- 1Department of Psychology, TU Dresden, Dresden, Germany
- 2School of Psychology, Aston University, Birmingham, United Kingdom
- 3School of Psychology, University College Dublin, Dublin, Ireland
Introduction: Intersections are particularly complex traffic situations and are often the scene of accidents. Driver behaviour and decision-making might be affected by specific factors such as the right of way, traffic volume, and the occurrence of a critical event directly before the intersection.
Methods: We developed a new driving scenario in virtual reality (VR) to test the impact of these factors using a fully immersive head-mounted display. Participants had to navigate through a series of intersections to reach their target destination. We recorded their driving behaviour as well as their brain activity using electroencephalography (EEG).
Results: Our results showed that participants engaged cognitive control processes when approaching an intersection with high traffic volume and when reacting to a critical event, as indexed by driving behaviour and proactively by increased theta power. We did not find differences for right of way in the EEG data, but driving behaviour was as expected, revealing a driving speed reduction when participants had to yield to traffic.
Discussion: We discuss advantages and potential challenges of an immersive VR-based approach to driving simulations and the challenges encountered when recording and analysing EEG data. We conclude that despite movement and electronic artefacts, EEG data in the theta and alpha bands can be analysed robustly and allow for novel insights into control processes in realistic VR scenarios.
Introduction
For many of us, driving a car is a normal part of our daily lives. At the same time, driving is a complex task that requires an intricate interplay of attentional processes, cognitive control and motor coordination. For example, drivers are required to manoeuvre their car (e.g., controlling speed and lane position, shifting gears) while at the same time constantly scanning their environment (e.g., for information from road signs or for monitoring the behaviour of other road users) and potentially reacting to obstacles (e.g., swerving or braking if a pedestrian unexpectedly enters the road). Lapses of attention or fatigue can have severe consequences in the form of accidents. One particular hotspot for accidents are intersections. For example, one-third of all crashes in the United States (NHTSA, 2020) and 43% of injury accidents in Europe occur at intersections (Simon et al., 2023).
One reason might be that intersections—especially ones not controlled by traffic lights—are highly complex traffic situations where right of way is governed by specific traffic rules that need to be obeyed, other road users might be involved, and navigational decisions have to be made. Drivers have to constantly shift and re-focus their attention (e.g., Huizeling et al., 2020). This is particularly relevant when they have to yield to other road users (Werneke and Vollrath, 2012; Ringhand et al., 2022), when they are distracted by an unexpected event such as a pedestrian crossing the road (Metz et al., 2011; Ericson et al., 2017), and when traffic volume is high (Werneke and Vollrath, 2014; Ericson et al., 2017; Ringhand et al., 2022). Hence, it is important to understand the underlying cognitive processes of how drivers deal with these situations and how these affect driving behaviour at intersections. To this aim, electroencephalography (EEG) can be used to record brain activity while driving. Taking advantage of the high temporal resolution of EEG, it is possible to use EEG to track drivers’ attention and fatigue levels (Lin et al., 2007; Guo et al., 2018; Wascher et al., 2023) and to predict when they are about to brake (Haufe et al., 2011; 2014; Nguyen and Chung, 2019). In addition, EEG provides insights into how drivers process and respond to increased cognitive load and allocate their attention before and after critical events (Huizeling et al., 2020). Here, theta power (oscillations between 4 and 7 Hz) and alpha power (oscillations between 8 and 12 Hz) have been reported to play an important role. Increased theta power is an indicator for increased cognitive control and mental effort and typically has a midfrontal topography (Nigbur et al., 2011; Cavanagh et al., 2012; Cavanagh and Frank, 2014; Cohen, 2016; Zavala et al., 2018), while decreased alpha power is an indicator for increased attention and typically has a posterior topography (Klimesch et al., 2007; Hanslmayr et al., 2011; Klimesch, 2012; Peylo et al., 2021). For example, Getzmann and colleagues (2018) found increased theta power and decreased alpha power under more difficult driving conditions (e.g., shaper bends of the road) compared to easy driving conditions. Huizeling et al. (2020) showed that theta power increased when participants reacted to a car braking in front of them and when refocusing their attention to task-relevant information (i.e., a road sign displaying directions to the target destination) after such a critical event, indicating increased mental effort. Further, younger (but not older) participants demonstrated decreased alpha power when they had to detect task-relevant information on a road sign if this was preceded by a critical event, indicating increased attention. While EEG studies focusing on driving behaviour at intersections are rare, there is evidence that alpha and theta power also track important processes in this context. For example, a driving simulation study by Zhang and Yan (2023) demonstrated that intersection collisions are preceded by lower theta power and increased alpha power, indicating a lack of attention. Further, a real world driving study found that preparing for a turn at an intersection was shown to be associated with an increase in alpha power variation (Kim et al., 2014). In summary, theta and alpha power are useful indicators of cognitive processing that allow further insights into the mechanisms underlying driving behaviours, including those required at intersections.
Systematically investigating how different intersection properties affect cognitive processing and driving performance requires a controlled, yet realistic driving environment. This trade-off between ecological validity on the one hand and experimental control on the other hand poses a key challenge in driving research. In naturalistic driving studies (i.e., driving an actual car), it is almost impossible to control all factors that might influence the behaviour of interest and it can be impossible or unethical to implement certain manipulations (e.g., having a pedestrian run across the road or having a car crash in front of the driver). These issues can be addressed in driving simulator studies, where participants drive a simulated car through a computer-generated virtual environment. However, driving simulators have certain limitations. They typically operate through various large screens in front of the driver, which has the disadvantage of imposing a fixed field of view onto the driver and restricting potential driving behaviours (e.g., it is typically not possible to drive backwards). These drawbacks can be addressed by fully immersive virtual reality (VR) that allows the driver free 360° movement by combining a simulated car with a VR headset. In addition, freely available video game engines such as Unity (Unity Technologies, 2024) and Unreal (EpicGames, 2024) offer researchers the possibility to construct a wide range of virtual environments and traffic scenarios, which not all commercial driving simulators provide. Recent research (Weber et al., 2021) also shows that, when recording EEG while wearing a virtual reality head-mounted displays (HMD), lower frequency brain oscillations such as theta and alpha are not affected by the electronic artefacts of HMDs such as the HTC Vive Pro (employed here), as these are typically limited to a higher frequency range.
The aim of this study was to develop a fully immersive VR driving scenario to assess driving behaviour at intersections. We manipulated three factors: 1) right of way, 2) presence of traffic, and 3) the presence of a pedestrian crossing the road. We recorded EEG to gain further insights into how these factors influence cognitive and attentional processes underlying driving behaviours. We expected that theta power should be increased and alpha power should be decreased 1) when drivers have to yield to traffic, 2) when traffic volume is high, and 3) when drivers have to react to an unexpected event.
Methods
Participants
We recruited 34 participants between the ages of 18 and 28 years through posters put up on the campus of Aston University (Birmingham, UK), through the Psychology participant data base of Aston University, and through word-of-mouth. Participants had to meet the following criteria: They had to possess a valid UK driving license and had to have driven in the UK within the last year; they must not suffer from motion sickness; never have had a diagnosis of a neurological disorder, i.e., not take psychotropic medication; had normal or corrected-to-normal vision and colour vision (for corrected vision: only small-framed glasses that fit in the virtual reality headset were allowed), and finally, excellent understanding of verbal and written English was required. Of the 34 participants who volunteered for our study (25 female, mean age = 20.56 years, SD = 2.27 years), 14 dropped out during the study due to cybersickness; the final sample consisted of 20 participants (12 female, mean age = 20.70 years, SD = 2.45 years). Participants received either class credit (in the case of undergraduate Aston University Psychology students) or reimbursement in the form of vouchers (£7.50/hour) for their participation. The study was approved by Aston University’s University Research Ethics Committee (REC ref: 1782).
Initially, we also aimed to compare different age groups, as driving performance and cognitive control processes have been shown to differ between younger and older drivers (Bennett et al., 2016; Getzmann et al., 2018; Huizeling et al., 2020). We therefore attempted to recruit an older participant group (65+ years), however, due to recruitment difficulties during the COVID-19 pandemic and high rates of cybersickness (8 dropouts out of 9 recruited participants) we stopped the recruitment process (see Supplementary Material for details).
Study procedure
The study consisted of one session lasting roughly three hours. First, participants gave informed consent and filled out a demographic questionnaire. Then, they received written instructions on the driving task and performed a practice version of the virtual driving task. In the practice version, there was no traffic and the pedestrian was always idle, so that participants could focus on familiarising themselves with driving in the virtual environment. They were encouraged to brake and accelerate several times and to practice driving at the speed limit of 30 mph. The practice block lasted for sixteen trials (intersections) but could be extended if participants stated that they needed more time. If participants felt comfortable with the virtual driving task, they then performed four trials (intersections) of the real task, where they experienced traffic and the pedestrian running across the road. After this practice, participants completed the Driving Habit Questionnaire (assesses driving habits and experience within the last year; Owsley et al., 1999), the Useful Field of View test (measures divided attention, selective attention and visual processing; Ball et al., 1988), and the Choice Reaction Time test (measures visual processing speed).
