersive effects of game audio perception on player experiences in virtual reality,” in Proceedings of the 2018 CHI conference on human factors in computing systems, 1–13.
Roßkopf, S., Kroczek, L. O., Stärz, F., Blau, M., Van de Par, S., and Mühlberger, A. (2023). The effect of audio-visual room divergence on the localization of real sound sources in virtual reality (DAGA). Fortschritte Akust, Hamburg: DAGA, 1431–1434.
Schröter, H., Rosenkranz, T., Maier, A., et al. (2023). Deepfilternet: perceptually motivated real-time speech enhancement. arXiv Prepr. arXiv:2305.08227. doi:10.48550/arXiv.2305.08227
Siddig, A., Sun, P. W., Parker, M., and Hines, A. (2019). Perception deception: audio-visual mismatch in virtual reality using the mcgurk effect. AICS 2019, 176–187. Available at https://api.semanticscholar.org/CorpusID:211125288
Singh, A. P., Rai, R. M., Bhatia, M. R., and Nayar, H. S. (1982). Effect of chronic and acute exposure to noise on physiological functions in man. Internat. Arc. Occupati. Environ. Health 50, 169–174.
Skarbez, R., Brooks, F. P., and Whitton, M. C. (2020). Immersion and coherence: research agenda and early results. IEEE Trans. Vis. Comput. Graph. 27, 3839–3850. doi:10.1109/tvcg.2020.2983701
Slater, M. (2009). Place illusion and plausibility can lead to realistic behaviour in immersive virtual environments. Philosophical Trans. R. Soc. B Biol. Sci. 364, 3549–3557. doi:10.1098/rstb.2009.0138
Sørensen, A. J. M., Fereczkowski, M., and MacDonald, E. N. (2021). Effects of noise and second language on conversational dynamics in task dialogue. Trends Hear. 25, 23312165211024482. doi:10.1177/23312165211024482
Steinmetz, C. J., Ithapu, V. K., and Calamia, P. (2021). “Filtered noise shaping for time domain room impulse response estimation from reverberant speech,” in 2021 IEEE workshop on applications of signal processing to audio and acoustics (WASPAA) (IEEE), 221–225.
Su, J., Jin, Z., and Finkelstein, A. (2020a). “Acoustic matching by embedding impulse responses,” in ICASSP 2020-2020 IEEE international conference on acoustics, speech and signal processing (ICASSP) (IEEE), 426–430.
Su, J., Jin, Z., and Finkelstein, A. (2020b). Hifi-gan: high-fidelity denoising and dereverberation based on speech deep features in adversarial networks. Proc. Interspeech 2020, 4506–4510. doi:10.21437/Interspeech.2020-2143
Sudheer Kumar, E., Jai Surya, K., Yaswanth Varma, K., Akash, A., and Nithish Reddy, K. (2023). “Noise reduction in audio file using spectral gatting and fft by python modules,” in Recent developments in electronics and communication systems (IOS Press), 510–515. doi:10.3233/ATDE221305
Thery, D., and Katz, B. F. (2019). “Anechoic audio and 3d-video content database of small ensemble performances for virtual concerts,” in Intl cong on acoustics (ICA).
Torcoli, M., Kastner, T., and Herre, J. (2021). Objective measures of perceptual audio quality reviewed: an evaluation of their application domain dependence. IEEE/ACM Trans. Audio, Speech, Lang. Process. 29, 1530–1541. doi:10.1109/taslp.2021.3069302
Välimäki, V., Parker, J., Savioja, L., Smith, J. O., and Abel, J. (2016). “More than 50 years of artificial reverberation,” in Audio engineering society conference: 60th international conference: dreams (dereverberation and reverberation of audio, music, and speech) (United States: Audio Engineering Society).
Van Kerrebroeck, B., Caruso, G., and Maes, P.-J. (2021). A methodological framework for assessing social presence in music interactions in virtual reality. Front. Psychol. 12, 663725. doi:10.3389/fpsyg.2021.663725
Van Segbroeck, M., Zaid, A., Kutsenko, K., Huerta, C., Nguyen, T., Luo, X., et al. (2019). Dipco–dinner party corpus. arXiv Prepr. arXiv:1909.13447. doi:10.48550/arXiv.1909.13447
Virag, N. (1999). Single channel speech enhancement based on masking properties of the human auditory system. IEEE Trans. speech audio Process. 7, 126–137. doi:10.1109/89.748118
Westhausen, N. L., Kayser, H., Jansen, T., and Meyer, B. T. (2024). Real-time multichannel deep speech enhancement in hearing aids: comparing monaural and binaural processing in complex acoustic scenarios. arXiv Prepr. arXiv:2405.01967 32, 4596–4606. doi:10.1109/taslp.2024.3473315
Willmore, B. D., and King, A. J. (2023). Adaptation in auditory processing. Physiol. Rev. 103, 1025–1058. doi:10.1152/physrev.00011.2022
Xu, Y., Du, J., Dai, L.-R., and Lee, C.-H. (2013). An experimental study on speech enhancement based on deep neural networks. IEEE Signal Process. Lett. 21, 65–68. doi:10.1109/lsp.2013.2291240
Yuliani, A. R., Amri, M. F., Suryawati, E., Ramdan, A., and Pardede, H. F. (2021). Speech enhancement using deep learning methods: a review. J. Elektron. Dan. Telekomun. 21, 19–26. doi:10.14203/jet.v21.19-26
Zheng, C., Zhang, H., Liu, W., Luo, X., Li, A., Li, X., et al. (2023). Sixty years of frequency-domain monaural speech enhancement: from traditional to deep learning methods. Trends Hear. 27, 23312165231209913. doi:10.1177/23312165231209913
Keywords: social interaction, extended reality, virtual acoustic simulation, acoustic matching, speech enhancement
Citation: Luberadzka J, Gusó Muñoz E, Sayin U and Garriga A (2025) Audio technology for improving social interaction in extended reality. Front. Virtual Real. 5:1442774. doi: 10.3389/frvir.2024.1442774
Received: 02 June 2024; Accepted: 31 December 2024;
Published: 23 January 2025.
Edited by:
Xueni Pan, Goldsmiths University of London, United KingdomReviewed by:
Weiya Chen, Huazhong University of Science and Technology, ChinaCopyright © 2025 Luberadzka, Gusó Muñoz, Sayin and Garriga. This is an open-access article distributed under the terms of the Creative Commons Attribution License (CC BY). The use, distribution or reproduction in other forums is permitted, provided the original author(s) and the copyright owner(s) are credited and that the original publication in this journal is cited, in accordance with accepted academic practice. No use, distribution or reproduction is permitted which does not comply with these terms.
*Correspondence: Joanna Luberadzka, am9hbm5hLmx1YmVyYWR6a2FAZXVyZWNhdC5vcmc=
Disclaimer: All claims expressed in this article are solely those of the authors and do not necessarily represent those of their affiliated organizations, or those of the publisher, the editors and the reviewers. Any product that may be evaluated in this article or claim that may be made by its manufacturer is not guaranteed or endorsed by the publisher.
Research integrity at Frontiers
Learn more about the work of our research integrity team to safeguard the quality of each article we publish.