In the second part, participants completed the virtual driving task while wearing an EEG cap. The task was organised in five blocks of sixteen trials (intersections) each. In the task, participants had to follow road signs towards a target destination. Participants took breaks between each block during which they had the opportunity to take off the HMD. The driving task took approximately 40 min on average. After the driving task, participants had the opportunity to wash the EEG gel out of their hair. They completed two virtual reality questionnaires after the experiment: The Virtual Reality Sickness Questionnaire (Kim et al., 2018) which measures cybersickness symptoms and is a reduced version of the Simulator Sickness Questionnaire (Kennedy et al., 1993) with high internal consistency (Cronbach’s alpha of .92; Sevinc and Berkman, 2020), and the Igroup Presence Questionnaire (IPQ; Igroup project consortium, 2024; Schubert et al., 2001) which measures how present participants felt in the virtual environment. The IPQ consists of the subscales spatial presence (Cronbach’s alpha of .82), involvement (Cronbach’s alpha of .87), and experienced realism (Cronbach’s alpha of .69), as well as one item to measure general presence (Regenbrecht and Schubert, 2002). At the end, participants were debriefed about the aims of the study.
Driving simulation task
Participants completed a driving simulator task in virtual reality where they navigated a series of intersections to drive towards a target destination.
Materials and apparatus
As depicted in Figure 1, participants were seated in an adjustable GT Ω Art Racing Simulator Cockpit (RS6 seat). They used a Logitech G27 steering wheel and pedal set to control the car in virtual reality. The steering wheel included paddles to the left and right of the steering wheel that served as indicators. Gear shifts were automatic, so participants only used the accelerator and brake pedals and did not manually shift gears. The virtual driving scenario was presented using the HTC Vive Pro head-mounted display (HMD). The HTC Vive Pro has a dual OLED 3.5 inch display with a resolution of 1,440 × 1,600 pixels per eye, a field of view of 110° and a refresh rate of 90 Hz. The interpupillary distance between the two lenses is adjustable and was adapted to each participant. The HTC Vive Pro also includes headphones.
The virtual driving scenario was created in Unity version 2019.4.21f1 and was controlled via SteamVR version 1.20.4 on a Windows 10 personal computer (i9 processor, 32 GB RAM, Nvidia GeForce RTX 2090 TI GDDR6). The Unity Experimental Framework (Brookes et al., 2020) was used to record behavioural data (including the position and rotation of the driver car, velocity, speed, accelerator input, brake input, indicator input, steering input, and lane position). Lab Streaming Layer (LSL) was used to send EEG triggers from Unity to the EEG recording system over a mobile hotspot.
For the virtual environment, all roads were created in Blender version 2.91 (Blender Development Team, 2024). Roads at intersections contained typical UK road markings to indicate right of way (white double broken lines and triangle sign for having to give way). All static objects in the environment were either free assets from the Unity asset store or were created in Unity (this includes all houses, nature elements and road signs; for a list of assets, see Supplementary Table S1). For the pedestrian, we used a free 3D model of the character “Sophie” including idle and running animations from http://mixamo.com (Adobe Systems). All cars were based on a realistic car model obtained from the Unity asset store (https://assetstore.unity.com/packages/3d/vehicles/land/realistic-car-hd-03-113200) and controlled by the Realistic Car Controller V3.4 asset (Buğra Özdoğanlar, 2024). The inside of the driver’s car included fully functional mirrors. Two virtual hands were placed at the 10 and 2 (o’clock) positions on the steering wheel. The dashboard contained a non-functional RPM dial and a functional digital speedometer in miles per hour (MPH). The driver’s car also produced audio output consistent with the car behaviour (e.g., an “engine start” sound when starting the engine, engine sounds that changed with the RPM while driving, indicator sounds when one of the indicators was activated). Road signs were created by editing 3D models in Blender and adding text using TextMeshPro in Unity. Road signs were brown with white text. Each road sign listed nine destinations, with the top three destinations pointing straight ahead, the middle three destinations pointing right, and the bottom three destinations pointing left. The road sign had a size of 5.35 × 3.80 m. A screenshot of the virtual driving environment is presented in Figure 2 (for a video, see https://osf.io/fqtve/).
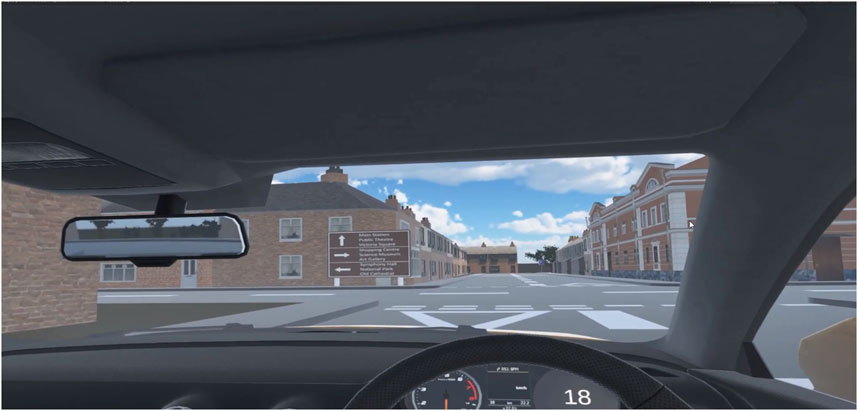
Figure 2. Screenshot of the virtual driving scenario. The driver is approaching a give way intersection without any traffic.
Intersection design
The virtual driving scenario consisted of a series of intersections. A schematic illustration of the intersection design can be found in Figure 3. Each intersection started with a straight road on which the participant’s car was moving. After 48 m, the road took a 90° corner (left or right). At the outer apex of the corner, there was a car park. 16 m after the corner, there was a four-way intersection. Road markings either indicated that participants had right of way or that they had to give way; there were no traffic lights. A road sign on the left side of the road displayed nine destinations. The order of the destinations was randomized at each intersection. 12 m before the intersection, a pedestrian stood either on the left or the right side of the road. The pedestrian was initially in idle mode; in half of the intersections, the pedestrian then ran across the road (added distractor event). The onset of the pedestrian’s movements was time-locked to 1s after participants crossed an invisible line before the turn and the speed of movement was varied randomly. At the intersection, there was traffic in half of the intersections (added cognitive load). Traffic consisted of five unique cars controlled by a simple artificial intelligence (AI); these AI cars could either be driving from left to right, right to left, or from straight ahead. The AI cars’ speed was adjusted based on the participants’ driving speed up until shortly before the intersection; this ensured that regardless of how fast participants were driving, there would be traffic when they arrived at the intersection. AI cars pulled into the car parks before the next intersection as to not further influence participants’ driving behaviour.
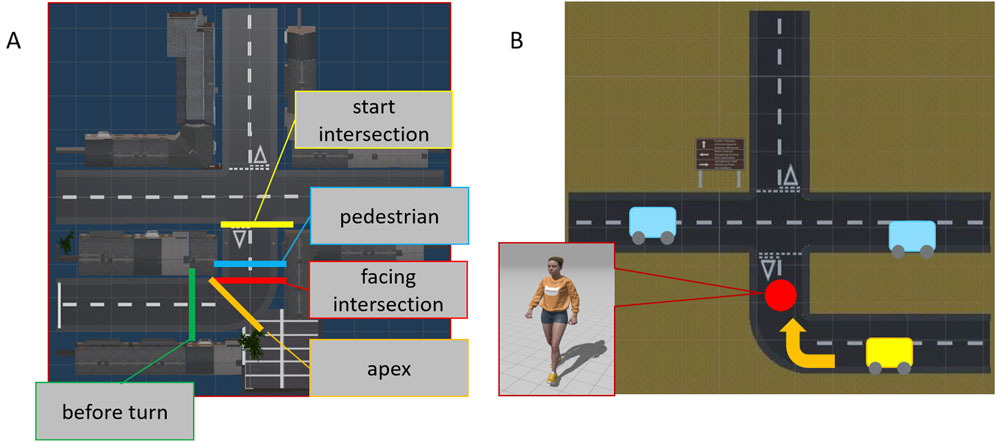
Figure 3. Intersection design and experimental manipulations. (A) Schematic of markers used for EEG epoching and behavioural analysis segments. The before turn marker was placed 8.6 m before the turn (green), the apex marker was placed at the apex of the turn (orange), the facing intersection marker was placed 17 m before the intersection (red), the pedestrian was placed 12 m before the intersection (blue), the start intersection marker was directly at the beginning of the intersection (yellow). Note that the pedestrian marker used for time-locking was the movement onset of the pedestrian, not the time when participants crossed the pedestrian’s position. (B) The experimental design was as follows: After a turn around a corner (left or right turn, balanced), a pedestrian stood on the pavement to the left or right (random). In half of trials, she started running across the road so that drivers had to brake and stop. At the intersection, there was a road sign with 9 destinations. We systematically varied the right of way (vs. give way) and the presence of traffic (vs. no traffic).
We systematically varied three factors in a full factorial within-subjects design (see Figure 3B): the presence of a distracting event (idle pedestrian vs. running pedestrian), the road type (right of way vs. give way), and the presence of traffic (no traffic vs. traffic). We also balanced the initial left vs. right turn before the intersection. This resulted in 2 × 2 × 2 × 2 = 16 unique intersections. We randomized the order of these intersections in each block. For each intersection, the position of the pedestrian (left vs. right side of the road), the position of the target destination on the road sign, and the number of AI cars in the traffic condition (4-5) was assigned randomly.
EEG data acquisition
We recorded scalp EEG from 64 Ag/AgCl electrodes using a 64 channel eego sports mobile EEG system (ANT Neuro, Enschede, Netherlands). The electrode layout followed the international 10–20 system. EEG data was recorded with a sample rate of 500 Hz. Electrode AFz was used as ground and electrode CPz was used as reference. Impedances were kept below 20 kOhm. We did not record data from mastoids or eye electrodes.
EEG preprocessing
EEG data were preprocessed using Matlab R2021b and eeglab 2024.2 (Delorme and Makeig, 2004). Preprocessing consisted of the following steps. We bandpass-filtered the data between 1 and 50 Hz (Hamming windowed FIR filter). A recent study by Weber et al. (2021) investigated EEG contamination by HMD artefacts (incl. HTC Vive Pro) and concluded that frequencies below 50 Hz remained largely unaffected (see also Alexander, 2024 for confirmation). We then extracted three different epoch types. As baseline, we extracted 12 s epochs (−10 to 2 s) time-locked to before participants reached the turn (see Figure 3A). For analyses of right of way and traffic, we extracted 20 s epochs (−10 s–10 s) time-locked to when participants first faced the intersection after the turn (see Figure 3A). In addition, we re-epoched the data to extract 8 s epochs (−2–6 s) time-locked to the onset of pedestrian movement for analysis of the pedestrian. In trials where the pedestrian did not move, we used an estimate based on the onset times of all other trials for that participant. For all epochs, we inspected the data visually to identify bad channels and remove trials that were affected by severe artefacts (baseline: M = 1.45 trials removed, SD = 3.68 trials; facing intersection and pedestrian: M = 1.35 trials removed, SD = 3.69 trials). We excluded bad channels before re-referencing to infinity using reference electrode standardization technique (REST; Yao, 2001) as implemented in Fieldtrip version 20,210,330 (Oostenveld et al., 2011). Next, we performed Infomax independent component analysis (ICA) to identify artefact components (as based on Bell and Sejnowski, 1995 as implemented in EEGLAB). We used ICLabel (Pion-Tonachini et al., 2019) to mark artifact components such as eye and muscle movements, which we then visually inspected before removing clear artifacts (average number of removed components: baseline: M = 6.85, SD = 2.03; facing intersection and pedestrian: M = 7.10, SD = 1.86). Finally, we interpolated bad channels using spherical spline interpolation as implemented in EEGLAB (Perrin et al., 1989). We then resampled to 250 Hz.
EEG time-frequency analysis
We analysed data using Matlab 2021b and Fieldtrip version 20210330 (Oostenveld et al., 2011). We carried out time-frequency analyses using complex Morlet wavelets for frequencies from 1 to 50 Hz in 50 logarithmically spaced steps. The cycle length of the complex Morlet wavelets increased from 3 cycles at 1 Hz to 8 cycles at 50 Hz in logarithmically spaced steps. As baseline, we averaged the time-frequency signal during four seconds of driving straight ahead (−5 to −1 s before the before turn trigger) across time and trials. We applied a decibel (dB) baseline normalisation where normalised power is dB = 10 * log10 (power spectrum/baseline) for each trial. We then averaged across trials per condition and across frequencies (theta: 4–7 Hz; alpha: 8–12 Hz) and ran cluster-based permutation tests (Maris and Oostenveld, 2007) using two-tailed dependent sample t-tests. We set the minimum number of neighbouring channels to 2, alpha level was 0.05, and the number of permutations was 10,000. We tested three comparisons of interest separately for theta and for alpha power: traffic vs. no traffic (time-locked to facing intersection, time window of interest: −0.5–2 s), give way vs. right of way (time-locked to facing intersection, time window of interest: −0.5–2 s), and pedestrian running vs. idle (time-locked to onset of pedestrian movement, time window of interest: −0.5–2 s).
Data statement
We report how we determined our sample size, all data exclusions (if any), all manipulations, and all measures in the study. We did not preregister this study. All data and analysis scripts are openly available from https://osf.io/v7yx4/. Due to licensing constraints, we can only share the Unity project with people who have obtained the necessary licenses, as some of the Unity assets used in our experiment are not openly available (see Supplementary Table S1 for a list of required Unity assets). Please contact the corresponding author for further details.
Results
Behavioural results
First, we looked at participants’ cybersickness and presence scores to evaluate how the experienced the virtual environment. Next, we checked if participants took the task seriously by analysing navigational errors, crashes, indicator activation and average driving speed. Then, we analysed participants’ speed, braking and acceleration patterns at the intersection as well as their reaction times in activating the indicators at the intersection (in case of a left or right turn).
The impact of the virtual environment
Participants had a general presence score of 3.85 (SD = 1.35) using the IPQ, indicating satisfactory presence (for results of the subscales, see Supplementary Material). Cybersickness symptoms were low to moderate, with an average VRSQ score of 19.42 out of 100 (SD = 14.39) for the 20 participants who completed the experiment. As the dropout rate was relatively high at 41%, we also looked at participants who did not complete the experiment. Notably, these participants reported descriptively higher cybersickness scores (M = 26.96, SD = 13.46). In addition, only 21% of participants who dropped out had prior experience with VR (compared to 65% of participants who completed the experiment). Finally, we ran exploratory correlational analyses between cybersickness and presence scores across all participants and found a significant negative correlation between cybersickness and spatial presence, rho = −.437, p = .01 (see Supplementary Material for details).
Basic check: realistic driving behaviour
To test if participants took the task seriously and drove in a realistic manner, we analysed their driving behaviour. Participants made very few navigational errors; they drove in the wrong direction at 0.6 out of 80 intersections on average (SD = 0.88). We found some variability when analysing crashes (collisions with other cars or the pedestrian); participants had an average number of 1.75 crashes (SD = 2.20), with 1.15 crashes (SD = 1.50) involving other cars and 0.60 crashes (SD = 1.23) involving the pedestrian on average; eight participants did not crash at all. This is comparable to traditional driving simulator studies (Werneke and Vollrath, 2012) and fits with the finding that participants make more errors in simulated compared to controlled driving (Bach et al., 2008). Most participants used their indicators correctly, with only three participants failing to indicate (two participants failed to indicate at nine intersections, one participant failed to indicate at three intersections). The average driving speed while driving on a straight road was 25.02 mph (SD = 3.51 mph). Overall, this indicates that participants obeyed traffic rules and drove in a realistic manner.
Dynamics of driving behaviours at intersections
Next, we focused on speed, acceleration and braking patterns during the different periods of the driving task. We divided each intersection into five segments (see Table 1; Figure 3A).
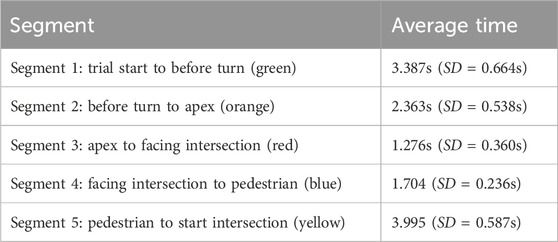
Table 1. Overview over driving behaviour segments. Colours in brackets refer to event markers in Figure 3.
We compared participants’ average driving speed, brake input and throttle input during each segment for each of our conditions (traffic vs. no traffic, pedestrian running vs. idle, give way vs. right of way) using two-tailed paired t-tests. The results are summarised in Figure 4 and descriptive statistics are presented in Supplementary Material (Supplementary Tables S1–S3). Participants reduced their driving speed well in advance when approaching an intersection where they had to give way (as compared to the right of way). Participants accelerated more when approaching an intersection without any traffic (as compared to high traffic volume), but their driving speed only differed in the last time segment directly before the start of the intersection. Participants braked for the pedestrian as expected, but then accelerated more in later time segments.
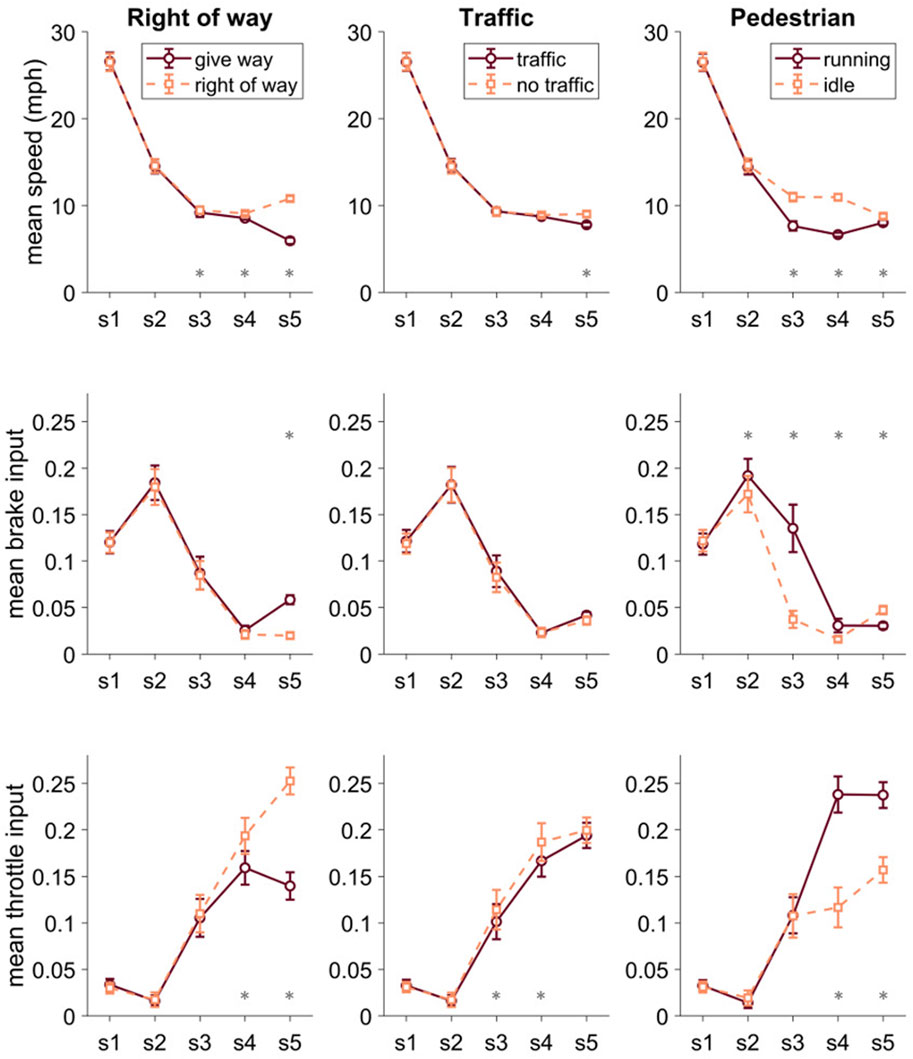
Figure 4. Average driving speed (top row), brake input (middle row) and throttle input (bottom row) for each of the comparisons of interest and all five time segments (s1 to s5, see Table 1). Significant differences are marked with a grey asterisk. Error bars represent standard errors.
Next, we looked at how fast participants were in activating their indicator in case they had to turn. We took this as a proxy for how quickly participants identified the target destination when approaching the intersection. We ran two-tailed paired t-tests on the time between passing the pedestrian (see Figure 3A, blue marker) until the activation of the indicator for each comparison of interest (see Figure 5; Supplementary Table S6). Indicator activation times were significantly faster in the pedestrian running as compared to idle condition, t(19) = −2.55, p = .020, dz = −0.57. When considering the acceleration patterns described above, this could indicate that participants took the time that they waited for the pedestrian to pass to prepare for the upcoming intersection, thus making them quicker at identifying the target destination and activating their indicator. Indicator activation times did not differ between traffic and no traffic conditions, t(19) = −0.69, p = 0.500, dz = −0.15, but were significantly faster in the right of way as compared to give way condition, t(19) = −2.41, p = .026, dz = −0.54. As the speed and acceleration patterns showed that participants slowed down before the intersection in the give way condition, this is likely due to participants being more cautious in the give way condition and hence taking longer to identify the target destination.
EEG results
We tested theta and alpha power for all comparisons of interest using cluster-based permutation tests (see Methods). Both the comparison of right of way vs. give way and traffic vs. no traffic were time-locked to when participants first faced the intersection (see Figure 3A, red event marker) to test for differences in cognitive processing while participants approached the intersection. For the comparison of give way vs. right of way, we did not find significant clusters for theta, all ps > .99, or alpha power, all ps > .59 (see Supplementary Figures S1, S2 for topographies). For the comparison of traffic vs. no traffic, there was a significant difference for theta with increased theta power in the traffic condition. Descriptively, we found two clusters: a posterior cluster (appr. 480–800 ms, p = .009) and a midfrontal cluster (appr. 760–1,040 ms, p = .03) (see Figure 6; Supplementary Figure S3). We did not find a significant difference in alpha power, all ps > .99 (see Supplementary Figure S4 for topographies).
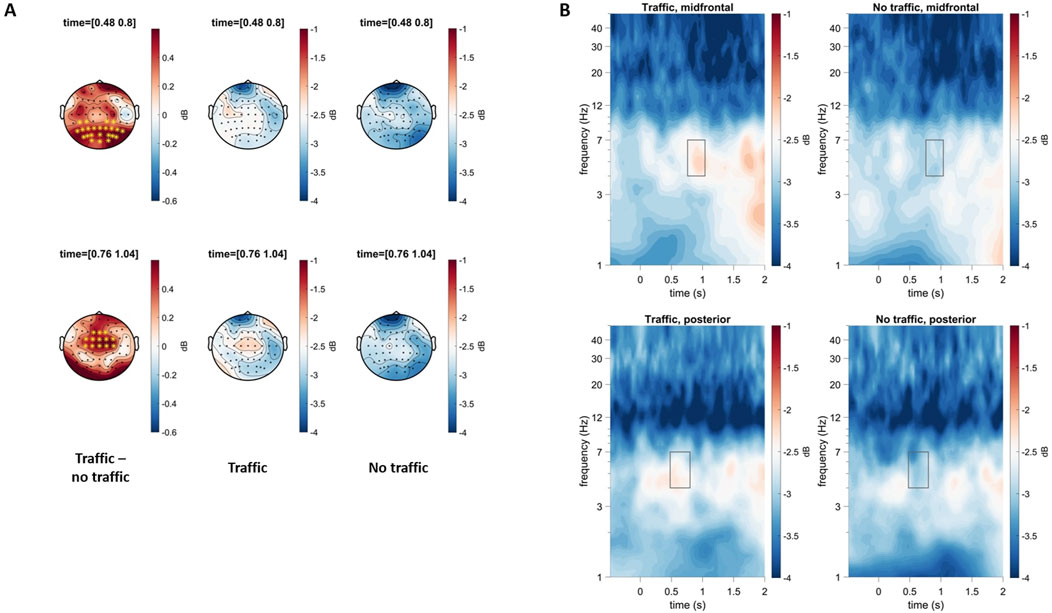
Figure 6. Time-frequency results for traffic vs. no traffic. (A) Topographic plots of baseline-corrected theta power for each significant cluster. Left topographies show the differences between both conditions, centre and right topographies show traffic and no traffic respectively. Significant clusters are marked with yellow asterisks. (B) Time-frequency-plots of baseline-corrected power for midfrontal electrodes and posterior electrodes. All results are time-locked to the moment of facing the intersection. The time-frequency windows of the significant theta clusters are represented by black rectangles. No significant alpha clusters were observed. Further explanation in the text.
For testing the effect of the pedestrian, we time-locked the data to the moment the pedestrian started moving. Here, we found significant differences for both theta and alpha power (see Figure 7). For theta power, we found an early cluster with increased theta power for when the pedestrian was running that included all electrodes, but was especially prominent across mid-frontal electrodes (appr. 80–600 ms, p < .001). We also found a later cluster with decreased theta power (appr. 680–2000 ms, p < .001) that included various electrodes distributed across the scalp (but the topography varied substantially across the time window, see Supplementary Figure S5 for a detailed time course). We found the same pattern for alpha power, with a significant early cluster of increased alpha power (appr. 160–400 ms, p < .001), and two significant later clusters of decreased alpha power (1,120–1,640 ms, p < .001 and 1,640–2000 ms, p = .003) (see Supplementary Figure S6 for a detailed time course).
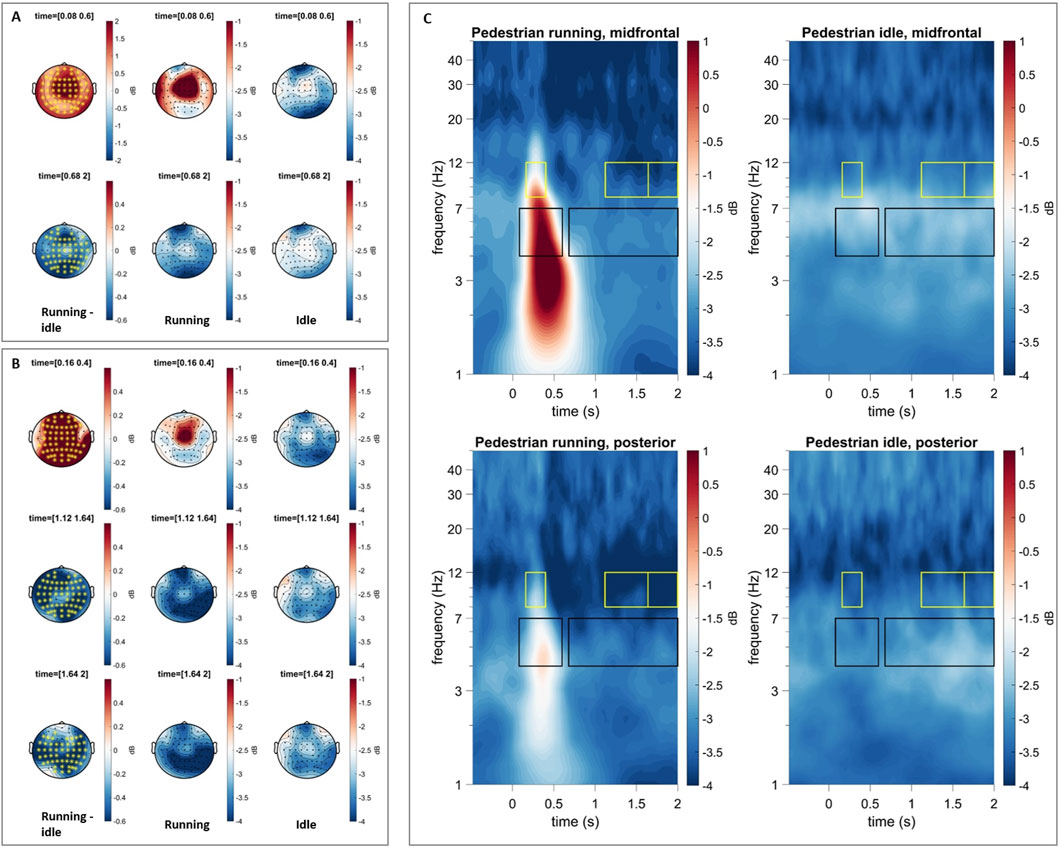
Figure 7. Time-frequency results for pedestrian running vs. idle. (A) shows topographic plots of significant theta power clusters, (B) shows topographic plots of significant alpha power clusters (all baseline-corrected). Please note the different scales of the colour bars for the difference maps (chosen to best illustrate the respective effects). Left topographies show the difference between both conditions, centre and right topographies show the running and idle condition respectively. Significant clusters are marked with yellow asterisks. (C) shows time-frequency-plots of baseline-corrected power for midfrontal electrodes and posterior electrodes. Black and yellow rectangles represent time-frequency windows for significant theta and alpha clusters, respectively. All results are time-locked to the onset of movement of the pedestrian.
One potential confound in our paradigm are eye movements, as some conditions are more likely to contain eye movements than others (e.g., the presence of moving stimuli such as other cars in the traffic condition or the running pedestrian). We ran additional time-frequency analyses on ocular ICA components in order to gain a better understanding of the influence of eye movements on our effects (see Supplementary Material for details). The results showed clearly distinct temporal-spatial patterns for the traffic vs. no traffic comparison between ocular and cleaned data (see Supplementary Figures S7, S8), whereas we found temporally similar patterns for the pedestrian running vs. idle comparison (see Figure 8, Supplementary Figures S8, S9). However, the topographies were very different to the clearly midfrontal peak that we observed in the cleaned data. It is important to emphasise that the ocular ICA components shown here and in Supplementary Material were removed from the data, thus, only residual ocular artefacts might have affected the cleaned data forming the basis of our analysis and results. Therefore, while we cannot fully exclude the possibility of ocular artefacts in our results, we believe that the differences in temporal and spatial patterns between the ocular data and the cleaned data indicate a neural source for the effects we observed.
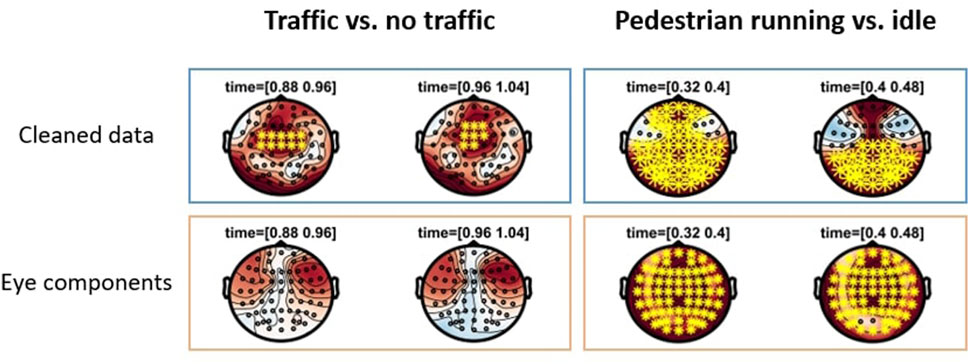
Figure 8. Topographies of dB-corrected theta power for cleaned data and eye component data. Detailed results and topographies can be found in the Supplement.
Finally, we wanted to explore if the observed brain signatures in the theta and alpha range (average power) during the baseline driving period (4s, see EEG preprocessing) correlate with cybersickness and/or presence scores. We only observed a significant positive correlation between average midfrontal theta power and cybersickness severity, rho = .341, p = .036 (for further details, see Supplementary Material; Supplementary Figure S11).
Discussion
In this study, we developed a fully immersive driving environment in VR to assess driving performance at intersections. We manipulated three experimental factors: 1) right of way, 2) amount of traffic, and 3) the presence of a pedestrian crossing the road. We also recorded EEG to analyse how these factors influence theta power (as an indicator of cognitive control) and alpha power (as an indicator of attention). We found expected behavioural patterns such as a slower approach of the intersection when traffic volume was high and when participants had to give way. We also found increased midfrontal theta power, a neural maker of cognitive control, when participants approach intersections with high traffic volume. Surprisingly, we found no such effect for giving way vs. right of way. When a critical event occurred (in the form of a pedestrian crossing the street directly before the intersection), participants also showed a strong increase in theta power. Taken together, our study offers new insights into the cognitive and attentional processes underlying driving behaviour at intersections. We will now discuss the implications of our results in more detail.
The impact of intersection factors on neuro-cognitive processing
Right of way
Participants slowed down early on when approaching an intersection where they had to give way. They were also slower to activate their indicator. This might reflect longer processing time due to additional demands, as it has been shown that more attention is allocated towards traffic coming from left and right when having to give way (Werneke and Vollrath, 2012; Ringhand et al., 2022). However, this might also reflect that participants simply waited for other cars to pass before indicating (i.e., passive waiting). Interestingly, we did not find any differences at the neural level. Neither alpha nor theta power showed significant differences between having the right of way and giving way. This is surprising in the light of the behavioural effects and in light of differences in attention reported in the literature (Werneke and Vollrath, 2012; 2014; Ringhand et al., 2022). It is possible that our data are either too noisy or too variable or both to find differences for this manipulation, particularly as there might be significant variability in when drivers realise that they have to give way or have the right of way. This could affect the timepoint of when exactly attentional control processes are increased when having to give way, and hence make it difficult to detect this effect. In addition, as the impact of right of way might be smaller than the impact of traffic volume and the occurrence of a critical event, it is possible that our sample size was too small to detect this effect (see Limitations).
Presence of traffic
When approaching an intersection with high traffic volume, participants slowed down relatively shortly before reaching the intersection. This fits well with other research showing that participants slow down and allocate their attention towards traffic when they are close to an intersection (Werneke and Vollrath, 2014; Ringhand et al., 2022). In addition, we found that theta power started to increase very quickly after participants were able to detect traffic. Descriptively, there seemed to be an early increase in posterior theta power directly after turning around the corner and facing the intersection. This could indicate increased visual attention when detecting cars moving left and right at the intersection (Huizeling et al., 2020; Asanowicz et al., 2023). Approximately 1s after facing the intersection, there seemed to be an increase in midfrontal theta power. Midfrontal theta power is associated with increased cognitive effort (Cohen et al., 2008; Nigbur et al., 2011; Cavanagh and Frank, 2014). In the context of driving, midfrontal theta has been shown to increase under complex driving conditions (Di Flumeri et al., 2018; Wascher et al., 2018; Diaz-Piedra et al., 2020; Huizeling et al., 2020; Depestele et al., 2023). Therefore, we interpret this increase in midfrontal theta power as a proactive engagement of cognitive control when participants detect that there is traffic at the intersection, as high traffic volume typically requires additional monitoring, preparation for driving actions (such as stopping) and complex decision making (e.g., predicting another driver’s intentions and driving behaviour and reacting accordingly). Our results also suggest that this proactive engagement of cognitive control happens relatively early on, whereas behavioural differences such as slowing down only emerge when participants are closer to the intersection. Therefore, it would be interesting to combine EEG and eye-tracking to examine the timeline of neural, attention and behavioural effects of traffic volume.
Critical event
Finally, we examined participants’ reactions to a critical event occurring directly before the intersection in form of a pedestrian crossing the road. We found a substantial increase in theta power across the whole scalp shortly after the movement onset of the pedestrian. This increase was maximal for midfrontal theta power. We interpret this effect as participants processing the critical event, increasing their attention and quickly carrying out a motor response (i.e., braking), which are processes that demand increased attention and cognitive control. Interestingly, there was also a decrease in theta power later on. This might indicate that participants disengaged their attentional focus while they waited for the pedestrian to pass, whereas in the idle condition participants continued driving and were already preparing for the intersection. In addition, the topography of this effect was not as focal as for the early midfrontal cluster, which might reflect a larger degree of variability. Unexpectedly, we observed similar patterns for alpha power. This is surprising because alpha power typically decreases when theta power increases (Klimesch et al., 1998; Klimesch, 1999). The time-frequency plots show that this might be due to a broadband peak across frequency bands when the pedestrian starts running (see Figure 7, Panel C, top left). However, exploratory analyses across all frequencies in the time window between 120 and 540 ms suggest that the peak is in mid-frontal theta power and might be leaking into higher frequency bands. This makes it difficult to interpret alpha in this time window in a meaningful way. On the one hand, the differences in alpha power might be simply due to short-comings of the time-frequency analysis. On the other hand, it might reflect a true cognitive process, as a previous study also found increased alpha power directly before a critical event in safe drivers (Zhang and Yan, 2023). Increased alpha power has often been associated with inhibition of task-irrelevant information (e.g., Klimesch et al., 2007; Sauseng et al., 2009; Jensen and Mazaheri, 2010). Given the complexity of driving - the current scenario included - alpha-based inhibition could indicate a temporary suppression of information and actions that are unrelated to a demanding event – in our case, braking and avoiding collision with the pedestrian. This interesting line of enquiry that should be addressed in future research.
Fully immersive driving simulations in VR and Neuro-VR: potential and challenges
In our novel driving simulation, participants demonstrated expected driving behaviours, such as slowing down when approaching an intersection with high traffic volume or when having to give way, being faster to activate their indicators when having the right of way, and braking for a pedestrian. Our study highlights several key advantages of using VR environments for driving research.
First, freely available VR game engines such as Unity and Unreal are very powerful and offer a large degree of freedom and flexibility to researchers. This makes it possible to create virtually any driving environment that might be of interest. For example, in the present study, we designed the environment in a modular way, such that the next intersection was only activated once drivers had crossed the current intersection. This made sure that participants always experienced the intended intersection types, regardless if they drove in the correct direction. In addition, the built-in features of VR gaming engines can easily track behavioural measures of driving performance, such as velocity, lane position or pedal inputs. VR game engines are highly compatible with other hardware devices, making it possible to record psychophysiological data such as EEG or eye-tracking (such as recommended by Menck et al., 2023). In combination with freely available VR headsets and steering wheels, this allows for a relatively inexpensive yet powerful driving simulator setup.
Second, VR driving scenarios offer a full range of motion and field of view, which makes them more immersive than traditional driving simulators. This also makes it possible for participants to turn around, which enables researchers to investigate driving situations involving turns or parking manoeuvres.
However, this also creates potential challenges for driving research. The main pitfall lies in the increased risk of cybersickness in VR driving scenarios. In our study, 41% of participants had to drop out due to cybersickness. While comparable dropout rates due to simulator sickness have been reported in traditional driving simulator studies (e.g., 35% in Shechtman et al., 2007; 45% in Werneke and Vollrath, 2012), symptoms have been shown to be more severe in studies comparing HMD and screen-based driving simulations (Weidner et al., 2017; Suwarno et al., 2019). This is important to consider, particularly for research on individuals who might have a higher risk for cybersickness, such people with motion sickness or older adults (Tian et al., 2022). In our study, we had to abort recruitment of an older driver group due to unacceptably high cybersickness rates; however, other studies successfully used VR driving simulations with older adults (e.g., Bennett et al., 2016). This highlights that VR driving environments differ in how well they are tolerated by participants. In addition, our study demonstrates that design factors within the virtual environment that induce a higher degree of visuo-vestibular discrepancies can increase cybersickness symptoms (Kemeny et al., 2017; Kemeny et al., 2020). This poses a challenge for research on intersections or on roads that involve sharp bends and turns.
Finally, when combining VR scenarios with neuroimaging techniques such as EEG (an approach we call Neuro-VR), it is important to consider the impact that the virtual environment and the VR setup have on the signal. On the one hand, the unique trade-off of experimental control and realism that VR offers makes it possible to capture EEG signals of more naturalistic processing. On the other hand, both the increased realism (including free range of movement) as well as the VR hardware itself can affect EEG data quality. In addition, the VR environment itself can affect the EEG signal, as indicated by a positive correlation between midfrontal theta power and cybersickness scores in an exploratory analysis. At the same time, our results show that, despite noisy conditions (body, head and eye movements, no Faraday cage), we were able to extract brain signatures that reflect the complexity of brain processing when driving towards an intersection. Hence, our study demonstrates that VR can be fruitfully combined with brain recordings such as EEG to understand complex brain processes in realistic scenarios (Sharma et al., 2017; Roberts et al., 2019; Gregory et al., 2022; Lenormand and Piolino, 2022; Peng et al., 2024). However, there are several limitations to consider, which we will discuss in the next section.
Limitations
One limitation of our study is the high rate of cybersickness-related dropouts. This raises the question if our results are confounded by cybersickness. However, participants’ cybersickness scores (assessed using the VRSQ) suggest that participants who were able to complete the experiment only experienced mild symptoms of cybersickness. At the same time, increased cybersickness was significantly correlated with decreased spatial presence. Notably, the majority of participants who completed the experiment had prior experience with VR, which was not the case for participants who dropped out. This is in line with research showing that past VR or video game experience is associated with a lower risk of cybersickness (Tian et al., 2022). This points toward a potential self-selection mechanism, as people who use VR might be more interested in participating in VR studies and are then more likely to not drop out. Hence, future work should control for past VR and gaming experience and consider how to mitigate cybersickness in a VR driving simulation. Another reason might lie in the driving simulator setup. In a VR driving simulation, latencies between driving actions (e.g., steering) and the corresponding behaviour of the VR environment (e.g., the car steering to the left) can increase cybersickness, as this increases visuo-vestibular conflict (Kemeny, 2014). While performance in our VR experiment appeared stable (i.e., the framerate did not drop below the refresh rate of 90 Hz), it is possible that irregular drops in performance might have occurred or that the coupling of the steering wheel and pedals to the virtual car model and physics was not optimal. In addition, reducing stimulus complexity (Davis et al., 2015), reducing the field of view (Kemeny et al., 2020) and including more breaks as well as additional practice sessions (Domeyer et al., 2013) could also mitigate cybersickness.
A second limitation is that our sample size is relatively low, which limits our statistical power. While EEG studies in the field of driving research often have comparably small sample sizes (e.g., Haufe et al., 2014; Di Flumeri et al., 2018; Wascher et al., 2023; Zhang and Yan, 2023), this makes it difficult to detect effects with smaller effect sizes. Hence, null effects like the give way manipulation in our study should be investigated again using a larger sample.
Another limitation that needs to be considered is EEG data quality. While movement of head, arms, legs and eyes is unavoidable in a driving task, this results in motion artifacts. This could be a particular issue for the critical event condition, where participants had to react to a pedestrian crossing the road. In addition, the HMD itself might introduce additional artifacts, as the straps of the HMD put pressure on some electrodes. We attempted to mitigate these issues by applying a thorough preprocessing pipeline and comparing cleaned and ocular data to assess potential issues. In addition, our findings of increased midfrontal theta power in the traffic volume and critical event conditions show that data quality is sufficiently high to detect such effects. However, it is still possible that some of our findings are confounded by eye movements. In the future, eye-tracking should be recorded simultaneously to allow for optimal separation of artifacts and signal.
Finally, we used LSL to send EEG event markers from Unity. While LSL is a powerful tool for synchronized recording of multiple data streams, it cannot take into account any latencies that occur within the acquisition devices (Kothe et al., 2024). This could introduce latencies or jitters to the EEG event markers. However, we argue that this is not a major issue for our findings, as time-frequency analyses, particularly in lower frequency bands, do not depend on millisecond precise timing to the extent that event-related potentials do. Still, to improve precision in EEG-VR studies, this should be investigated to identify if such a latency exists in a Unity-LSL setup and to characterize its duration and stability.
Conclusion
In this paper, we developed a new experiment to investigate driving behaviour at intersections in virtual reality. We manipulated three factors: right of way, traffic volume, and occurrence of a critical event in the form of a pedestrian crossing the road. Recording both behavioural and EEG data, our study shows that humans proactively engage cognitive control processes when approaching an intersection with high traffic volume and when reacting to a critical event. Our study also highlights both the potential and the challenges of VR-based driving simulations and neuro-VR investigations more generally.
Data availability statement
The datasets presented in this study can be found in online repositories. The names of the repository/repositories and accession number(s) can be found below: https://osf.io/v7yx4/.
Ethics statement
The studies involving humans were approved by the Research Ethics Committee, Aston University, Birmingham, UK (REC ref: 1782). The studies were conducted in accordance with the local legislation and institutional requirements. The participants provided their written informed consent to participate in this study.
Author contributions
US: Conceptualization, Data curation, Formal Analysis, Investigation, Project administration, Visualization, Writing–original draft, Writing–review and editing. KK: Conceptualization, Funding acquisition, Supervision, Writing–original draft, Writing–review and editing.
Funding
The author(s) declare that financial support was received for the research, authorship, and/or publication of this article. This research was supported by the Rees Jeffrey Road Fund and by the RAC Foundation. Publication was supported by the Open Access Fund of SLUB Dresden.
Acknowledgments
We thank Razan Elobeid and Rebekah Harnett for their support in data collection.
Conflict of interest
The authors declare that the research was conducted in the absence of any commercial or financial relationships that could be construed as a potential conflict of interest.
The author(s) declared that they were an editorial board member of Frontiers, at the time of submission. This had no impact on the peer review process and the final decision.
Publisher’s note
All claims expressed in this article are solely those of the authors and do not necessarily represent those of their affiliated organizations, or those of the publisher, the editors and the reviewers. Any product that may be evaluated in this article, or claim that may be made by its manufacturer, is not guaranteed or endorsed by the publisher.
Supplementary material
The Supplementary Material for this article can be found online at: https://www.frontiersin.org/articles/10.3389/frvir.2024.1433829/full#supplementary-material
References
Alexander, N. A., Kelly, C. A., Wang, H., Nash, R. A., Beebe, S., Brookes, M. J., et al. (2024). Oscillatory neural correlates of police firearms decision-making in virtual reality. eNeuro 11 (7), 1–13. doi:10.1523/ENEURO.0112-24.2024
Asanowicz, D., Panek, B., Kotlewska, I., and van der Lubbe, R. (2023). On the relevance of posterior and midfrontal theta activity for visuospatial attention. J. Cognitive Neurosci. 35 (12), 1972–2001. doi:10.1162/jocn_a_02060
Bach, K. M., Jæger, M. G., Skov, M. B., and Thomassen, N. G. (2008). Evaluating driver attention and driving behaviour: comparing controlled driving and simulated driving. Proc. 22nd Br. HCI Group Annu. Conf. People Comput. Cult. Creativity, Interact. BCS HCI 2008 1, 193–201. doi:10.14236/ewic/hci2008.19
Ball, K. K., Beard, B. L., Roenker, D. L., Miller, R. L., and Griggs, D. S. (1988). Age and visual search: expanding the useful field of view. J. Opt. Soc. Am. A 5 (12), 2210–2219. doi:10.1364/JOSAA.5.002210
Bell, A. J., and Sejnowski, T. J. (1995). An information-maximization approach to blind separation and blind deconvolution. Neural Comput. 7 (6), 1129–1159. doi:10.1162/neco.1995.7.6.1129
Bennett, C. R., Corey, R. R., Giudice, U, and Giudice, N. A. (2016). “Immersive virtual reality simulation as a tool for aging and driving research,” in Human aspects of IT for the aged population. Healthy and active aging. ITAP 2016. Lecture notes in computer science. Editors J. Zhou, and G. Salvendy (Springer), 377–385. doi:10.1007/978-3-319-39949-2_36
Blender Development Team (2024). Amsterdam: Blender. Available at: https://www.blender.org/.
Brookes, J., Warburton, M., Alghadier, M., Mon-Williams, M., and Mushtaq, F. (2020). Studying human behavior with virtual reality: the Unity Experiment Framework. Behav. Res. Methods 52, 455–463. doi:10.3758/s13428-019-01242-0
Buğra Özdoğanlar, E. (2024). Realistic car controller. BoneCracker Games. Available at: https://www.bonecrackergames.com/realistic-car-controller/.
Cavanagh, J. F., and Frank, M. J. (2014). Frontal theta as a mechanism for cognitive control. Trends Cognitive Sci. 18 (8), 414–421. doi:10.1016/j.tics.2014.04.012
Cavanagh, J. F., Zambrano-Vazquez, L., and Allen, J. J. B. (2012). Theta lingua franca: a common mid-frontal substrate for action monitoring processes. Psychophysiology 49 (2), 220–238. doi:10.1111/j.1469-8986.2011.01293.x
Cohen, M. X. (2016). Midfrontal theta tracks action monitoring over multiple interactive time scales. NeuroImage 141, 262–272. doi:10.1016/j.neuroimage.2016.07.054
Cohen, M. X., Ridderinkhof, K. R., Haupt, S., Elger, C. E., and Fell, J. (2008). Medial frontal cortex and response conflict: evidence from human intracranial EEG and medial frontal cortex lesion. Brain Res. 1238, 127–142. doi:10.1016/j.brainres.2008.07.114
Davis, S., Nesbitt, K., and Nalivaiko, E. (2015). Comparing the onset of cybersickness using the Oculus Rift and two virtual roller coasters, 27–30.
Delorme, A., and Makeig, S. (2004). EEGLAB: an open source toolbox for analysis of single-trial EEG dynamics including independent component analysis. J. Neurosci. Methods 134 (1), 9–21. doi:10.1016/j.jneumeth.2003.10.009
Depestele, S., van Dun, K., Verstraelen, S., Ross, V., Van Hoornweder, S., Brijs, K., et al. (2023). Age-related changes in midfrontal theta activity during steering control: a driving simulator study. Neurobiol. Aging 123, 145–153. doi:10.1016/j.neurobiolaging.2022.11.014
Diaz-Piedra, C., Sebastián, M. V., and Di Stasi, L. L. (2020). EEG theta power activity reflects workload among army combat drivers: an experimental study. Brain Sci. 10 (4), 199. doi:10.3390/brainsci10040199
Di Flumeri, G., Borghini, G., Aricò, P., Sciaraffa, N., Lanzi, P., Pozzi, S., et al. (2018). EEG-based mental workload neurometric to evaluate the impact of different traffic and road conditions in real driving settings. Front. Hum. Neurosci. 12 (December), 1–18. doi:10.3389/fnhum.2018.00509
Domeyer, J. E., Cassavaugh, N. D., and Backs, R. W. (2013). The use of adaptation to reduce simulator sickness in driving assessment and research. Accid. Analysis Prev. 53, 127–132. doi:10.1016/j.aap.2012.12.039
EpicGames (2024). Unreal engine. Available at: https://www.unrealengine.com/.
Ericson, J. M., Parr, S. A., Beck, M. R., and Wolshon, B. (2017). Compensating for failed attention while driving. Transp. Res. Part F Traffic Psychol. Behav. 45, 65–74. doi:10.1016/j.trf.2016.11.015
Getzmann, S., Arnau, S., Karthaus, M., Reiser, J. E., and Wascher, E. (2018). Age-related differences in pro-active driving behavior revealed by EEG measures. Front. Hum. Neurosci. 12 (August), 1–10. doi:10.3389/fnhum.2018.00321
Gregory, S. E. A., Wang, H., and Kessler, K. (2022). EEG alpha and theta signatures of socially and non-socially cued working memory in virtual reality. Soc. Cognitive Affect. Neurosci. 17 (6), 531–540. doi:10.1093/scan/nsab123
Guo, Z., Pan, Y., Zhao, G., Cao, S., and Zhang, J. (2018). Detection of driver vigilance level using EEG signals and driving contexts. IEEE Trans. Reliab. 67 (1), 370–380. doi:10.1109/TR.2017.2778754
Hanslmayr, S., Gross, J., Klimesch, W., and Shapiro, K. L. (2011). The role of alpha oscillations in temporal attention. Brain Res. Rev. 67 (1–2), 331–343. doi:10.1016/j.brainresrev.2011.04.002
Haufe, S., Kim, J. W., Kim, I. H., Sonnleitner, A., Schrauf, M., Curio, G., et al. (2014). Electrophysiology-based detection of emergency braking intention in real-world driving. J. Neural Eng. 11 (5), 056011. doi:10.1088/1741-2560/11/5/056011
Haufe, S., Treder, M. S., Gugler, M. F., Sagebaum, M., Curio, G., and Blankertz, B. (2011). EEG potentials predict upcoming emergency brakings during simulated driving. J. Neural Eng. 8 (530), 1–22. doi:10.1088/1741-2560/8/5/056001
Huizeling, E., Wang, H., Holland, C., and Kessler, K. (2020). Age-related changes in attentional refocusing during simulated driving. Brain Sci. 10 (530), 1–22. doi:10.3390/brainsci10080530
Igroup project consortium (2024). igroup presence questionnaire. Available at: http://www.igroup.org/pq/ipq/items.php (Accessed October 12, 2021).
Jensen, O., and Mazaheri, A. (2010). Shaping functional architecture by oscillatory alpha activity: gating by inhibition. Front. Hum. Neurosci. 4 (November), 186–188. doi:10.3389/fnhum.2010.00186
Kemeny, A. (2014) “From driving simulation to virtual reality,” in Proceedings of the 2014 virtual reality international conference.
Kemeny, A., Chardonnet, J.-R., and Colombet, F. (2020) Getting Rid of Cybersickness in virtual reality, augmented reality, and simulators. Cham, Switzerland: Springer Nature Switzerland. doi:10.1007/978-3-030-59342-1
Kemeny, A., George, P., Mérienne, F., and Colombet, F. (2017). New VR navigation techniques to reduce cybersickness. Proc. - Int. Symposium Eletronic Imaging Eng. Real. Virtual Real. 29, 48–53. doi:10.2352/ISSN.2470-1173.2017.3.ERVR-097
Kennedy, R. S., Lane, N. E., Berbaum, K. S., and Lilienthal, M. G. (1993). Simulator sickness questionnaire: an enhanced method for quantifying simulator sickness. Int. J. Aviat. Psychol. 3 (3), 203–220. doi:10.1207/s15327108ijap0303_3
Kim, H. K., Park, J., Choi, Y., and Choe, M. (2018). Virtual reality sickness questionnaire (VRSQ): motion sickness measurement index in a virtual reality environment. Appl. Ergon. 69, 66–73. doi:10.1016/j.apergo.2017.12.016
Kim, H. S., Hwang, Y., Yoon, D., Choi, W., and Park, C. H. (2014). Driver workload characteristics analysis using EEG data from an urban road. IEEE Trans. Intelligent Transp. Syst. 15 (4), 1844–1849. doi:10.1109/TITS.2014.2333750
Klimesch, W. (1999). EEG alpha and theta oscillations reflect cognitive and memory performance: a review and analysis. Brain Res. Rev. 29, 169–195. doi:10.1016/s0165-0173(98)00056-3
Klimesch, W. (2012). Alpha-band oscillations, attention, and controlled access to stored information. Trends Cognitive Sci. 16 (12), 606–617. doi:10.1016/j.tics.2012.10.007
Klimesch, W., Doppelmayr, M., Russegger, H., Pachinger, T., and Schwaiger, J. (1998). Induced alpha band power changes in the human EEG and attention. Neurosci. Lett. 244, 73–76. doi:10.1016/s0304-3940(98)00122-0
Klimesch, W., Sauseng, P., and Hanslmayr, S. (2007). EEG alpha oscillations: the inhibition-timing hypothesis. Brain Res. Rev. 53 (1), 63–88. doi:10.1016/j.brainresrev.2006.06.003
Kothe, C., Shirazi, S. Y., Stenner, T., Medine, D., Boulay, C., Grivich, M. I., et al. (2024). The lab streaming layer for synchronized multimodal recording. bioRxiv, 2024.02.13.580071. doi:10.1101/2024.02.13.580071
Lenormand, D., and Piolino, P. (2022). In search of a naturalistic neuroimaging approach: exploration of general feasibility through the case of VR-fMRI and application in the domain of episodic memory. Neurosci. Biobehav. Rev. 133, 104499. doi:10.1016/j.neubiorev.2021.12.022
Lin, C.-T., Chung, I.-F., Ko, L.-W., Chen, Y.-C., Liang, S.-F., and Duann, J.-R. (2007). EEG-based assessment of driver cognitive responses in a dynamic virtual-reality driving environment. IEEE Trans. Biomed. Eng. 54 (7), 1349–1352. doi:10.1109/TBME.2007.891164
Maris, E., and Oostenveld, R. (2007). Nonparametric statistical testing of EEG- and MEG-data. J. Neurosci. Methods 164 (1), 177–190. doi:10.1016/j.jneumeth.2007.03.024
Menck, J. H. D., Lechte, G., Lembcke, T., Brendel, A. B., and Kolbe, L. M. (2023). “Towards design principles for experimental simulations in virtual reality - learning from driving simulators,” in Proceedings of the Annual Hawaii International Conference on System Sciences, 1303–1312.
Metz, B., Schömig, N., and Krüger, H. P. (2011). Attention during visual secondary tasks in driving: adaptation to the demands of the driving task. Transp. Res. Part F Traffic Psychol. Behav. 14 (5), 369–380. doi:10.1016/j.trf.2011.04.004
Nguyen, T. H., and Chung, W. Y. (2019). Detection of driver braking intention using EEG signals during simulated driving. Sensors Switz. 19 (13), 2863. doi:10.3390/s19132863
Nigbur, R., Ivanova, G., and Stürmer, B. (2011). Theta power as a marker for cognitive interference. Clin. Neurophysiol. 122 (11), 2185–2194. doi:10.1016/j.clinph.2011.03.030
NHTSA (2020). Crash factors in intersection-related crashes: an on-scene perspective (No. DOT HS 811 366). Available at: https://crashstats.nhtsa.dot.gov/Api/Public/ViewPublication/811366.
Oostenveld, R., Fries, P., Maris, E., and Schoffelen, J. M. (2011). FieldTrip: open source software for advanced analysis of MEG, EEG, and invasive electrophysiological data. Comput. Intell. Neurosci. 2011, 1–9. doi:10.1155/2011/156869
Owsley, C., Stalvey, B., Wells, J., and Sloane, M. E. (1999). Older drivers and cataract: driving habits and crash risk. Journals Gerontology - Ser. A Biol. Sci. Med. Sci. 54 (4), 203–211. doi:10.1093/gerona/54.4.M203
Peng, K., Moussavi, Z., Karunakaran, K. D., Borsook, D., Lesage, F., and Nguyen, D. K. (2024). iVR-fNIRS: studying brain functions in a fully immersive virtual environment. Neurophotonics 11 (02), 020601. doi:10.1117/1.nph.11.2.020601
Perrin, F., Pernier, J., Bertrand, O., and Echallier, J. (1989). Spherical splines for scalp potential and current density mapping. Electroencephalogr. Clin. Neurophysiology 72, 184–187. doi:10.1016/0013-4694(89)90180-6
Peylo, C., Hilla, Y., and Sauseng, P. (2021). Cause or consequence? Alpha oscillations in visuospatial attention. Trends Neurosci. 44 (9), 705–713. doi:10.1016/j.tins.2021.05.004
Pion-Tonachini, L., Kreutz-Delgado, K., and Makeig, S. (2019). ICLabel: an automated electroencephalographic independent component classifier, dataset, and website. NeuroImage 198 (January), 181–197. doi:10.1016/j.neuroimage.2019.05.026
Regenbrecht, H., and Schubert, T. (2002). Real and illusory interactions enhance presence in virtual environments. Presence Teleoperators Virtual Environ. 11 (4), 425–434. doi:10.1162/105474602760204318
Ringhand, M., Siebke, C., Bäumler, M., and Petzoldt, T. (2022). Approaching intersections: gaze behavior of drivers depending on traffic, intersection type, driving maneuver, and secondary task involvement. Transp. Res. Part F Traffic Psychol. Behav. 91, 116–135. doi:10.1016/j.trf.2022.09.010
Roberts, G., Holmes, N., Alexander, N., Boto, E., Leggett, J., Hill, R. M., et al. (2019). Towards OPM-MEG in a virtual reality environment. NeuroImage 199, 408–417. doi:10.1016/j.neuroimage.2019.06.010
Sauseng, P., Klimesch, W., Gerloff, C., and Hummel, F. (2009). Spontaneous locally restricted EEG alpha activity determines cortical excitability in the motor cortex. Neuropsychologia 47 (1), 284–288. doi:10.1016/j.neuropsychologia.2008.07.021
Schubert, T., Friedmann, F., and Regenbrecht, H. (2001). The experience of presence: factor analytic insights. Presence 10 (3), 266–281. doi:10.1162/105474601300343603
Sevinc, V., and Berkman, M. I. (2020). Psychometric evaluation of Simulator Sickness Questionnaire and its variants as a measure of cybersickness in consumer virtual environments. Appl. Ergon. 82, 102958. doi:10.1016/j.apergo.2019.102958
Sharma, G., Kaushal, Y., Chandra, S., Singh, V., Mittal, A. P., and Dutt, V. (2017). Influence of landmarks on wayfinding and brain connectivity in immersive virtual reality environment. Front. Psychol. 8 (JUL), 1–12. doi:10.3389/fpsyg.2017.01220
Shechtman, O., Classen, S., Stephens, B., Bendixen, R., Belchior, P., Sandhu, M., et al. (2007). The impact of intersection design on simulated driving performance of young and senior adults. Traffic Inj. Prev. 8 (1), 78–86. doi:10.1080/15389580600994321
Simon, M. C., Hermitte, T., and Page, Y. (2023). “Intersection road accident causation: a European view,” in 21st international technical conference on the enhanced safety of vehicles, 09–0370. Available at: https://pdfs.semanticscholar.org/d78b/7d378927efd497f68883e1bf1e85502f5928.pdf.
Suwarno, Di.C. D., Wijayanto, T., and Trapsilawati, F. (2019). “Cybersickness evaluation while using driving simulator in a head-mounted display environment,” in Proceedings of the 2019 International Conference on Mechatronics, Robotics and Systems Engineering, MoRSE 2019, 103–106. doi:10.1109/MoRSE48060.2019.8998692
Tian, N., Lopes, P., and Boulic, R. (2022) A review of cybersickness in head-mounted displays: raising attention to individual susceptibility, Virtual Reality. London: Springer. doi:10.1007/s10055-022-00638-2
Unity Technologies (2024) “Unity Technologies”. San Francisco: Unity. Available at: https://unity.com/.
Wascher, E., Alyan, E., Karthaus, M., Getzmann, S., Arnau, S., and Reiser, J. E. (2023). Tracking drivers’ minds: continuous evaluation of mental load and cognitive processing in a realistic driving simulator scenario by means of the EEG. Heliyon 9 (7), e17904. doi:10.1016/j.heliyon.2023.e17904
Wascher, E., Arnau, S., Gutberlet, I., Karthaus, M., and Getzmann, S. (2018). Evaluating Pro- and Re-active driving behavior by means of the EEG. Front. Hum. Neurosci. 12 (May), 1–8. doi:10.3389/fnhum.2018.00205
Weber, D., Hertweck, S., Alwanni, H., Fiederer, L. D. J., Wang, X., Unruh, F., et al. (2021). A structured approach to test the signal quality of electroencephalography measurements during use of head-mounted displays for virtual reality applications. Front. Neurosci. 15 (November), 733673. doi:10.3389/fnins.2021.733673
Weidner, F., Hoesch, A., Poeschl, S., and Broll, W. (2017) ‘Comparing VR and non-VR driving simulations: an experimental user study. 2017 IEEE Virtual Reality (VR) 281–282. doi:10.1109/VR.2017.7892286
Werneke, J., and Vollrath, M. (2012). What does the driver look at? the influence of intersection characteristics on attention allocation and driving behavior. Accid. Analysis Prev. 45, 610–619. doi:10.1016/j.aap.2011.09.048
Werneke, J., and Vollrath, M. (2014). How do environmental characteristics at intersections change in their relevance for drivers before entering an intersection: analysis of drivers’ gaze and driving behavior in a driving simulator study. Cognition, Technol. Work 16 (2), 157–169. doi:10.1007/s10111-013-0254-y
Yao, D. (2001). A method to standardize a reference of scalp EEG recordings to a point at infinity. Physiol. Meas. 22 (4), 693–711. doi:10.1088/0967-3334/22/4/305
Zavala, B., Jang, A., Trotta, M., Lungu, C. I., Brown, P., and Zaghloul, K. A. (2018). Cognitive control involves theta power within trials and beta power across trials in the prefrontal-subthalamic network. Brain [Preprint] 141, 3361–3376. doi:10.1093/brain/awy266
Keywords: virtual reality, driving simulation, intersections, attentional processing, EEG, cognitive control
Citation: Senftleben U and Kessler K (2024) EEG oscillatory signatures of increased cognitive control at intersections: a virtual reality driving simulation. Front. Virtual Real. 5:1433829. doi: 10.3389/frvir.2024.1433829
Received: 16 May 2024; Accepted: 15 November 2024;
Published: 29 November 2024.
Edited by:
Jorge Peña, University of California, Davis, United StatesReviewed by:
Hakim Si-Mohammed, Université de Lille, FranceCédric Cannard, Institute of Noetic Sciences, United States
Copyright © 2024 Senftleben and Kessler. This is an open-access article distributed under the terms of the Creative Commons Attribution License (CC BY). The use, distribution or reproduction in other forums is permitted, provided the original author(s) and the copyright owner(s) are credited and that the original publication in this journal is cited, in accordance with accepted academic practice. No use, distribution or reproduction is permitted which does not comply with these terms.
*Correspondence: Ulrike Senftleben, dWxyaWtlLnNlbmZ0bGViZW5AdHUtZHJlc2Rlbi5kZQ==
†ORCID: Ulrike Senftleben, orcid.org/0000-0002-8403-0359; Klaus Kessler, orcid.org/0000-0001-7307-9539